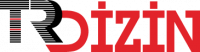
Görsel Sanatlar-Yapay Zekâ İş Birliğine Yönelik İşlevsel Sınıflandırma Derlemesi
Yıl: 2020 Cilt: 15 Sayı: 3 Sayfa Aralığı: 373 - 388 Metin Dili: Türkçe DOI: 10.47844/TurkishStudies.43972 İndeks Tarihi: 05-05-2021
Görsel Sanatlar-Yapay Zekâ İş Birliğine Yönelik İşlevsel Sınıflandırma Derlemesi
Öz: Yapay zekâ, alt alanları olan makine öğrenmesi ve hesaplamalı yaratıcılık uygulamaları aracılığıyla; görüntüleri tanıyıp değerlendirebilmekte ve bunları sentezleyipyenigörüntülerüretebilmektedir.Bu uygulamalar; sanatçıları ve eserlerini yaratıcılık skorları bakımından sınıflandırabilmekte, estetik değerlendirme yapabilmekte, artistik üslupları analiz edebilmektedir. Böylece ekspertiz yapabilmeyi ve sahteciliğin engellenmesini kolaylaştırmaktadır. Bahsedilen işlevleriyle, restorasyon çalışmalarında ya da dijital sanat arşivlerinin düzenlenmesinde kılavuz araç olarak kullanılmaktadır. Yapay zekâ, yaratıcı ve estetik sanat yapıtları ortaya koyabilme potansiyeline sahiptir. Stil transferi, duygusal modelleme, metinden görüntü üretimi yapabilmekte; basit eskizleri perspektif ve doku ekleyerek fotogerçekçi hale getirebilmektedir.Bu algoritmalar, çevrimiçi veri tabanlarına erişerek; oluşturacağı esere ilişkin kaynaklardan derleme yapabilmekte, kendi kendini geliştirebilmekte ya da daha gelişmiş versiyonlarını üretebilmektedir. Bir sanatçının veya akımın karakteristik özelliklerini taşıyan ya da mevcut üslupların dışında benzersiz nitelikteki yapay zekâ ürünü eserler, müze ve galerilerde izleyici karşısına çıkmaktadır. Modern hayatın geniş bir bölümünde etki yaratan bu teknoloji; sanatın sınır deneyimlerine ulaşmakta, özgün bileşkeler oluşturmakta faydalanılabilecek, deneyselliğe açık bir araçtır. Çağdaş sanatçının kimliğinin ya da sanat eserinin sorgulanması, modern kavramların irdelenmesi için yeni bir alandır. Yapay zekâ, çağın ruhunu sanat eserine yansıtabilecek gelişmiş bir asistan ve kavramsal sanatın yeni anlatım biçimidir.Çalışma, görsel sanatlar-yapay zekâ iş birlikli araştırma ve projelerin sınıflandırılması amacıyla hazırlanmıştır. Literatür taraması neticesinde, bahsedilençalışmaların; sanat eseri analizi ya da eser sentezleme olmak üzere iki temel işlevden birisini açıklamak üzere hazırlandığı görülmüştür. Çalışma bu doğrultuda şekillendirilmiş olup; yapay zekânın sanat eserlerini analiz etme biçimlerinin ardından,görüntü sentezleme biçimleri açıklanmıştır.
Anahtar Kelime: Functional Classification Compilation for the Visual Arts-Artificial Intelligence Collaboration
Öz: Artificial intelligence applications can recognize and evaluate the images and generate new ones by synthesizing them with the help of its subfields –the machine learningand computational creativity-. These applicationsarecapable of classifying artists and their works in terms of creativity scores, evaluating aesthetics or analyze artistic styles; thus, makes it easier to make expertise and prevent forgery. It is also used as a guiding vehicle in the restorations or digital archieve organization processes with its mentioned properties.Artificial intelligence has a potential to produce creative and aesthetic artworks. It can make style transfer, emotional modelling, text-to-image generation or create photorealistic images from sketches by adding them perspective and texture. By accessing online databases, these algorithms are capable of compiling from the resources related to the work it will create, improving itself or producing more advanced versions of itself.Artificialintelligence artifacts with the characteristic of an artist or a movement, or of a unique quality outside of the existing styles, are being exhibited at museums and galleries.Having an impact on a large part of modern life, this technology is atoolopento experimentation which can be used to reach the limits of artistic experiences and to create original components. It is a new areafor examining the identity of the contemporary artist and work of art, and for explicating modern concepts. Artificial intelligence is an advanced assistant which is capable of reflecting the spirit of the age to the work of art; and is the novel form of expression for conceptual art.This study aims to classify the researches and projects of visual arts-artificial intelligence collaboration. As a result of the literature review, it was determined that the mentioned works were prepared to perform one of the two main functions: artwork analysis or synthesis. This study is shaped accordingly; followingthe section on theartificial intelligence’s methods of artistic analysis, its image synthesizing methods are explained.
Anahtar Kelime: Belge Türü: Makale Makale Türü: Araştırma Makalesi Erişim Türü: Erişime Açık
- Ackerman, M., Goel, A., Johnson, C. G., Jordanous, A., Leon, C., Perez, R. P., Toivonen, H. & Ventura, D. (2017). Teaching computational creativity. (Eds.) A. Goel, A. Jordanous & A. Pease, Eighth International Conference on Computational Creativity. (ss. 9-16). Georgia Institute of Technology.
- Alpaydın, E. (2016). Machine learning: The new AI. MIT Press.
- Bo, Y., Yu, J. & Zhang, K. (2018). Computational aesthetics and applications. Visual Computing for Industry, Biomedicine, and Art, 1(6). 1-19. https://doi.org/10.1186/s42492-018-0006-1
- Cellan-Jones, R. (2016, Ekim 20). Stephen Hawking: Will AI kill or save humankind? BBC News. https://www.bbc.com/news/technology-37713629
- Colton, S. (2012). The painting fool: Stories from building and automated painter. (Eds.) J. McCormack & M. d’Inverno, Computers and Creativity. (ss. 3-38). Springer.
- Çimen, Ü. (2020). Analog fotoğraftan dijital fotoğrafa geçişte fotoğrafik gerçekliğin dönüşümü, Turkish Studies-Applied Sciences, 15(1), 15-37. https://dx.doi.org/10.29228/TurkishStudies.40533
- Deepcoder (2017). https://www.microsoft.com/en-us/research/publication/deepcoder-learningwrite- programs/. Erişim tarihi: 13.05.2020.
- Elgammal, A. & Saleh, B. (2015). Quantifiying creativity in art networks. (Eds.) H. Toivonen, S. Colton, M. Cook & D. Ventura. Sixth International Conference on Computational Creativity (ss. 39-46). Brigham Young University.
- Elgammal, A., Liu, B., Elhoseiny & M., Mazzone, M. (2017). CAN: Creative adversarial networks generating art by learning about styles and deviating from style norms. (Eds.) A. Goel, A. Jordanous & A. Pease. Eighth International Conference on Computational Creativity (ss.96- 103). Georgia Institute of Technology.
- Elgammal, A., Kang, Y. & Leeuw, M. D. (Şubat 2018). Picasso, Matisse, or a Fake? Automated analysis of drawings at the stroke level for attribution and authentication. AAAI Conference on Artificial Intelligence. New Orleans.
- Gatys, L. A., Ecker, A. S. & Bethge. M. (Haziran 2016). Image style transfer using convolutional neural networks. IEEE Conference on Computer Vision and Pattern Recognition, Las Vegas.
- Gelecek Rembrandt. (2016). https://www.nextrembrandt.com. Erişim tarihi: 07.08.2019.
- Gülzow, J. M., Gravyer, L. & Deussen, O. (2018). Self-improving robotic brushstroke replication. Arts, 7(84), 1-28. https://doi.org/10.3390/arts7040084
- Hertzmann, A. (2018). Can computers create art? Arts, 7(18), 1-25. https:// doi.org/10.3390/arts7020018
- Jacobs, R. A. & Bates, C. J. (2018). Comparing the visual representations and performance of humans and deep neural networks. Current Directions in Psychological Science, 28(1), 1-6. https://doi.org/10.1177%2F0963721418801342
- Knowles, K. (2017, Kasım 21). This AI spots art fakes in a single brush stroke. http://authenticationinart.org/pdf/artmarket/ai-forgery-detective.pdf Erişim tarihi: 11.10.2019.
- Li, Y., Zhang, K. & Li, D. (2017). Rule-based automatic generation of logo design. Leonardo, 50(2), 177-181. https://doi.org/10.1162/LEON_a_00961
- Marr, B. (2019, Haziran 21). Meet Hemingway: The artificial intelligence robot that can copy your handwriting. Forbes. https://www.forbes.com/sites/bernardmarr/2019/06/21/meethemingway- the-artificial-intelligence-robot-that-can-copy-yourhandwriting/# 9de9d7e4dabe Erişim Tarihi: 22.12.2019.
- Nilsson, N. J. (2019). Yapay zekâ: Geçmişi ve geleceği (2. baskı). Boğaziçi Üniversitesi Yayınevi.
- Norton, D., Heath, D. & Ventura, D. (2013). Finding creativity in an artificial artist. The Journal of Creative Behavior, 47(2), 106-124. https://doi.org/10.1002/jocb.27
- Park, T., Liu, M, Wang, T. & Zhu, J. (Haziran 2019). Semantic image synthesis with spatiallyadaptive normalization. CVPR 2019 Conference, Long Beach, CA.
- Re, R. M. & Solow-Niederman, A. (2019). Developing Artificially Intelligent Justice. Stanford Technology Law Review, 22(2), 242-289.
- Ridler, A. (2017). Misremembering and Mistranslating: the Associations of GANs in an Art Context. 31st Conference on Neural Information Processing Systems, Long Beach, CA, USA.
- Sabetsarvestani, Z., Sober, B., Higgitt, C., Daubechies, I. & Rodrigues, M.R.D. (2019). Artificial intelligence for art investigation: Meeting the challenge of separating X-ray images of the Ghent Altarpiece. Science Advances, 5(8), 1-8. https://doi.org/10.1126/sciadv.aaw7416
- Saleh, B., Abe, K., Arora, R. S. & Elgammal, A. (2014). Toward automated discovery of artistic influence. Multimed Tools Appl, 75(7), 3565–3591. https://doi.org/10.1007/s11042-014- 2193-x
- Smith, G. W. & Leymarie, F. F. (2017). The machine as artist: An introduction. Arts, 6(5), 1-7. https://doi.org/10.3390/arts6020005
- Tao, W., Liu, Y. & Zhang, K. (2014). Automatically generating abstract paintings in Malevich style. (Eds.) Y. Hang, W. Song, S. Xu, L. Chen & R. Lee. International Conference on Computer and Information Science 2014 (ss. 201-205). Taiyuan, China.
- Xiong, L. & Zhang, K. (Eylül 2016). Generation of Miro’s surrealism. (Eds.) A. Kerren & K. Zhang. VINCI '16: 9th International Symposium on Visual Information Communication and Interaction (ss. 130-137), Dallas.
- Xu, T., Zhang, P., Huang, Q., Zhang, H., Gan, Z., Huang, X. & He, X. (Haziran 2018). AttnGAN: Fine-grained text to image generation with attentional generative adversarial networks. 2018 IEEE/CVF Conference on Computer Vision and Pattern Recognition (ss. 1316-1324), Utah.
- Yanisky-Ravid, S. (2017). Generating Rembrandt: Artificial intelligence, copyright, and accountability in the 3A era —the human-like authors are already here —a new model. Michigan State Law Review, 4, 659-726. https://dx.doi.org/10.2139/ssrn.2957722
- y Arcas, B. A. (2017). Art in the age of machine intelligence. Arts, 6(18), 1-9. https://doi:10.3390/arts6040018
- Yılmaz, M. (2006). Modernizmden Postmodernizme sanat. Ütopya Yayınevi.
- Zhang, K. & Yu, J. (Kasım 2013). Generating abstract paintings in Kandinsky style. SIGGRAPH Asia 2013 Conference, No: 18, Hong Kong.
- Zhang, K. & Yu, J. (2016). Generation of Kandinsky art. Leonardo, 49(1), 48–54. https://doi.org/10.1162/LEON_a_00908
- Zheng, Y., Nie, X., Meng, Z., Feng, W. & Zhang, K. (2015). Layered modeling and generation of Pollock’s drip style. The Visual Computer, 31(5), 589–600. https://doi.org/10.1007/s00371- 014-0985-7
APA | YAKAR G (2020). Görsel Sanatlar-Yapay Zekâ İş Birliğine Yönelik İşlevsel Sınıflandırma Derlemesi. , 373 - 388. 10.47844/TurkishStudies.43972 |
Chicago | YAKAR GÜLLÜ Görsel Sanatlar-Yapay Zekâ İş Birliğine Yönelik İşlevsel Sınıflandırma Derlemesi. (2020): 373 - 388. 10.47844/TurkishStudies.43972 |
MLA | YAKAR GÜLLÜ Görsel Sanatlar-Yapay Zekâ İş Birliğine Yönelik İşlevsel Sınıflandırma Derlemesi. , 2020, ss.373 - 388. 10.47844/TurkishStudies.43972 |
AMA | YAKAR G Görsel Sanatlar-Yapay Zekâ İş Birliğine Yönelik İşlevsel Sınıflandırma Derlemesi. . 2020; 373 - 388. 10.47844/TurkishStudies.43972 |
Vancouver | YAKAR G Görsel Sanatlar-Yapay Zekâ İş Birliğine Yönelik İşlevsel Sınıflandırma Derlemesi. . 2020; 373 - 388. 10.47844/TurkishStudies.43972 |
IEEE | YAKAR G "Görsel Sanatlar-Yapay Zekâ İş Birliğine Yönelik İşlevsel Sınıflandırma Derlemesi." , ss.373 - 388, 2020. 10.47844/TurkishStudies.43972 |
ISNAD | YAKAR, GÜLLÜ. "Görsel Sanatlar-Yapay Zekâ İş Birliğine Yönelik İşlevsel Sınıflandırma Derlemesi". (2020), 373-388. https://doi.org/10.47844/TurkishStudies.43972 |
APA | YAKAR G (2020). Görsel Sanatlar-Yapay Zekâ İş Birliğine Yönelik İşlevsel Sınıflandırma Derlemesi. Turkish Studies - Information Technologies and Applied Sciences, 15(3), 373 - 388. 10.47844/TurkishStudies.43972 |
Chicago | YAKAR GÜLLÜ Görsel Sanatlar-Yapay Zekâ İş Birliğine Yönelik İşlevsel Sınıflandırma Derlemesi. Turkish Studies - Information Technologies and Applied Sciences 15, no.3 (2020): 373 - 388. 10.47844/TurkishStudies.43972 |
MLA | YAKAR GÜLLÜ Görsel Sanatlar-Yapay Zekâ İş Birliğine Yönelik İşlevsel Sınıflandırma Derlemesi. Turkish Studies - Information Technologies and Applied Sciences, vol.15, no.3, 2020, ss.373 - 388. 10.47844/TurkishStudies.43972 |
AMA | YAKAR G Görsel Sanatlar-Yapay Zekâ İş Birliğine Yönelik İşlevsel Sınıflandırma Derlemesi. Turkish Studies - Information Technologies and Applied Sciences. 2020; 15(3): 373 - 388. 10.47844/TurkishStudies.43972 |
Vancouver | YAKAR G Görsel Sanatlar-Yapay Zekâ İş Birliğine Yönelik İşlevsel Sınıflandırma Derlemesi. Turkish Studies - Information Technologies and Applied Sciences. 2020; 15(3): 373 - 388. 10.47844/TurkishStudies.43972 |
IEEE | YAKAR G "Görsel Sanatlar-Yapay Zekâ İş Birliğine Yönelik İşlevsel Sınıflandırma Derlemesi." Turkish Studies - Information Technologies and Applied Sciences, 15, ss.373 - 388, 2020. 10.47844/TurkishStudies.43972 |
ISNAD | YAKAR, GÜLLÜ. "Görsel Sanatlar-Yapay Zekâ İş Birliğine Yönelik İşlevsel Sınıflandırma Derlemesi". Turkish Studies - Information Technologies and Applied Sciences 15/3 (2020), 373-388. https://doi.org/10.47844/TurkishStudies.43972 |