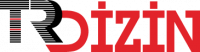
Toplantı tutanaklarının analizi ile bir karar destek sistemi
Yıl: 2018 Cilt: 22 Sayı: 2 Sayfa Aralığı: 257 - 268 Metin Dili: Türkçe DOI: 10.16984/saufenbilder.341742 İndeks Tarihi: 27-11-2019
Toplantı tutanaklarının analizi ile bir karar destek sistemi
Öz: Günümüzde veri madenciliği firmalar açısından çok önemli hale gelmiştir. Firmalar sektörde rekabetavantajı sağlayabilmek için veri madenciliği tekniklerini kullanarak büyük veriden işlerine yarayacak, dahaönceden keşfedilmemiş, kullanılabilir örüntüler elde eder. Gelişen haberleşme teknolojileri sonucufirmalarda biriken veri yığınları, firmalar için hayati önem taşıyan bilgileri içinde barındırır. Karar vericiler,klasik tekniklerle bu verilerden çıkarımlarda bulunurken, önemli bilgileri gözden kaçırırlar. Veriyi doğruyönetemeyen firmalar ise işlerine yaramayan veri yığınlarında kaybolur. Bir işletmeye ait sayısalplatformdaki bu verilerin %80’i metin formundadır. Ancak yapısal olmayan verileri de içeren büyük veriklasik istatistiksel tekniklerle analiz edilen veriler kadar kolay işlenemez. Doğal dil işleme tekniklerindenfaydalanılması gerekmektedir. Böylece, soyut ve yığın yapısal olmayan bilgiler, sayısal somut ifadeleredönüştürülebilmektedir. Bu araştırma, Kayseri’de bir imalat fabrikasında yapılan üst düzey toplantılarınmetin formatındaki tutanaklarını analiz ederek bilgi çıkarımı gerçekleştirmektedir. Yöneticilerin verdiğistratejik kararlarda önemli toplantı sonuçları çok etkilidir. Araştırmanın en genel amacı toplantılarınkalitesini artırmaktır. Araştırmada, toplantı tutanaklarından kelime çıkarımı yapılarak, toplantılara ait genelkonu başlıkları metin madenciliği ile elde edilecektir. Yöneticiler çeşitli madenleme teknikleriylegruplanmış konu başlıklarına göre değerlendirme yaparak sonraki toplantıların kalitesini artırarak zamankazanabilir.
Anahtar Kelime: Konular:
A decision support system by analysis of the meeting reports
Öz: Recently, data mining has become crucial for firms. Using data mining, Firms, in order to have comparative advantage in industry / sector / market, obtain patterns that they can utilize and that have not been discovered before. The data accumulated as a result of the advanced communication channels within firms contain crucial information. Decision makers’ undersees important information while they use classical techniques for data analysis. Firms that cannot manage data accurately get lost in piles of data that would not be useful for them. 80% of the data in the quantitative platform belonging to a firm is in text format. However, large data containing non-structural data cannot be analyzed as easily as the data analyzed by using classical statistical techniques. Natural language analysis techniques should be used. In this way, abstract and non-structural data can be converted into concrete and quantitative statements. In this analysis, information is inferred by the analysis of transcripts—in text format—of meetings among senior managers at a manufacturing company in Kayseri. Outcomes of the important meetings are very crucial in the decisions the directors take. The main goal of the study is to increase the efficiency of the meetings. In this research, the general themes of the meetings are found out by word inference from the meeting transcripts. Directors can have better time-management by increasing the quality of the future meetings by conducting evaluations according to the topics categorized by the data mining techniques.
Anahtar Kelime: Konular:
Belge Türü: Makale Makale Türü: Araştırma Makalesi Erişim Türü: Erişime Açık
- D. Thorleuchter, D. V. Den Poel ve A. Prinzie, (2010). “Mining ideas from textual information,” Expert Systems with Applications, vol. 37, pp. 7182–7188.
- S. Rose, D. Engel, N. Cramer ve W. Cowley, (2010), “Automatic keyword extraction from individual documents”, in: M.W. BERRY and J. KOGAN (Ed.), TM: Applications and Theory, Wiley, p.3-19.
- S. Kumar, A. Nassehi, S. T. Newman ve M. K. Tiwari, M. K., (2007), “Process control in CNC manufacturing for discrete components: A STEP-NC compliant framework”, Robotics and Computer Integrated Manufacturing, 23, pp.667-676.
- D.C. Li ve C.W. Yeh, (2008), “A nonparametric learning algorithm for small manufacturing data sets”, Expert Systems with Applications, 34, pp.391– 398.
- C. Çı̇flı̇klı̇ ve E. Kahya-Özyı̇rmı̇dokuz, (2010), “Implementing A Data Mining Solution For Enhancing Carpet Manufacturing Productivity”, Knowledge Based Systems, 23 (8) Pp.783-788.
- S. Gebus ve K. Leiviska, (2009), “Knowledge acquisition for decision support systems on an electronic assembly line”, Expert Systems with Applications, 36 (1), pp. 93-101.
- A. Kusiak ve M. Smith, (2007), “Data mining in design of products and production systems”, Annual Reviews in Control, 31, pp.147–156.
- P. Kang, H. Lee, S. Cho, D. Kim, J. Park, J. K., Park ve S. Doh, (2009), “A virtual metrology system for semiconductor manufacturing”, Expert Systems with Applications, 36, pp.12554–12561.
- O. Durán, N. Rodriguez ve L.A. Consalter, (2010), “Collaborative particle swarm optimization with a data mining technique for manufacturing cell design”, Expert Systems with Applications, 37, pp.1563– 1567.
- S. H. Liao, P. H. Chu ve P. Y. Hsiao, (2012), “Data mining techniques and applications – A decade review from 2000 to 2011”, Expert Systems with Applications, 39, pp.11303–11311.
- J.A., Harding, M. Shahbaz, Srinivas ve A. Kusiak, (2006), “Data mining in manufacturing: A review”, Journal of Manufacturing Science and Engineering, Manufacturing Engineering Division of Asme 128, pp. 969- 976.
- K. Wang, (2007), “Applying data mining to manufacturing: the nature and implications”, Journal of Intelligent Manufacturing, 18 pp.487–495.
- C.Çı̇flı̇klı̇ ve E. Kahya-Özyı̇rmı̇dokuz, (2012), "Enhancing Product Quality Of a Process", Industrial Management and Data Systems, 112, pp.1181-1200.
- A. Ittoo ve G. Bouma, (2013), “Term extraction from sparse, ungrammatical domain-specific documents”, Expert Systems with Applications, 40, pp.2530– 2540.
- D. Thorleuchter ve D. Van Den Poel, (2014), “Semantic compared cross impact analysis”, Expert Systems with Applications 41, pp. 3477– 3483.
- E. Kahya-Özyı̇Rmı̇Dokuz, (2014), “Analyzing Social Network Unstructured Data”, Information Development, doi: 10.1177/0266666914528523.
- E. Kahya Özyı̇rmı̇dokuz ve M. H. Özyı̇rmı̇dokuz, (2014) “Analyzing Customer Complaints : A Web Text Mining Application", in International Conference on Education and Social Sciences (INTCESS14), Ferit USLU (Ed.), İstanbul, 3-5 February 2014, pp.734-743.
- Y. Liu, W. F. Lu ve H. T. Loh, (2006), “A Framework of information and knowledge management for product design and development: A text mining approach”, Information Control Problems in Manufacturing IFAC 12th, in INCOM 2006, Information control problems in manufacturing, pp. 635-640.
- A. Negahban ve J. S. Smith, (2014), “Simulation for manufacturing system design and operation: Literature review and analysis”, Journal of Manufacturing Systems, 33 (2), pp.241–261.
- C. W. Chang, C. T. Lin ve L.Q. Wang, (2009). “Mining the text information to optimizing the customer relationship management,” Expert Systems with Applications, vol. 36, pp. 1433–1443.
- M.Gamon, (2004). “Sentiment classification on customer feedback data: Noisy data, large feature vectors, and the role of linguistic analysis,” in Proc. the 20th international conference on Computational Linguistics, pp. 841-847, PA, USA: Association for Computational Linguistics Stroudsburg.
- M.Gamon, A. Aue, S.Corston-Oliver ve E. Ringger, (2005). “Pulse: Mining customer opinions from free text,” LNCS, pp. 121- 132, Heidelberg, Berlin: Springer-Verlag.
- A. R. Ittoo, Y. R. Zhang ve J. Jiao, (2006). “A TM based recommendation system for customer decision making in online product customization,” in Proc. International Conference on Management of innovation and technology, vol. 1, pp. 473-477, Singapore, China: IEEE.
- K.Coussement ve D. V.Den Poel, (2008). “Improving customer complaint management by automatic email classification using linguistic style features as predictors,” Decision Support Systems, vol. 44, pp. 870–882.
- S.S.Weng ve C.K. Liu, (2004). “Using text classification and multiple concepts to answer e-mails,” Expert Systems with Applications, vol. 26, pp. 529–543.
- Ö. Özyurt ve C. Köse, (2010). “Chatmining: Automatically determination of chat conversations’ topic in Turkish text based chat mediums,” Expert Systems with Applications, vol. 37, pp. 8705–8710.
- S.Tsai ve A. T. Kwee, (2011). “Database optimization for novelty mining of business blogs,” Expert Systems with Applications, vol. 38, pp. 11040–11047.
- R. D.Gopal, J. R.Marsden ve J. Vanthienen, (2011). “Information mining - Reflections on recent advancements and the road ahead in data, text, and media mining,” Decision Support Systems, vol. 51, pp. 727–731.
- A.Sunikka ve J. Bragge, (2012). “Applying text-mining to personalization and customization research literature – Who, what and where?” Expert Systems with Applications, vol. 39, pp. 10049–10058.
- H. Onishi ve P. Manchanda, (2012). “Marketing activity, blogging and sales,” Intern. J. of Research in Marketing, vol. 29, pp. 221–234.
- M. G.Armentano, D. Godoy ve A. A. Amandi, (2013). “Followee recommendation based on text analysis of micro-blogging activity,” Information Systems, vol. 38, pp. 1116-1127.
- D.Thorleuchter ve D.V. DenPoel, (2012). “Predictinge-commercecompany success by mining the text of its publicly-accessible website,” Expert Systems with Applications, vol. 39, pp. 13026–13034.
- D.Thorleuchter, D. V. Den Poel ve A. Prinzie, (2012). “Analyzing existing customers’ websites to improve the customer acquisition process as well as the profitability prediction in B-to-B marketing,” Expert Systems with Applications, vol. 39, pp. 2597–2605.
- N.Ur-Rahman ve J. A. Harding, (2012). “Textual DM for industrial knowledge management and text classification: A business oriented approach,” Expert Systems with Applications, vol. 39, pp. 4729–4739.
- Z.G. Hao, (2012). “A new text clustering method based on KSEP,” Journal of Software, vol. 7, no. 6, pp. 1421-1425.
- W. He, S. Zha ve L. Li, (2013). “Social media competitive analysis and TM: A case study in the pizza industry,” International Journal of Information Management, vol.33, no.3, pp. 464–472.
- E. Kahya Özyirmidokuz, (2014). “Analyzing unstructured facebook social network data through web TM: A study of online shopping firms in Turkey,” Information Development, pp. 1–12, 2014.
- F. V.Ordenes, B. Theodoulidis, J. Burton, T.Gruber ve M. Zaki, (2014). “Analyzing customer experience feedback using TM: A linguistics-based approach,” Journal of Service Research, pp. 1-18.
- V. Stray, I.K. Dag ve T. D. Sjøberg, (2016). “The daily stand-up meeting: A grounded theory study”, The Journal of Systems and Software 114, 101–124.
- S. H. Kim, S. Park, M. R. Sun ve J. H. Lee, (2016). “A Study of Smart Beacon-based Meeting, Incentive Trip, Convention, Exhibition and Event (MICE) Services Using Big Data”, Procedia Computer Science 91, 761 – 768.
- S. F. Hussain ve A. Suryani, (2015). “On retrieving intelligently plagiarized documents using semantic similarity”, Engineering Applications of Artificial Intelligence 45, 246–258.
- H. Zhang ve T.W.S. Chow, (2012). “A multi-level matching method with hybrid similarity for document retrieval”, Expert Systems with Applications 39, 2710–2719.
- A. Hotho, A. Nurnberger ve G. Paaß, (2005). “A Brief Survey of Text Mining. LDV Forum – GLDV”, Journal for Computational Linguistics and Language Technology 20(1), 19-62.
- S. Tuffery, (2011), DM and Statistics for Decision Making. Wiley
- D. T. Larose, (2005), Discovering Knowledge in Data: An Introduction to Data Mining, USA: Wiley.
- S. Sumathi ve S.N. Sivanandam, (2006). Introduction to DM and its Applications, Verlag Berlin Heidelberg: Springer.
- Hoffmann and Klinkenberg, 2014 RapidMiner: Data Mining Use Cases and Business Analytics Applications, Markus Hofmann, Ralf Klinkenberg, CRC Press, Taylor and Francis
- G.S. Ingersoll, T.S. Morton ve A.L. Farris, (2013) Taming Text: How to Find, Organize, and Manipulate it, Liddy, L. (Ed.). NY: Manning Publications and Co
- J. Ghosh ve A. Strehl, (2006). “Similarity- Based Text Clustering: A Comparative Study, in: Grouping Multidimensional Data: Recent Advances in Clustering”, Jacob Kogan, Charles Nicholas, Marc Teboulle (Eds.), Springer-Verlag Berlin Heidelberg, pp. 73-98.
APA | GÜRBÜZ F, Kahya Ozyirmidokuz E (2018). Toplantı tutanaklarının analizi ile bir karar destek sistemi. , 257 - 268. 10.16984/saufenbilder.341742 |
Chicago | GÜRBÜZ FEYZA,Kahya Ozyirmidokuz Esra Toplantı tutanaklarının analizi ile bir karar destek sistemi. (2018): 257 - 268. 10.16984/saufenbilder.341742 |
MLA | GÜRBÜZ FEYZA,Kahya Ozyirmidokuz Esra Toplantı tutanaklarının analizi ile bir karar destek sistemi. , 2018, ss.257 - 268. 10.16984/saufenbilder.341742 |
AMA | GÜRBÜZ F,Kahya Ozyirmidokuz E Toplantı tutanaklarının analizi ile bir karar destek sistemi. . 2018; 257 - 268. 10.16984/saufenbilder.341742 |
Vancouver | GÜRBÜZ F,Kahya Ozyirmidokuz E Toplantı tutanaklarının analizi ile bir karar destek sistemi. . 2018; 257 - 268. 10.16984/saufenbilder.341742 |
IEEE | GÜRBÜZ F,Kahya Ozyirmidokuz E "Toplantı tutanaklarının analizi ile bir karar destek sistemi." , ss.257 - 268, 2018. 10.16984/saufenbilder.341742 |
ISNAD | GÜRBÜZ, FEYZA - Kahya Ozyirmidokuz, Esra. "Toplantı tutanaklarının analizi ile bir karar destek sistemi". (2018), 257-268. https://doi.org/10.16984/saufenbilder.341742 |
APA | GÜRBÜZ F, Kahya Ozyirmidokuz E (2018). Toplantı tutanaklarının analizi ile bir karar destek sistemi. Sakarya Üniversitesi Fen Bilimleri Enstitüsü Dergisi, 22(2), 257 - 268. 10.16984/saufenbilder.341742 |
Chicago | GÜRBÜZ FEYZA,Kahya Ozyirmidokuz Esra Toplantı tutanaklarının analizi ile bir karar destek sistemi. Sakarya Üniversitesi Fen Bilimleri Enstitüsü Dergisi 22, no.2 (2018): 257 - 268. 10.16984/saufenbilder.341742 |
MLA | GÜRBÜZ FEYZA,Kahya Ozyirmidokuz Esra Toplantı tutanaklarının analizi ile bir karar destek sistemi. Sakarya Üniversitesi Fen Bilimleri Enstitüsü Dergisi, vol.22, no.2, 2018, ss.257 - 268. 10.16984/saufenbilder.341742 |
AMA | GÜRBÜZ F,Kahya Ozyirmidokuz E Toplantı tutanaklarının analizi ile bir karar destek sistemi. Sakarya Üniversitesi Fen Bilimleri Enstitüsü Dergisi. 2018; 22(2): 257 - 268. 10.16984/saufenbilder.341742 |
Vancouver | GÜRBÜZ F,Kahya Ozyirmidokuz E Toplantı tutanaklarının analizi ile bir karar destek sistemi. Sakarya Üniversitesi Fen Bilimleri Enstitüsü Dergisi. 2018; 22(2): 257 - 268. 10.16984/saufenbilder.341742 |
IEEE | GÜRBÜZ F,Kahya Ozyirmidokuz E "Toplantı tutanaklarının analizi ile bir karar destek sistemi." Sakarya Üniversitesi Fen Bilimleri Enstitüsü Dergisi, 22, ss.257 - 268, 2018. 10.16984/saufenbilder.341742 |
ISNAD | GÜRBÜZ, FEYZA - Kahya Ozyirmidokuz, Esra. "Toplantı tutanaklarının analizi ile bir karar destek sistemi". Sakarya Üniversitesi Fen Bilimleri Enstitüsü Dergisi 22/2 (2018), 257-268. https://doi.org/10.16984/saufenbilder.341742 |