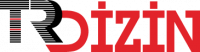
Akciğer nodül özelliklerinin tahmininde çeşitli sınıflama stratejilerinin incelenmesi
Yıl: 2019 Cilt: 34 Sayı: 2 Sayfa Aralığı: 709 - 725 Metin Dili: Türkçe DOI: 10.17341/gazimmfd.416530 İndeks Tarihi: 30-12-2020
Akciğer nodül özelliklerinin tahmininde çeşitli sınıflama stratejilerinin incelenmesi
Öz: Nodül özellikleri, akciğer nodüllerinin değerlendirilmesinde kullanılan, genelde uzman görüşüne göre özneldeğerlendirmelerdir. Bunlardan en bilineni ve çalışmalarda tahmin için en çok kullanılanı habislikderecesidir. Literatürde yapılan sınıflama çalışmalarında, nodül görünümü ve morfolojik yapısı üzerindençıkarılan geleneksel özniteliklerin yanında derin öznitelikler de kullanılmaktadır. Bu çalışmada gelenekselöznitelikler, derin öznitelikler ve bunların kombinasyonları nodül özelliklerinin tahmininde kullanılmıştır.Dört farklı yapıdaki sınıflama algoritması nodül özelliklerinin tahmini için değerlendirilmiştir. Nodülözelliklerinin referans veri kümeleri, doktorların öznel değerlendirmelerinden çoğunluk oylama yöntemi ileoluşturulmuştur. Oluşturulan bu veri kümeleri büyük oranda dengesiz sınıf dağılımına sahiptir. Bu durumunsınıflama sonuçlarına etkisini incelemek için veri dengeleme prosedürü uygulanmıştır. Bu tüm yöntemlerinkombinasyonlarıyla, farklı sınıflama modellerinin sınıflama başarımına, duyarlılığa ve belirliliğe etkileriincelenmiştir. Deneyler sonucunda, ulaşılmak istenen başarım kriterine göre, kullanılacak özniteliklerdensınıflama algoritmasına kadar, sınıflama stratejisinin özel olarak belirlenmesi gerektiği tespit edilmiştir.
Anahtar Kelime: Examination of various classification strategies in classification of lung nodule characteristics
Öz: Nodule characteristics used in the evaluation of lung nodules are generally subjective assessments of theexpert opinions. Among these characteristics, the most known and used one for prediction is the degree of malignancy. In the classification studies in the literature, deep features are used besides the traditional features extracted from the nodule appearance and morphological structure. In this study, traditional features, deep features, and the combinations both used in predicting the nodule characteristics. Four classification algorithms with different structures are evaluated for predicting nodule characteristics. Reference data sets of nodule characteristics were generated by means of majority voting from subjective assessments of radiologists. These generated data sets generally have large unbalanced class distributions. Data balancing procedure has been applied to examine the effect of this condition on classification results. With the combinations of these methods, effects of different classification models on the classification accuracy, sensitivity and specificity are examined. The results of the experiments shown that the classification strategy needs to be specifically determined starting from the used features to the classification algorithm according to the performance criterion to be achieved.
Anahtar Kelime: Belge Türü: Makale Makale Türü: Araştırma Makalesi Erişim Türü: Erişime Açık
- 1. Ferlay J., Soerjomataram I., Dikshit R., Eser S., Mathers C., Rebelo M., Parkin D.M., Forman D., Bray F., Cancer incidence and mortality worldwide: Sources, methods and major patterns in GLOBOCAN 2012, International Journal of Cancer, 136 (5), E359-E386, 2015.
- 2. Austin J.H.M., Muller N.L., Friedman P.J., Hansell D.M., Naidich D.P., RemyJardin,M., Webb,W.R., Zerhouni,E.A., Glossary of terms for CT of the lungs: Recommendations of the Nomenclature Committee of the Fleischner Society, Radiology, 200 (2), 327-331, 1996.
- 3. Armato S.G., McLennan G., Bidaut L., McNitt-Gray M.F., Meyer C.R., Reeves A.P., Zhao, B.S., Aberle, D.R., Henschke C.I., Hoffman E.A., Kazerooni E.A., MacMahon H., van Beek E.J.R., Yankelevitz D., Biancardi A.M., Bland P.H., Brown M.S., Engelmann R.M., Laderach G.E., Max D., Pais R.C., Qing D.P.Y., Roberts R.Y., Smith A.R., Starkey A., Batra P., Caligiuri, P., Farooqi, A., Gladish, G.W., Jude, C.M., Munden, R.F., Petkovska, I., Quint, L.E., Schwartz, L.H., Sundaram B., Dodd, L.E., Fenimore, C., Gur, D., Petrick N., Freymann J., Kirby, J., Hughes, B., Casteele, A.V., Gupte, S., Sallam, M., Heath, M.D., Kuhn, M.H., Dharaiya, E., Burns, R., Fryd, D.S., Salganicoff, M., Anand, V., Shreter, U., Vastagh, S., Croft, B.Y., Clarke, L.P., The Lung Image Database Consortium, (LIDC) and Image Database Resource Initiative (IDRI): A Completed Reference Database of Lung Nodules on CT Scans, Medical Physics, 38 (2), 915-931, 2011.
- 4. van Klaveren R.J., Oudkerk M., Prokop M., Scholten, E.T., Nackaerts, K., Vernhout, R., van Iersel, C.A., van den Bergh K.A.M., van't Westeinde S., van der Aalst C., Thunnissen, E., Xu, D.M., Wang, Y., Zhao, Y.R., Gietema, H.A., de Hoop, B.J., Groen, H.J.M., de Bock, G.H., van Ooijen, P., Weenink, C., Verschakelen, J., Lammers, J.W.J., Timens, W., Willebrand D., Vink, A., Mali, W., de Koning, H.J., Management of Lung Nodules Detected by Volume CT Scanning, New England Journal of Medicine, 361 (23), 2221-2229, 2009.
- 5. Jabon S.A., Raicu D.S., Furst J.D., Content-based versus semantic-based retrieval: an LIDC case study, Medical Imaging 2009: Image Perception, Observer Performance, and Technology Assessment. International Society for Optics and Photonics, 72631L, 2009.
- 6. Junior J.R.F., Oliveira, M.C., de Azevedo-Marques, P.M., Cloud-based NoSQL open database of pulmonary nodules for computer-aided lung cancer diagnosis and reproducible research, Journal of digital imaging, 29 (6), 716-729, 2016.
- 7. Vinay K., Rao, A., Kumar, G.H., Predication of Lung Nodule Characteristic Rating using Best Classifier Model, International Journal of Computer Applications, 56 (18), 2012.
- 8. Zinovev D., Furst J., Raicu D., Building an ensemble of probabilistic classifiers for lung nodule interpretation, IEEE 10th International Conference on Machine Learning and Applications and Workshops, Honolulu, ABD, 155-161, 18-21 Aralık, 2011.
- 9. Zinovev D., Raicu D., Furst J., Armato III S.G., Predicting radiological panel opinions using a panel of machine learning classifiers, Algorithms, 2 (4), 1473- 1502, 2009.
- 10. Kaya A., Can A.B., A weighted rule based method for predicting malignancy of pulmonary nodules by nodule characteristics, Journal of Biomedical Informatics, 56, 69-79, 2015.
- 11. Kaya,A., Can A.B., eFis: A Fuzzy Inference Method for Predicting Malignancy of Small Pulmonary Nodules Image Analysis and Recognition. ICIAR 2014. Lecture Notes in Computer Science, Springer, 8815, 2014.
- 12. Li G., Kim H., Tan, J.K., Ishikawa, S., Hirano, Y., Kido, S., Tachibana, R., Semantic characteristics prediction of pulmonary nodule using Artificial Neural Networks, 35th Annual International Conference of the IEEE Engineering in Medicine and Biology Society, Osaka, Japonya, 5465-5468, 3-7 Haziran, 2013.
- 13. Lee M.C., Boroczky L., Sungur-Stasik, K., Cann, A.D., Borczuk, A.C., Kawut, S.M., Powell, C.A., Computeraided diagnosis of pulmonary nodules using a two-step approach for feature selection and classifier ensemble construction, Artificial Intelligence in Medicine, 50(1) 43-53, 2010.
- 14. Zhao L., Lee M.C., Boroczky L., Vloemans V., Opfer, R., Comparison of computer-aided diagnosis performance and radiologist readings on the LIDC pulmonary nodule dataset, International Society for Optics and Photonics Medical Imaging, 6915, 69151I 2008.
- 15. El-Baz A., Beache, G.M., Gimel'farb, G., Suzuki, K., Okada, K., Elnakib, A., Soliman, A., Abdollahi, B., Computer-aided diagnosis systems for lung cancer: challenges and methodologies, International Journal of Biomedical Imaging, 2013, 942353, 2013.
- 16. Hua K.L., Hsu, C.H., Hidayati, H.C., Cheng, W.H., Chen, Y.J., Computer-aided classification of lung nodules on computed tomography images via deep learning technique, Oncotargets and Therapy, 8, 2015- 2022, 2015.
- 17. Roth H.R., Lu, L., Liu, J.M., Yao, J.H., Seff, A., Cherry, K., Kim, L., Summers, R.M., Improving ComputerAided Detection Using Convolutional Neural Networks and Random View Aggregation, IEEE Transactions on Medical Imaging, 35 (5), 1170-1181, 2016.
- 18. Dou Q., Chen, H., Yu, L.Q., Zhao, L., Qin, J., Wang, D.F., Mok, V.C.T., Shi, L., Heng, P.A., Automatic Detection of Cerebral Microbleeds From MR Images via 3D Convolutional Neural Networks, IEEE Transactions on Medical Imaging, 35 (5), 1182-1195, 2016.
- 19. Cheng J.Z., Ni, D., Chou, Y.H., Qin, J., Tiu, C.M., Chang, Y.C., Huang, C.S., Shen, D., Chen, C.M., Computer-Aided Diagnosis with Deep Learning Architecture: Applications to Breast Lesions in US Images and Pulmonary Nodules in CT Scans, Scientific Reports, 6, 2016.
- 20. Pan W.K., A survey of transfer learning for collaborative recommendation with auxiliary data, Neurocomputing, 177, 447-453, 2016.
- 21. Shin H.C., Roth, H.R., Gao, M.C., Lu, L., Xu, Z.Y., Nogues, I., Yao, J.H., Mollura, D., Summers, R.M., Deep Convolutional Neural Networks for ComputerAided Detection: CNN Architectures, Dataset Characteristics and Transfer Learning, IEEE Transactions on Medical Imaging, 35 (5), 1285-1298, 2016.
- 22. Deng J., Dong, W., Socher, R., Li, L.J., Li, K., Li, F.F., ImageNet: A Large-Scale Hierarchical Image Database, IEEE Conference on Computer Vision and Pattern Recognition, 248-255, Miami, ABD, 20-25 Haziran, 2009.
- 23. Krizhevsky A., Sutskever, I., Hinton, G.E., Imagenet classification with deep convolutional neural networks, 25th International Conference on Neural Information Processing Systems, Siem Reap, Kamboçya, 1097- 1105, 14-16 Aralık, 2012.
- 24. Cortes C., Vapnik, V., Support-Vector Networks, Machine Learning, 20 (3), 273-297, 1995. 25. Breiman L., Random forests, Machine Learning, 45 (1), 5-32, 2001.
- 26. Fix E., Hodges, J.L., Discriminatory Analysis - Nonparametric Discrimination - Consistency Properties, International Statistical Review, 57 (3), 238-247, 1989.
- 27. Freund Y., Schapire, R.E., A decision-theoretic generalization of on-line learning and an application to boosting, Journal of Computer and System Sciences, 55 (1), 119-139, 1997.
- 28. He H., Bai, Y., Garcia, E.A., Li, S., ADASYN: Adaptive synthetic sampling approach for imbalanced learning, IEEE International Joint Conference on Neural Networks, (IEEE World Congress on Computational Intelligence), Hong Kong, Çin, 1-8 Haziran, 2008.
- 29. Haralick R.M., Shapiro, L.G., Computer and Robot Vision, 1st Edition, Addison-Wesley, 1992.
- 30. Shen W., Zhou, M., Yang, F., Dong, D., Yang, C., Zang, Y., Tian, J., Learning from Experts: Developing Transferable Deep Features for Patient-Level Lung Cancer Prediction, Medical Image Computing and Computer-Assisted Intervention – MICCAI 2016: 19th International Conference, Atina, Yunanistan, 124-131, 17-21 Kasım, 2016.
- 31. Raykar V.C., Yu, S.P., Zhao, L.H., Valadez, G.H., Florin, C., Bogoni, L., Moy, L., Learning From Crowds, Journal of Machine Learning Research, 11, 1297-1322, 2010.
- 32. Kononenko I., Simec, E., RobnikSikonja, M., Overcoming the myopia of inductive learning algorithms with RELIEFF, Applied Intelligence, 7 (1), 39-55, 1997.
- 33. Celik C., Bilge, H.S., Feature Selection with Weighted Conditional Mutual Information, Journal of the Faculty of Engineering and Architecture of Gazi University, 30 (4), 585-596, 2015.
- 34. Akben S.B., Alkan A., Density-Based Feature Extraction to Improve the Classification Performance in the Datasets Having Low Correlation between Attributes, Journal of the Faculty of Engineering and Architecture of Gazi University, 30 (4), 597-603, 2015.
- 35. Ochs R., Kim H.J., Angel, E., Panknin, C., McNittGraya, M., Brown, M., Forming a reference standard from LIDC data: Impact of reader agreement on reported CAD performance - art. no. 65142A, Medical Imaging 2007: Computer-Aided Diagnosis, Pts 1 and 2, 6514, A5142-A5142, 2007.
- 36. Xie Y., Zhang, J., Xia, Y., Fulham, M., Zhang, Y., Fusing texture, shape and deep model-learned information at decision level for automated classification of lung nodules on chest CT, Information Fusion, 42, 102-110, 2018.
APA | Kaya A, Keçeli A, Can A (2019). Akciğer nodül özelliklerinin tahmininde çeşitli sınıflama stratejilerinin incelenmesi. , 709 - 725. 10.17341/gazimmfd.416530 |
Chicago | Kaya Aydın,Keçeli Ali Seydi,Can Ahmet Burak Akciğer nodül özelliklerinin tahmininde çeşitli sınıflama stratejilerinin incelenmesi. (2019): 709 - 725. 10.17341/gazimmfd.416530 |
MLA | Kaya Aydın,Keçeli Ali Seydi,Can Ahmet Burak Akciğer nodül özelliklerinin tahmininde çeşitli sınıflama stratejilerinin incelenmesi. , 2019, ss.709 - 725. 10.17341/gazimmfd.416530 |
AMA | Kaya A,Keçeli A,Can A Akciğer nodül özelliklerinin tahmininde çeşitli sınıflama stratejilerinin incelenmesi. . 2019; 709 - 725. 10.17341/gazimmfd.416530 |
Vancouver | Kaya A,Keçeli A,Can A Akciğer nodül özelliklerinin tahmininde çeşitli sınıflama stratejilerinin incelenmesi. . 2019; 709 - 725. 10.17341/gazimmfd.416530 |
IEEE | Kaya A,Keçeli A,Can A "Akciğer nodül özelliklerinin tahmininde çeşitli sınıflama stratejilerinin incelenmesi." , ss.709 - 725, 2019. 10.17341/gazimmfd.416530 |
ISNAD | Kaya, Aydın vd. "Akciğer nodül özelliklerinin tahmininde çeşitli sınıflama stratejilerinin incelenmesi". (2019), 709-725. https://doi.org/10.17341/gazimmfd.416530 |
APA | Kaya A, Keçeli A, Can A (2019). Akciğer nodül özelliklerinin tahmininde çeşitli sınıflama stratejilerinin incelenmesi. Gazi Üniversitesi Mühendislik Mimarlık Fakültesi Dergisi, 34(2), 709 - 725. 10.17341/gazimmfd.416530 |
Chicago | Kaya Aydın,Keçeli Ali Seydi,Can Ahmet Burak Akciğer nodül özelliklerinin tahmininde çeşitli sınıflama stratejilerinin incelenmesi. Gazi Üniversitesi Mühendislik Mimarlık Fakültesi Dergisi 34, no.2 (2019): 709 - 725. 10.17341/gazimmfd.416530 |
MLA | Kaya Aydın,Keçeli Ali Seydi,Can Ahmet Burak Akciğer nodül özelliklerinin tahmininde çeşitli sınıflama stratejilerinin incelenmesi. Gazi Üniversitesi Mühendislik Mimarlık Fakültesi Dergisi, vol.34, no.2, 2019, ss.709 - 725. 10.17341/gazimmfd.416530 |
AMA | Kaya A,Keçeli A,Can A Akciğer nodül özelliklerinin tahmininde çeşitli sınıflama stratejilerinin incelenmesi. Gazi Üniversitesi Mühendislik Mimarlık Fakültesi Dergisi. 2019; 34(2): 709 - 725. 10.17341/gazimmfd.416530 |
Vancouver | Kaya A,Keçeli A,Can A Akciğer nodül özelliklerinin tahmininde çeşitli sınıflama stratejilerinin incelenmesi. Gazi Üniversitesi Mühendislik Mimarlık Fakültesi Dergisi. 2019; 34(2): 709 - 725. 10.17341/gazimmfd.416530 |
IEEE | Kaya A,Keçeli A,Can A "Akciğer nodül özelliklerinin tahmininde çeşitli sınıflama stratejilerinin incelenmesi." Gazi Üniversitesi Mühendislik Mimarlık Fakültesi Dergisi, 34, ss.709 - 725, 2019. 10.17341/gazimmfd.416530 |
ISNAD | Kaya, Aydın vd. "Akciğer nodül özelliklerinin tahmininde çeşitli sınıflama stratejilerinin incelenmesi". Gazi Üniversitesi Mühendislik Mimarlık Fakültesi Dergisi 34/2 (2019), 709-725. https://doi.org/10.17341/gazimmfd.416530 |