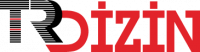
Çekişmeli üretici ağ ile ölçeklenebilir görüntü oluşturma ve süper çözünürlük
Yıl: 2020 Cilt: 35 Sayı: 2 Sayfa Aralığı: 953 - 966 Metin Dili: Türkçe DOI: 10.17341/gazimmfd.587010 İndeks Tarihi: 11-01-2021
Çekişmeli üretici ağ ile ölçeklenebilir görüntü oluşturma ve süper çözünürlük
Öz: Çekişmeli eğitimin, derin öğrenme konusundaki ileri gelen araştırmacılar tarafından son 10 yılın en heyecanverici konularından biri olarak ilan edilmesi ile birlikte birçok araştırmacı, Üretici Çekişmeli Ağ (GAN)üzerine yoğunlaşmıştır. Bu gelişmeler ile birlikte Otokodlayıcı (AE) olarak daha önceden bilinen modelinbir versiyonu olan Varyasyonel Otokodlayıcı (VAE) ile birlikte Otokodlayıcı modeller literatürde yenidenönem kazanmıştır. GAN modellerinin bazı kısıtlamaları ile çıkarım mekanizması gibi bazı eksiklikleri, GANve VAE hibrit modellerini ortaya çıkarmıştır. Bu gelişmelerin etkisiyle, bu çalışmada da bir GAN ve VAEhibrit modeline odaklanılmıştır. Sentetik görüntüler ile eğitim yapılmaksızın istenen çözünürlüktegörüntüleri elde edebilmek üzere, Kompozisyonel Örüntü Üreten Ağlar (CPPN) ile GAN ve VAE modelleribir arada kullanılarak VAE/CPGAN olarak adlandırılan model sunulmuştur. Ayrıca, VAE / GAN hibritmodelinde kullanılan objektif fonksiyonu önerilen model ile bir araya getirilmiştir. Model performanslarınıdeğerlendirmek üzere, deney çalışmalarında VAE/CPGAN modeli, bu modele temel olan GAN, VAE veVAE / GAN modelleri ile karşılaştırılmıştır. Önerilen modelin el yazısı görüntülerinden oluşan popüler verikümesi üzerinde gerçekleştirilen deneyler ile gerçekçi ve süper çözünürlüklü, ölçeklenebilir, sentetikgörüntüler oluşturmadaki başarısı bu çalışma ile ortaya koyulmuştur.
Anahtar Kelime: Scalable image generation and super resolution using generative adversarial networks
Öz: Generative adversarial training has been one of the most active research topics and many researchers have conducted their studies on Generative Adversarial Network (GAN) shortly after it is claimed to be one of the most promising research area of the last decade by pioneers of the deep learning community. On the other hand, the idea behind generators has also reemerged autoencoder models such as Variational Autoencoder (VAE). Therefore, autoencoder models have gained their popularity back. Some restrictions of GAN models such as lack of inference mechanism, GAN and VAE based hybrid models have proposed addressing image generation. With the effect of these notions and studies, we have also considered VAE and GAN hybrid models. For obtaining synthetic but at the same time high-resolution handwritten-looking images without any training, Compositional Pattern Producing Network (CPPN) is adapted from previous studies for combining with VAE and adversarial training. For improving generation capabilities, an objective from a previous VAE/GAN model is also adapted for our VAE/CPGAN hybrid model. For analyzing the proposed model performance, baseline models such as GAN, VAE and VAE/GAN are also evaluated for comparisons. In this paper. it is clearly seen the proposed model is capable of the generating realistic and scalable super resolution synthetic images on a common dataset.
Anahtar Kelime: Belge Türü: Makale Makale Türü: Araştırma Makalesi Erişim Türü: Erişime Açık
- 1. Goodfellow, I., Pouget-Abadie, J., Mirza, M., Xu, B., Warde-Farley, D., Ozair, S., ... & Bengio, Y., Generative adversarial nets, Advances in neural information processing systems, 2014.
- 2. Goodfellow, I. J., Jonathon S., Christian S., Explaining and harnessing adversarial examples, arXiv preprint arXiv:1412.6572, 2014.
- 3. Gauthier, J., Conditional generative adversarial nets for convolutional face generation, Class Project for Stanford CS231N: Convolutional Neural Networks for Visual Recognition, Winter semester, 2014:5, 2014.
- 4. Radford, A., Metz, L., Chintala. S., Unsupervised representation learning with deep convolutional generative adversarial networks, arXiv preprint arXiv:1511.06434, 2015.
- 5. Reed, S., Akata, Z., Yan, X., Logeswaran, L., Schiele, B., Lee, H., Generative adversarial text to image synthesis. In Proceedings of The 33rd International Conference on Machine Learning, 2016.
- 6. Zhang, H., Xu, T., Li, H., Zhang, S., Wang, X., Huang, X. and Metaxas, D.N., Stackgan: Text to photo-realistic image synthesis with stacked generative adversarial networks, In Proceedings of the IEEE International Conference on Computer Vision, 5907-5915, 2017.
- 7. Yeh, R.A., Chen, C., Yian Lim, T., Schwing, A.G., Hasegawa-Johnson, M. and Do, M.N., Semantic image inpainting with deep generative models, In Proceedings of the IEEE Conference on Computer Vision and Pattern Recognition, 5485-5493, 2017.
- 8. Iizuka, S., Simo-Serra, E. and Ishikawa, H., Globally and locally consistent image completion, ACM Transactions on Graphics (ToG), 36 (4), 2017.
- 9. Liu, M.Y., Breuel, T. and Kautz, J., Unsupervised image-to-image translation networks. In Advances in Neural Information Processing Systems, 700-708, 2017.
- 10. Isola, P., Zhu, J.Y., Zhou, T. and Efros, A.A, Image-toimage translation with conditional adversarial networks, In Proceedings of the IEEE conference on computer vision and pattern recognition, 1125-1134, 2017.
- 11. Kingma, D.P. and Welling, M., Auto-encoding variational bayes, arXiv preprint arXiv:1312.6114, 2013.
- 12. Stanley, K.O., Compositional pattern producing networks: A novel abstraction of development. Genetic programming and evolvable machines, 8 (2), pp.131- 162, 2007.
- 13. Mirza, M. and Osindero, S., Conditional generative adversarial nets, arXiv preprint arXiv:1411.1784, 2014.
- 14. Denton, E.L., Chintala, S. and Fergus, R., Deep generative image models using a laplacian pyramid of adversarial networks, In Advances in neural information processing systems, 1486-1494, 2015.
- 15. Radford, A., Metz, L. and Chintala, S., Unsupervised representation learning with deep convolutional generative adversarial networks, arXiv preprint arXiv:1511.06434, 2015.
- 16. Hinton, G.E. and Zemel, R.S., Autoencoders, minimum description length and Helmholtz free energy, In Advances in neural information processing systems, 3- 10, 1994.
- 17. Makhzani, A., Shlens, J., Jaitly, N., Goodfellow, I. and Frey, B., Adversarial autoencoders, arXiv preprint arXiv:1511.05644, 2015.
- 18. Larsen, A.B.L., Sønderby, S.K., Larochelle, H. and Winther, O., Autoencoding beyond pixels using a learned similarity metric, arXiv preprint arXiv:1512.09300, 2015.
- 19. Lamb, A., Dumoulin, V. and Courville, A., Discriminative regularization for generative models, arXiv preprint arXiv:1602.03220, 2016.
- 20. Dosovitskiy, A. and Brox, T., Generating images with perceptual similarity metrics based on deep networks, In Advances in Neural Information Processing Systems, 658-666, 2016.
- 21. Dumoulin, V., Belghazi, I., Poole, B., Mastropietro, O., Lamb, A., Arjovsky, M. and Courville, A.,. Adversarially learned inference, arXiv preprint arXiv:1606.00704, 2016.
- 22. Gulrajani, I., Ahmed, F., Arjovsky, M., Dumoulin, V., & Courville, A. C., Improved training of wasserstein gans, In Advances in neural information processing systems, 5767-5777, 2017.
- 23. Kodali, N., Abernethy, J., Hays, J., & Kira, Z. On convergence and kararlıity of gans. arXiv preprint arXiv:1705.07215, 2017.
- 24. Stanley, K.O. and Miikkulainen, R., Evolving neural networks through augmenting topologies. Evolutionary computation, 10 (2), 99-127, 2002.
- 25. Dong, C., Loy, C.C., He, K. and Tang, X., Learning a deep convolutional network for image super-resolution, In European conference on computer vision, 184-199, 2014.
- 26. Dong, C., Loy, C.C. and Tang, X., Accelerating the super-resolution convolutional neural network, In European conference on computer vision, 391-407, 2016.
- 27. Kim, J., Kwon Lee, J. and Mu Lee, K., Deeply-recursive convolutional network for image super-resolution, In Proceedings of the IEEE conference on computer vision and pattern recognition, 1637-1645, 2016.
- 28. Yamanaka, J., Kuwashima, S. and Kurita, T., Fast and accurate image super resolution by deep CNN with skip connection and network in network, In International Conference on Neural Information Processing, 217-225, 2017.
- 29. Lai, W.S., Huang, J.B., Ahuja, N. and Yang, M.H., Fast and accurate image super-resolution with deep laplacian pyramid networks, IEEE transactions on pattern analysis and machine intelligence, 2018.
- 30. Lim, B., Son, S., Kim, H., Nah, S. and Mu Lee, K., Enhanced deep residual networks for single image super-resolution, In Proceedings of the IEEE Conference on Computer Vision and Pattern Recognition Workshops, 136-144, 2017.
- 31. Johnson, J., Alahi, A. and Fei-Fei, L., Perceptual losses for real-time style transfer and super-resolution, In European conference on computer vision, 694-711, 2016.
- 32. Simonyan, K. and Zisserman, A, Very deep convolutional networks for large-scale image recognition, arXiv preprint arXiv:1409.1556, 2014.
- 33. Kim, J., Kwon Lee, J. and Mu Lee, K., Accurate image super-resolution using very deep convolutional networks, In Proceedings of the IEEE conference on computer vision and pattern recognition, 1646-1654, 2016.
- 34. Ledig, C., Theis, L., Huszár, F., Caballero, J., Cunningham, A., Acosta, A., Aitken, A., Tejani, A., Totz, J., Wang, Z. and Shi, W., Photo-realistic single image super-resolution using a generative adversarial network, In Proceedings of the IEEE conference on computer vision and pattern recognition, 4681-4690, 2017.
- 35. He, K., Zhang, X., Ren, S. and Sun, J., Deep residual learning for image recognition, In Proceedings of the IEEE conference on computer vision and pattern recognition, 770-778, 2016.
- 36. Sajjadi, M.S., Scholkopf, B. and Hirsch, M., Enhancenet: Single image super-resolution through automated texture synthesis, In Proceedings of the IEEE International Conference on Computer Vision, 4491- 4500, 2017.
- 37. Turhan, C. G., Bılge, H. S., Single image super resolution using deep convolutional generative neural networks, In 2018 26th Signal Processing and Communications Applications Conference (SIU), 1-4, 2018.
- 38. Wang, Y., Perazzi, F., McWilliams, B., SorkineHornung, A., Sorkine-Hornung, O., & Schroers, C., A fully progressive approach to single-image superresolution, In Proceedings of the IEEE Conference on Computer Vision and Pattern Recognition Workshops, 864-873, 2018.
- 39. Wang, X., Yu, K., Wu, S., Gu, J., Liu, Y., Dong, C., ... & Change Loy, C., Esrgan: Enhanced super-resolution generative adversarial networks, In Proceedings of the European Conference on Computer Vision (ECCV), 2018.
- 40. Zhang, Y., Li, K., Li, K., Wang, L., Zhong, B., & Fu, Y., Image super-resolution using very deep residual channel attention networks, In Proceedings of the European Conference on Computer Vision (ECCV), 286-301, 2018.
- 41. Li, Z., Yang, J., Liu, Z., Yang, X., Jeon, G., & Wu, W., Feedback Network for Image Super-Resolution, In Proceedings of the IEEE Conference on Computer Vision and Pattern Recognition, 3867-3876, 2019.
- 42. Dai, T., Cai, J., Zhang, Y., Xia, S. T., & Zhang, L., Second-order Attention Network for Single Image Super-Resolution, In Proceedings of the IEEE Conference on Computer Vision and Pattern Recognition, 11065-11074, 2019.
- 43. Secretan, J., Beato, N., D Ambrosio, D.B., Rodriguez, A., Campbell, A. and Stanley, K.O., Picbreeder: evolving pictures collaboratively online, In Proceedings of the SIGCHI Conference on Human Factors in Computing Systems, 1759-1768, 2008.
- 44. LeCun, Y., Cortes, C. and Burges, C.J., MNIST handwritten digit database, AT&T Labs. http://yann. lecun. com/exdb/mnist, 02.18.2010, 03.05.2019.
- 45. Maas, A.L., Hannun, A.Y. and Ng, A.Y., Rectifier nonlinearities improve neural network acoustic models, In Proc. Icml, 30 (1), 3, 2013.
- 46. Kingma, D.P. and Ba, J., A method for stochastic optimization, arXiv preprint arXiv:1412.6980, 2014.
- 47. Salimans, T., Goodfellow, I., Zaremba, W., Cheung, V., Radford, A. and Chen, X, Improved techniques for training gans, In Advances in neural information processing systems, 2234-2242, 2016.
APA | Guzel Turhan C, BILGE H (2020). Çekişmeli üretici ağ ile ölçeklenebilir görüntü oluşturma ve süper çözünürlük. , 953 - 966. 10.17341/gazimmfd.587010 |
Chicago | Guzel Turhan Ceren,BILGE HASAN SAKIR Çekişmeli üretici ağ ile ölçeklenebilir görüntü oluşturma ve süper çözünürlük. (2020): 953 - 966. 10.17341/gazimmfd.587010 |
MLA | Guzel Turhan Ceren,BILGE HASAN SAKIR Çekişmeli üretici ağ ile ölçeklenebilir görüntü oluşturma ve süper çözünürlük. , 2020, ss.953 - 966. 10.17341/gazimmfd.587010 |
AMA | Guzel Turhan C,BILGE H Çekişmeli üretici ağ ile ölçeklenebilir görüntü oluşturma ve süper çözünürlük. . 2020; 953 - 966. 10.17341/gazimmfd.587010 |
Vancouver | Guzel Turhan C,BILGE H Çekişmeli üretici ağ ile ölçeklenebilir görüntü oluşturma ve süper çözünürlük. . 2020; 953 - 966. 10.17341/gazimmfd.587010 |
IEEE | Guzel Turhan C,BILGE H "Çekişmeli üretici ağ ile ölçeklenebilir görüntü oluşturma ve süper çözünürlük." , ss.953 - 966, 2020. 10.17341/gazimmfd.587010 |
ISNAD | Guzel Turhan, Ceren - BILGE, HASAN SAKIR. "Çekişmeli üretici ağ ile ölçeklenebilir görüntü oluşturma ve süper çözünürlük". (2020), 953-966. https://doi.org/10.17341/gazimmfd.587010 |
APA | Guzel Turhan C, BILGE H (2020). Çekişmeli üretici ağ ile ölçeklenebilir görüntü oluşturma ve süper çözünürlük. Gazi Üniversitesi Mühendislik Mimarlık Fakültesi Dergisi, 35(2), 953 - 966. 10.17341/gazimmfd.587010 |
Chicago | Guzel Turhan Ceren,BILGE HASAN SAKIR Çekişmeli üretici ağ ile ölçeklenebilir görüntü oluşturma ve süper çözünürlük. Gazi Üniversitesi Mühendislik Mimarlık Fakültesi Dergisi 35, no.2 (2020): 953 - 966. 10.17341/gazimmfd.587010 |
MLA | Guzel Turhan Ceren,BILGE HASAN SAKIR Çekişmeli üretici ağ ile ölçeklenebilir görüntü oluşturma ve süper çözünürlük. Gazi Üniversitesi Mühendislik Mimarlık Fakültesi Dergisi, vol.35, no.2, 2020, ss.953 - 966. 10.17341/gazimmfd.587010 |
AMA | Guzel Turhan C,BILGE H Çekişmeli üretici ağ ile ölçeklenebilir görüntü oluşturma ve süper çözünürlük. Gazi Üniversitesi Mühendislik Mimarlık Fakültesi Dergisi. 2020; 35(2): 953 - 966. 10.17341/gazimmfd.587010 |
Vancouver | Guzel Turhan C,BILGE H Çekişmeli üretici ağ ile ölçeklenebilir görüntü oluşturma ve süper çözünürlük. Gazi Üniversitesi Mühendislik Mimarlık Fakültesi Dergisi. 2020; 35(2): 953 - 966. 10.17341/gazimmfd.587010 |
IEEE | Guzel Turhan C,BILGE H "Çekişmeli üretici ağ ile ölçeklenebilir görüntü oluşturma ve süper çözünürlük." Gazi Üniversitesi Mühendislik Mimarlık Fakültesi Dergisi, 35, ss.953 - 966, 2020. 10.17341/gazimmfd.587010 |
ISNAD | Guzel Turhan, Ceren - BILGE, HASAN SAKIR. "Çekişmeli üretici ağ ile ölçeklenebilir görüntü oluşturma ve süper çözünürlük". Gazi Üniversitesi Mühendislik Mimarlık Fakültesi Dergisi 35/2 (2020), 953-966. https://doi.org/10.17341/gazimmfd.587010 |