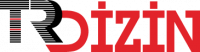
Comparative Performance Analysis of Techniques for Automatic Drug Review Classification
Yıl: 2018 Cilt: 14 Sayı: 4 Sayfa Aralığı: 485 - 490 Metin Dili: İngilizce DOI: 10.18466/cbayarfbe.481096 İndeks Tarihi: 22-01-2020
Comparative Performance Analysis of Techniques for Automatic Drug Review Classification
Öz: This study analyses the effectiveness of six text feature selection methods for automatic classification of drugreviews written in English using two different widely-known classifiers namely Support Vector Machines(SVM) and naïve Bayes (NB). In the study, a recently published public dataset namely Druglib includingdrug reviews in English was utilized in the experiments. For evaluation, Micro-F1 and Macro-F1 successmeasures were used. Also, 3-fold cross-validation is preferred to perform a fair evaluation. The featureselection methods used in the study are Distinguishing Feature Selector (DFS), Information Gain (IG), chi-square (CHI2), Discriminative Features Selection (DFSS), Improved Comprehensive Measurement FeatureSelection (ICMFS), and Relative Discrimination Criterion (RDC). However, experiments were performedusing two settings in which stemming was applied and not applied. Experiments indicated that ICMFS featureselection method is generally superior to the other feature selection methods according to the overall highestMicro-F1 and Macro-F1 scores achieved on drug reviews. While the highest Micro-F1 score was achievedwith the combination of NB classifier and ICMFS feature selection method, the highest Macro-F1 score wasachieved with the combination of NB classifier and DFSS feature selection method. The highest Micro-F1and Macro-F1 scores were achieved for the cases that stemming algorithm was not applied.
Anahtar Kelime: Belge Türü: Makale Makale Türü: Araştırma Makalesi Erişim Türü: Erişime Açık
- Uysal, A. K., Murphey, Y. L. Sentiment classification: Feature selection based approaches versus deep learning, proceedings of 17th IEEE International Conference on Computer and Information Technology (CIT), 2017, pp. 23-30.
- Pang, B., Lee, L. A sentimental education: Sentiment analysis using subjectivity summarization based on minimum cuts, proceedings of the 42nd annual meeting on Association for Computational Linguistics, 2004, pp. 1-8: Association for Computational Linguistics.
- Gan, Q., Ferns, B. H., Yu, Y., Jin, L., A Text Mining and Multidimensional Sentiment Analysis of Online Restaurant Reviews, Journal of Quality Assurance in Hospitality & Tourism, 2017, 18(4), 465-492.
- Gui, L., Zhou, Y., Xu, R., He, Y., Lu, Q., Learning representations from heterogeneous network for sentiment classification of product reviews, Knowledge-Based Systems, 2017, 124, 34-45.
- Gräßer, F., Kallumadi, S., Malberg, H., Zaunseder, S. Aspect-Based Sentiment Analysis of Drug Reviews Applying Cross-Domain and Cross-Data Learning, proceedings of 2018 International Conference on Digital Health, 2018, pp. 121-125: ACM.
- Na, J.-C., Kyaing, W. Y. M., Khoo, C. S. G., Foo, S., Chang, Y.-K., Theng, Y.-L. Sentiment Classification of Drug Reviews Using a Rule-Based Linguistic Approach, proceedings of The Outreach of Digital Libraries: A Globalized Resource Network, Berlin, Heidelberg, 2012, pp. 189-198: Springer Berlin Heidelberg.
- Cavalcanti, D., Prudencio, R. Aspect-Based Opinion Mining in Drug Reviews, proceedings of Portuguese Conference on Artificial Intelligence, 2017, pp. 815-827: Springer.
- Gopalakrishnan, V., Ramaswamy, C., Patient opinion mining to analyze drugs satisfaction using supervised learning, Journal of Applied Research and Technology, 2017, 15(4), 311-319.
- Uysal, A. K., Gunal, S., A novel probabilistic feature selection method for text classification, Knowledge-Based Systems, 2012, 36, 226-235.
- Forman, G., An extensive empirical study of feature selection metrics for text classification, Journal of Machine Learning Research, 2003, 3, 1289-1305.
- Zong, W., Wu, F., Chu, L.-K., Sculli, D., A discriminative and semantic feature selection method for text categorization, International Journal of Production Economics, 2015, 165, 215-222.
- Feng, L., Zuo, W., Wang, Y. Improved comprehensive measurement feature selection method for text categorization, proceedings of Network and Information Systems for Computers (ICNISC), 2015 International Conference on, 2015, pp. 125-128.
- Rehman, A., Javed, K., Babri, H. A., Saeed, M., Relative discrimination criterion – A novel feature ranking method for text data, Expert Systems with Applications, 2015, 42(7), 3670-3681.
- Joachims, T. Text categorization with support vector machines: Learning with many relevant features, proceedings of 10th European Conference on Machine Learning, Chemnitz, Germany, 1998, vol. 1398, pp. 137-142.
- Chang, C.-C., Lin, C.-J., LIBSVM: A library for support vector machines, ACM Transactions on Intelligent Systems and Technology, 2011, 2(3), 1-27.
- Jiang, L., Cai, Z., Zhang, H., Wang, D., Naive Bayes text classifiers: A locally weighted learning approach, Journal of Experimental & Theoretical Artificial Intelligence, 2013, 25(2), 273-286.
- Porter, M. F., An algorithm for suffix stripping, Program, 1980, 14(3), 130-137.
APA | Uysal A (2018). Comparative Performance Analysis of Techniques for Automatic Drug Review Classification. , 485 - 490. 10.18466/cbayarfbe.481096 |
Chicago | Uysal Alper Comparative Performance Analysis of Techniques for Automatic Drug Review Classification. (2018): 485 - 490. 10.18466/cbayarfbe.481096 |
MLA | Uysal Alper Comparative Performance Analysis of Techniques for Automatic Drug Review Classification. , 2018, ss.485 - 490. 10.18466/cbayarfbe.481096 |
AMA | Uysal A Comparative Performance Analysis of Techniques for Automatic Drug Review Classification. . 2018; 485 - 490. 10.18466/cbayarfbe.481096 |
Vancouver | Uysal A Comparative Performance Analysis of Techniques for Automatic Drug Review Classification. . 2018; 485 - 490. 10.18466/cbayarfbe.481096 |
IEEE | Uysal A "Comparative Performance Analysis of Techniques for Automatic Drug Review Classification." , ss.485 - 490, 2018. 10.18466/cbayarfbe.481096 |
ISNAD | Uysal, Alper. "Comparative Performance Analysis of Techniques for Automatic Drug Review Classification". (2018), 485-490. https://doi.org/10.18466/cbayarfbe.481096 |
APA | Uysal A (2018). Comparative Performance Analysis of Techniques for Automatic Drug Review Classification. Celal Bayar Üniversitesi Fen Bilimleri Dergisi, 14(4), 485 - 490. 10.18466/cbayarfbe.481096 |
Chicago | Uysal Alper Comparative Performance Analysis of Techniques for Automatic Drug Review Classification. Celal Bayar Üniversitesi Fen Bilimleri Dergisi 14, no.4 (2018): 485 - 490. 10.18466/cbayarfbe.481096 |
MLA | Uysal Alper Comparative Performance Analysis of Techniques for Automatic Drug Review Classification. Celal Bayar Üniversitesi Fen Bilimleri Dergisi, vol.14, no.4, 2018, ss.485 - 490. 10.18466/cbayarfbe.481096 |
AMA | Uysal A Comparative Performance Analysis of Techniques for Automatic Drug Review Classification. Celal Bayar Üniversitesi Fen Bilimleri Dergisi. 2018; 14(4): 485 - 490. 10.18466/cbayarfbe.481096 |
Vancouver | Uysal A Comparative Performance Analysis of Techniques for Automatic Drug Review Classification. Celal Bayar Üniversitesi Fen Bilimleri Dergisi. 2018; 14(4): 485 - 490. 10.18466/cbayarfbe.481096 |
IEEE | Uysal A "Comparative Performance Analysis of Techniques for Automatic Drug Review Classification." Celal Bayar Üniversitesi Fen Bilimleri Dergisi, 14, ss.485 - 490, 2018. 10.18466/cbayarfbe.481096 |
ISNAD | Uysal, Alper. "Comparative Performance Analysis of Techniques for Automatic Drug Review Classification". Celal Bayar Üniversitesi Fen Bilimleri Dergisi 14/4 (2018), 485-490. https://doi.org/10.18466/cbayarfbe.481096 |