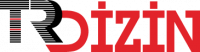
Multiobjective PID controller design for active suspension system: scalarization approach
Yıl: 2018 Cilt: 8 Sayı: 2 Sayfa Aralığı: 183 - 194 Metin Dili: İngilizce DOI: 10.11121/ijocta.01.2018.00399 İndeks Tarihi: 03-12-2019
Multiobjective PID controller design for active suspension system: scalarization approach
Öz: In this study, the PID tuning method (controller design scheme) is proposed fora linear quarter model of active suspension system installed on the vehicles.The PID tuning scheme is considered as a multiobjective problem which issolved by converting this multiobjective problem into single objective problemwith the aid of scalarization approaches. In the study, three different scalarizationapproaches are used and compared to each other. These approachesare called linear scalarization (weighted sum), epsilon-constraint and Bensonsmethods. The objectives of multiobjective optimization are selected from thetime-domain properties of the transient response of the system which are overshoot,rise time, peak time and error (in total there are four objectives). Theaim of each objective is to minimize the corresponding property of the timeresponse of the system. First, these four objective is applied to the scalarizationfunctions and then single objective problem is obtained. Finally, thesesingle objective problems are solved with the aid of heuristic optimization algorithms.For this purpose, four optimization algorithms are selected, which arecalled Particle Swarm Optimization, Differential Evolution, Firefly, and CulturalAlgorithms. In total, twelve implementations are evaluated with the samenumber of iterations. In this study, the aim is to compare the scalarizationapproaches and optimization algorithm on active suspension control problem.The performance of the corresponding cases (implementations) are numericallyand graphically demonstrated on transient responses of the system.
Anahtar Kelime: Konular:
Belge Türü: Makale Makale Türü: Araştırma Makalesi Erişim Türü: Erişime Açık
- Astrom K.J., and Hagglund T., The future of PID control, Control Engineering Practice, 9, 11, 1163-1175 (2001).
- Arruda L.V.R, Swiech M.C.S, Delgado M.R.B, and Neves-Jr F., PID control of MIMO process based on rank niching genetic algorithm, Applied Intelligence, 29, 3, 290-305 (2008).
- Neath M.J., Swain A.K., Madawala U.K., and Thrimawithana D.J., An Optimal PID Controller for a Bidirectional Inductive Power Transfer System Using Multiobjective Genetic Algorithm, IEEE Transactions on Power Electronics, 29, 3, 1523-1531 (2014).
- Lin C.-L., Jan H.-Y., and Shieh N.-C, GA-based multiobjective PID control for a linear brushless DC motor, IEEE/ASME Transactions on Mechatronics, 8, 1, 56-65 (2003).
- Herreros A., Baeyens E., and Pern J.R., Design of PID-type controllers using multiobjective genetic algorithms, ISA Transactions, 41, 4, 457-472 (2002).
- Reynoso-Meza G., Garcia-Nieto S., Sanchis J., and Blasco F.X., Controller Tuning by Means of MultiObjective Optimization Algorithms: A Global Tuning Framework, IEEE Transactions on Control Systems Technology, 21, 2, 445-458 (2013).
- Reynoso-Meza G., Sanchis J., Blasco X., and Herrero J.M., Multiobjective evolutionary algorithms for multivariable PI controller design, Expert Systems with Applications, 39, 9, 7895-7907 (2012).
- Zhaoa S.-Z, Willjuice Iruthayarajanb M., Baskarc S., and Suganthan P.N., Multi-objective robust PID controller tuning using two lbests multi-objective particle swarm optimization, Information Sciences, 181, 16, 3323-3335 (2011).
- C.M. Seaman, A.A. Desrochers, and G.F. List, Multiobjective optimization of a plastic injection molding process, IEEE Transactions on Control Systems Technology, 2, 3, 157-168 (1994).
- Gambier A., MPC and PID control based on MultiObjective Optimization, American Control Conference, 4727-4732 (2008).
- Naidua K., Mokhlisa H., and Bakar A.H.A., Multiobjective optimization using weighted sum Artificial Bee Colony algorithm for Load Frequency Control, International Journal of Electrical Power and Energy Systems, 55, 657-667 (2014).
- Hung M.-H., Shu L.-S., Ho S.-J., and Hwang S.-F., A Novel Intelligent Multiobjective Simulated Annealing Algorithm for Designing Robust PID Controllers, IEEE Transactions on Systems, Man, and Cybernetics - Part A: Systems and Humans, 38, 2, 319-330 (2008).
- Tseng C.-S., and Chen B.-S., Multiobjective PID control design in uncertain robotic systems using neural network elimination scheme, IEEE Transactions on Systems, Man, and Cybernetics - Part A: Systems and Humans, 31, 6, 632-644 (2001).
- Takahashi R.H.C., Peres P.L.D., and Ferreira P.A.V., Multiobjective H2/H∞ guaranteed cost PID design , IEEE Control Systems, 17, 5, 37-47 (2002).
- Tang K.S., Man K.F., Chen G., and Kwong S., An optimal fuzzy PID controller, IEEE Transactions on Industrial Electronics, 48, 4, 757-765 (2001).
- Liua G.P., and Daley S., Optimal-tuning PID control for industrial systems, Control Engineering Practice, 9, 11, 1185-1194 (2001).
- Ayalaa H.V.H., and Coelho L.S., Tuning of PID controller based on a multiobjective genetic algorithm applied to a robotic manipulator, Expert Systems with Applications, 49, 10, 8968-8974 (2012).
- Panda S., Multi-objective PID controller tuning for a FACTS-based damping stabilizer using Nondominated Sorting Genetic Algorithm-II, International Journal of Electrical Power and Energy Systems, 33, 7, 12961308 (2011).
- Bourmistrova, A., Storey, I., and Subic A., Multiobjective optimisation of active and semi-active suspension systems with application of evolutionary algorithm, International Conference on Modelling and Simulation, 1217-1223 (2005).
- Xue X.D., Cheng K.W.E., and Xu C.D., Optimization of spring stiffness in automotive and rail active suspension systems, International Conference on Electrical Systems for Aircraft, Railway, Ship Propulsion and Road Vehicles, International Transportation Electrification, 1-6 (2016).
- Aldair A.A., Abdalla T.Y., and Alsaedee E.B., Design of PID controller using ABC for full vehicle nonlinear active suspension system with passenger seat, International Journal of Computer Applications, 167, 18-27 (2017).
- Rastegar N., and Khorram E., A combined scalarizing method for multiobjective programming problems, European Journal of Operational Research, 236, 1, 229237 (2014).
- Haimes Y., Ladson L., and Wismer D., On a bi criterion formulation of the problems of integrated system identification and system optimization, IEEE Transactions on System, Man, and Cybernetics, 1, 296-297 (1971).
- Benson H.P., Existence of efficient solutions for vector maximization problems, Journal of Optimization Theory and Applications, 26, 4, 569-580 (1978).
- Benson H.P., and Morin T.L., The vector maximization problem: Proper Efficiency and Stability, SIAM Journal of Applied Mathematics, 1-18 (1974).
- Soleimani-Damaneh M., and Zamani M., On Bensons scalarization in multiobjective optimization, Optimization Letters, Early Access, 1-6 (2016).
- Ehrgott M., Approximation algorithms for combinatorial multicriteria optimization problems, International Transactions in Operational Research, 7, 531 (2000).
- Deb K., Multi-Objective Optimization Using Evolutionary Algorithms, Publisher : Chichester, UK : Wiley (2002).
- Kennedy J., and Eberhart R.C., Particle Swarm Optimization, Proceedings of IEEE International Conference on Neural Networks, 1942-1948 (1995).
- Storn R., and Price K., Differential Evolution - a simple and efficient adaptive scheme for global optimization over continuous space, International Computer Science Institute, Technical Report, TR-95-012 (1995).
- Yang X.-S., Firefly Algorithm, Nature Inspired Metaheuristic Algorithms,79-90 (2008).
- Fister I., Yang X.-S., and Brest J., A Comprehensive Review of Firefly Algorithm, Swarm and Evolut,onary Computation, 13, 34-46 (2013).
- Yang X.-S., Firefly Algorithm, Stochastic Test Functions and Design Optimization, International Journal of Bio-Inspired Computation, 2, 78-84 (2010).
- Yang X.-S., Firefly Algorithms for Multimodal Optimization, Lecture Notes in Computer Science, 5792 LNCS, 169-178 (2009).
- Yang X.-S., Hosseini S., and Gandomi A.H., Firefly Algorithm for Solving Non-convex Economic Dispatch Problems with Valve Loading Effect, Applied Soft Computing Journal, 12, 1180-1186 (2012).
- Reynolds R.G., An Adaptive Computer Model of the Evolution of Agriculture for Hunter-Gatherers in the Valley of Oaxaca Mexico, PH.D. Thesis, Department of Computer Science, University of Michigan, (1976).
- Reynolds R.G., and Peng B. Cultural Algorithms: Modeling of How Cultures Learn to Solve Problems, International Conference on Tools with Artificial Intelligence, 1-7 (2004).
- Rychtyckyj, N., Using Cultural Algorithm in Industry, IEEE Swarm Intelligence Symposium, 1-8 (2003).
- Chung C., and Reynolds R.G., CAEP: An Evolutionbased tool for Real-Valued Function Optimization using Cultural Algorithms, International Journal on Artificial Intelligence Tools, 7, 239-291 (1998).
- Jin X., and Reynolds R.G., Using Knowledge-based Evolutionary Computation to Solve Nonlinear Constraint Optimization Problems: A Cultural Algorithm Approach, Congress on Evolutionary Computation, 1672-1678 (1999).
- Reynolds R.G., and Saleem S. The Impact of Environmental Dynamics on Cultural Emergence, Oxford University Press, 1-10 (2003).
APA | ALTINÖZ O (2018). Multiobjective PID controller design for active suspension system: scalarization approach. , 183 - 194. 10.11121/ijocta.01.2018.00399 |
Chicago | ALTINÖZ O. Tolga Multiobjective PID controller design for active suspension system: scalarization approach. (2018): 183 - 194. 10.11121/ijocta.01.2018.00399 |
MLA | ALTINÖZ O. Tolga Multiobjective PID controller design for active suspension system: scalarization approach. , 2018, ss.183 - 194. 10.11121/ijocta.01.2018.00399 |
AMA | ALTINÖZ O Multiobjective PID controller design for active suspension system: scalarization approach. . 2018; 183 - 194. 10.11121/ijocta.01.2018.00399 |
Vancouver | ALTINÖZ O Multiobjective PID controller design for active suspension system: scalarization approach. . 2018; 183 - 194. 10.11121/ijocta.01.2018.00399 |
IEEE | ALTINÖZ O "Multiobjective PID controller design for active suspension system: scalarization approach." , ss.183 - 194, 2018. 10.11121/ijocta.01.2018.00399 |
ISNAD | ALTINÖZ, O. Tolga. "Multiobjective PID controller design for active suspension system: scalarization approach". (2018), 183-194. https://doi.org/10.11121/ijocta.01.2018.00399 |
APA | ALTINÖZ O (2018). Multiobjective PID controller design for active suspension system: scalarization approach. An International Journal of Optimization and Control: Theories & Applications (IJOCTA), 8(2), 183 - 194. 10.11121/ijocta.01.2018.00399 |
Chicago | ALTINÖZ O. Tolga Multiobjective PID controller design for active suspension system: scalarization approach. An International Journal of Optimization and Control: Theories & Applications (IJOCTA) 8, no.2 (2018): 183 - 194. 10.11121/ijocta.01.2018.00399 |
MLA | ALTINÖZ O. Tolga Multiobjective PID controller design for active suspension system: scalarization approach. An International Journal of Optimization and Control: Theories & Applications (IJOCTA), vol.8, no.2, 2018, ss.183 - 194. 10.11121/ijocta.01.2018.00399 |
AMA | ALTINÖZ O Multiobjective PID controller design for active suspension system: scalarization approach. An International Journal of Optimization and Control: Theories & Applications (IJOCTA). 2018; 8(2): 183 - 194. 10.11121/ijocta.01.2018.00399 |
Vancouver | ALTINÖZ O Multiobjective PID controller design for active suspension system: scalarization approach. An International Journal of Optimization and Control: Theories & Applications (IJOCTA). 2018; 8(2): 183 - 194. 10.11121/ijocta.01.2018.00399 |
IEEE | ALTINÖZ O "Multiobjective PID controller design for active suspension system: scalarization approach." An International Journal of Optimization and Control: Theories & Applications (IJOCTA), 8, ss.183 - 194, 2018. 10.11121/ijocta.01.2018.00399 |
ISNAD | ALTINÖZ, O. Tolga. "Multiobjective PID controller design for active suspension system: scalarization approach". An International Journal of Optimization and Control: Theories & Applications (IJOCTA) 8/2 (2018), 183-194. https://doi.org/10.11121/ijocta.01.2018.00399 |