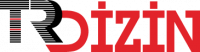
Comparison of the Global, Local and Semi-Local Chaotic Prediction Methods for Stock Markets: The Case of FTSE-100 Index
Yıl: 2019 Cilt: 7 Sayı: 2 Sayfa Aralığı: 289 - 300 Metin Dili: İngilizce DOI: 10.17093/alphanumeric.629722 İndeks Tarihi: 14-04-2020
Comparison of the Global, Local and Semi-Local Chaotic Prediction Methods for Stock Markets: The Case of FTSE-100 Index
Öz: Chaotic prediction methods are classified as global, local and semi-local methods. In this paper, unlike the studies in the literature,it is aimed to compare all these methods together for stock markets in terms of prediction performance and to determine thebest prediction method for stock markets. For this purpose, Multi-Layer Perceptron (MLP) neural networks from global methods,nearest neighbour method from local methods, radial basis functions from semi-local methods are used. The FTSE-100 index isselected to represent the stock market and applied the all methods to these data. The prediction performance is measured interm of root mean square error (RMSE) and normalized mean square error (NMSE). As a result of the analysis; it has beendetermined that the best prediction method for the FTSE-100 index is the semi-local method. While it is possible to make amaximum of 5 days prediction with global and local methods, it has been determined that up to 20 days prediction can be madewith the semi-local prediction methods. The results show that semi-local prediction methods are successful in predicting thebehavior of stock market.
Anahtar Kelime: Konular:
Belge Türü: Makale Makale Türü: Araştırma Makalesi Erişim Türü: Erişime Açık
- Abarbanel, H. (1996). Analysis Of Observed Chaotic Data. New York: Spinger-Verlag.
- Abarbanel, H. D., Brown, R., Kadtke, J. B. (1990). “Prediction in chaotic nonlinear systems: methods for time series with broadband fourier spectra”. Physical Review A, 41(4), 1782-1807.
- Abhyankar, A., Copeland, L. S., Wong, W. (1995). “Nonlinear dynamics in real-time equity market indices: evidence from the United Kingdom”. The Economic Journal, 864-880.
- Brock, W. A., Hsieh, D. A., Lebaron, B. D. (1991). Nonlinear Dynamics, Chaos and Instability: Statistical Theory and Economic Evidence. MIT Press.
- Casdagli, M. (1989). “Nonlinear prediction of chaotic time series”. Physica D: Nonlinear Phenomena, 35(3), 335-356.
- Chan, K.S., Tong, H. (2001). Chaos: A Statistical Perspective, New York: Springer-Verlag.
- Eckmann, J. P., Ruelle, D. (1985). “Ergodic theory of chaos and strange attractors”. Reviews of Modern Physics, 57(3), 617-656.
- Eldridge, R. M., Coleman, M. P. (1993). “The British FTSE-100 Index: Chaotically Deterministic or Random”. Working Paper, School of Business, Fairfield University.
- Elshorbagy, A., Simonovic, S. P., Panu, U. S. (2002). “Estimation of missing streamflow data using principles of chaos theory”. Journal of Hydrology, 255(1), 123-133.
- Elsner, J.B. (1992). “Predicting time series using a neural network as a method of distinguishing chaos from noise”. Journal of Physics A: Mathematical and General, 25(4) 843–850.
- Farmer, J.D., Sidorowich, J.J. (1987). “Predicting chaotic time series”. Physical Review Letters, 59(8), 845-848.
- Fraedrich, K. (1986). “Estimating the dimensions of weather and climate attractors”. Journal of The Atmospheric Sciences, 43(5), 419-432.
- Fraser, A. M., Swinney, H. L. (1986). “Independent coordinates for strange attractors from mutual information”. Physical Reviews A, 33, 1134-1140.
- Grassberger, P., Procaccia, I. (1983a). “Characterization of strange attractors”. Physical Review Letters, 50(5), 346-349.
- Grassberger, P., Procaccia, I. (1983b). “Measuring the strangeness of strange attractors”. Physica D: Nonlinear Phenomena, 9(1-2), 189-208.
- Guegan, D., Mercier, L. (2005). “Prediction in chaotic time series: methods and comparisons with an application to financial intra-day data”. The European Journal Of Finance, 11(2), 137-150.
- Hanias M., Magafas L., Konstantaki P. (2013). “Nonlinear analysis of S&P index”. Equilibrium Quarterly Journal of Economics and Economic Policy, 8(4), 125-135.
- Hassan M.R., Nath, B. (2005). “Stock market forecasting using Hidden Markov Model: A New Approach”. Proceedings of the 5th International Conference on Intelligent Systems Design and Applications, 192–196.
- Hsieh, D.A. (1991). “Chaos and nonlinear dynamics: Application to financial markets”. Journal of Finance, 46(5), 1839–1877.
- Huang, S. C., Chuang, P. J., Wu, C. F., Lai, H. J. (2010). “Chaos-based support vector regressions for exchange rate forecasting”. Expert Systems with Applications, 37(12), 8590-8598.
- Kantz, H. (1994). “A robust method to estimate the maximal Lyapunov exponent of a time series”. Physics Letters A, 185(1), 77-87.
- Kantz, H., Schreiber, T. (2004). Nonlinear Time Series Analysis. UK: Cambridge University Press.
- Karunasinghe, D.S., Liong, S.Y. (2006). “Chaotic time series prediction with a global model: artificial neural network”. Journal of Hydrology, 323(1), 92-105.
- Kazem, A., Sharifi, E., Hussain, F. K., Saberi, M., Hussain, O. K. (2013). “Support vector regression with chaos-based firefly algorithm for stock market price forecasting”. Applied Soft Computing, 13(2), 947-958.
- Kennel, M.B., Brown, R., Abarbanel, H.D.I. (1992). “Determining embedding dimension for phasespace reconstruction using a geometrical construction”. Physical Review A, 45(6), 3403- 3411.
- Lapedes, A., Farber, R., (1987). Nonlinear Signal Processing Using Neural Networks: Prediction and System Modelling. Technical Report, Los Alamos National Laboratory, Los Alamos, NM.
- Lillekjendlie, B., Kugiumtzis, D., Christophersen, N. (1994). “Chaotic time series part II: System identification and prediction”. Modeling, Identification And Control, 15, 225-243.
- Mayfield, E. S., Mizrach, B. (1992). “On determining the dimension of real-time stock-price data”. Journal of Business and Economic Statistics, 10(3), 367-374.
- Özdemir, S. D., Akgül, I. (2014). “Hisse senedi piyasalarının kaotik yapısı ve yapay sinir ağları ile öngörüsü: İMKB-100 örneği”. İktisat İşletme ve Finans, 29 (336), 31-58.
- Shang, P., Li, X., Kamae, S. (2005). “Chaotic analysis of traffic time series”. Chaos, Solitons and Fractals, 25(1), 121-128.
- Sivakumar, B., Jayawardena, A. W., Fernando, T. M. K. G. (2002). “River flow forecasting: use of phase-space reconstruction and artificial neural networks approaches”. Journal of Hydrology, 265(1), 225-245.
- Smaoui, N. (1999). “An artificial neural network noise reduction method for chaotic attractors”. International Journal of Computer Mathematics, 73(4), 417-431.
- Sprott, J. C. (2003). Chaos and Time Series Analysis. Oxford Press.
- Sprott, J. C. (2010). Elegant Chaos: Algebraically Simple Chaotic Flows. World Scientific.
- Takens, F., (1981). in Dynamical Systems and Turbulence, Warwick Vol. 898 of Lecture Notes in Mathematics, edited by D.A. Rand, L. S. Young, Springer, Berlin.
- Vaidyanathan, R., Krehbiel, T. (1992). “Does the S&P 500 futures mispricing series exhibit nonlinear dependence across time?”. Journal of Futures Markets, 12(6), 659-677.
- Webel, K. (2012). “Chaos in German stock returns-new evidence from the 0–1 test”. Economics Letters, 115(3), 487-489.
- Wolf, A., Swift, J. B., Swinney, H. L., Vastano, J. A. (1985). “Determining Lyapunov exponents from a time series”. Physica D: Nonlinear Phenomena,16(3), 285-317.
- Xiaofeng, G., Lai, C. H. (1999). “Improvement of the local prediction of chaotic time series”. Physical Review E, 60(5), 5463-5468.
APA | İŞİ A, Cemrek F (2019). Comparison of the Global, Local and Semi-Local Chaotic Prediction Methods for Stock Markets: The Case of FTSE-100 Index. , 289 - 300. 10.17093/alphanumeric.629722 |
Chicago | İŞİ AYŞE,Cemrek Fatih Comparison of the Global, Local and Semi-Local Chaotic Prediction Methods for Stock Markets: The Case of FTSE-100 Index. (2019): 289 - 300. 10.17093/alphanumeric.629722 |
MLA | İŞİ AYŞE,Cemrek Fatih Comparison of the Global, Local and Semi-Local Chaotic Prediction Methods for Stock Markets: The Case of FTSE-100 Index. , 2019, ss.289 - 300. 10.17093/alphanumeric.629722 |
AMA | İŞİ A,Cemrek F Comparison of the Global, Local and Semi-Local Chaotic Prediction Methods for Stock Markets: The Case of FTSE-100 Index. . 2019; 289 - 300. 10.17093/alphanumeric.629722 |
Vancouver | İŞİ A,Cemrek F Comparison of the Global, Local and Semi-Local Chaotic Prediction Methods for Stock Markets: The Case of FTSE-100 Index. . 2019; 289 - 300. 10.17093/alphanumeric.629722 |
IEEE | İŞİ A,Cemrek F "Comparison of the Global, Local and Semi-Local Chaotic Prediction Methods for Stock Markets: The Case of FTSE-100 Index." , ss.289 - 300, 2019. 10.17093/alphanumeric.629722 |
ISNAD | İŞİ, AYŞE - Cemrek, Fatih. "Comparison of the Global, Local and Semi-Local Chaotic Prediction Methods for Stock Markets: The Case of FTSE-100 Index". (2019), 289-300. https://doi.org/10.17093/alphanumeric.629722 |
APA | İŞİ A, Cemrek F (2019). Comparison of the Global, Local and Semi-Local Chaotic Prediction Methods for Stock Markets: The Case of FTSE-100 Index. Alphanumeric Journal, 7(2), 289 - 300. 10.17093/alphanumeric.629722 |
Chicago | İŞİ AYŞE,Cemrek Fatih Comparison of the Global, Local and Semi-Local Chaotic Prediction Methods for Stock Markets: The Case of FTSE-100 Index. Alphanumeric Journal 7, no.2 (2019): 289 - 300. 10.17093/alphanumeric.629722 |
MLA | İŞİ AYŞE,Cemrek Fatih Comparison of the Global, Local and Semi-Local Chaotic Prediction Methods for Stock Markets: The Case of FTSE-100 Index. Alphanumeric Journal, vol.7, no.2, 2019, ss.289 - 300. 10.17093/alphanumeric.629722 |
AMA | İŞİ A,Cemrek F Comparison of the Global, Local and Semi-Local Chaotic Prediction Methods for Stock Markets: The Case of FTSE-100 Index. Alphanumeric Journal. 2019; 7(2): 289 - 300. 10.17093/alphanumeric.629722 |
Vancouver | İŞİ A,Cemrek F Comparison of the Global, Local and Semi-Local Chaotic Prediction Methods for Stock Markets: The Case of FTSE-100 Index. Alphanumeric Journal. 2019; 7(2): 289 - 300. 10.17093/alphanumeric.629722 |
IEEE | İŞİ A,Cemrek F "Comparison of the Global, Local and Semi-Local Chaotic Prediction Methods for Stock Markets: The Case of FTSE-100 Index." Alphanumeric Journal, 7, ss.289 - 300, 2019. 10.17093/alphanumeric.629722 |
ISNAD | İŞİ, AYŞE - Cemrek, Fatih. "Comparison of the Global, Local and Semi-Local Chaotic Prediction Methods for Stock Markets: The Case of FTSE-100 Index". Alphanumeric Journal 7/2 (2019), 289-300. https://doi.org/10.17093/alphanumeric.629722 |