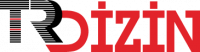
EVALUATING THE EFFECT OF BLENDING RATIO ON THE CO-GASIFICATION OF HIGH ASH COAL AND BIOMASS IN A FLUIDIZED BED GASIFIER USING MACHINE LEARNING
Yıl: 2019 Cilt: 5 Sayı: 1 Sayfa Aralığı: 1 - 12 Metin Dili: İngilizce İndeks Tarihi: 08-07-2020
EVALUATING THE EFFECT OF BLENDING RATIO ON THE CO-GASIFICATION OF HIGH ASH COAL AND BIOMASS IN A FLUIDIZED BED GASIFIER USING MACHINE LEARNING
Öz: Co-gasification is a process that converts coal and biomass into useful products, such as syngas. Analytical and numericalapproaches for modeling co-gasification process either require enormous amount of time or make a lot of assumptionswhich reduce consistency of the models in practical applications. Artificial Intelligence based modeling methods areused to simulate and to make predictions of outcomes of the co-gasification process. Even though previous studies resultin successful modelling for specific cases, limited selection of methods and lack of implementation of cross-validationtechniques causes insufficiency to explain unbiased performance evaluations and up-scale usability of the methods. Inthis paper, six different regression methods are employed to predict outputs of co-gasification process using a datasetcontaining 56 observations. Moreover, the original dataset is randomly resampled so that each model’s generalizationability is further assessed. The prediction performance of the proposed techniques on both datasets is evaluated andpractical usability is discussed.
Anahtar Kelime: AKIŞKAN YATAK GAZLAŞTIRICIDA YÜKSEK KÜLLÜ KÖMÜR VE BİYOKÜTLENİN BİRLİKTE GAZLAŞTIRILMASINDA KARIŞIM ORANI ETKİLERİNİN MAKİNE ÖĞRENMESİ İLE DEĞERLENDİRİLMESİ
Öz: Birlikte gazlaştırma, kömür ve biyokütlenin sentez gazı gibi faydalı ürünlere dönüştürülmesini sağlayan bir süreçtir. Birlikte gazlaştırma sürecinin modellenmesi için analitik ve sayısal yaklaşımlar ya çok uzun zaman ya da pratik uygulamalarda modellerin tutarlılığını azaltan çok sayıda varsayım gerektirir. Yapay zeka tabanlı modelleme yöntemleri, birlikte gazlaştırma sürecini simüle etmek ve sonuçlarını tahmin etmek için kullanılmıştır. Her ne kadar önceki çalışmalar belirli durumlar için başarılı bir modelleme ile sonuçlanmışsa da, sınırlı yöntem seçimi ve çapraz doğrulama tekniklerinin uygulanmaması, yansız performans değerlendirmelerini ve yöntemlerin büyük ölçekli kullanılabilirliğini açıklamakta yetersizliğe neden olmuştur. Bu çalışmada, 56 gözlem içeren bir veri seti kullanılarak birlikte gazlaştırma işleminin çıktılarını tahmin etmek için altı farklı regresyon yöntemi kullanılmıştır. Ardından, orijinal veri kümesi rastgele yeniden örneklendirilip, böylece her modelin genelleme yeteneği daha fazla değerlendirilmiştir. Önerilen tekniklerin her iki veri seti üzerinde tahmin performansı değerlendirilmiş ve pratik kullanılabilirlik tartışılmıştır.
Anahtar Kelime: Belge Türü: Makale Makale Türü: Araştırma Makalesi Erişim Türü: Erişime Açık
- [1] BRITISH PETROLEUM COMPANY, “Statistical Review of World Energy,” Statistical Review of World Energy, Available: http://www.bp.com/ productlanding.do?categoryId=6929&contentId=70 44622, 2018.
- [2] Panwar, N. L., Kaushik, S. C., and Kothari, S., “Role of renewable energy sources in environmental protection: A review,” Renewable and Sustainable Energy Reviews, vol. 15, no. 3. Pergamon, pp. 1513– 1524, 2011.
- [3] Srivastava, T., “Renewable Energy (Gasification),” Adv. Electron. Electr. Eng., vol. 3, no. 9, pp. 1243– 1250, 2013.
- [4] Mutlu, A. Y. and Yucel, O., “An artificial intelligence based approach to predicting syngas composition for downdraft biomass gasification,” Energy, vol. 165, pp. 895–901, 2018.
- [5] Kamińska-Pietrzak, N. and Smoliński, A., “Selected Environmental Aspects of Gasification and Co- Gasification of Various Types of Waste,” J. Sustain. Min., vol. 12, no. 4, pp. 6–13, 2013.
- [6] Akia, M., Arandiyan, H., Yazdani, F., Han, D., and Motaee, E., “A review on conversion of biomass to biofuel by nanocatalysts,” Biofuel Res. J., vol. 01, no. 01, pp. 16–25, 2015.
- [7] Ud Din, Z. and Zainal, Z. A., “Biomass integrated gasification-SOFC systems: Technology overview,” Renewable and Sustainable Energy Reviews, vol. 53. pp. 1356–1376, 2016.
- [8] Karayilmazlar, S. et al., “Biyokütlenin Türkiye’de Enerji Üretiminde Değerlendirilmesi,” J. Bartin Fac. For., vol. 19, no. 13, pp. 63–75, 2011.
- [9] Arabloo, M., Bahadori, A., Ghiasi, M. M., Lee, M., Abbas, A., and Zendehboudi, S., “A novel modeling approach to optimize oxygen-steam ratios in coal gasification process,” Fuel, vol. 153, pp. 1–5, 2015.
- [10] Seo, H. K. et al., “Effects of operating factors in the coal gasification reaction,” Korean Journal of Chemical Engineering, vol. 28, no. 9. pp. 1851– 1858, 2011.
- [11] Kalina, J., “Retrofitting of municipal coal fired heating plant with integrated biomass gasification gas turbine based cogeneration block,” Energy Convers. Manag., vol. 51, no. 5, pp. 1085–1092, May 2010.
- [12] Sueyasu, T., Oike, T., Mori, A., Kudo, S., Norinaga, K., and Hayashi, J. I., “Simultaneous steam reforming of tar and steam gasification of char from the pyrolysis of potassium-loaded woody biomass,” in Energy and Fuels, vol. 26, no. 1, pp. 199–208, 2012.
- [13] Guo, B., Li, D., Cheng, C., Lü, Z. A., and Shen, Y., “Simulation of biomass gasification with a hybrid neural network model,” Bioresour. Technol., vol. 76, no. 2, pp. 77–83, 2001.
- [14] Puig-Arnavat, M., Hernández, J. A., Bruno, J. C., and Coronas, A., “Artificial neural network models for biomass gasification in fluidized bed gasifiers,” Biomass and Bioenergy, vol. 49, pp. 279–289, 2013.
- [15] Pandey, D. S., Das, S., Pan, I., Leahy, J. J., and Kwapinski, W., “Artificial neural network based modelling approach for municipal solid waste gasification in a fluidized bed reactor,” Waste Manag., vol. 58, pp. 202–213, 2016.
- [16] Kohavi, R., “A study of cross-validation and bootstrap for accuracy estimation and model selection,” in Proceedings of the 14th international joint conference on Artificial intelligence - Volume 2, vol. 2, no. 0, pp. 1137–1143, 1995.
- [17] Saha, S. et al., “Co-gasification of High Ash Coal– Biomass Blends in a Fluidized Bed Gasifier: Experimental Study and Computational Intelligence-Based Modeling,” Waste and Biomass Valorization, pp. 1–19, 2018.
- [18] Astorino, A., Gorgone, E., Gaudioso, M., and Pallaschke, D., “Data preprocessing in semisupervised SVM classification,” Optimization, vol. 60, no. 1–2, pp. 143–151, 2011.
- [19] Robert, C., “Machine Learning, a Probabilistic Perspective,” CHANCE, vol. 27, no. 2, pp. 62–63, 2015.
- [20] Takajo, H. and Takahashi, T., “Noniterative method for obtaining the exact solution for the normal equation in least-squares phase estimation from the phase difference,” J. Opt. Soc. Am. A, vol. 5, no. 11, p. 1818, 2008.
- [21] Ecology, S. and Apr, N., “Nonlinear Redundancy Analysis and Canonical Correspondence Analysis Based on Polynomial Regression,” Ecology, vol. 83, no. 4, pp. 1146–1161, 2013.
- [22] Hawkins, D. M., “The Problem of Overfitting,” J. Chem. Inf. Comput. Sci., vol. 44, no. 1, pp. 1–12, 2004.
- [23] Prasad, A. M., Iverson, L. R., and Liaw, A., “Newer classification and regression tree techniques: Bagging and random forests for ecological prediction,” Ecosystems, vol. 9, no. 2, pp. 181–199, 2006.
- [24] Bai, Y., Li, Y., Liu, Y., and Ma, Z., “Short-term prediction of distribution network faults based on support vector machine,” in Proceedings of the 2017 12th IEEE Conference on Industrial Electronics and Applications, ICIEA 2017, vol. 2018-Febru, pp. 1421–1426, 2018.
- [25] Lu, C. J., Lee, T. S., and Chiu, C. C., “Financial time series forecasting using independent component analysis and support vector regression,” Decis. Support Syst., vol. 47, no. 2, pp. 115–125, 2009.
- [26] Williams, C. K. I., “Learning With Kernels: Support Vector Machines, Regularization, Optimization, and Beyond,” J. Am. Stat. Assoc., vol. 98, no. 462, pp. 489–489, 2009.
- [27] Zhang, G., Eddy Patuwo, B., and Y. Hu, M., “Forecasting with artificial neural networks:,” Int. J. Forecast., vol. 14, no. 1, pp. 35–62, 2002.
- [28] Gudise, V. G. and Venayagamoorthy, G. K., “Comparison of particle swarm optimization and backpropagation as training algorithms for neural networks,” in 2003 IEEE Swarm IntelligenceSymposium, SIS 2003 - Proceedings, pp. 110–117, 2013.
- [29] Ambroise, C. and McLachlan, G. J., “Selection bias in gene extraction on the basis of microarray geneexpression data,” Proc. Natl. Acad. Sci., vol. 99, no. 10, pp. 6562–6566, 2002.
APA | Elmaz F, yücel ö, Mutlu A (2019). EVALUATING THE EFFECT OF BLENDING RATIO ON THE CO-GASIFICATION OF HIGH ASH COAL AND BIOMASS IN A FLUIDIZED BED GASIFIER USING MACHINE LEARNING. , 1 - 12. |
Chicago | Elmaz Furkan,yücel özgün,Mutlu Ali EVALUATING THE EFFECT OF BLENDING RATIO ON THE CO-GASIFICATION OF HIGH ASH COAL AND BIOMASS IN A FLUIDIZED BED GASIFIER USING MACHINE LEARNING. (2019): 1 - 12. |
MLA | Elmaz Furkan,yücel özgün,Mutlu Ali EVALUATING THE EFFECT OF BLENDING RATIO ON THE CO-GASIFICATION OF HIGH ASH COAL AND BIOMASS IN A FLUIDIZED BED GASIFIER USING MACHINE LEARNING. , 2019, ss.1 - 12. |
AMA | Elmaz F,yücel ö,Mutlu A EVALUATING THE EFFECT OF BLENDING RATIO ON THE CO-GASIFICATION OF HIGH ASH COAL AND BIOMASS IN A FLUIDIZED BED GASIFIER USING MACHINE LEARNING. . 2019; 1 - 12. |
Vancouver | Elmaz F,yücel ö,Mutlu A EVALUATING THE EFFECT OF BLENDING RATIO ON THE CO-GASIFICATION OF HIGH ASH COAL AND BIOMASS IN A FLUIDIZED BED GASIFIER USING MACHINE LEARNING. . 2019; 1 - 12. |
IEEE | Elmaz F,yücel ö,Mutlu A "EVALUATING THE EFFECT OF BLENDING RATIO ON THE CO-GASIFICATION OF HIGH ASH COAL AND BIOMASS IN A FLUIDIZED BED GASIFIER USING MACHINE LEARNING." , ss.1 - 12, 2019. |
ISNAD | Elmaz, Furkan vd. "EVALUATING THE EFFECT OF BLENDING RATIO ON THE CO-GASIFICATION OF HIGH ASH COAL AND BIOMASS IN A FLUIDIZED BED GASIFIER USING MACHINE LEARNING". (2019), 1-12. |
APA | Elmaz F, yücel ö, Mutlu A (2019). EVALUATING THE EFFECT OF BLENDING RATIO ON THE CO-GASIFICATION OF HIGH ASH COAL AND BIOMASS IN A FLUIDIZED BED GASIFIER USING MACHINE LEARNING. Mugla Journal of Science and Technology, 5(1), 1 - 12. |
Chicago | Elmaz Furkan,yücel özgün,Mutlu Ali EVALUATING THE EFFECT OF BLENDING RATIO ON THE CO-GASIFICATION OF HIGH ASH COAL AND BIOMASS IN A FLUIDIZED BED GASIFIER USING MACHINE LEARNING. Mugla Journal of Science and Technology 5, no.1 (2019): 1 - 12. |
MLA | Elmaz Furkan,yücel özgün,Mutlu Ali EVALUATING THE EFFECT OF BLENDING RATIO ON THE CO-GASIFICATION OF HIGH ASH COAL AND BIOMASS IN A FLUIDIZED BED GASIFIER USING MACHINE LEARNING. Mugla Journal of Science and Technology, vol.5, no.1, 2019, ss.1 - 12. |
AMA | Elmaz F,yücel ö,Mutlu A EVALUATING THE EFFECT OF BLENDING RATIO ON THE CO-GASIFICATION OF HIGH ASH COAL AND BIOMASS IN A FLUIDIZED BED GASIFIER USING MACHINE LEARNING. Mugla Journal of Science and Technology. 2019; 5(1): 1 - 12. |
Vancouver | Elmaz F,yücel ö,Mutlu A EVALUATING THE EFFECT OF BLENDING RATIO ON THE CO-GASIFICATION OF HIGH ASH COAL AND BIOMASS IN A FLUIDIZED BED GASIFIER USING MACHINE LEARNING. Mugla Journal of Science and Technology. 2019; 5(1): 1 - 12. |
IEEE | Elmaz F,yücel ö,Mutlu A "EVALUATING THE EFFECT OF BLENDING RATIO ON THE CO-GASIFICATION OF HIGH ASH COAL AND BIOMASS IN A FLUIDIZED BED GASIFIER USING MACHINE LEARNING." Mugla Journal of Science and Technology, 5, ss.1 - 12, 2019. |
ISNAD | Elmaz, Furkan vd. "EVALUATING THE EFFECT OF BLENDING RATIO ON THE CO-GASIFICATION OF HIGH ASH COAL AND BIOMASS IN A FLUIDIZED BED GASIFIER USING MACHINE LEARNING". Mugla Journal of Science and Technology 5/1 (2019), 1-12. |