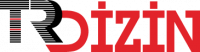
Uydu verilerinden karar ağaçları kullanarak orman yangını tahmini
Yıl: 2020 Cilt: 11 Sayı: 3 Sayfa Aralığı: 899 - 906 Metin Dili: Türkçe DOI: 10.24012/dumf.661925 İndeks Tarihi: 01-06-2021
Uydu verilerinden karar ağaçları kullanarak orman yangını tahmini
Öz: Tüm canlılık için önemli olan ormanların, yangınlar ile yok edilmesi doğaya verdiği zararın yanı sıra can ve malgüvenliğini de ciddi şekilde tehdit eder. Orman yangınları doğal yollarla veya bilinçli insan davranışları ilegerçekleşir. Orman yangınlarının önceden tahmini veya erken keşfi hızlı müdahale ve önlem almayı sağlayacaktır.Literatürde orman yangınlarını tahmin etmek için meteorolojik verileri ve uzaktan algılama verilerikullanılmaktadır. Bununla birlikte meteorolojik veriler ile mevcut orman yangının davranışı dabelirlenebilmektedir. Bu çalışmada uydulardan alınan veriler ile orman yangınlarının tahmini yapılmıştır.Uydudan alınan verilerden hesaplanan Normalize Edilmiş Fark Bitki Örtüsü İndeksi (NVDI), Arazi YüzeyiSıcaklığı (LST) ve Termal anomali (TA) verileri kullanılarak orman yangınları tahmin edilmiştir. Bahsedilenverilerden tahmin yapmak için karar ağaçları kullanılmıştır. Karar ağaçlarının eğitiminde kullanılmak için verisetindeki verilerin %70’ i kullanılmıştır. Geri kalan %30 veri ile oluşturulan modelin testi gerçekleştirilmiştir.Eğitim ve test işlemi farklı veriler ile 10 defa tekrarlı yapılarak uygulanan yöntemin ortalama performansıbelirlenmiştir. Gerçekleştirilen denemelerde ortalama %98,62 duyarlılık oranı ile gerçekleşen yangınlar doğrutahmin edilmiştir. Tüm denemelerde yapılan tahminler için ortalama %93,11 doğruluk ile gerçek durumbelirlenmiştir.
Anahtar Kelime: Prediction of wildfire using decision trees from satellite data
Öz: Destruction of forests, which are important for all vitality, with fires, seriously threatens the safety of life and property. Wildfires occur by natural ways and conscious human behavior. The prediction and early detection of wildfires will allow for rapid intervention and prevention. In the literature, meteorological data and remote sensing data were used to predict forest fires. In addition, by using meteorological data, the behavior of existing wildfires could also be determined. In this study, wildfires were predicted from data received from satellites. Wildfires were predicted by using Normalized Differential Vegetation Index (NVDI), Land Surface Temperature (LST) and Thermal Anomaly (TA) data calculated from downloaded satellite data. Decision trees were used to make predictions using mentioned data. 70% of the data in the data set were used for the training of decision trees. The trained model was tested with the remaining 30% data. Training and testing process were repeated 10 times with different data and the average performance of the proposed model was determined. Occurred fires were correctly predicted on the average of 98.62% sensitivity in the experiments. For the predictions in all trials, the actual situation was determined with an average of 93.11% accuracy
Anahtar Kelime: Belge Türü: Makale Makale Türü: Araştırma Makalesi Erişim Türü: Erişime Açık
- [1] Dayananda P. W. A., “Stochastic models for forest fires”, Ecological Modeling, Volume 3 (1977), 309-313.
- [2] Altan, G., Türkeş, M., Tatlı, H., “Çanakkale ve Muğla 2009 yılı orman yangınlarının KeetchByram Kuraklık İndisi ile klimatolojik ve meteorolojik analizi.” In: 5th Atmospheric Science Symposium Proceedings Book: 263-274. Istanbul Technical University, 27-29 April 2011, Istanbul. Turkey. [3] Türkiye Cumhuriyeti Orman genel müdürlüğü,
- https://www.ogm.gov.tr/ekutuphane/Sayfalar/Ist atistikler.aspx (Erişim 10/01/2019)
- [4] C.S. Eastaugh, H. Hasenauer, “Deriving forest fire ignition risk with biogeochemical process modelling Environ.” Model. Softw., 55 (2014), pp. 132-142
- [5] Tedim F., Leone V., Amraoui M., Bouillon C., Coughlan M., Delogu G., Fernandes P., Ferreira C., McCaffrey S., McGee T., Parente J., Paton D., Pereira M., Ribeiro L., Viegas D., Xanthopoulos G., “Defining extreme wildfire events: difficulties, challenges, and impacts Fire” 1 (1) (2018), p. 9
- [6] Gümüşçü A., Tenekeci M. E., “Estimation of active sperm count in spermiogram using motion detection methods”, Journal of the Faculty of Engineering and Architecture of Gazi University (2018), https://doi.or./10.17341/gazimmfd.460524
- [7] Karadağ K., Tenekeci M.E., Taşaltın R., Bilgili A., “Detection of pepper fusarium disease using machine learning algorithms based on spectral reflectance”, Sustainable Computing: Informatics and Systems, (2019), Article in Press.
- [8] Gümüşçü A., Tenekeci M.E., Bilgili A.V. “Estimation of wheat planting date using machine learning algorithms based on available climate data”, (2019) Sustainable Computing: Informatics and Systems. Article in Press.
- [9] Lejdel B., “Conceptual Framework for Analyzing Knowledge in Social Big Data.”, Proceeding Big Data and Smart Digital Environment. ICBDSDE (2018). Studies in Big Data, vol 53, pp 347-358.
- [10]Chi M., Plaza A., Benediktsson J.A., Sun Z., Shen J., Zhu Y., “Big data for remote sensing: challenges and opportunities”, Proc. IEEE, 104 (11) (2016), pp. 2207-2219
- [11]Ramapriyan H., Brennan J., Walter J., Behnke J., “Managing big Data: NASA tackles complex Data challenges”, Earth Imaging J. (2013) [Online].
- [12]Padilla M., Vega-García C. (2011) On the comparative importance of fire danger rating indices and their integration with spatial and temporal variables for predicting daily humancaused fire occurrences in Spain. International Journal of Wildland Fire 20, 46-58.
- [13]Cheng T., Wang J., “Integrated spatio-temporal data mining for forest fire prediction”, Trans. GIS, 12 (5) (2008), pp. 591-611
- [14]Satir O., Berberoglu S., Donmez C., “Mapping regional forest fire probability using artificial neural network model in a Mediterranean forest ecosystem Geomatics”, Natural Hazards and Risk (2015), pp. 1-14
- [15]Dieu Tien Bui, Quang-Thanh Bui, Quoc-Phi Nguyen, Biswajeet Pradhan, Haleh Nampak, Phan Trong Trinh,"A hybrid artificial intelligence approach using GIS-based neural-fuzzy inference system and particle swarm optimization for forest fire susceptibility modeling at a tropical area",Agricultural and Forest Meteorology, Volume 233, (2017), Pages 32-44,ISSN 0168- 1923.
- [16]Arpaci A., Malowerschnig B., Sass O., Vacik H., “Using multi variate data mining techniques for estimating fire susceptibility of Tyrolean”, forests Appl. Geogr., 53 (2014), pp. 258-270
- [17]Castelli M., Vanneschi L., Popovic A., “Predicting burned areas of forest fires: an artificial intelligence approach”, Fire Ecol., 11 (2015), pp. 106-118
- [18]Arrue B., Ollero A., Matinez de Dios J., “An intelligent system for false alarm reduction in infrared forest-fire detection”, IEEE Intell. Syst., 15 (3) (2000), pp. 64-73
- [19]Cortez P., Morais A., “A data mining approach to predict wildfires using meteorological data”, Proc. 13th Port. Conf. Artif. Intell (2007), pp. 512-523
- [20]Sayad, Y. O., Mousannif, H., Moatassime, H. A., “Predictive modeling of wildfires: A new dataset and machine learning approach”, Fire Safety Journal, Volume 104, (2019), Pages 130-146
- [21]The Canadian Wildland Fire Information System (CWFIS) http://cwfis.cfs.nrcan.gc.ca/.
- [22]MODIS data products, Courtesy of the NASA Land Processes Distributed Active Archive Center (LP DAAC), USGS/Earth Resources Observation and Science (EROS) Center, Sioux Falls, South Dakota, https://lpdaac.usgs.gov.
- [23]Quinlan J.R., “C4.5: Programs for Machine Learning”, Morgan Kaufmann, San Mateo, CA, (1993),302 s
- [24]Zhang J., Gruenwald L., Gertz M., VDM-RS: A visual data mining system for exploring and classifying remotely sensed images,Computers & Geosciences, Volume 35, Issue 9, (2009),Pages 1827-1836, ISSN 0098-3004, https://doi.org/10.1016/j.cageo.2009.02.006.
- [25]Hansen, M. C., Stehman, S. V., Potapov, P. V., Arunarwati, B., Stolle, F., & Pittman, K. "Quantifying changes in the rates of forest clearing in Indonesia from 1990 to 2005 using remotely sensed data sets." Environmental Research Letters 4.3 (2009): 034001.
- [26]Pal M., Mather P.M., “An assessment of the effectiveness of decision tree methods for land cover classification”, Remote Sensing of Environment, 86, (2003), 554-565
- [27]Li Z., Dunham M.H., Xiao Y., Zaïane O.R., Simoff S.J., Djeraba C. (Eds.), “STIFF: a forecasting framework for SpatioTemporal data”, Mining Multimedia and Complex Data. PAKDD 2002. Lecture Notes in Computer Science, vol. 2797, Springer, Berlin, Heidelberg (2003)
- [28]Cheng T., Wang J., “Integrated spatiotemporal Data Mining for forest fire prediction”, Trans. GIS, 12 (5) (2008), pp. 591-611
APA | BEŞLİ N, TENEKECI M (2020). Uydu verilerinden karar ağaçları kullanarak orman yangını tahmini. , 899 - 906. 10.24012/dumf.661925 |
Chicago | BEŞLİ Nurettin,TENEKECI MEHMET EMIN Uydu verilerinden karar ağaçları kullanarak orman yangını tahmini. (2020): 899 - 906. 10.24012/dumf.661925 |
MLA | BEŞLİ Nurettin,TENEKECI MEHMET EMIN Uydu verilerinden karar ağaçları kullanarak orman yangını tahmini. , 2020, ss.899 - 906. 10.24012/dumf.661925 |
AMA | BEŞLİ N,TENEKECI M Uydu verilerinden karar ağaçları kullanarak orman yangını tahmini. . 2020; 899 - 906. 10.24012/dumf.661925 |
Vancouver | BEŞLİ N,TENEKECI M Uydu verilerinden karar ağaçları kullanarak orman yangını tahmini. . 2020; 899 - 906. 10.24012/dumf.661925 |
IEEE | BEŞLİ N,TENEKECI M "Uydu verilerinden karar ağaçları kullanarak orman yangını tahmini." , ss.899 - 906, 2020. 10.24012/dumf.661925 |
ISNAD | BEŞLİ, Nurettin - TENEKECI, MEHMET EMIN. "Uydu verilerinden karar ağaçları kullanarak orman yangını tahmini". (2020), 899-906. https://doi.org/10.24012/dumf.661925 |
APA | BEŞLİ N, TENEKECI M (2020). Uydu verilerinden karar ağaçları kullanarak orman yangını tahmini. Dicle Üniversitesi Mühendislik Fakültesi Mühendislik Dergisi, 11(3), 899 - 906. 10.24012/dumf.661925 |
Chicago | BEŞLİ Nurettin,TENEKECI MEHMET EMIN Uydu verilerinden karar ağaçları kullanarak orman yangını tahmini. Dicle Üniversitesi Mühendislik Fakültesi Mühendislik Dergisi 11, no.3 (2020): 899 - 906. 10.24012/dumf.661925 |
MLA | BEŞLİ Nurettin,TENEKECI MEHMET EMIN Uydu verilerinden karar ağaçları kullanarak orman yangını tahmini. Dicle Üniversitesi Mühendislik Fakültesi Mühendislik Dergisi, vol.11, no.3, 2020, ss.899 - 906. 10.24012/dumf.661925 |
AMA | BEŞLİ N,TENEKECI M Uydu verilerinden karar ağaçları kullanarak orman yangını tahmini. Dicle Üniversitesi Mühendislik Fakültesi Mühendislik Dergisi. 2020; 11(3): 899 - 906. 10.24012/dumf.661925 |
Vancouver | BEŞLİ N,TENEKECI M Uydu verilerinden karar ağaçları kullanarak orman yangını tahmini. Dicle Üniversitesi Mühendislik Fakültesi Mühendislik Dergisi. 2020; 11(3): 899 - 906. 10.24012/dumf.661925 |
IEEE | BEŞLİ N,TENEKECI M "Uydu verilerinden karar ağaçları kullanarak orman yangını tahmini." Dicle Üniversitesi Mühendislik Fakültesi Mühendislik Dergisi, 11, ss.899 - 906, 2020. 10.24012/dumf.661925 |
ISNAD | BEŞLİ, Nurettin - TENEKECI, MEHMET EMIN. "Uydu verilerinden karar ağaçları kullanarak orman yangını tahmini". Dicle Üniversitesi Mühendislik Fakültesi Mühendislik Dergisi 11/3 (2020), 899-906. https://doi.org/10.24012/dumf.661925 |