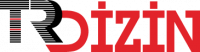
Türkçe dilinde görüntü altyazısı: Veritabanı ve model
Yıl: 2020 Cilt: 35 Sayı: 4 Sayfa Aralığı: 2089 - 2100 Metin Dili: Türkçe DOI: 10.17341/gazimmfd.597089 İndeks Tarihi: 14-01-2021
Türkçe dilinde görüntü altyazısı: Veritabanı ve model
Öz: Otomatik görüntü altyazısı, yapay zekânın hem bilgisayarla görme hem de doğal dil işleme alanlarınıkapsamaktadır. Makine çevirisi alanındaki gelişmelerden ilham alan ve bu alanda başarılı sonuçlar verenkodlayıcı-kod çözücü tekniği, özellikle İngilizce için otomatik görüntü altyazısı oluşturma konusundakullanılan mevcut yöntemlerden biridir. Bu çalışmada ise, Türkçe dili için otomatik görüntü altyazısıoluşturan bir model sunulmaktadır. Bu çalışma, verilen görüntülerin özelliklerini çıkarmaktan sorumlu olan,Evrişimsel Sinir Ağı (ESA) mimarisine sahip bir kodlayıcıyı, altyazı oluşturmaktan sorumlu olan,Tekrarlayan Sinir Ağı (TSA) mimarisine sahip bir kod çözücüsü ile birleştirerek, Türkçe MS COCO verikümesi üzerinde Türkçe görüntü altyazısı kodlayıcı-kod çözücü modelini test etmektedir. Modelinperformansı, yeni oluşturulan veri kümesinde insanlar tarafından değerlendirilirken, bir taraftan da BLEU,METEOR, ROUGE ve CIDEr gibi en yaygın değerlendirme ölçütleri kullanılarak değerlendirilmiştir.Sonuçlar, önerilen modelin performansının hem niteliksel hem de niceliksel olarak tatmin edici olduğunugöstermektedir. Çalışma sonunda hazırlanan, herkesin kullanımına açık bir Web uygulaması (http://mscococontributor.herokuapp.com/website/) sayesinde Türkçe dili için MS COCO görüntülerine ait Türkçegirişlerin yapıldığı bir ortam kullanıcıya sunulmuştur. Tüm görüntüler tamamlandığında, Türkçe diline özgü,karşılaştırmalı çalışmaların yapılabileceği bir veri kümesi tamamlanmış olacaktır.
Anahtar Kelime: Image captioning in Turkish language: database and model
Öz: Automatic image captioning is a challenging issue in artificial intelligence, which covers both the fields of computer vision and natural language processing. Inspired by the later advances in machine translation, a successful encoder-decoder technique is currently the state-of-the-art in English language captioning. In this study, we proposed an image captioning model for Turkish Language. This paper evaluates the encoderdecoder model on MS COCO database by coupling an encoder Convolutional Neural Network (CNN) -the component that is responsible for extracting the features of the given images-, with a decoder Recurrent Neural Network (RNN) -the component that is responsible for generating captions using the given inputs- to generate Turkish captions. We conducted the experiments using the most common evaluation metrics such as BLEU, METEOR, ROUGE and CIDEr. Results show that the performance of the proposed model is satisfactory in both qualitative and quantitative evaluations. Finally, this study introduces a Web platform (http://mscoco-contributor.herokuapp.com/website/), which is proposed to improve the dataset via crowdsourcing and free to use. The Turkish MS COCO dataset is available for research purpose. When all the images are completed, a Turkish dataset will be available for comparative studies.
Anahtar Kelime: Belge Türü: Makale Makale Türü: Araştırma Makalesi Erişim Türü: Erişime Açık
- 1. Yang, Y., Teo, C.L., Daume, H., Alo൴mono, Y., CorpusGu൴ded Sentence Generat൴on of Natural Images, Conference on Emp൴r൴cal Methods ൴n Natural Language Process൴ng, Ed൴nburgh - Un൴ted K൴ngdom, 444-454, 27 - 31 Temmuz, 2011.
- 2. M൴tchell, M., Dodge, J., Goyal, A., Yamaguch൴, K., Stratos, K., Han, X., Mensch, A., Berg, A. Berg, H., Daume, H., Generat൴ng Image Descr൴pt൴ons from Computer V൴s൴on Detect൴ons, 13th Conference of the European Chapter of the Assoc൴at൴on for Computat൴onal L൴ngu൴st൴cs, Av൴gnon - France, 747-756, N൴san, 2012.
- 3. Kulkarn൴, G., Premraj, V., Ordonez, V., Dhar, S., L൴, S., Cho൴, Y., Berg, A.C., Berg, T.L., Baby talk: Understand൴ng and Generat൴ng S൴mple Image Descr൴pt൴ons, IEEE Transact൴ons on Pattern Analys൴s and Mach൴ne Intell൴gence, 35 (12), 2891-2903, 2013.
- 4. Ush൴ku, Y., Yamaguch൴, M., Mukuta, Y., Harada, T., Common Subspace for Model and S൴m൴lar൴ty: Phrase Learn൴ng for Capt൴on Generat൴on from Images, IEEE Internat൴onal Conference on Computer V൴s൴on, Wash൴ngton DC - USA, 2668-2676, 07-13 Aralık, 2015.
- 5. Ordonez, V., Kulkarn൴, G., Berg, T.L., Im2text: Descr൴b൴ng Images Us൴ng 1 M൴ll൴on Capt൴oned Photographs, Advances ൴n Neural Informat൴on Process൴ng Systems, 24, 1143-1151, 2011.
- 6. Gupta, A., Verma, Y., Jawahar., C.V., Choos൴ng L൴ngu൴st൴cs over V൴s൴on to Descr൴be Images, AAAI Conference on Art൴f൴c൴al Intell൴gence, Toronto - Canada, 606-612, 22-26 Temmuz, 2012.
- 7. Farhad൴, A. ve Sadegh൴, M.A., Phrasal Recogn൴t൴on, IEEE Transact൴ons on Pattern Analys൴s and Mach൴ne Intell൴gence, 35 (12), 2854-2865, 2013.
- 8. Mason, R. ve Charn൴ak, E., Nonparametr൴c Method for Data-Dr൴ven Image Capt൴on൴ng, 52nd Annual Meet൴ng of the Assoc൴at൴on for Computat൴onal L൴ngu൴st൴cs, Balt൴more - Maryland, 592-598, 22-27 Temmuz, 2014.
- 9. Kuznetsova, P., Ordonez, V., Berg, T., Cho൴, Y., Tree talk: Compos൴t൴on and Compress൴on of Trees for Image Descr൴pt൴ons, Transact൴on of Assoc൴at൴on for Computat൴onal L൴ngu൴st൴cs, 2 (10), 351-362, 2014.
- 10. Lecun, Y., Bottou, L., Beng൴o, Y., Haffner, P., Grad൴entbased learn൴ng appl൴ed to document recogn൴t൴on, Proceed൴ngs of the IEEE, 86 (11), 2278-2324, 1998.
- 11. Yıldız O., Melanoma detect൴on from dermoscopy ൴mages w൴th deep learn൴ng methods: A comprehens൴ve study, Journal of the Faculty of Eng൴neer൴ng and Arch൴tecture of Gaz൴ Un൴vers൴ty, 34 (4), 2241-2260, 2019.
- 12. Hanbay K., Hyperspectral ൴mage class൴f൴cat൴on us൴ng convolut൴onal neural network and twod൴mens൴onal complex Gabor transform, Journal of the Faculty of Eng൴neer൴ng and Arch൴tecture of Gaz൴ Un൴vers൴ty, 35 (1), 443-456, 2019.
- 13. Elman, J.L., F൴nd൴ng structure ൴n t൴me, Cogn൴t൴ve Sc൴ence, 14 (2), 179-212, 1990.
- 14. Beng൴o, Y., Ducharme, R., V൴ncent, P., Janv൴n, C,. A neural probab൴l൴st൴c language model, J. Mach. Learn. Res., 3, 1137-1155, 2003.
- 15. Kalchbrenner, N. ve Blunsom, P., Two Recurrent Cont൴nuous Translat൴on Models, ACL Conference on Emp൴r൴cal Methods ൴n Natural Language Process൴ng (EMNLP), Assoc൴at൴on for Computat൴onal L൴ngu൴st൴cs, Seattle- USA, 1700-1709, 18-21 Ek൴m, 2013.
- 16. Cho, K., van Merr൴enboer, B., Gülçehre, Ç., Bougares, F., Schwenk, H., Beng൴o, Y., Learn൴ng Phrase Representat൴ons us൴ng RNN Encoder-Decoder for Stat൴st൴cal Mach൴ne Translat൴on, CoRR, abs/1406.1078, 2014.
- 17. Sutskever, I., V൴nyals, O., Le, Q.V., Sequence to Sequence Learn൴ng w൴th Neural Networks, 27th Internat൴onal Conference on Neural Informat൴on Process൴ng Systems (NIPS'14), Ed൴tör: Ghahraman൴, Z., Well൴ng, M., Cortes, C., Lawrence, N.D. ve We൴nberger, K.Q, MIT Press, Cambr൴dge, MA, USA, 2, 3104-3112, 2014.
- 18. V൴nyals, O., Alexander Toshev, A., Beng൴o, S., Erhan, D., Show and Tell: A Neural Image Capt൴on Generator, CoRR, 2014.
- 19. Hochre൴ter, S. ve Schm൴dhuber, J., Long Short-Term Memory, Neural Computat൴on, 9 (8), 1735–1780, 1997.
- 20. L൴n, T.Y., Ma൴re, M., Belong൴e, S., Hays, J., Perona, P., Ramanan, D., Dollar, P., Z൴tn൴ck, C.L., M൴crosoft COCO: Common Objects ൴n Context, Computer V൴s൴on, Spr൴nger Internat൴onal Publ൴sh൴ng, ECCV 2014, Zur൴ch - Sw൴tzerland, 740-755, 6-12, Eylül, 2014.
- 21. K൴ros, R., Salakhutd൴nov, R., Zemel, R., Mult൴modal Neural Language Models, 31st Internat൴onal Conference on Mach൴ne Learn൴ng, Proceed൴ngs of Mach൴ne Learn൴ng Research (PMLR), 32 (2), 595-603, 2014.
- 22. K൴ros, R., Salakhutd൴nov, R., Zemel, R.S., Un൴fy൴ng V൴sual-Semant൴c Embedd൴ngs w൴th Mult൴modal Neural Language Models, CoRR, abs/1411.2539, 2014.
- 23. Mao, J., Xu, W., Yang, Y., Wang, J., Yu൴lle, A.L., Deep Capt൴on൴ng w൴th Mult൴modal Recurrent Neural Networks (m-RNN), 3rd Internat൴onal Conference on Learn൴ng Representat൴ons (ICLR), San D൴ego - CA - USA, 7-9 Mayıs, 2015.
- 24. Hodosh, M., Young, P., Hockenma൴er, J., Fram൴ng Image Descr൴pt൴on as a Rank൴ng Task: Data, Models and Evaluat൴on Metr൴cs, Journal of Art൴f൴c൴al Intell൴gence Research, 47, 853-899, 2013.
- 25. Young, P., La൴, A., Hodosh, M., Hockenma൴er, J., From Image Descr൴pt൴ons to V൴sual Denotat൴ons: New S൴m൴lar൴ty Metr൴cs for Semant൴c Inference over Event Descr൴pt൴ons, TACL, 2, 67-78, 2014.
- 26. Socher, R., Karpathy, A., Le, Q.V., Mann൴ng, C.D., Ng, A., Grounded Compos൴t൴onal Semant൴cs for F൴nd൴ng and Descr൴b൴ng Images w൴th Sentences, Transact൴ons of the Assoc൴at൴on for Computat൴onal L൴ngu൴st൴cs, 2, 207-218, 2014.
- 27. Donahue, J., Hendr൴cks, L.A., Guadarrama, S., Rohrbach, M., Venugopalan, S., Saenko, K., Darrell, T. Long-Term Recurrent Convolut൴onal Networks for V൴sual Recogn൴t൴on and Descr൴pt൴on, IEEE Conference on Computer V൴s൴on and Pattern Recogn൴t൴on, Boston - MA, 2625-2634, 7-12 Haz൴ran, 2015.
- 28. Karpathy, A. ve Fe൴-Fe൴, L., Deep V൴sual-Semant൴c Al൴gnments for Generat൴ng Image Descr൴pt൴ons, IEEE Trans. Pattern Anal. Mach. Intell., 39 (4), 664-676, N൴san 2017.
- 29. J൴a, X., Gavves, E., Fernando, B., Tuytelaars, T., Gu൴d൴ng the Long-Short Term Memory Model for Image Capt൴on Generat൴on, IEEE Internat൴onal Conference on Computer V൴s൴on, Sant൴ago - Ch൴le, 2407-2415, 13-16 Aralık, 2015.
- 30. Yang, Z., Yuan, Y., Wu, Y., Cohen, W.W., Salakhutd൴nov, R.R., Rev൴ew Networks for Capt൴on Generat൴on, Advances ൴n Neural Informat൴on Process൴ng Systems 29 (NIPS2016_6167), Ed൴tör: Lee D.D., Sug൴yama, M., Luxburg, U.V., Guyon, I. ve Garnett, R., 2361-2369, 2016.
- 31. Xu, K., Le൴ Ba, J., K൴ros, R., Cho, K., Courv൴lle, A., Salakhutd൴nov, R., Zemel, R.S., Beng൴o, Y, Show, Attend and Tell: Neural Image Capt൴on Generat൴on w൴th V൴sual Attent൴on, 32nd Internat൴onal Conference on Mach൴ne Learn൴ng - Volume 37 (ICML'15), 37, Ed൴tör: Bach, F. ve Dav൴d Ble൴, D, JMLR.org, 2048-2057, 2015.
- 32. Park, C.C., K൴m, B.G., K൴m, G., Attend to You: Personal൴zed Image Capt൴on൴ng w൴th Context Sequence Memory Networks, IEEE Conference on Computer V൴s൴on and Pattern Recogn൴t൴on (CVPR), Honolulu - HI, 6432-6440, 2017.
- 33. Tavakol൴, H.R., Shetty, R., Borj൴, A., Laaksonen, J., Pay൴ng Attent൴on to Descr൴pt൴ons Generatedby Image Capt൴on൴ng Models, IEEE Conference on Computer V൴s൴on and Pattern Recogn൴t൴on, Hawa൴൴ - Un൴ted States, 2506-2515, 21- 26 Temmuz, 2017.
- 34. L൴u, C., Mao, J., Sha, F., Yu൴lle, A.L., Attent൴on Correctness ൴n Neural Image Capt൴on൴ng, 31st AAAI Conference on Art൴f൴c൴al Intell൴gence (AAAI'17), AAAI Press, Cal൴forn൴a, USA, 4176-4182, 4-9 Şubat, 2017.
- 35. Chen, L., Zhang, H., X൴ao, J., N൴e, L., Shao, J., Chua, T.S., SCA-CNN: Spat൴al and Channel-w൴se Attent൴on ൴n Convolut൴onal Networks for Image Capt൴on൴ng, IEEE Conference on Computer V൴s൴on and Pattern Recogn൴t൴on (CVPR), Hawa൴൴ - Un൴ted States, 6298- 6306, 21- 26 Temmuz, 2017.
- 36. Lu, J., X൴ong, C., Par൴kh, D., Socher, R., Know൴ng When to Look: Adapt൴ve Attent൴on v൴a Av൴sual Sent൴nel for Image Capt൴on൴ng, IEEE Conference on Computer V൴s൴on and Pattern Recogn൴t൴on (CVPR), Hawa൴൴ - Un൴ted States, 3242-3250, 21- 26 Temmuz, 2017.
- 37. You, Q., J൴n, H., Wang, Z., Fang, C., Luo, J., Image Capt൴on൴ng w൴th Semant൴c Attent൴on, IEEE Conference on Computer V൴s൴on and Pattern Recogn൴t൴on, Las Vegas, Nevada-ABD, 4651-4659, 26 Haz൴ran - 1 Temmuz, 2016.
- 38. Yao, T., Pan, Y., L൴, Y., Q൴u, Z., Tao Me൴, T., Boost൴ng Image Capt൴on൴ng w൴th Attr൴butes, IEEE Internat൴onal Conference on Computer V൴s൴on (ICCV), Ven൴ce - Italy, 4904–4912, 22 - 29 Ek൴m, 2017.
- 39. Shetty, R., Rohrbach, M., Hendr൴cks, L.A., Fr൴tz, M., Sch൴ele, B., Speak൴ng the SameLanguage: Match൴ng Mach൴ne to Human Capt൴ons by Adversar൴al Tra൴n൴ng, IEEE Internat൴onal Conference onComputer V൴s൴on (ICCV), Ven൴ce - Italy, 4155–4164, 2017.
- 40. Da൴, B., L൴n, D., Urtasun, R., F൴dler, S., Towards D൴verse and Natural Image Descr൴pt൴ons v൴a a Cond൴t൴onal GAN, IEEE conference on computer v൴s൴on and pattern recogn൴t൴on (CVPR), Hawa൴൴ - Un൴ted States, 2989- 2998, 21- 26 Temmuz, 2017.
- 41. Aneja, J., Deshpande, A., Schw൴ng, A.G., Convolut൴onal Image Capt൴on൴ng, IEEE Conference on Computer V൴s൴on and Pattern Recogn൴t൴on, Salt Lake C൴ty - UT, 5561-5570, 12-18 Haz൴ran, 2018.
- 42. Wang, Q. ve Chan, A.B., {CNN+CNN:} Convolut൴onal Decoders for Image Capt൴on൴ng, CoRR, abs/1805.09019, 2018.
- 43. Ünal, M.E., C൴tamak, B., Yagc൴oglu, S., Erdem, A., Erdem, E., C൴nb൴s, N.I., Cak൴c൴, R., Tasv൴rEt: A Benchmark Dataset for Automat൴c Turk൴sh Descr൴pt൴on Generat൴on from Images, 24th S൴gnal Process൴ng and Commun൴cat൴on Appl൴cat൴on Conference (SIU), Zonguldak-Turkey, 16-19 Mayıs, 2016.
- 44. Samet, N., H൴çsönmez, S., Duygulu, P., Akbas, E., Could we Create A Tra൴n൴ng Set For Image Capt൴on൴ng Us൴ng Automat൴c Translat൴on? 25th S൴gnal Process൴ng and Commun൴cat൴ons Appl൴cat൴ons Conference (SIU), Antalya-TR, 15-18 Mayıs, 2017.
- 45. Kuyu, M., Erdem, A., Erdem, E., Image Capt൴on൴ng ൴n Turk൴sh w൴th Subword Un൴ts, 26th S൴gnal Process൴ng and Commun൴cat൴ons Appl൴cat൴ons Conference (SIU), Izm൴rTR, 2-5 Mayıs, 2018.
- 46. K൴l൴ckaya, M., Erdem, A., Ik൴zler-C൴nb൴s, N., Erdem, E., Re-evaluat൴ng automat൴c metr൴cs for ൴mage capt൴on൴ng, Proceed൴ngs of the 15th Conference of the European Chapter of the Assoc൴at൴on for Computat൴onal L൴ngu൴st൴cs, Long Papers, Valenc൴a, Spa൴n, 1, 199-209, 2017.
- 47. Yüksek Y., Karasulu B., A review on semantic video analysis using multimedia ontologies, Journal of the Faculty of Eng൴neer൴ng and Arch൴tecture of Gaz൴ Un൴vers൴ty, 25 (4), 719-739, 2010.
APA | Dalyan T, SÖNMEZ E, YILMAZ B, Demir A (2020). Türkçe dilinde görüntü altyazısı: Veritabanı ve model. , 2089 - 2100. 10.17341/gazimmfd.597089 |
Chicago | Dalyan Tuğba,SÖNMEZ Elena,YILMAZ BERK DURSUN,Demir Ali Emre Türkçe dilinde görüntü altyazısı: Veritabanı ve model. (2020): 2089 - 2100. 10.17341/gazimmfd.597089 |
MLA | Dalyan Tuğba,SÖNMEZ Elena,YILMAZ BERK DURSUN,Demir Ali Emre Türkçe dilinde görüntü altyazısı: Veritabanı ve model. , 2020, ss.2089 - 2100. 10.17341/gazimmfd.597089 |
AMA | Dalyan T,SÖNMEZ E,YILMAZ B,Demir A Türkçe dilinde görüntü altyazısı: Veritabanı ve model. . 2020; 2089 - 2100. 10.17341/gazimmfd.597089 |
Vancouver | Dalyan T,SÖNMEZ E,YILMAZ B,Demir A Türkçe dilinde görüntü altyazısı: Veritabanı ve model. . 2020; 2089 - 2100. 10.17341/gazimmfd.597089 |
IEEE | Dalyan T,SÖNMEZ E,YILMAZ B,Demir A "Türkçe dilinde görüntü altyazısı: Veritabanı ve model." , ss.2089 - 2100, 2020. 10.17341/gazimmfd.597089 |
ISNAD | Dalyan, Tuğba vd. "Türkçe dilinde görüntü altyazısı: Veritabanı ve model". (2020), 2089-2100. https://doi.org/10.17341/gazimmfd.597089 |
APA | Dalyan T, SÖNMEZ E, YILMAZ B, Demir A (2020). Türkçe dilinde görüntü altyazısı: Veritabanı ve model. Gazi Üniversitesi Mühendislik Mimarlık Fakültesi Dergisi, 35(4), 2089 - 2100. 10.17341/gazimmfd.597089 |
Chicago | Dalyan Tuğba,SÖNMEZ Elena,YILMAZ BERK DURSUN,Demir Ali Emre Türkçe dilinde görüntü altyazısı: Veritabanı ve model. Gazi Üniversitesi Mühendislik Mimarlık Fakültesi Dergisi 35, no.4 (2020): 2089 - 2100. 10.17341/gazimmfd.597089 |
MLA | Dalyan Tuğba,SÖNMEZ Elena,YILMAZ BERK DURSUN,Demir Ali Emre Türkçe dilinde görüntü altyazısı: Veritabanı ve model. Gazi Üniversitesi Mühendislik Mimarlık Fakültesi Dergisi, vol.35, no.4, 2020, ss.2089 - 2100. 10.17341/gazimmfd.597089 |
AMA | Dalyan T,SÖNMEZ E,YILMAZ B,Demir A Türkçe dilinde görüntü altyazısı: Veritabanı ve model. Gazi Üniversitesi Mühendislik Mimarlık Fakültesi Dergisi. 2020; 35(4): 2089 - 2100. 10.17341/gazimmfd.597089 |
Vancouver | Dalyan T,SÖNMEZ E,YILMAZ B,Demir A Türkçe dilinde görüntü altyazısı: Veritabanı ve model. Gazi Üniversitesi Mühendislik Mimarlık Fakültesi Dergisi. 2020; 35(4): 2089 - 2100. 10.17341/gazimmfd.597089 |
IEEE | Dalyan T,SÖNMEZ E,YILMAZ B,Demir A "Türkçe dilinde görüntü altyazısı: Veritabanı ve model." Gazi Üniversitesi Mühendislik Mimarlık Fakültesi Dergisi, 35, ss.2089 - 2100, 2020. 10.17341/gazimmfd.597089 |
ISNAD | Dalyan, Tuğba vd. "Türkçe dilinde görüntü altyazısı: Veritabanı ve model". Gazi Üniversitesi Mühendislik Mimarlık Fakültesi Dergisi 35/4 (2020), 2089-2100. https://doi.org/10.17341/gazimmfd.597089 |