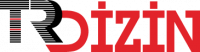
Determination of Increased Mental Workload Condition From EEG by the Use of Classification Techniques
Yıl: 2019 Cilt: 31 Sayı: 1 Sayfa Aralığı: 47 - 52 Metin Dili: İngilizce DOI: 10.7240/jeps.459420 İndeks Tarihi: 13-04-2020
Determination of Increased Mental Workload Condition From EEG by the Use of Classification Techniques
Öz: Extraction of the information hidden in the brain electrical signal enhances the classification of the current mental status. In this study, 16 channel electroencephalogram (EEG) data were collected from 15 volunteers under three conditions. Participants were asked to rest with eyes open and eyes closed states each with a duration of three minutes. Finally, a task has been imposed to increase the mental workload (MW). EEG data were epoched with a duration of one second and power spectrum was computed for each time window. The power spectral features of all channels in traditional bands were calculated for all subjects and the results were concatenated to form the input data to be used in classification. Decision tree, K-nearest neighbor (KNN) and Support Vector Machine (SVM) techniques were implemented in order to classify the one-second epochs. The accuracy value obtained from KNN was found to be 0.94 while it was 0.88 for decision tree and SVM. KNN was found to outperform the two methods when all channel and power spectral features were used. It can be concluded that, even with the use of input features formed by concatenating all subject’s data, high classification accuracies can be obtained in the determination of the increased MW state.
Anahtar Kelime: Sınıflandırma Teknikleri Kullanılarak EEG’den Artan Mental İşyükü Durumunun Belirlenmesi
Öz: Beyin sinyallerinin sınıflandırılması kişilerin mental durumu hakkında bilgi sahibi olmamızı kolaylaştırır. Bu çalışma kapsamında onbeş katılımcıdan 16 kanallı EEG verisi üç farklı durum için toplanmıştır. Katılımcılardan üçer dakika gözleri kapalı ve açık şekilde dinlenmeleri istenmiştir. Sonrasında mental işyükünü arttıracak bir ödevde uygulanmıştır. EEG verileri birer saniyelik pencerelere ayrılmış ve her pencere için güç spektrumları hesaplanmıştır. Geleneksel frekans bantlarına ait güç spektrumları her katılımcı için hesaplanmış ve sonuçlar sınıflandırmada kullanılmak üzere arka arkaya getirilmiştir. Karar ağaçları, K-en yakım komşuluk (KNN) ve Destek Vektör Makinaları (DVM) teknikleri birer saniyelik pencere verilerinin sınıflandırılması için uygulanmıştır. KNN için doğruluk değeri 0.94 olurken karar ağaçları ve DVM için 0.88 değerine ulaşılmıştır. KNN yöntemi diğer iki yönteme göre tüm kanalların güç spektrumu kullanıldığı durumda daha yüksek doğruluk oranı vermiştir. Sonuç olarak, tüm katılımcıların verilerinin bir havuza konulup sınıflandırma işlemi yapıldığında dahi yüksek mental işyükü durumunun ayrıştırılabildiği görülmüştür.
Anahtar Kelime: Belge Türü: Makale Makale Türü: Araştırma Makalesi Erişim Türü: Erişime Açık
- [1] Mumtaz W, Ali S.S.A., Yasin M.A.M. & Malik A.S., (2018) A machine learning framework involving EEG-based functional connectivity to diagnose major depressive disorder (MDD). Med Biol Eng Comput. Feb;56(2):233-246. doi: 10.1007/s11517.017.1685-z.
- [2] Kanda P.A.F.M., Trambaiolli L.R., Lorena A.C., Fraga F.J., Basile L.F.I., Nitrini R., & Anghinah R. (2014), Clinician’s Road Map to Wavelet EEG as an Alzheimer’s disease Biomarker, Clinical EEG and Neuroscience, doi: 10.1177/155.005.9413486272
- [3] Babiloni, C., Triggiani, A. I., Lizio, R., Cordone, S., Tattoli, G., Bevilacqua, V., Soricelli, A., Ferri, R., Nobili, F., Gesualdo, L., Millán-Calenti, J. C., Buján, A., Tortelli, R., Cardinali, V., Barulli, M. R., Giannini, A., Spagnolo, P., Armenise, S., Buenza, G., Scianatico, G., Logroscino, G., Frisoni, G. B., … Del Percio, C. (2016). Classification of Single Normal and Alzheimer’s Disease Individuals from Cortical Sources of Resting State EEG Rhythms. Frontiers in neuroscience, 10(47). doi:10.3389/fnins.2016.00047
- [4] Bellotti R., De Carlo F., Massafra R, de Tommaso M., Sciruicchio V., (2004) Topographic classification of EEG patterns in Huntington’s disease. Neurol Clin Neurophysiol. Nov 30;2004:37.
- [5] Cruz-Garza, J. G., Hernandez, Z. R., Nepaul, S., Bradley, K. K., & Contreras-Vidal, J. L. (2014). Neural decoding of expressive human movement from scalp electroencephalography (EEG). Frontiers in human neuroscience, 8, 188. doi:10.3389/fnhum.2014.00188
- [6] OberMaier B., Guger C., Neuper C. & Pfurtscheller G., (2001) Hidden Markov models for online classification of single trial EEG data, Pattern Recognition Letters, 22, 1299-1309
- [7] Lin C.J. & Hsieh M.H., (2009) Classification of mental task from EEG data using neural networks based on particle swarm optimization, Neurocomputing, 72, 1121-1130
- [8] Nemrodov D., Niemeier M., Patel A., Nestor A., (2018) The Neural Dynamics of Facial Identity Processing: insights from EEG-Based Pattern Analysis and Image Reconstruction. eNeuro 29, ENEURO.0358-17.2018; DOI: 10.1523/ ENEURO.0358-17.2018
- [9] Iturrate I., Chavarriaga R., Montesano L., Minguez J. & Millan J.D.R, (2015) Teaching brain-machine interfaces as an alternative paradigm to neuroprosthetics control, Scientific Reports, 5
- [10] Amin H. U., Mumtaz W., Subhani A. R., Saad M. N. M. & Malik A. S., (2017) Classification of EEG Signals Based on Pattern Recognition Approach, Front. Comput. Neurosci.
- 11(103) doi: 10.3389/fncom.2017.00103 [11] V. N. Vapnik, (1999) An Overview of Statistical Learning Theory, IEEE Transactions on Neural Networks 10(5)
- [12] J. R. Quinlan. (1986) Induction of decision trees. Machine Learning, 1:81–106
- [13] Wang X.W., Nie D. & Lu B.L., (2014) Emotional state classification from EEG data using machine learning approach, Neurocomputing 129 94–106
- [14] Gutierrez D. & Escalona-Vargas D.I., (2010) EEG data classification through signal spatial redistribution and optimized linear discriminants, Computer Methods and Programs in Biomedicine 97, 39–47
- [15] Parvinnia E., Sabeti M., Jahromi M.Z. & Boostani R., (2014) Classification of EEG Signals using adaptive weighted distance nearest neighbor algorithm, Journal of King Saud University – Computer and Information Sciences, 26(1), Pages 1-6
- [16] Quinlan J.R., (1993) Programs for Machine Learning, Morgan Kaufman, San Francisco, USA
APA | Duru A (2019). Determination of Increased Mental Workload Condition From EEG by the Use of Classification Techniques. , 47 - 52. 10.7240/jeps.459420 |
Chicago | Duru Adil Deniz Determination of Increased Mental Workload Condition From EEG by the Use of Classification Techniques. (2019): 47 - 52. 10.7240/jeps.459420 |
MLA | Duru Adil Deniz Determination of Increased Mental Workload Condition From EEG by the Use of Classification Techniques. , 2019, ss.47 - 52. 10.7240/jeps.459420 |
AMA | Duru A Determination of Increased Mental Workload Condition From EEG by the Use of Classification Techniques. . 2019; 47 - 52. 10.7240/jeps.459420 |
Vancouver | Duru A Determination of Increased Mental Workload Condition From EEG by the Use of Classification Techniques. . 2019; 47 - 52. 10.7240/jeps.459420 |
IEEE | Duru A "Determination of Increased Mental Workload Condition From EEG by the Use of Classification Techniques." , ss.47 - 52, 2019. 10.7240/jeps.459420 |
ISNAD | Duru, Adil Deniz. "Determination of Increased Mental Workload Condition From EEG by the Use of Classification Techniques". (2019), 47-52. https://doi.org/10.7240/jeps.459420 |
APA | Duru A (2019). Determination of Increased Mental Workload Condition From EEG by the Use of Classification Techniques. International journal of advances in engineering and pure sciences (Online), 31(1), 47 - 52. 10.7240/jeps.459420 |
Chicago | Duru Adil Deniz Determination of Increased Mental Workload Condition From EEG by the Use of Classification Techniques. International journal of advances in engineering and pure sciences (Online) 31, no.1 (2019): 47 - 52. 10.7240/jeps.459420 |
MLA | Duru Adil Deniz Determination of Increased Mental Workload Condition From EEG by the Use of Classification Techniques. International journal of advances in engineering and pure sciences (Online), vol.31, no.1, 2019, ss.47 - 52. 10.7240/jeps.459420 |
AMA | Duru A Determination of Increased Mental Workload Condition From EEG by the Use of Classification Techniques. International journal of advances in engineering and pure sciences (Online). 2019; 31(1): 47 - 52. 10.7240/jeps.459420 |
Vancouver | Duru A Determination of Increased Mental Workload Condition From EEG by the Use of Classification Techniques. International journal of advances in engineering and pure sciences (Online). 2019; 31(1): 47 - 52. 10.7240/jeps.459420 |
IEEE | Duru A "Determination of Increased Mental Workload Condition From EEG by the Use of Classification Techniques." International journal of advances in engineering and pure sciences (Online), 31, ss.47 - 52, 2019. 10.7240/jeps.459420 |
ISNAD | Duru, Adil Deniz. "Determination of Increased Mental Workload Condition From EEG by the Use of Classification Techniques". International journal of advances in engineering and pure sciences (Online) 31/1 (2019), 47-52. https://doi.org/10.7240/jeps.459420 |