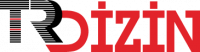
Predicting coronary artery disease using different artificial neural network models
Yıl: 2008 Cilt: 8 Sayı: 4 Sayfa Aralığı: 249 - 254 Metin Dili: İngilizce İndeks Tarihi: 29-07-2022
Predicting coronary artery disease using different artificial neural network models
Öz: Amaç: Bu çalışmada, koroner arter hastalığının (KAH) tahmin edilebilmesi için değişik sekiz öğrenme algoritması ile farklı yapay sinir ağımodelleri oluşturulmuş ve tanıtılması amaçlanmıştır.Yöntemler: Bu çalışma geriye dönük bir vaka kontrol araştırması olarak gerçekleştirilmiştir. Çalışmaya, anjiyografik olarak majör epikardiyal arterlerin en az bir tanesinde %50’den fazla darlığı olan 124 ardışık birey dâhil edildi. Anjiyografik olarak normal koroner arterlere sahip olan 113 birey ise kontrol grubu olarak alınmıştır. Çok katmanlı “perseptron” yapay sinir ağları uygulandı. Değişik sekiz öğrenme algoritması ile eğitilen farklı yapay sinir ağı modelleri, toplam 237 kayıtta, 171’i eğitimde ve 66’sı ise teste kullanılarak oluşturuldu. Tahminin performansı, duyarlılık, seçicilik ve doğruluk oranlarına dayalı olarak değerlendirilmiştir. Bulgular: Çalışmanın sonuçları, oluşturulan yapay sinir ağı modelleri ile KAH’ın tahmininde yüksek oranda (%71.0’den daha yüksek) duyarlılık, seçicilik ve doğruluk oranları elde edildiği için modellerin performansının iyi olduğunu göstermiştir. Doğruluk, duyarlılık ve seçicilik değerleri eğitimde sırasıyla %83.63 - %100, %86.46 - %100 ve %74.67 - %100 arasında iken, testte ise duyarlılık %71’den daha büyük, seçicilik %76’dan daha büyük ve doğruluk %81’den daha büyük olarak elde edilmiştir. Sonuç: Geriye yayılım algoritmasından başka farklı öğrenme algoritmalarının ve daha büyük örnek çaplarının kullanılması, tahmininperformansını artırabilir. Değişik sekiz öğrenme algoritması ile eğitilen farklı yapay sinir ağı modelleri, KAH’ın tahmin edilmesinde ümit verici sonuçlar vermektedir ve ileriye yönelik klinik tanı sürecinde kullanılabilir.
Anahtar Kelime: Belge Türü: Makale Makale Türü: Araştırma Makalesi Erişim Türü: Erişime Açık
- 1.Haykin S. Neural networks: a comprehensive foundation. New York; Macmillan College Publishing Company: 1994.
- 2.Maren A, Harston C, Pap R. Handbook of neural computing applications. London: Academic Press; 1990.
- 3.Eapen BR. ‘Neural network’ algorithm to predict severity in epidermolysis bullosa simplex. Indian J Dermatol Venereol Leprol 2005; 71: 106-8.
- 4.Allison JS, Heo J, Iskandrian AE. Artificial neural network modeling of stress single-photon emission computed tomographic imaging for detecting extensive coronary artery disease. Am J Cardiol 2005;95: 178-81.
- 5.Dubey AK. Using rough sets, neural networks, and logistic regression to predict compliance with cholesterol guidelinesgoals in patients with coronary artery disease. AMIA Ann Symp Proc 2003; 834.
- 6.Scott JA, Aziz K, Yasuda T, Gewirtz H. Integration of clinical and imaging data to predict the presence of coronary artery disease with the use of neural networks. Coron Artery Dis 2004; 15: 427-34.
- 7.Tham CK, Heng CK, Chin WC. Predicting risk of coronary artery disease from DNA microarray-based genotyping using neural networks and other statistical analysis tool. J Bioinform Comput Biol. 2003; 1: 521-39.
- 8.Chobanian AV, Bakris GL, Black HR, Cushman WC, Green LA, IzzoJL Jr, et al. National heart, lung, and blood institute joint nationalcommittee on prevention, detection, evaluation, and treatment ofhigh blood pressure; national high blood pressure education program coordinating committee. JAMA 2003; 289: 2560-72.
- 9. Alberti KG, Zimmet PZ. New diagnostic criteria and classification of diabetes-again? Diabet Med 1998; 15: 535–6.
- 10. Report of the expert committee on the diagnosis and classification of diabetes mellitus. Diabetes Care 1997; 20: 1183–97.
- 11. Clinical guidelines on the identification, evaluation, and treatment of overweight and obesity in adults: the evidence report. National Institutes of Health. Obes Res 1998; 6: 51S-209S.
- 12. Onat A. Risk Factors and cardiovascular disease in Turkey. Atherosclerosis 2001; 156: 1-10.
- 13. Linlon MF, Fazio S. A practical approach to risk assessment to prevent coronary artery disease and its complications. Am J Cardiol 2003; 92: 191-261.
- 14. Kim HK, Chang SA, Choi EK, Kim YJ, Kim HS, Sohn DW, et al. Association between plasma lipids, and apolipoproteins and coronary artery disease: a cross-sectional study in a low-risk Korean population. Int J Cardiol 2005; 101: 435-40.
- 15. Levenberg K. A method for the solution of certain nonlinear problems in least squares. Quart Appl Math 1944; 2: 164-8.
- 16. Marquardt DW. An algorithm for least-squares estimation of nonlinear parameters. J Soc Ind Appl Math 1963; 11; 431-41.
- 17. Gill PE, Murray W, Wright MH. Practical optimization. New York: Academic Press; 1981.
- 18. Battiti R. First and second order methods for learning: between steepest descent and Newton's method. Neur Comp 1992; 4: 141-66.
- 19. Powell MJD. Restart procedures for the conjugate gradien tmethod. Math Progr 1977; 12: 241-54.
- 20. Scales LE. Introduction to non-linear optimization. New York: Springer-Verlag; 1985.
- 21. Rumelhart DE, McClelland JL. Parallel distributed processing. Cambridge: The MIT Press; 1986.
- 22. Principe J, Euliano NR, Lefebvre WC. Neural and adaptive systems: fundamentals through simulations. New York: John Wiley & SonsInc; 1999.
- 23. Maseri A. Ischemic heart disease. In a rational basis for clinical practice and research. New York: Churchill Livingstone; 1995.
- 24. Murray CJ, Lopez AD. Mortality by cause for eight regions of the world: Global Burden of Disease Study. Lancet 1997; 349: 1269-76.
- 25. Bonow RO, Smaha LA, Smith SC Jr, Mensah GA, Lenfant C. World Heart Day 2002; The International burden of cardiovascular disease-responding to the emerging global epidemic. Circulation 2002; 106; 1602-5.
- 26. Gibbons RJ, Balady GJ, Bricker JT, Chaitman BR, Fletcher GF,Froelicher VF, et al. ACC/AHA 2002 Guideline update for exercise testing: a report of The American College of Cardiology /American Heart Association Task Force on Practice Guideline (Committee onExercise Testing) J Am Coll Cardiol 2002; 40: 1531-40.
- 27. Alexander C, Oberhausen E. Myocardial scintigraphy. Semin Nucl Med 1995; 25: 195-201.
- 28. Beller GA. Current status of nuclear cardiology techniques. Curr Probl Cardiol 1991:16:451-535.
- 29. Fleischmann KE, Hunink MG, Kuntz KM, Douglas PS. Exercise echocardiography or exercise SPECT imaging? A meta-analysis of diagnostic test performance. JAMA 1998; 280: 913-20.
- 30. Bonow RO. Identification of viable myocardium. Circulation 1996: 94: 2674-80.
- 31. Nandalur KR, Dwamena BA, Chodhri AF, Nandalur MR, Carlos RC. Diagnostic performance of stress cardiac mangnetic resonance imaging in the detection of coronary artery disease: a meta-analysis. J Am Coll Cardiol 2007; 50: 1343-53.
- 32. Krause KJ. Cost-benefit analysis of electron beam CT as a life insurance coronary disease risk assessment tool. J Insur Med 2003; 35: 183-92.
- 33. Bigi R, Gregori D, Cortigiani L, Desideri A, Chiarotto FA, Toffolo GM. Artificial neural networks and robust Bayesian classifiers for risk stratification following uncomplicated myocardial infarction. Int J Cardiol 2005; 101: 481-7.
- 34. Lindahl D, Toft J, Hesse B, Palmer J, Ali S, Lundin A, et al. Scandinavian test of artificial neural network for classification of myocardial perfusion images. Clin Physiol 2000; 20: 253-61.
- 35. Mobley BA, Schechter E, Moore WE, McKee PA, Eichner JE. Predictions of coronary artery stenosis by artificial neural network. Artif Intell Med 2000; 18: 187-203.
- 36. Lindahl D, Palmer J, Edenbrandt L. Myocardial SPET: artificial neural networks describe extent and severity of perfusion defects.Clin Physiol 1999; 19: 497-503
APA | ÇOLAK M, ÇOLAK C, KOCATÜRK H, SAGIROGLU S, BARUTÇU İ (2008). Predicting coronary artery disease using different artificial neural network models. , 249 - 254. |
Chicago | ÇOLAK M. Cengiz,ÇOLAK Cemil,KOCATÜRK Hasan,SAGIROGLU SEREF,BARUTÇU İrfan Predicting coronary artery disease using different artificial neural network models. (2008): 249 - 254. |
MLA | ÇOLAK M. Cengiz,ÇOLAK Cemil,KOCATÜRK Hasan,SAGIROGLU SEREF,BARUTÇU İrfan Predicting coronary artery disease using different artificial neural network models. , 2008, ss.249 - 254. |
AMA | ÇOLAK M,ÇOLAK C,KOCATÜRK H,SAGIROGLU S,BARUTÇU İ Predicting coronary artery disease using different artificial neural network models. . 2008; 249 - 254. |
Vancouver | ÇOLAK M,ÇOLAK C,KOCATÜRK H,SAGIROGLU S,BARUTÇU İ Predicting coronary artery disease using different artificial neural network models. . 2008; 249 - 254. |
IEEE | ÇOLAK M,ÇOLAK C,KOCATÜRK H,SAGIROGLU S,BARUTÇU İ "Predicting coronary artery disease using different artificial neural network models." , ss.249 - 254, 2008. |
ISNAD | ÇOLAK, M. Cengiz vd. "Predicting coronary artery disease using different artificial neural network models". (2008), 249-254. |
APA | ÇOLAK M, ÇOLAK C, KOCATÜRK H, SAGIROGLU S, BARUTÇU İ (2008). Predicting coronary artery disease using different artificial neural network models. Anadolu Kardiyoloji Dergisi, 8(4), 249 - 254. |
Chicago | ÇOLAK M. Cengiz,ÇOLAK Cemil,KOCATÜRK Hasan,SAGIROGLU SEREF,BARUTÇU İrfan Predicting coronary artery disease using different artificial neural network models. Anadolu Kardiyoloji Dergisi 8, no.4 (2008): 249 - 254. |
MLA | ÇOLAK M. Cengiz,ÇOLAK Cemil,KOCATÜRK Hasan,SAGIROGLU SEREF,BARUTÇU İrfan Predicting coronary artery disease using different artificial neural network models. Anadolu Kardiyoloji Dergisi, vol.8, no.4, 2008, ss.249 - 254. |
AMA | ÇOLAK M,ÇOLAK C,KOCATÜRK H,SAGIROGLU S,BARUTÇU İ Predicting coronary artery disease using different artificial neural network models. Anadolu Kardiyoloji Dergisi. 2008; 8(4): 249 - 254. |
Vancouver | ÇOLAK M,ÇOLAK C,KOCATÜRK H,SAGIROGLU S,BARUTÇU İ Predicting coronary artery disease using different artificial neural network models. Anadolu Kardiyoloji Dergisi. 2008; 8(4): 249 - 254. |
IEEE | ÇOLAK M,ÇOLAK C,KOCATÜRK H,SAGIROGLU S,BARUTÇU İ "Predicting coronary artery disease using different artificial neural network models." Anadolu Kardiyoloji Dergisi, 8, ss.249 - 254, 2008. |
ISNAD | ÇOLAK, M. Cengiz vd. "Predicting coronary artery disease using different artificial neural network models". Anadolu Kardiyoloji Dergisi 8/4 (2008), 249-254. |