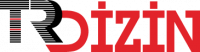
Modelling of Effects of Various Chip Breaker Forms on Surface Roughness in Turning Operations by Utilizing Artificial Neural Networks
Yıl: 2016 Cilt: 19 Sayı: 1 Sayfa Aralığı: 71 - 83 Metin Dili: İngilizce DOI: 10.2339/2016.19.1 71-83 İndeks Tarihi: 20-06-2021
Modelling of Effects of Various Chip Breaker Forms on Surface Roughness in Turning Operations by Utilizing Artificial Neural Networks
Öz: In this study, the effects of different chip breaker forms and cutting parameters on the surface roughness on machined surfaceswere investigated experimentally in turning of AISI 1050 steel; and values of surface roughness obtained from experiments weredetermined with empirical equations using artificial neural networks. The utilizing of ANN was offered to determine the surfaceroughness depending on chip breaker forms and cutting parameters of AISI 1050 steel. The back propagation learning algorithmand fermi transfer function were used in artificial neural network. Experimental measurements data were employed as trainingand test data in order to train the neural network created. The best fitting training data set was attained with ten neurons in twohidden layers 6 of which were at first hidden layer and 4 of which were at second hidden layer, making it possible to predictsurface roughness with precision at least as good as that of the experimental error over the entire experimental range. Afternetwork training, R2value was found as 0.978, and average error as 0.018%. When the results of mathematical modelling areexamined, the computed surface roughness is observed to be apparently within acceptable values.
Anahtar Kelime: Tornalama Operasyonlarında Farklı Talaş Kırıcı Formlarının Yüzey Pürüzlülüğü Üzerinde Etkilerinin Yapay Sinir Ağları Kullanılarak Modellenmesi
Öz: Bu çalışmada, AISI 1050 çeliğinin tornalanmasında, farklı talaş kırıcı formlarının ve kesme parametrelerinin işlenmiş yüzeylerdeki yüzey pürüzlülüğü üzerinde etkileri deneysel olarak araştırılmış ve deneylerden elde edilen yüzey pürüzlülük değerleri yapay sinir ağları kullanılarak ampirik eşitlikler ile belirlenmiştir. AISI 1050 çeliğinin talaş kırıcı formlarına ve kesme parametrelerine bağlı olarak yüzey pürüzlülüğünü belirlemek için yapay sinir ağların kullanımı önerilmiştir. Yapay sinir ağında geri yayılım öğrenme algoritması ve fermi transfer fonksiyonu kullanılmıştır. Oluşturulan sinir ağını eğitmek amacıyla eğitim ve test verisi olarak deneysel ölçüm verileri uygulanmıştır. Bütün deneysel aralık üzerinde yüzey pürüzlülüğünü en iyi hassasiyet ile tahmin etmek için, en uygun eğitim veri seti, mümkün oldukça deneysel hatanın en az olduğu, on nöronlu iki gizli katmanlı ilk gizli katmanında 6, ikinci gizli katmanda 4 nöron ile elde edilmiştir. Ağ eğitildikten sonra, R2 değeri; 0.978 ve ortalama hata değeri; 0.018% olarak bulunmuştur. Matematiksel modellemenin sonuçları incelendiğinde, hesaplanan yüzey pürüzlülüğünün açık bir şekilde kabul edilebilir değerler içerisinde olduğu görülmüştür.
Anahtar Kelime: Belge Türü: Makale Makale Türü: Araştırma Makalesi Erişim Türü: Erişime Açık
- 1. Palanikumara K., Mata F., Paulo Davim J., “Analysis of surface roughness parameters in turning of FRP tubes by PCD tool”, Journal of Materials Processing Technology, 204: 469-474, (2008)
- 2. Cakir M.C., Ensarioglu C., Demirayak I., “Mathematical modeling of surface roughness for evaluating the effects of cutting parameters and coating material”, Journal of Materials Processing Technology, 209: 102-109, (2009)
- 3. Abouelatte O.B., Madl J., “Surface roughness prediction based on cutting parameters and tool vibrations in turning operations”, Journal of Materials Processing Technology, 118: 269-277, (2001)
- 4. Lin W.S., Lee B.Y., Wu C.L., “Modeling the surface roughness and cutting force for turning”, Journal of Materials Processing Technology, 108: 286-293, (2001)
- 5. Lalwani D.I., Mehta N.K., Jain P.K., “Experimental investigations of cutting parameters influence on cutting forces and surface roughness in finish hard turning of MDN250 steel”, Journal of Materials Processing Technology, 206: 167-179, (2008)
- 6. Karataş C., Sozen A., Dulek, E., “Modelling of residual stresses in the shot peened material C-1020 by artificial neural network”, Expert Systems with Applications, 36: 3514-3521, (2009)
- 7. Zuperl U., Cus F., Mursec B., and Ploj T., “A hybrid analytical-neural network approach to the determination of optimal cutting conditions”, Journal of Materials Processing Technology, 157(158): 82-90, (2004)
- 8. Özek C., Çelik Y.H., “Calculating molding parameters in plastic injection molds with ANN and developing software”, Materials and Manufacturing Processes, 27(2): 160-168, (2012)
- 9. Kurt A., “Modelling of the cutting tool stresses in machining of Inconel 718 using artificial neural networks”, Expert Systems with Applications, 36: 9645- 9657, (2009)
- 10. Adam A.C., Hiram A.F., Matthew T.B., Steven Y.L., “Workpiece dynamic analysis and prediction during chatter of turning process”, Mechanical Systems and Signal Processing, 22: 1481-1494, (2008)
- 11. Jamali A., Nariman-zadeh N., Darvizeh A., Masoumi A., Hamrang S., “Multi-objective evolutionary optimization of polynomial neural networks for modelling and prediction of explosive cutting process”, Engineering Applications of Artificial Intelligence, 22: 676-687, (2009)
- 12. Kim H.G., Sim J.H., Kweon H.J., “Performance evaluation of chip breaker utilizing neural network”, Journal of Materials Processing Technology, 209: 647- 656, (2009)
- 13. Karayel D., “Prediction and control of surface roughness in CNC lathe using artificial neural network”, Journal of Materials Processing Technology, 209: 3125-3137, (2009)
- 14. Ezugwua E.O., Fadarea D.A., Bonney J., Da Silva R.B., Sales W.F., “Modelling the correlation between cutting and process parameters in high-speed machining of Inconel 718 alloy using an artificial neural network”, International Journal of Machine Tools & Manufacture, 45: 1375-1385, (2005)
- 15. Ranganathan S., Senthilvelan T., Sriram G., “Evaluation of machining parameters of hot turning of stainless steel (Type 316) by applying ANN and RSM”, Materials and Manufacturing Processes, 25(10): 1131-1141, (2010)
- 16. Venkata Rao K., Murthy B.S.N., Mohan Rao N., “Prediction of cutting tool wear, surface roughness and vibration of work piece in boring of AISI 316 steel with artificial neural network”, Measurement, 51: 63–70, (2014)
- 17. Kumar R., Chauhan S., “Study on surface roughness measurement for turning of Al 7075/10/SiCp and Al 7075 hybrid composites by using response surface methodology (RSM) and artificial neural networking (ANN)”, Measurement, 65: 166–180, (2015)
- 18. Natarajan C., Muthu S., Karuppuswamy P., “Prediction and analysis of surface roughness characteristics of a non-ferrous material using ANN in CNC turning”, Int J Adv Manuf Technol, 57:1043–1051, (2011)
- 19. Nalbant M., Gökkaya H., Toktaş İ., Sur G., “The experimental investigation of the effects of uncoated, PVD-and CVD-coated cemented carbide inserts and cutting parameters on surface roughness in CNC turning and its prediction using artificial neural Networks”, Robotics and Computer-Integrated Manufacturing, 25: 211-223, (2009)
- 20. Soleimanimehr H., Nategh M.J., Amini S., “Prediction of machining force and surface roughness in ultrasonic vibration-assisted turning using neural networks”, Advanced Materials Research, 83(86): 326-334, (2010)
- 21. Paulo Davim J., Gaitondeb V.N., Karnik S.R., “Investigations into the effect of cutting conditions on surface roughness in turning of free machining steel by ANN models”, Journal of Materials Processing Technology, 205: 16-23, (2008)
- 22. Gürbüz H., Şeker U., “The effects of chip breaker forms on the surface quality in turning operations”, I. National Manufacturing Symposium, October 2-3, Yıldız Technical University, Istanbul, Turkey, 198-206, (2009)
- 23. Gürbüz, H., “The Effect on The Cutting Tool Stresses of The Different Chip Breaker Form in Turning”, MSc. Thesis (in Turkish), Gazi University, Institute of Science and Technology, Ankara, Turkey, (2006)
- 24. Mitsubishi Carbide, “Turning tools, Rotating tools, Tooling solutions”, General Catalogue, A002-A005, (2005)
- 25. Mohandes M., Rehman S., Halawani T.O., “Estimation of global solar radiation using artificial neural networks”, Renewable Energy, 14(1-4): 179-84, (1998)
- 26. Jang J.S.R., Sun C.T., Mizutani E., “Neuro-fuzzy and soft computing: a computational approach to learning and machine intelligence”, Prentice-Hall International, (1997)
- 27. Sözen A., Özalp M., Arcaklioğlu E., “Investigation of thermodynamic properties of refrigerant/absorbent couples using artificial neural networks”, Chemical Engineering and Processing, 43: 1253–1264, (2004)
- 28. Uluer O., Kırmacı V., Atas Ş., “Using the artificial neural network model for modeling the performance of the counter flow vortex tube”, Expert Systems with Applications, 36: 12256–12263, (2009)
- 29. Boothroyd G., “Fundamentals of metal machining and machine tools”, Second edition, McGraw-Hill, New York, 166-172, (1989)
- 30. Shaw M.C., “Metal cutting principles”, Oxford University Press, London, ISBN 0-19-859002-4, 594, (1984)
- 31. Çiftçi İ., “The influence of cutting tool coating and cutting Speed on cutting forces and surface roughness in machining of austenitic stainless steels”, Gazi University Faculty of Engineering and Architecture Journal, 20(2): 205-209, (2005)
- 32. Munoz–Escalona P., Cassier Z., “Influence of the critical cutting speed on the surface finish of turned steel”, Wear, 218: 103-109, (1998)
- 33. Thamizhmanii S., Kamarudin K., Rahim E.A., Saparudin A., Hassan S., “Tool wear and surface roughness in turning AISI 8620 using coated ceramic tool”. Proceedings of the World Congress on Engineering (July 2-4), Vol II WCE, London, U.K. (2007)
- 34. Javidi A., Rieger U., Eichlseder W., “The effect of machining on the surface integrity and fatigue life”, International Journal of Fatigue, 30: 2050-2055, (2008)
- 35. Sozen A., “Future projection of the energy dependency of Turkey using artificial neural network”. Energy Policy, 37(11): 4827-4833 (2009)
APA | GÜRBÜZ. H, SÖZEN A, ŞEKER U (2016). Modelling of Effects of Various Chip Breaker Forms on Surface Roughness in Turning Operations by Utilizing Artificial Neural Networks. , 71 - 83. 10.2339/2016.19.1 71-83 |
Chicago | GÜRBÜZ. Hüseyin,SÖZEN Adnan,ŞEKER Ulvi Modelling of Effects of Various Chip Breaker Forms on Surface Roughness in Turning Operations by Utilizing Artificial Neural Networks. (2016): 71 - 83. 10.2339/2016.19.1 71-83 |
MLA | GÜRBÜZ. Hüseyin,SÖZEN Adnan,ŞEKER Ulvi Modelling of Effects of Various Chip Breaker Forms on Surface Roughness in Turning Operations by Utilizing Artificial Neural Networks. , 2016, ss.71 - 83. 10.2339/2016.19.1 71-83 |
AMA | GÜRBÜZ. H,SÖZEN A,ŞEKER U Modelling of Effects of Various Chip Breaker Forms on Surface Roughness in Turning Operations by Utilizing Artificial Neural Networks. . 2016; 71 - 83. 10.2339/2016.19.1 71-83 |
Vancouver | GÜRBÜZ. H,SÖZEN A,ŞEKER U Modelling of Effects of Various Chip Breaker Forms on Surface Roughness in Turning Operations by Utilizing Artificial Neural Networks. . 2016; 71 - 83. 10.2339/2016.19.1 71-83 |
IEEE | GÜRBÜZ. H,SÖZEN A,ŞEKER U "Modelling of Effects of Various Chip Breaker Forms on Surface Roughness in Turning Operations by Utilizing Artificial Neural Networks." , ss.71 - 83, 2016. 10.2339/2016.19.1 71-83 |
ISNAD | GÜRBÜZ., Hüseyin vd. "Modelling of Effects of Various Chip Breaker Forms on Surface Roughness in Turning Operations by Utilizing Artificial Neural Networks". (2016), 71-83. https://doi.org/10.2339/2016.19.1 71-83 |
APA | GÜRBÜZ. H, SÖZEN A, ŞEKER U (2016). Modelling of Effects of Various Chip Breaker Forms on Surface Roughness in Turning Operations by Utilizing Artificial Neural Networks. Politeknik Dergisi, 19(1), 71 - 83. 10.2339/2016.19.1 71-83 |
Chicago | GÜRBÜZ. Hüseyin,SÖZEN Adnan,ŞEKER Ulvi Modelling of Effects of Various Chip Breaker Forms on Surface Roughness in Turning Operations by Utilizing Artificial Neural Networks. Politeknik Dergisi 19, no.1 (2016): 71 - 83. 10.2339/2016.19.1 71-83 |
MLA | GÜRBÜZ. Hüseyin,SÖZEN Adnan,ŞEKER Ulvi Modelling of Effects of Various Chip Breaker Forms on Surface Roughness in Turning Operations by Utilizing Artificial Neural Networks. Politeknik Dergisi, vol.19, no.1, 2016, ss.71 - 83. 10.2339/2016.19.1 71-83 |
AMA | GÜRBÜZ. H,SÖZEN A,ŞEKER U Modelling of Effects of Various Chip Breaker Forms on Surface Roughness in Turning Operations by Utilizing Artificial Neural Networks. Politeknik Dergisi. 2016; 19(1): 71 - 83. 10.2339/2016.19.1 71-83 |
Vancouver | GÜRBÜZ. H,SÖZEN A,ŞEKER U Modelling of Effects of Various Chip Breaker Forms on Surface Roughness in Turning Operations by Utilizing Artificial Neural Networks. Politeknik Dergisi. 2016; 19(1): 71 - 83. 10.2339/2016.19.1 71-83 |
IEEE | GÜRBÜZ. H,SÖZEN A,ŞEKER U "Modelling of Effects of Various Chip Breaker Forms on Surface Roughness in Turning Operations by Utilizing Artificial Neural Networks." Politeknik Dergisi, 19, ss.71 - 83, 2016. 10.2339/2016.19.1 71-83 |
ISNAD | GÜRBÜZ., Hüseyin vd. "Modelling of Effects of Various Chip Breaker Forms on Surface Roughness in Turning Operations by Utilizing Artificial Neural Networks". Politeknik Dergisi 19/1 (2016), 71-83. https://doi.org/10.2339/2016.19.1 71-83 |