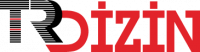
Akciğer Seslerinin Derin Öğrenme ile Sınıflandırılması
Yıl: 2020 Cilt: 8 Sayı: 4 Sayfa Aralığı: 830 - 844 Metin Dili: Türkçe DOI: 10.29109/gujsc.758325 İndeks Tarihi: 02-04-2021
Akciğer Seslerinin Derin Öğrenme ile Sınıflandırılması
Öz: Akciğer hastalıkları, insan sağlığını ciddi olarak tehdit eden hastalıklar arasındadır ve günümüzde birçok ölüm akciğer hastalıklarından kaynaklanmaktadır. Akciğer sesleri sayesinde akciğer hastalıkları ile ilgili önemli çıkarımlar yapılabilmektedir. Akciğer rahatsızlığı olan hastaları değerlendirmek için doktorlar genellikle oskültasyon tekniğini kullanırlar. Ancak, bu tekniğin bazı dezavantajları vardır. Örneğin, doktor iyi bir tıp eğitimi almamışsa, bu yanlış tanıya neden olabilir. Ayrıca akciğer sesleri durağan olmadığından dolayı analiz, tanıma süreci karmaşıktır. Bu nedenle otomatik tanıma sistemlerinin geliştirilmesi daha kesin ve doğru tanılar koymada yardımcı olacaktır. Akciğer hastalıklarını teşhis etmek ve uzmanlara teşhislerinde yardımcı olmak için geleneksel ses işleme rutinlerine dayanan birçok çalışma önerilmiştir. Bu çalışmada akciğer seslerinin sınıflandırılması için derin öğrenmeye dayanan bir yöntem önerilmiştir. Bu amaç doğrultusunda Evrişimsel Sinir Ağı (ESA) tasarlanmıştır. Ayrıca özellik çıkarımına dayanan farklı makine öğrenmesi yöntemleri kullanılarak da deneyler gerçekleştirilmiştir. Deneyler, farklı yöntemlerin etkinliğini değerlendirmek için literatürde yaygın olarak kullanılan, dört sınıftan oluşan ICBHI 2017 veri seti ile yapılmıştır. Ortalama olarak %64,5 doğruluk önerilen yöntemden elde edilmiştir.
Anahtar Kelime: Classification of Lung Sounds with Deep Learning
Öz: Lung diseases are among the diseases that seriously threaten human health, and many deaths today are caused by lung diseases. Thanks to the lung sounds, important inferences can be made about lung diseases. Doctors often use the auscultation technique to evaluate patients with lungconditions. However, this technique has some drawbacks. For example, this may lead to a misdiagnosis if the doctor has not received a good medical education. In addition, since the lung sounds are nonstationary, the analysis and recognition process is complex. Therefore, the development of automatic recognition systems will help in making more precise and accurate diagnoses. Many studies based on traditional sound processing routines have been proposed to diagnose lung diseases and to assist professionals in their diagnosis. In this study, a method based on deep learning has been proposed for the classification of lung sounds. For this purpose, the Convolutional Neural Network (CNN) has been designed. In addition, experiments are carried out using different machine learning methods based on feature extraction. Experiments to evaluate the effectiveness of different methods are carried out using the ICBHI 2017 data set consisting of four classes commonly used in the literature. On average, 64.5% accuracy is obtained from the proposed method. In addition, when the results obtained from the proposed method are compared with the latest methods in the literature, it is seen that it has a better performance in terms of classification success.
Anahtar Kelime: Belge Türü: Makale Makale Türü: Araştırma Makalesi Erişim Türü: Erişime Açık
- [1] F. Demir, A. Sengur, and V. Bajaj, “Convolutional neural networks based efficient approach for classification of lung diseases,” Heal. Inf. Sci. Syst., vol. 8, no. 1, p. 4, Dec. 2019, doi: 10.1007/s13755-019-0091-3.
- [2] S. İçer and Ş. Gengeç, “Classification and analysis of non-stationary characteristics of crackle and rhonchus lung adventitious sounds,” Digit. Signal Process., vol. 28, pp. 18–27, 2014, doi: 10.1016/j.dsp.2014.02.001.
- [3] R. Naves, B. H. G. Barbosa, and D. D. Ferreira, “Classification of lung sounds using higher-order statistics: A divide-and-conquer approach,” Comput. Methods Programs Biomed., vol. 129, pp. 12– 20, 2016, doi: 10.1016/j.cmpb.2016.02.013.
- [4] G.-C. Chang and Y.-P. Cheng, “Investigation of noise effect on lung sound recognition,” 2008 International Conference on Machine Learning and Cybernetics. IEEE, 2008, doi: 10.1109/icmlc.2008.4620605.
- [5] S. Reichert, R. Gass, C. Brandt, and E. Andrès, “Analysis of respiratory sounds: state of the art,” Clin. Med. Circ. Respirat. Pulm. Med., vol. 2, pp. 45–58, May 2008, doi: 10.4137/ccrpm.s530.
- [6] A. Kandaswamy, C. S. Kumar, R. P. Ramanathan, S. Jayaraman, and N. Malmurugan, “Neural classification of lung sounds using wavelet coefficients,” Comput. Biol. Med., vol. 34, no. 6, pp. 523–537, 2004, doi: https://doi.org/10.1016/S0010-4825(03)00092-1.
- [7] S. ULUKAYA, G. SERBES, İ. ŞEN, and Y. P. KAHYA, “Akciğer Solunum Seslerinin Spektral Öznitelikler ile Sınıflandırılması,” Süleyman Demirel Üniversitesi Fen Bilim. Enstitüsü Derg., vol. 22, no. 2, p. 711, 2017, doi: 10.19113/sdufbed.84471.
- [8] A. Sovijärvi et al., “Characteristic of breath sounds and adventitious respiratory sounds,” Charact. Breath Sounds Adventitious Respir. Sounds, vol. 10, pp. 591–596, Jan. 2000.
- [9] G. Serbes, C. O. Sakar, Y. P. Kahya, and N. Aydin, “Pulmonary crackle detection using time– frequency and time–scale analysis,” Digit. Signal Process., vol. 23, no. 3, pp. 1012–1021, 2013, doi: https://doi.org/10.1016/j.dsp.2012.12.009.
- [10] F. Jin, F. Sattar, and D. Y. T. Goh, “New approaches for spectro-temporal feature extraction with applications to respiratory sound classification,” Neurocomputing, vol. 123, pp. 362–371, 2014, doi: 10.1016/j.neucom.2013.07.033.
- [11] M. Bahoura, “Pattern recognition methods applied to respiratory sounds classification into normal and wheeze classes.,” Comput. Biol. Med., vol. 39, no. 9, pp. 824–843, Sep. 2009, doi: 10.1016/j.compbiomed.2009.06.011.
- [12] A. D. Orjuela-Cañón, D. F. Gómez-Cajas, and R. Jiménez-Moreno, “Artificial Neural Networks for Acoustic Lung Signals Classification,” Advanced Information Systems Engineering. Springer Berlin Heidelberg, pp. 214–221, 2014, doi: 10.1007/978-3-319-12568-8_27.
- [13] S. O. Maruf, M. U. Azhar, S. G. Khawaja, and M. U. Akram, “Crackle separation and classification from normal Respiratory sounds using Gaussian Mixture Model,” 2015 IEEE 10th International Conference on Industrial and Information Systems (ICIIS). IEEE, 2015, doi: 10.1109/iciinfs.2015.7399022.
- [14] M. Yeginer and Y. P. Kahya, “Elimination of vesicular sounds from pulmonary crackle waveforms,” Comput. Methods Programs Biomed., vol. 89, no. 1, pp. 1–13, 2008, doi: 10.1016/j.cmpb.2007.10.002.
- [15] G. Serbes, S. Ulukaya, and Y. P. Kahya, “An Automated Lung Sound Preprocessing and Classification System Based OnSpectral Analysis Methods,” Precision Medicine Powered by pHealth and Connected Health. Springer Singapore, pp. 45–49, 2017, doi: 10.1007/978-981-10- 7419-6_8.
- [16] G. Chambres, P. Hanna, and M. Desainte-Catherine, “Automatic Detection of Patient with Respiratory Diseases Using Lung Sound Analysis,” 2018 International Conference on Content- Based Multimedia Indexing (CBMI). IEEE, 2018, doi: 10.1109/cbmi.2018.8516489.
- [17] D. Bardou, K. Zhang, and S. M. Ahmad, “Lung sounds classification using convolutional neural networks,” Artif. Intell. Med., vol. 88, pp. 58–69, 2018, doi: https://doi.org/10.1016/j.artmed.2018.04.008.
- [18] C. Jácome, J. Ravn, E. Holsbø, J. C. Aviles-Solis, H. Melbye, and L. Ailo Bongo, “Convolutional Neural Network for Breathing Phase Detection in Lung Sounds,” Sensors (Basel)., vol. 19, no. 8, p. 1798, Apr. 2019, doi: 10.3390/s19081798.
- [19] K. Kochetov, E. Putin, M. Balashov, A. Filchenkov, and A. Shalyto, “Noise Masking Recurrent Neural Network for Respiratory Sound Classification,” Artificial Neural Networks and Machine Learning – ICANN 2018. Springer International Publishing, pp. 208–217, 2018, doi: 10.1007/978- 3-030-01424-7_21.
- [20] Y. Fu and C. Aldrich, “Flotation froth image recognition with convolutional neural networks,” Miner. Eng., vol. 132, pp. 183–190, 2019, doi: 10.1016/j.mineng.2018.12.011.
- [21] A. GÜLCÜ and Z. KUŞ, “Konvolüsyonel Sinir Ağlarında Hiper-Parametre Optimizasyonu Yöntemlerinin İncelenmesi,” Gazi Üniversitesi Fen Bilimleri Dergisi Part C: Tasarım ve Teknoloji, vol. 7. Gazi Üniversitesi, pp. 503–522, 2019, doi: 10.29109/gujsc.514483.
- [22] D. C. Cireundefinedan, U. Meier, J. Masci, L. M. Gambardella, and J. Schmidhuber, “Flexible, High Performance Convolutional Neural Networks for Image Classification,” in Proceedings of the Twenty-Second International Joint Conference on Artificial Intelligence - Volume Volume Two, 2011, pp. 1237–1242.
- [23] M. Bilal Er and I. B. Aydilek, “Music emotion recognition by using chroma spectrogram and deep visual features,” Int. J. Comput. Intell. Syst., vol. 12, no. 2, 2019, doi: 10.2991/ijcis.d.191216.001.
- [24] J. Salamon and J. P. Bello, “Deep Convolutional Neural Networks and Data Augmentation for Environmental Sound Classification,” IEEE Signal Process. Lett., vol. 24, no. 3, pp. 279–283, 2017, doi: 10.1109/LSP.2017.2657381.
- [25] “Support Vector Machines, 1992; Boser, Guyon, Vapnik,” in SpringerReference, Springer-Verlag.
- [26] C. Li et al., “Using the K-Nearest Neighbor Algorithm for the Classification of Lymph Node Metastasis in Gastric Cancer,” Comput. Math. Methods Med., vol. 2012, pp. 1–11, 2012, doi: 10.1155/2012/876545.
- [27] B. M. Rocha et al., “An open access database for the evaluation of respiratory sound classification algorithms,” Physiol. Meas., vol. 40, no. 3, p. 35001, 2019, doi: 10.1088/1361-6579/ab03ea.
- [28] M. B. ER and H. ÇİĞ, “Türk Müziği Uyaranları Kullanılarak İnsan Duygularının Makine Öğrenmesi Yöntemi İle Tanınması,” Gazi Üniversitesi Fen Bilim. Derg. Part C Tasarım ve Teknol., vol. 8, no. 2, pp. 458–474, 2020, doi: 10.29109/gujsc.687199.
- [29] K. Kochetov, E. Putin, M. Balashov, A. Filchenkov, and A. Shalyto, “Noise Masking Recurrent Neural Network for Respiratory Sound Classification,” Artificial Neural Networks and Machine Learning – ICANN 2018. Springer International Publishing, pp. 208–217, 2018, doi: 10.1007/978- 3-030-01424-7_21.
- G. Serbes, S. Ulukaya, and Y. P. Kahya, “An Automated Lung Sound Preprocessing and Classification System Based OnSpectral Analysis Methods,” Precision Medicine Powered by pHealth and Connected Health. Springer Singapore, pp. 45–49, 2017, doi: 10.1007/978-981-10- 7419-6_8.
- G. Chambres, P. Hanna, and M. Desainte-Catherine, “Automatic Detection of Patient with Respiratory Diseases Using Lung Sound Analysis,” 2018 International Conference on Content- Based Multimedia Indexing (CBMI). IEEE, 2018, doi: 10.1109/cbmi.2018.8516489.
APA | er M (2020). Akciğer Seslerinin Derin Öğrenme ile Sınıflandırılması. , 830 - 844. 10.29109/gujsc.758325 |
Chicago | er Mehmet Bilal Akciğer Seslerinin Derin Öğrenme ile Sınıflandırılması. (2020): 830 - 844. 10.29109/gujsc.758325 |
MLA | er Mehmet Bilal Akciğer Seslerinin Derin Öğrenme ile Sınıflandırılması. , 2020, ss.830 - 844. 10.29109/gujsc.758325 |
AMA | er M Akciğer Seslerinin Derin Öğrenme ile Sınıflandırılması. . 2020; 830 - 844. 10.29109/gujsc.758325 |
Vancouver | er M Akciğer Seslerinin Derin Öğrenme ile Sınıflandırılması. . 2020; 830 - 844. 10.29109/gujsc.758325 |
IEEE | er M "Akciğer Seslerinin Derin Öğrenme ile Sınıflandırılması." , ss.830 - 844, 2020. 10.29109/gujsc.758325 |
ISNAD | er, Mehmet Bilal. "Akciğer Seslerinin Derin Öğrenme ile Sınıflandırılması". (2020), 830-844. https://doi.org/10.29109/gujsc.758325 |
APA | er M (2020). Akciğer Seslerinin Derin Öğrenme ile Sınıflandırılması. Gazi Üniversitesi Fen Bilimleri Dergisi Part C: Tasarım ve Teknoloji, 8(4), 830 - 844. 10.29109/gujsc.758325 |
Chicago | er Mehmet Bilal Akciğer Seslerinin Derin Öğrenme ile Sınıflandırılması. Gazi Üniversitesi Fen Bilimleri Dergisi Part C: Tasarım ve Teknoloji 8, no.4 (2020): 830 - 844. 10.29109/gujsc.758325 |
MLA | er Mehmet Bilal Akciğer Seslerinin Derin Öğrenme ile Sınıflandırılması. Gazi Üniversitesi Fen Bilimleri Dergisi Part C: Tasarım ve Teknoloji, vol.8, no.4, 2020, ss.830 - 844. 10.29109/gujsc.758325 |
AMA | er M Akciğer Seslerinin Derin Öğrenme ile Sınıflandırılması. Gazi Üniversitesi Fen Bilimleri Dergisi Part C: Tasarım ve Teknoloji. 2020; 8(4): 830 - 844. 10.29109/gujsc.758325 |
Vancouver | er M Akciğer Seslerinin Derin Öğrenme ile Sınıflandırılması. Gazi Üniversitesi Fen Bilimleri Dergisi Part C: Tasarım ve Teknoloji. 2020; 8(4): 830 - 844. 10.29109/gujsc.758325 |
IEEE | er M "Akciğer Seslerinin Derin Öğrenme ile Sınıflandırılması." Gazi Üniversitesi Fen Bilimleri Dergisi Part C: Tasarım ve Teknoloji, 8, ss.830 - 844, 2020. 10.29109/gujsc.758325 |
ISNAD | er, Mehmet Bilal. "Akciğer Seslerinin Derin Öğrenme ile Sınıflandırılması". Gazi Üniversitesi Fen Bilimleri Dergisi Part C: Tasarım ve Teknoloji 8/4 (2020), 830-844. https://doi.org/10.29109/gujsc.758325 |