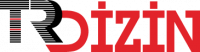
A Spectral Band Based Comparison of Unsupervised Segmentation Evaluation Methods for Image Segmentation Parameter Optimization
Yıl: 2020 Cilt: 7 Sayı: 2 Sayfa Aralığı: 132 - 139 Metin Dili: İngilizce DOI: 10.30897/ ijegeo.641216 İndeks Tarihi: 21-10-2020
A Spectral Band Based Comparison of Unsupervised Segmentation Evaluation Methods for Image Segmentation Parameter Optimization
Öz: Very high-resolution images obtained with recently launched satellite sensors have been used intensively in the remote sensing area. The widespread use of high-resolution images has greatly facilitated the creation and updating of land use/land cover (LULC) maps. Traditional pixel-based image analysis methods that extract information based solely on the spectral values of pixels are generally notsuitable for high-resolution images. Unlike pixel-based approaches, object-based image analysis (OBIA) uses pixel clustering (image objects) instead of pixels by considering the shape, texture, context and spectral features and provides richer information extraction. Image segmentation is an important process and prerequisite for the OBIA process. It is essential to evaluate the performance of segmentation algorithms for the determination of effective segmentation methods and optimization of segmentation parameters. In this study, the multi-resolution segmentation algorithm is used for the segmentation process. The effect of spectral bands onsegmentation quality was analysed using a Worldview-2 high-resolution satellite image. In order to analyse segmentation quality, two unsupervised quality metrics, namely, F-measure and Plateau Objective Function (POF) values were calculated for each band separately. In this manner, optimum parameter values were determined using different variations of Moran's I Index and variancevalues. Image segmentation was performed by using different scale, shape and compactness parameter values. In this context, 30 segmentation analyses were performed considering three different spectral bands (red, green and near-infrared bands). The results showed that the highest segmentation quality was acquired for the NIR band among the spectral bands for the F-measure method,while the highest segmentation quality value was achieved for the green band for the POF metric. In addition, the optimum segmentation parameter values of the scale, shape and compactness were determined as 30-0.3-0.5 and 50-0.1-0.3, for F-measure and POF approaches, respectively
Anahtar Kelime: Belge Türü: Makale Makale Türü: Araştırma Makalesi Erişim Türü: Erişime Açık
- Aguilar, M.A., Aguilar, F.J., García Lorca, A., Guirado, E., Betlej, M., Cichon, P., Nemmaoui, A., Vallario, A., Parente, C. (2016). Assessment of multiresolution seg-mentation for extracting greenhouses from WorldView-2 imagery. International Archives of the Photogrammetry, Remote Sensing and Spatial Information Sciences (ISPRS archives) 41, 145-152.
- Algancı, U , Sertel, E , Kaya, Ş. (2018). Determination of the Olive Trees with Object Based Classification of Pleiades Satellite Image. International Journal of Environment and Geoinformatics, 5 (2) , 132-139. DOI: 10.30897/ijegeo.396713
- Baatz, M., Schape, A. (2000). Multiresolution segmentation – An optimization approach for high quality multi-scale image segmentation. In: Strobl J. et al. (Eds.), Angewandte Geographische Informationsverarbeitung (pp. 12–23), Herbert Wichmann Verlag.
- Blaschke, T. (2010). Object based image analysis for remote sensing. ISPRS Journal of Photogrammetry and Remote Sensing, 65(1), 2-16.
- Blaschke, T., Burnett, C., Pekkarinen, A. (2004). New contextual approaches using image segmentation for object-based classification. In: De Meer, F., de Jong, S (Eds.), Remote Sensing Image Analysis: Including the spatial domain (pp. 211–236)., Kluver Academic publishers, Dordrecht.
- Blaschke, T., Lang, S., Hay, G.J. (2008). Object- Based Image Analysis- Spatial concepts for knowledge driven remote sensing applications. Springer, Heidelberg, Berlin, New York.
- Clinton, N., Holt, A., Scarborough, J., Yan, L., Gong, P. (2010). Accuracy assessment measure for objectbased image segmentation goodness. Photogrammetric Engineering and Remote Sensing, 76, 289–299.
- Çölkesen, I., Kavzoğlu, T. (2017) The use of logistic model tree (LMT) for pixel- and object-based classifications using high-resolution WorldView-2 imagery. Geocarto International, 32, 71-86.
- Esetlili, M, Bektas Balcik, F., Balik Sanli, F., Kalkan, K., Ustuner, M., Goksel, C., Gazioğlu, C., Kurucu, Y. (2018). Comparison of Object and Pixel-Based Classifications For Mapping Crops Using Rapideye Imagery: A Case Study Of Menemen Plain, Turkey. International Journal of Environment and Geoinformatics, 5(2), 231-243. DOI: 10.30897/ijegeo.442002
- Espindola, G.M., Camara, G., Reis, I.A., Bins, L.S., Monteiro, A.M. (2006). Parameter selection for region-growing image segmentation algorithms using spatial autocorrelation. International Journal of Remote Sensing, 27(14), 3035–3040.
- Gao, Y., Mas, J. F., Kerle, N., Navarrete Pacheco, J. A. (2011). Optimal region growing segmentation and its effect on classification accuracy. International Journal of Remote Sensing, 32(13), 3747-3763.
- Grybas, H., Melendy, L., Congalton, R.G. (2017). A comparison of unsupervised segmentation parameter optimization approaches using moderate-and highresolution imagery. GIScience Remote Sensing, 54(4), 515-533.
- Hossain, M.D., Chen, D. (2019). Segmentation for object-based image analysis (OBIA): A review of algorithms and challenges from remote sensing perspective. ISPRS Journal of Photogrammetry and Remote Sensing, 150, 115-134.
- Jensen, J.R. (2016). Introductory digital image processing: a remote sensing perspective. 4th ed. Upper Saddle River, NJ: Pearson Prentice Hall.
- Johnson, B., Bragais, M., Endo, I., Magcale-Macandog, D., Macandog, P. (2015). Image segmentation parameter optimization considering within- and between-segment heterogeneity at multiple scale levels: test case for mapping residential areas using Landsat imagery. ISPRS International Journal of Geo-Information, 4, 2292-2305.
- Johnson, B., Xie, Z. (2011). Unsupervised image segmentation evaluation and refinement using a multi- scale approach. ISPRS Journal of Photogrammetry and Remote Sensing, 66(4), 473- 483.
- Kavzoğlu, T. (2017). Object-Oriented Random Forest for High Resolution Land Cover Mapping Using Quickbird-2 Imagery. In: Samui, P., Sekhar, S., Balas, V. E. (Eds.), Handbook of Neural Computation (pp. 607-619), Elsevier.
- Kavzoğlu, T., Çölkesen, I. (2013). An assessment of the effectiveness of a Rotation Forest ensemble for landuse and land-cover mapping. International Journal of Remote Sensing, 34(12), 4224-4241.
- Kavzoğlu, T., Tonbul H. (2018). An Experimental Comparison of Multi-Resolution Segmentation, SLIC and K-Means Clustering for Object-Based Classification of VHR Imagery. International Journal of Remote Sensing, 39(18), 6020-6036.
- Kavzoğlu, T., Yildiz Erdemir, M. and Tonbul, H. (2017). Classification of semiurban landscapes from very high-resolution satellite images using a regionalized multiscale segmentation approach. Journal of Applied Remote Sensing, 11(3), 035016.
- Martha, T.R., Kerle, N., van Westen, C.J., Jetten, V., Kumar, K.V. (2011). Segment optimization and datadriven thresholding for knowledge-based landslide detection by object-based image analysis. IEEE Transactions on Geoscience and Remote Sensing, 49, 4928-4943.
- Saba, F., Zoej, M.J.V., Mokhtarzade, M. (2016). Optimization of Multiresolution Segmentation for Object-Oriented Road Detection from HighResolution Images. Canadian Journal of Remote Sensing, 42, 75-84.
- Su, T. (2019). Scale-variable region-merging for high resolution remote sensing image segmentation. ISPRS Journal of Photogrammetry and Remote Sensing, 147, 319-334.
- Tonbul, H., Kavzoğlu, T. (2019). Application of Taguchi Optimization and ANOVA Statistics in Optimal Parameter Setting of MultiResolution Segmentation. Proc. Symposium on 9th Recent Advances in Space Technologies (RAST), Istanbul, Turkey, 387-391.
- Yang, J., He, Y., Weng, Q. (2015). An automated method to parameterize segmentation scale by enhancing intrasegment homogeneity and intersegment heterogeneity. IEEE Geoscience Remote Sensing Letters, 12 (6), 1282-1286.
- Zhang, H., Fritts, J., Goldman, S. (2008). Image segmentation evaluation: a survey of unsupervised methods. Computer Vision and Image Understanding, 110(2), 260-280.
- Zhang, Y.J., 1996, A survey on evaluation methods for image segmentation. Pattern Recognition, 29(8), 1335-1346..
APA | TONBUL H, KAVZOĞLU T (2020). A Spectral Band Based Comparison of Unsupervised Segmentation Evaluation Methods for Image Segmentation Parameter Optimization. , 132 - 139. 10.30897/ ijegeo.641216 |
Chicago | TONBUL Hasan,KAVZOĞLU Taşkın A Spectral Band Based Comparison of Unsupervised Segmentation Evaluation Methods for Image Segmentation Parameter Optimization. (2020): 132 - 139. 10.30897/ ijegeo.641216 |
MLA | TONBUL Hasan,KAVZOĞLU Taşkın A Spectral Band Based Comparison of Unsupervised Segmentation Evaluation Methods for Image Segmentation Parameter Optimization. , 2020, ss.132 - 139. 10.30897/ ijegeo.641216 |
AMA | TONBUL H,KAVZOĞLU T A Spectral Band Based Comparison of Unsupervised Segmentation Evaluation Methods for Image Segmentation Parameter Optimization. . 2020; 132 - 139. 10.30897/ ijegeo.641216 |
Vancouver | TONBUL H,KAVZOĞLU T A Spectral Band Based Comparison of Unsupervised Segmentation Evaluation Methods for Image Segmentation Parameter Optimization. . 2020; 132 - 139. 10.30897/ ijegeo.641216 |
IEEE | TONBUL H,KAVZOĞLU T "A Spectral Band Based Comparison of Unsupervised Segmentation Evaluation Methods for Image Segmentation Parameter Optimization." , ss.132 - 139, 2020. 10.30897/ ijegeo.641216 |
ISNAD | TONBUL, Hasan - KAVZOĞLU, Taşkın. "A Spectral Band Based Comparison of Unsupervised Segmentation Evaluation Methods for Image Segmentation Parameter Optimization". (2020), 132-139. https://doi.org/10.30897/ ijegeo.641216 |
APA | TONBUL H, KAVZOĞLU T (2020). A Spectral Band Based Comparison of Unsupervised Segmentation Evaluation Methods for Image Segmentation Parameter Optimization. International Journal of Environment and Geoinformatics, 7(2), 132 - 139. 10.30897/ ijegeo.641216 |
Chicago | TONBUL Hasan,KAVZOĞLU Taşkın A Spectral Band Based Comparison of Unsupervised Segmentation Evaluation Methods for Image Segmentation Parameter Optimization. International Journal of Environment and Geoinformatics 7, no.2 (2020): 132 - 139. 10.30897/ ijegeo.641216 |
MLA | TONBUL Hasan,KAVZOĞLU Taşkın A Spectral Band Based Comparison of Unsupervised Segmentation Evaluation Methods for Image Segmentation Parameter Optimization. International Journal of Environment and Geoinformatics, vol.7, no.2, 2020, ss.132 - 139. 10.30897/ ijegeo.641216 |
AMA | TONBUL H,KAVZOĞLU T A Spectral Band Based Comparison of Unsupervised Segmentation Evaluation Methods for Image Segmentation Parameter Optimization. International Journal of Environment and Geoinformatics. 2020; 7(2): 132 - 139. 10.30897/ ijegeo.641216 |
Vancouver | TONBUL H,KAVZOĞLU T A Spectral Band Based Comparison of Unsupervised Segmentation Evaluation Methods for Image Segmentation Parameter Optimization. International Journal of Environment and Geoinformatics. 2020; 7(2): 132 - 139. 10.30897/ ijegeo.641216 |
IEEE | TONBUL H,KAVZOĞLU T "A Spectral Band Based Comparison of Unsupervised Segmentation Evaluation Methods for Image Segmentation Parameter Optimization." International Journal of Environment and Geoinformatics, 7, ss.132 - 139, 2020. 10.30897/ ijegeo.641216 |
ISNAD | TONBUL, Hasan - KAVZOĞLU, Taşkın. "A Spectral Band Based Comparison of Unsupervised Segmentation Evaluation Methods for Image Segmentation Parameter Optimization". International Journal of Environment and Geoinformatics 7/2 (2020), 132-139. https://doi.org/10.30897/ ijegeo.641216 |