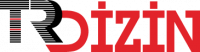
Derin öğrenme yöntemleriyle dermoskopi görüntülerinden melanom tespiti: Kapsamlı bir çalışma
Yıl: 2019 Cilt: 34 Sayı: 4 Sayfa Aralığı: 2241 - 2260 Metin Dili: Türkçe DOI: 10.17341/gazimmfd.435217 İndeks Tarihi: 06-01-2021
Derin öğrenme yöntemleriyle dermoskopi görüntülerinden melanom tespiti: Kapsamlı bir çalışma
Öz: Cilt kanseri yaygın görülen ve tedavi edilmemesi durumunda ölüme neden olan ciddi bir hastalıktır.Melanom ise nadir görülmesine rağmen ölüme en çok neden olan cilt kanseri türüdür. Tüm hastalıklardaolduğu gibi cilt kanserinin erken ve doğru tespit edilmesi hayati önem taşımaktadır. Bilgisayar destekli tanısistemleri cilt kanseri tespitinde hekimlere ve hastalara yardımcı olabilir. Bilgisayar destekli tanısistemlerinde özellikle makine öğrenmesi ve derin öğrenme modelleri etkin bir şekilde kullanılmaktadır.Gerçekleştirilen bu çalışmada cilt kanseri türü olan melanom için otomatik tanı koyabilecek bir sistemönerilmektedir. Melanom tanısı için tasarlanan C4Net derin sinir ağ modeli ile beraber literatürde ön planaçıkmış AlexNet, GoogLeNet, ResNet, VGGNet derin öğrenme modelleri ve Yapay sinir ağları, En yakınkomşu algoritması ve Destek vektör makinesi gibi geleneksel makine öğrenme yöntemlerini de kapsayandetaylı bir deneysel çalışma yapılmıştır. Gerçekleştirilen deneysel çalışmalarda, tasarlanan C4Net derin sinirağ modeli %96,94 doğrulukta melanom tanısı koyarak, diğer yöntemlere göre daha yüksek sınıflandırmabaşarısı göstermiştir.
Anahtar Kelime: Melanoma detection from dermoscopy images with deep learning methods: A comprehensive study
Öz: Skin cancer is common and a serious disease, which can lead to death if not treated in time. Melanoma is the rarest and most dangerous type of skin cancer. It causes the most deaths. As in all diseases, early and correct detection of skin cancer are very important. Computer Aided Diagnosis systems can help physicians and patients make better decisions. Especially, machine learning and deep learning use effectively in Computer Aided Diagnosis systems. In this study, an automatic detection system for melanoma is suggested. To illustrate the advantage of the proposed CNN model C4Net, a comprehensive experimental study has been carried out. In addition, the proposed C4Net has been compared with not only the existing deep learning algorithms such as AlexNet, GoogLeNet, ResNet and VGGNet but also conventional machine learning algorithms such as Artificial neural networks, k-Nearest neighbor algorithm and Support vector machine. In experimental studies, C4Net, which is designed as deep neural network model for melanoma detection, has obtained more classification accuracy than other methods with 96.94%.
Anahtar Kelime: Belge Türü: Makale Makale Türü: Araştırma Makalesi Erişim Türü: Erişime Açık
- 1. Halk Sağlığı Genel Müdürlüğü - https://hsgm.saglik.gov.tr. Erişim tarihi Nisan 10, 2018
- 2. Ye, Q. H., Qin, L. X., Forgues, M., He, P., Kim, J. W., Peng, A. C., & Ma, Z. C., Predicting hepatitis B virus– positive metastatic hepatocellular carcinomas using gene expression profiling and supervised machine learning. Nature medicine, 9 (4), 416, 2003.
- 3. Kononenko, I., Machine learning for medical diagnosis: history, state of the art and perspective. Artificial Intelligence in medicine, 23 (1), 89-109, 2001.
- 4. Berikol, G. B., Yildiz, O., & Özcan, İ. T., Diagnosis of acute coronary syndrome with a support vector machine. Journal of medical systems, 40 (4), 84, 2016.
- 5. Litjens, G., Sánchez, C. I., Timofeeva, N., Hermsen, M., Nagtegaal, I., Kovacs, I., & Van Der Laak, J., Deep learning as a tool for increased accuracy and efficiency of histopathological diagnosis. Scientific reports, 6, 26286, 2016
- 6. Liu, S., Cai, W., Pujol, S., Kikinis, R., & Feng, D., Early diagnosis of Alzheimer's disease with deep learning. In Biomedical Imaging (ISBI), 2014 IEEE 11th International Symposium on (pp. 1015-1018), IEEE, 2014.
- 7. Wang, G., Li, W., Zuluaga, M. A., Pratt, R., Patel, P. A., Aertsen, M., Vercauteren, T., Interactive medical image segmentation using deep learning with image-specific fine-tuning. IEEE Transactions on Medical Imaging, 37 (7), 1562-1573, 2018
- 8. Acharya, U. R., Oh, S. L., Hagiwara, Y., Tan, J. H., & Adeli, H., Deep convolutional neural network for the automated detection and diagnosis of seizure using EEG signals. Computers in biology and medicine, 100, 270- 278, 2018
- 9. Shin, H. C., Roth, H. R., Gao, M., Lu, L., Xu, Z., Nogues, I., Summers, R. M., Deep convolutional neural networks for computer-aided detection: CNN architectures, dataset characteristics and transfer learning, IEEE transactions on medical imaging, 35 (5), 1285-1298, 2016.
- 10. Gu, J., Wang, Z., Kuen, J., Ma, L., Shahroudy, A., Shuai, B., Chen, T., Recent advances in convolutional neural networks. Pattern Recognition, 2017.
- 11. Chen, X., Xiang, S., Liu, C. L., & Pan, C. H., Vehicle detection in satellite images by hybrid deep convolutional neural networks, IEEE Geoscience and remote sensing letters, 11 (10), 1797-1801, 2014.
- 12. Pal, A., Garain, U., Chandra, A., Chatterjee, R., & Senapati, S., Psoriasis skin biopsy image segmentation using Deep Convolutional Neural Network, Computer methods and programs in biomedicine, 159, 59-69, 2018.
- 13. Chan, T. H., Jia, K., Gao, S., Lu, J., Zeng, Z., & Ma, Y., PCANet: A simple deep learning baseline for image classification?, IEEE Transactions on Image Processing, 24 (12), 5017-5032, 2015
- 14. Chen, Y., Lin, Z., Zhao, X., Wang, G., & Gu, Y., Deep learning-based classification of hyperspectral data, IEEE Journal of Selected topics in applied earth observations and remote sensing, 7 (6), 2094-2107, 2014.
- 15. Krizhevsky, A., Sutskever, I., & Hinton, G. E., Imagenet classification with deep convolutional neural networks, In Advances in neural information processing systems (pp. 1097-1105), 2012.
- 16. Szegedy, C., Liu, W., Jia, Y., Sermanet, P., Reed, S., Anguelov, D., Rabinovich, A., Going deeper with convolutions. In Proceedings of the IEEE conference on computer vision and pattern recognition (pp. 1-9), 2015.
- 17. Simonyan, K., & Zisserman, A., Very deep convolutional networks for large-scale image recognition. arXiv preprint arXiv:1409.1556, 2014.
- 18. He, K., Zhang, X., Ren, S., & Sun, J., Deep residual learning for image recognition. In Proceedings of the IEEE conference on computer vision and pattern recognition (pp. 770-778), 2016.
- 19. Litjens, G., Kooi, T., Bejnordi, B. E., Setio, A. A. A., Ciompi, F., Ghafoorian, M., Sánchez, C. I., A survey on deep learning in medical image analysis, Medical image analysis, 42, 60-88, 2017
- 20. Esteva, A., Kuprel, B., Novoa, R. A., Ko, J., Swetter, S. M., Blau, H. M., & Thrun, S., Dermatologist-level classification of skin cancer with deep neural networks. Nature, 542 (7639), 115, 2017.
- 21. Codella, N., Cai, J., Abedini, M., Garnavi, R., Halpern, A., & Smith, J. R., Deep learning, sparse coding, and SVM for melanom recognition in dermoscopy images, In International Workshop on Machine Learning in Medical Imaging (pp. 118-126), Springer, Cham, 2015.
- 22. The International Society for Digital Imaging of the Skin (ISDIS) Archive - https://isic-archive.com/. Erişim tarihi Aralık 5, 2017.
- 23. Li, Y., Nie, X., & Huang, R., Web spam classification method based on deep belief networks, Expert Systems with Applications, 96, 261-270, 2018.
- 24. Bengio, Y., Learning deep architectures for AI. Foundations and trends in Machine Learning, 2 (1), 1- 127, 2009.
- 25. LeCun, Y., Bottou, L., Bengio, Y., & Haffner, P., Gradient-based learning applied to document recognition. Proceedings of the IEEE, 86 (11), 2278- 2324, 1998.
- 26. Haralick, R., Shanmugam, K., & Dinstein, I., Textural features for image classification. Systems, Man and Cybernetics, IEEE Transactions on, SMC-3 (6), 610– 621. doi: 10.1109/TSMC.1973.4309314, 1973.
- 27. The Skin Cancer Foundation - SkinCancer.org - https://www.skincancer.org/skin-cancerinformation/melanoma#panel1-5 - Erişim tarihi Nisan 3, 2018.
APA | YILDIZ O (2019). Derin öğrenme yöntemleriyle dermoskopi görüntülerinden melanom tespiti: Kapsamlı bir çalışma. , 2241 - 2260. 10.17341/gazimmfd.435217 |
Chicago | YILDIZ Oktay Derin öğrenme yöntemleriyle dermoskopi görüntülerinden melanom tespiti: Kapsamlı bir çalışma. (2019): 2241 - 2260. 10.17341/gazimmfd.435217 |
MLA | YILDIZ Oktay Derin öğrenme yöntemleriyle dermoskopi görüntülerinden melanom tespiti: Kapsamlı bir çalışma. , 2019, ss.2241 - 2260. 10.17341/gazimmfd.435217 |
AMA | YILDIZ O Derin öğrenme yöntemleriyle dermoskopi görüntülerinden melanom tespiti: Kapsamlı bir çalışma. . 2019; 2241 - 2260. 10.17341/gazimmfd.435217 |
Vancouver | YILDIZ O Derin öğrenme yöntemleriyle dermoskopi görüntülerinden melanom tespiti: Kapsamlı bir çalışma. . 2019; 2241 - 2260. 10.17341/gazimmfd.435217 |
IEEE | YILDIZ O "Derin öğrenme yöntemleriyle dermoskopi görüntülerinden melanom tespiti: Kapsamlı bir çalışma." , ss.2241 - 2260, 2019. 10.17341/gazimmfd.435217 |
ISNAD | YILDIZ, Oktay. "Derin öğrenme yöntemleriyle dermoskopi görüntülerinden melanom tespiti: Kapsamlı bir çalışma". (2019), 2241-2260. https://doi.org/10.17341/gazimmfd.435217 |
APA | YILDIZ O (2019). Derin öğrenme yöntemleriyle dermoskopi görüntülerinden melanom tespiti: Kapsamlı bir çalışma. Gazi Üniversitesi Mühendislik Mimarlık Fakültesi Dergisi, 34(4), 2241 - 2260. 10.17341/gazimmfd.435217 |
Chicago | YILDIZ Oktay Derin öğrenme yöntemleriyle dermoskopi görüntülerinden melanom tespiti: Kapsamlı bir çalışma. Gazi Üniversitesi Mühendislik Mimarlık Fakültesi Dergisi 34, no.4 (2019): 2241 - 2260. 10.17341/gazimmfd.435217 |
MLA | YILDIZ Oktay Derin öğrenme yöntemleriyle dermoskopi görüntülerinden melanom tespiti: Kapsamlı bir çalışma. Gazi Üniversitesi Mühendislik Mimarlık Fakültesi Dergisi, vol.34, no.4, 2019, ss.2241 - 2260. 10.17341/gazimmfd.435217 |
AMA | YILDIZ O Derin öğrenme yöntemleriyle dermoskopi görüntülerinden melanom tespiti: Kapsamlı bir çalışma. Gazi Üniversitesi Mühendislik Mimarlık Fakültesi Dergisi. 2019; 34(4): 2241 - 2260. 10.17341/gazimmfd.435217 |
Vancouver | YILDIZ O Derin öğrenme yöntemleriyle dermoskopi görüntülerinden melanom tespiti: Kapsamlı bir çalışma. Gazi Üniversitesi Mühendislik Mimarlık Fakültesi Dergisi. 2019; 34(4): 2241 - 2260. 10.17341/gazimmfd.435217 |
IEEE | YILDIZ O "Derin öğrenme yöntemleriyle dermoskopi görüntülerinden melanom tespiti: Kapsamlı bir çalışma." Gazi Üniversitesi Mühendislik Mimarlık Fakültesi Dergisi, 34, ss.2241 - 2260, 2019. 10.17341/gazimmfd.435217 |
ISNAD | YILDIZ, Oktay. "Derin öğrenme yöntemleriyle dermoskopi görüntülerinden melanom tespiti: Kapsamlı bir çalışma". Gazi Üniversitesi Mühendislik Mimarlık Fakültesi Dergisi 34/4 (2019), 2241-2260. https://doi.org/10.17341/gazimmfd.435217 |