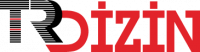
S.cerevisiae ile REMOZAL SARI (RR) GİDERİMİNE YAPAY SİNİR AĞI (YSA) YAKLAŞIMI
Yıl: 2019 Cilt: 24 Sayı: 2 Sayfa Aralığı: 289 - 297 Metin Dili: Türkçe DOI: 10.17482/uumfd.461122 İndeks Tarihi: 28-04-2021
S.cerevisiae ile REMOZAL SARI (RR) GİDERİMİNE YAPAY SİNİR AĞI (YSA) YAKLAŞIMI
Öz: Bu çalışma kesikli sistemde S. cerevisiae ile Remazol Sarı (RR) gideriminin incelendiği bir önceki çalışmamızın devamı niteliğinde olup deneysel sistemden elde edilen veriler ile biyosorpsiyon işlemine Yapay Sinir Ağı (YSA) yaklaşımı 2 farklı aşamada uygulanmıştır. İlk kısımda biyosorpsiyon sistemi için YSA sistem girdi verisi olarak pH, başlangıç boya konsantrasyonu ve başlangıç biyosorbent konsantrasyonu tanımlanırken çıktı katmanında % boya giderim değeri tahmin edilmiştir. YSA eğitimi Levenberg–Marquardt ileri besleme algoritması ile yapılmış olup deneysel veriler %60 eğitim, %20 validasyon ve %20 test olarak bölünmüştür. Maksimum devir (epoch) değeri 12000 iterasyon olarak belirlenmiştir. Sisteme ait R2 değerleri eğitim için %98, validasyon için %96 ve tüm biyosorpsiyon sistemi için %98 olarak belirlenmiştir. Çalışmanın ikinci aşamasında biyosorpsiyon sistem parametrelerinin biyosorpsiyon işlemine ayrı ayrı etkilerinin YSA ile modellenmesi gerçekleştirilmiştir. Deneysel veriler bu kısımda %50 eğitim, %25 validasyon ve %25 test olarak bölünmüştür. Çalışma sonucunda deneysel ve model tahmini % giderim değerleri karşılaştırıldığında, YSA ile sistemin iyi bir şekilde modellendiği ve modelin iyi bir tahmin yeteneğine sahip olduğu görülmüştür.
Anahtar Kelime: Artificial Neural Network (ANN) Approach to Remazol Yellow (RR) Removal with S. cerevisiae
Öz: This study was a continuation of the our previous study in which the Remazol Yellow (RR) removal with S. cerevisiae was examined in the batch system and Artificial Neural Network (ANN) approach has been applied in 2 different stages to the biosorption process with the data obtained from this experimental system. In the first part, pH, initial dye concentration and initial biosorbent concentration are defined as system input data and the % dye removal value in the output layer is estimated with the artificial neural network. ANN training was conducted with the Levenberg-Marquardt feed forward algorithm and the experimental data were divided into 60% training, 20% validation and 20% testing. The maximum epoch value is determined as 12000 iterations. The system R2 value was set at 98% for training, 96% for validation and 98% for the entire biosorption system. In the second stage of the study, the effects of biosorption system parameters (pH, initial dye and biosorbent concentrations and temperature ) on the biosorption process were modeled by ANN. Experimental data is divided into 50% training, 25% validation and 25% test in this section. At the end of this study experimental and model estimates % dye removal values were compared and it was seen that the system is well modelled with ANN and the model has a good estimation ability.
Anahtar Kelime: Belge Türü: Makale Makale Türü: Araştırma Makalesi Erişim Türü: Erişime Açık
- 1. Abdurrahman, F.B., Akter, M., Abedin, Z. (2013) Dyes Removal From Textile Wastewater Using Orange Peels, International Journal of Scientific & Technology Research, 2-9.
- 2. Amouei A., Amooey A.A., Asgharzadeh F. (2013) A study of cadmium removal from aqueous solutions by sunflower powders and its modeling using artificial neural network, Iranian Journal of Health Sciences., 1(3): 28-34. doi: 10.18869/acadpub.jhs.1.3.28
- 3. Argun, Y.A., Karacalı A., Çalışır U., Kılınç, N., İrak H. (2017) Biosorption method and biosorbents for dye removal from industrial wastewater: A Review, International Journal of Advanced Research, 5(8), 707-714. doi: 10.21474/IJAR01/5110
- 4. Arı A., Berberler M.E. (2017) Yapay Sinir Ağları ile Tahmin ve Sınıflandırma Problemlerinin Çözümü İçin Arayüz Tasarımı, Acta Infologica, 1(2).
- 5. Asgher, M. (2012) Biosorption of Reactive Dyes: A Review, Water, Air, & Soil Pollution, 223(5), 2417–2435. doi: 10.1007/s11270-011-1034-z
- 6. Aslay F., Özen Ü. (2013) Meteorolojik Parametreler Kullanılarak Yapay Sinir Ağları ile Toprak Sıcaklığının Tahmini, Politeknik Dergisi, 16(4), 139-145. doi: 10.2339/2013.16.4, 139-145
- 7. Ataseven B. (2013) Yapay Sinir Ağları ile Öngörü Modellemesi, Öneri Dergisi, 10(39), 101-105.
- 8. Bingöl D., Kılıç E., Hercan M. (2016) Bakır biyosorpsiyon işlemine Yapay Sinir Ağı (ANN) yaklaşımı, Sakarya Üniversitesi Fen Bilimleri Üniversitesi Dergisi, 20(3), 433-440. doi: 10.16984/saufenbilder.25723.
- 9. Chu K.H. (2003) Prediction of two-metal biosorption equilibria using a neural network, The European Journal of Mineral Processing and Environmental Protection, 3(1), 119-127.
- 10. Çavuşlu M.A., Becerikli Y., Karakuzu C. (2012) Levenberg-Marquardt Algoritması ile YSA Eğitiminin Donanımsal Gerçeklenmesi, Türkiye Bilişim Vakfı Bilgisayar Bilimleri ve Mühendisliği Dergisi, 5(1).
- 11. Çoruh S., Kılıç E., Geyikci F. (2014) Prediction of adsorption efficiency for the removal malachite green and acid blue 161 dyes by waste marble dust using ANN, Global Nest Journal, 16(4):676-689. doi: 10.30955/gnj.001366
- 12. Dalkılınç, M. (2015). Düşük Maliyetli Biyosorbent ile Sabit Yataklı Kolonda Reaktif Mavi 21 ve Reaktif Kırmızı 250 Biyar Maddelerinin Giderimi ve Atılım Eğrilerinin Modellenmesi, Yüksek Lisans Tezi, Atatürk Üniversitesi Fen Bilimleri Enstitüsü, Erzurum.
- 13. Donut N., Cavas L. (2017) Artificial Neural Network Modeling of Tetracycline Biosorption by Pre-treated Posidonia oceanica, Turkish Journal of Fisheries and Aquatic Sciences 17, 1317-1333. doi: 10.4194/1303-2712-v17_6_50
- 14. Erdem F., Tosun A., Ergun M. (2016) S. cerevisiae ile Remazol Sarı (RR) boyasının kesikli sistemde biyosorpsiyonu, Journal of the Faculty of Engineering and Architecture of Gazi University, 31(4), 971-978. doi: 10.17341/gazimmfd.278452
- 15. Faisal A., Nassir Z.S. (2016) Modelling the removal of Cadmium ions from aqueous solutions onto olive pips using neural network technique, Al-Khwarizmi Engineering Journal, 12(3), 1-9. doi: 10.1016/j.procbio.2004.11.007
- 16. Fiona N.(2001) Neural Networks algorithms and applications, Neil’s Brock Business College.
- 17. Garza-González M.T., Alcalá-Rodríguez M.M., Pérez-Elizond R., Cerino-Córdova F.J. Garcia-Reyes R.B., Loredo-Medrano J.A., Soto-Regalado, E. (2011) Artificial neural network for predicting biosorption of methylene blue by Spirulina sp., Water scence and technology, 75(5), 977-983. doi: 10.2166/wst.2011.279
- 18. Karaman Ş. Deniz F. (2014) Pinus brutia Ten. (Kızılçam) Kozalak ve Yaprak Biyomasının Boya Biyosorpsiyon/Desorpsiyon Potansiyeli, Kahramanmaraş Sütçü İmam Üniversitesi Tarım ve Doğa Dergisi, 17(3), 19-25. doi: 10.18016/ksujns.19406
- 19. Kardam A. Raj K.R., Arora J.K., Srivastava S. (2014) Simulation and Optimization of Artificial Neural Network Modeling for Prediction of Sorption Efficiency of Nanocellulose Fibers for Removal of Cd (II) Ions from Aqueous System, Engineering and Physical Sciences, 11(6): 497‐508. doi: 10.2004/wjst.v11i6.625
- 20. Krose B., Smagt P. (1998) An introduction to Neural Networks, 8. Baskı, Amsterdam.
- 21. Nasrullaha A., Bhata A.H., Isab M.H., Danishc M., Naeemd A., Muhammade N., Khanb T. (2017) Efficient removal of methylene blue dye using mangosteen peel waste: kinetics, isotherms and artificial neural network (ANN) modeling, Desalination and Water Treatment, 86, 191-202. doi: 10.5004/dwt.2017.21295
- 22. Özdemir H. (2013) Yapay Sinir Ağları ve Dokuma Teknolojisinde Kullanımı, Tekstil Teknolojileri Elektronik Dergisi, 7(1), 51-68.
- 23. Saibaba K.V.N., King P. (2012) Application of artificial neural networks and response surface methodology for dye removal using a novel adsorbent chemical technology. Chemical Technology: An Indian Journal, 7(1), 9-15. doi: 10.1177/0263617416675625
- 24. Yaseen D. A. , Scholz M. (2019) Textile dye wastewater characteristics and constituents of synthetic effluents: a critical review, International Journal of Environmental Science and Technology, 16(2), 1193–1226. doi: 10.1007/s13762-018-2130-z 25. Yu L., Wang S. Keung Lai K. (2007) Basic Learning Principles of Artificial Neural Networks. In: Foreign-Exchange-Rate Forecasting With Artificial Neural Networks. International Series in Operations Research & Management Science, 107, Springer, Boston. doi: 10.1007/978-0-387-71720-3.
APA | Erdem F (2019). S.cerevisiae ile REMOZAL SARI (RR) GİDERİMİNE YAPAY SİNİR AĞI (YSA) YAKLAŞIMI. , 289 - 297. 10.17482/uumfd.461122 |
Chicago | Erdem Fatma S.cerevisiae ile REMOZAL SARI (RR) GİDERİMİNE YAPAY SİNİR AĞI (YSA) YAKLAŞIMI. (2019): 289 - 297. 10.17482/uumfd.461122 |
MLA | Erdem Fatma S.cerevisiae ile REMOZAL SARI (RR) GİDERİMİNE YAPAY SİNİR AĞI (YSA) YAKLAŞIMI. , 2019, ss.289 - 297. 10.17482/uumfd.461122 |
AMA | Erdem F S.cerevisiae ile REMOZAL SARI (RR) GİDERİMİNE YAPAY SİNİR AĞI (YSA) YAKLAŞIMI. . 2019; 289 - 297. 10.17482/uumfd.461122 |
Vancouver | Erdem F S.cerevisiae ile REMOZAL SARI (RR) GİDERİMİNE YAPAY SİNİR AĞI (YSA) YAKLAŞIMI. . 2019; 289 - 297. 10.17482/uumfd.461122 |
IEEE | Erdem F "S.cerevisiae ile REMOZAL SARI (RR) GİDERİMİNE YAPAY SİNİR AĞI (YSA) YAKLAŞIMI." , ss.289 - 297, 2019. 10.17482/uumfd.461122 |
ISNAD | Erdem, Fatma. "S.cerevisiae ile REMOZAL SARI (RR) GİDERİMİNE YAPAY SİNİR AĞI (YSA) YAKLAŞIMI". (2019), 289-297. https://doi.org/10.17482/uumfd.461122 |
APA | Erdem F (2019). S.cerevisiae ile REMOZAL SARI (RR) GİDERİMİNE YAPAY SİNİR AĞI (YSA) YAKLAŞIMI. Uludağ Üniversitesi Mühendislik Fakültesi Dergisi, 24(2), 289 - 297. 10.17482/uumfd.461122 |
Chicago | Erdem Fatma S.cerevisiae ile REMOZAL SARI (RR) GİDERİMİNE YAPAY SİNİR AĞI (YSA) YAKLAŞIMI. Uludağ Üniversitesi Mühendislik Fakültesi Dergisi 24, no.2 (2019): 289 - 297. 10.17482/uumfd.461122 |
MLA | Erdem Fatma S.cerevisiae ile REMOZAL SARI (RR) GİDERİMİNE YAPAY SİNİR AĞI (YSA) YAKLAŞIMI. Uludağ Üniversitesi Mühendislik Fakültesi Dergisi, vol.24, no.2, 2019, ss.289 - 297. 10.17482/uumfd.461122 |
AMA | Erdem F S.cerevisiae ile REMOZAL SARI (RR) GİDERİMİNE YAPAY SİNİR AĞI (YSA) YAKLAŞIMI. Uludağ Üniversitesi Mühendislik Fakültesi Dergisi. 2019; 24(2): 289 - 297. 10.17482/uumfd.461122 |
Vancouver | Erdem F S.cerevisiae ile REMOZAL SARI (RR) GİDERİMİNE YAPAY SİNİR AĞI (YSA) YAKLAŞIMI. Uludağ Üniversitesi Mühendislik Fakültesi Dergisi. 2019; 24(2): 289 - 297. 10.17482/uumfd.461122 |
IEEE | Erdem F "S.cerevisiae ile REMOZAL SARI (RR) GİDERİMİNE YAPAY SİNİR AĞI (YSA) YAKLAŞIMI." Uludağ Üniversitesi Mühendislik Fakültesi Dergisi, 24, ss.289 - 297, 2019. 10.17482/uumfd.461122 |
ISNAD | Erdem, Fatma. "S.cerevisiae ile REMOZAL SARI (RR) GİDERİMİNE YAPAY SİNİR AĞI (YSA) YAKLAŞIMI". Uludağ Üniversitesi Mühendislik Fakültesi Dergisi 24/2 (2019), 289-297. https://doi.org/10.17482/uumfd.461122 |