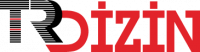
TÜRKÇE METİNLERİN SINIFLANDIRMA BAŞARISINI ARTIRMAK İÇİN YENİ BİR YÖNTEM ÖNERİSİ
Yıl: 2019 Cilt: 24 Sayı: 1 Sayfa Aralığı: 125 - 136 Metin Dili: Türkçe DOI: https://doi.org/10.17482/uumfd.484525 İndeks Tarihi: 30-04-2021
TÜRKÇE METİNLERİN SINIFLANDIRMA BAŞARISINI ARTIRMAK İÇİN YENİ BİR YÖNTEM ÖNERİSİ
Öz: Bu çalışma, yazarı bilinmeyen bir dokümanının yazarını tahmin etmeyi amaçlamaktadır. Bunun için 6 farklı köşe yazarına ait 6 köşe yazısı öncelikle ön-işlem aşamasına sokulmuştur. Ardından bu metinler üzerinden n-gram (2-3) ile özellikler çıkarılmıştır. Çıkarılan özellikler üzerinden sistem 6 farklı makine öğrenmesi üzerinde çapraz geçerleme (10) ile test edilmiştir. Buraya kadar olan kısım literatürde şimdiye kadar uygulanmış olan yöntemdir. Bizim önerimiz ön işlem aşamasının ardından eldeki metinleri LZW algoritması ile kayıpsız sıkıştırarak özellik sayısını azaltmak ve bunun sistemin başarısı üzerindeki etkileri araştırmak üzerinedir. Ön-işlemden geçmiş olan metinler LZW algoritması ile binary (ikili) ve decimal (onlu) olarak sıkıştırılır. Sıkıştırmanın ardından n-gram (2-3) ile çıkarılan özellikler ile sistem 6 farklı makine öğrenmesi yönteminde test edilmiş ve çalışma sonuçları 5 farklı metrik için incelenmiştir. Yapılan çalışma sonucunda ikili olarak sıkıştırılmış metinler hem 2-gram hem de 3-gramda, 6 farklı makine öğrenmesi algoritmasında da daha iyi sonuçlar elde etmiştir. Random Tree ve Naïve bayes algoritmasında onlu sıkıştırma, ham verinin gerisinde kalsa da diğer 4 algoritmada daha iyi elde sonuçlar elde etmiş ama ortalama başarı değerlerinde geride kalmıştır. Yapılan çalışma sonucunda ikili sıkıştırma tüm metriklerinde diğer iki yönteme göre daha başarılıdır. Yapılan çalışmada yazar tanıma işlemi yapılmış olsa da önerilen bu yöntemin tüm metin sınıflandırma işlemlerinde kullanılabileceği düşünülmektedir.
Anahtar Kelime: A Novel Method Proposal to Increase the Classification Success of Turkish Text
Öz: This study aims to estimate the author of an unknown document. For this purpose, first of all, six different columns of 6 different columnists were pre-processed. Then with n-grams (2-3) features were extracted from these texts. The system has been tested with 10-fold cross-validation on 6 different machine learning algorithms. This part of the study is the method that has been applied so far in the literature. Our suggestion is to reduce the number of features with the LZW algorithm and to investigate the effects on the success of the system. The pre-processed texts are compressed binary and decimal with the LZW algorithm. After compression, the system has been tested with 6 different machine learning algorithms, and the study results has been analyzed for 5 different metrics. As a result of the study, the compressed binary text has obtained better results in both 2-gram and 3-gram, for 6 different machine learning algorithms. In the Random-Tree and Naïve Bayes algorithm, decimal compression is behind the raw data. In the other four algorithms, it achieved better results but remained behind the average success values. As a result of the study, binary compression is more successful in all metrics than the other two methods. In the study, although the author recognition process has been done, it can be thought that the proposed method can be used in all text classification procedures.
Anahtar Kelime: Belge Türü: Makale Makale Türü: Araştırma Makalesi Erişim Türü: Erişime Açık
- 1. Amasyalı .M.F. ve Yıldırım T. (2004) Otomatik Haber Metinleri Sınıflandırma, 12.IEEE Sinyal İşleme ve İletişim Uygulamaları Kurultayı, Kuşadası, Aydın, Türkiye, 224-226.
- 2. Amasyalı, M. F. ve Diri, B. (2006) Automatic Turkish Text Categorization in Terms of Author, Genre and Gender, International Conference on Applications of Natural Language to Information Systems, Klagenfurt, Austria, 221-226. Doi: 10.1007/11765448_22
- 3. Amasyalı, M.F., Davletov, F., Arslan, T. ve Çiftçi, Ü. (2010) Text2arff: Automatic feature extraction software for Turkish texts, 18.IEEE Sinyal İşleme ve İletişim Uygulamaları Kurultayı, Diyarbakır, Türkiye, 629-632. Doi: 10.1109/SIU.2010.5651686
- 4. Bekkerman R., El-Yaniv, R., Naftali T. ve Yoad W. (2002) Distributional Word Clusters vs. Words for Text Categorization, Journal of Machine Learning Research, 3,1-48. Doi: 10.1.1.19.7938
- 5. Bilgin, M. (2018) Makine Öğrenmesi Teorisi ve Algoritmaları, Papatya Bilim, İstanbul.
- 6. Bilgin, M. ve Şentürk, İ.F. (2017) Sentiment analysis on Twitter data with semi-supervised Doc2Vec, International Conference Computer Science and Engineering, 661-666. Doi: 10.1109/UBMK.2017.8093492
- 7. Ciya L., Shamim A., ve Paul D. (2001) Feature Preparation in Text Categorization, Oracle Text Selected Papers and Presentations, 1-8.
- 8. Çatal Ç., Erbakırcı K. ve Erenler Y. (2003) Computer-based Authorship Attribution for Turkish Documents, Turkish Symposium on Artificial Intelligence and Neural Networks, Çanakkale,Türkiye, 539-541.
- 9. Çiltik, A. ve Güngör, T. (2008) Time-Efficient Spam E-mail Filtering Using N-gram Models, Pattern Recognition Letters, 29(1), 19-33. Doi: 10.1016/j.patrec.2007.07.018
- 10. Çoban, Ö., Ö. B. ve Özyer, G.T. (2015) Sentiment analysis for Turkish Twitter feeds. 23.IEEE Sinyal İşleme ve İletişim Uygulamaları Kurultayı, Malatya, Türkiye, 2388-2391. Doi: 10.1109/SIU.2015.7130362
- 11. Diri B. ve Amasyalı M.F. (2003) Automatic Author Detection for Turkish Texts, Artificial Neural Networks and Neural Information Processing, İstanbul, Türkiye, 138-141.
- 12. Doğan, S. (2006) Türkçe Dokümanlar için N-gram Tabanlı Sınıflandırma: Yazar, Tür ve Cinsiyet, Yıldız Teknik Üniversitesi, Yüksek Lisans Tezi, İstanbul.
- 13. Doğan, S. ve Diri, B. (2010) Türkçe Dokümanlar İçin N-gram Tabanlı Yeni Bir Sınıflandırma (Ng-ind): Yazar, Tür ve Cinsiyet, Türkiye Bilişim Vakfı Bilgisayar Bilimleri ve Mühendisliği Dergisi, 3(1), 11-19.
- 14. Fattah, M.A. (2017) A Novel Statistical Feature Selection Approach for Text Categorization, Journal of Information Processing Systems, 13(5), 1397-1409. Doi: 10.3745/JIPS.02.0076
- 15. Fürnkranz J. (1998) A Study using n-gram Features for Text Categorization, Austrian Research Institute for Artifical Intelligence, 3(1998), 1-10. Doi: 10.1.1.49.133
- 16. Gaines, B.J. ve Carney, H.E. (2018) Communication and management of electronic mail classification information, U.S. Patent No. 9,942,184.
- 17. Gezici, G. ve Yanıkoğlu, B. (2018) Sentiment Analysis in Turkish, Turkish Natural Language Processing. Springer, Cham, 255-271. Doi: 10.1007/978-3-319-90165-7_12
- 18. Holmes, D. I. (1998) The Evolution of Stylometry in Humanities Scholarship, Literary and Linguistic Computing, 13 (3), 111-117. Doi: 10.1093/llc/13.3.111
- 19. Johnson, R. ve Zhang, T. (2017) Deep pyramid convolutional neural networks for text categorization, 55.Annual Meeting of the Association for Computational Linguistics, Vancouver, Canada,562-570. Doi: 10.18653/v1/P17-1052
- 20. Kemik, (2019). Kemik-Veri Kümelerimiz. Erişim Adresi: http://www.kemik.yildiz.edu.tr/data/File/2500koseyazisi.rar (Erişim Tarihi: 12.02.2019)
- 21. Levent, V.E. ve Diri, B. (2014) Türkçe Dokümanlarda Yapay Sinir Ağları İle Yazar Tanıma, Akademik Bilişim, Mersin, Türkiye.
- 22. Özel, B. (2004) Küresel k-Ortalamalı Gruplama Yöntemi ile Metinlerin ve Terimlerin Saklı Anlam İndekslenmeleri, Akıllı Sistemlerde Yenilikler ve Uygulamaları Konferansı, İstanbul, Türkiye, 223-227.
- 23. Peng F. ve Schuurmans D. (2003) Combining Naive Bayes and N-gram Language Models for Test Classification, European Conference on Information Retrieval, Berlin, Almanya, 335-350. Doi: 10.1.1.2.1184
- 24. Peng F., Keselj V., Cerconey N. ve Thomasy C. (2003) N-Gram-Based Author Profiles For Authorship Attribution, The conference pacific association for computational linguistics Nova Scotia, Canada,255-264. Doi: 10.1.1.9.7388
- 25. Song, F., Liu, S. ve Yang, J. (2005) A comparative study on text representation schemes in text categorization, Pattern analysis and applications, 8(1-2), 199–209. Doi: 10.1007/s10044-005-0256-3
- 26. Stamatatos E., Fakotakis N. ve Kokkinakis G. (2000) Automatic Text Categorization in Terms of Genre and Author, Computational Linguistics, 26(4), 471-495. Doi: 10.1162/089120100750105920
- 27. Tan C.M., Wang Y.F. ve Lee C.D. (2002) The Use of Bi-grams to Enhance Text Categorization, Journal Information Processing and Management, 30(4), 529-546. Doi: 10.1016/S0306-4573(01)00045-0
- 28. Türkoğlu F., Diri B. ve Amasyalı M.F. (2006) Farklı Özellik Vektörleri ile Türkçe Dokümanların Yazarlarının Belirlenmesi, Turkish Symposium on Artificial Intelligence and Neural Networks, Muğla, Türkiye.
- 29. Türkoğlu, F., Diri, B. ve Amasyalı, M. F. (2007) Author Attribution of Turkish Texts by Feature Mining, 3.International Conference on Intelligent Computing, Qingdao, China, 1086-1093. Doi: 10.1007/978-3-540-74171-8_110
- 30. Welch, T. A. (1984) A Technique for High-Performance Data Compression, IEEE Computer, 17(6), 8-19. Doi: 10.1109/MC.1984.1659158
APA | Bilgin M (2019). TÜRKÇE METİNLERİN SINIFLANDIRMA BAŞARISINI ARTIRMAK İÇİN YENİ BİR YÖNTEM ÖNERİSİ. , 125 - 136. https://doi.org/10.17482/uumfd.484525 |
Chicago | Bilgin Metin TÜRKÇE METİNLERİN SINIFLANDIRMA BAŞARISINI ARTIRMAK İÇİN YENİ BİR YÖNTEM ÖNERİSİ. (2019): 125 - 136. https://doi.org/10.17482/uumfd.484525 |
MLA | Bilgin Metin TÜRKÇE METİNLERİN SINIFLANDIRMA BAŞARISINI ARTIRMAK İÇİN YENİ BİR YÖNTEM ÖNERİSİ. , 2019, ss.125 - 136. https://doi.org/10.17482/uumfd.484525 |
AMA | Bilgin M TÜRKÇE METİNLERİN SINIFLANDIRMA BAŞARISINI ARTIRMAK İÇİN YENİ BİR YÖNTEM ÖNERİSİ. . 2019; 125 - 136. https://doi.org/10.17482/uumfd.484525 |
Vancouver | Bilgin M TÜRKÇE METİNLERİN SINIFLANDIRMA BAŞARISINI ARTIRMAK İÇİN YENİ BİR YÖNTEM ÖNERİSİ. . 2019; 125 - 136. https://doi.org/10.17482/uumfd.484525 |
IEEE | Bilgin M "TÜRKÇE METİNLERİN SINIFLANDIRMA BAŞARISINI ARTIRMAK İÇİN YENİ BİR YÖNTEM ÖNERİSİ." , ss.125 - 136, 2019. https://doi.org/10.17482/uumfd.484525 |
ISNAD | Bilgin, Metin. "TÜRKÇE METİNLERİN SINIFLANDIRMA BAŞARISINI ARTIRMAK İÇİN YENİ BİR YÖNTEM ÖNERİSİ". (2019), 125-136. https://doi.org/https://doi.org/10.17482/uumfd.484525 |
APA | Bilgin M (2019). TÜRKÇE METİNLERİN SINIFLANDIRMA BAŞARISINI ARTIRMAK İÇİN YENİ BİR YÖNTEM ÖNERİSİ. Uludağ Üniversitesi Mühendislik Fakültesi Dergisi, 24(1), 125 - 136. https://doi.org/10.17482/uumfd.484525 |
Chicago | Bilgin Metin TÜRKÇE METİNLERİN SINIFLANDIRMA BAŞARISINI ARTIRMAK İÇİN YENİ BİR YÖNTEM ÖNERİSİ. Uludağ Üniversitesi Mühendislik Fakültesi Dergisi 24, no.1 (2019): 125 - 136. https://doi.org/10.17482/uumfd.484525 |
MLA | Bilgin Metin TÜRKÇE METİNLERİN SINIFLANDIRMA BAŞARISINI ARTIRMAK İÇİN YENİ BİR YÖNTEM ÖNERİSİ. Uludağ Üniversitesi Mühendislik Fakültesi Dergisi, vol.24, no.1, 2019, ss.125 - 136. https://doi.org/10.17482/uumfd.484525 |
AMA | Bilgin M TÜRKÇE METİNLERİN SINIFLANDIRMA BAŞARISINI ARTIRMAK İÇİN YENİ BİR YÖNTEM ÖNERİSİ. Uludağ Üniversitesi Mühendislik Fakültesi Dergisi. 2019; 24(1): 125 - 136. https://doi.org/10.17482/uumfd.484525 |
Vancouver | Bilgin M TÜRKÇE METİNLERİN SINIFLANDIRMA BAŞARISINI ARTIRMAK İÇİN YENİ BİR YÖNTEM ÖNERİSİ. Uludağ Üniversitesi Mühendislik Fakültesi Dergisi. 2019; 24(1): 125 - 136. https://doi.org/10.17482/uumfd.484525 |
IEEE | Bilgin M "TÜRKÇE METİNLERİN SINIFLANDIRMA BAŞARISINI ARTIRMAK İÇİN YENİ BİR YÖNTEM ÖNERİSİ." Uludağ Üniversitesi Mühendislik Fakültesi Dergisi, 24, ss.125 - 136, 2019. https://doi.org/10.17482/uumfd.484525 |
ISNAD | Bilgin, Metin. "TÜRKÇE METİNLERİN SINIFLANDIRMA BAŞARISINI ARTIRMAK İÇİN YENİ BİR YÖNTEM ÖNERİSİ". Uludağ Üniversitesi Mühendislik Fakültesi Dergisi 24/1 (2019), 125-136. https://doi.org/https://doi.org/10.17482/uumfd.484525 |