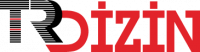
Marmara Denizi’ndeki Müsilaj Oluşumlarının Çok Zamanlı Optik ve Termal Uydu Görüntülerinden Makine Öğrenme Algoritması ile Tespiti ve Analizi
Yıl: 2021 Cilt: 87 Sayı: 166 Sayfa Aralığı: 1 - 9 Metin Dili: Türkçe İndeks Tarihi: 15-10-2021
Marmara Denizi’ndeki Müsilaj Oluşumlarının Çok Zamanlı Optik ve Termal Uydu Görüntülerinden Makine Öğrenme Algoritması ile Tespiti ve Analizi
Öz: ÖZKüresel ısınmanın karasal alanların yanında denizelalanlarda da doğal dengeyi bozan etkileribulunmaktadır. Bu bozucu etkilerden biri olan müsilaj yada deniz salyası, iç deniz ve körfezlerde ortaya çıkandoğal felaketlerden biridir. Müsilaj, Marmara Denizi’nde2021 yılının Mayıs ayından itibaren hızlı bir şekildeyayılarak akıntı ve rüzgâr etkisiyle Gemlik ve İzmitkörfezleriyle İstanbul’un kıyı bölgelerinde etkili olmuştur.Bu çalışmada, Sentinel-2 ve Landsat-8 uydu görüntüleriyardımıyla müsilajın Marmara Denizi’nin İstanbul,Kocaeli, Yalova ve Bursa illerine ait sahilleri kapsayanyaklaşık 4000 km²’lik alanda başlangıçtan itibarenyayılımı, kapladığı alan ve deniz yüzeyinde müsilajyoğunluğuna bağlı olarak oluşan ısıl değişimler detaylışekilde analiz edilmiştir. Analizler için 14, 19 ve 24Mayıs 2021 tarihlerinde çekilen Sentinel-2A görüntüleriile 17 Mayıs tarihli Landsat-8 görüntüsünün termalbandı kullanılmıştır. Görüntü işleme teknikleri vepopüler makine öğrenme algoritması olan rastgeleorman ile deniz yüzeyi müsilaj oluşumları %99doğrulukla tespit edilmiştir. Müsilaj kaplı alanbüyüklüğü, 14 Mayıs 2021 tarihinde 49,71 km² olarakhesaplanırken 24 Mayıs 2021 tarihinde 70,37 km²olarak hesaplanmıştır. Bu sonuç, 10 günlük süreçte%41’lik bir artışı işaret ederek durumun ciddiyetiniortaya koymuştur. Diğer taraftan, deniz suyu sıcaklığıkıyıdan uzak müsilaj olmayan alanlarda ortalama14,5°C iken müsilajın yoğun olduğu bölgelerde 17°C’ninüzerinde tespit edilmiştir. Tüm bu sonuçlar MarmaraDenizi’nde 2007 yılında ilk kez görülen müsilajolgusunun yılın özellikle bahar dönemlerinde anisıcaklık artışları ve diğer etkenlerle birlikte kendinigöstermeye devam edeceğini işaret etmektedir.
Anahtar Kelime: Konular:
(Detection and Analysis of Marine Mucilage Bloom in the Sea of Marmara by a Machine Learning Algorithm from Multi-Temporal Optical and Thermal Satellite Images
Öz: Global warming has effects that disrupt the natural balance in marine areas as well as terrestrial areas. One of these disruptive effects, mucilage, or sea saliva, is one of the natural disasters in the inland seas and bays. Mucilage has been spreading rapidly in the Sea of Marmara since May 2021 and has been effective in the Gemlik and Izmit Bays, and the coastal areas of Istanbul with the effects of currents and wind. In this study, the distribution of mucilage in an area of approximately 4000 km² covering the coasts of the Sea of Marmara Istanbul, Kocaeli, Yalova and Bursa. With the help of Sentinel-2 and Landsat-8 satellite images, the area covered and the thermal changes caused by the density of mucilage on the sea surface were analyzed in detail from the beginning of the phenomenon. In the analysis, May 14, 19 and 24, 2021 Sentinel-2A images and May 17, 2021 Landsat-8 thermal image were employed. With digital image processing techniques and random forest, a popular machine learning algorithm, sea surface mucilage formations were estimated with 99% accuracy. Whilst the size of the mucilage covered area was calculated as 49.71 km² on May 14, 2021, it was calculated as 70.37 km² on May 24. This result marked a 41% increase in the 10-day period, indicating the severity of the situation. On the other hand, the sea water temperature was found to be 14.5°C on average in areas without mucilage far from shore, while it was over 17°C in areas where mucilage was dense. All these results indicate that the mucilage phenomenon, first observed in the Marmara Sea in 2007, will continue to manifest itself together with sudden temperature increases and other factors, especially in the spring periods of the year.
Anahtar Kelime: Konular:
Belge Türü: Makale Makale Türü: Araştırma Makalesi Erişim Türü: Bibliyografik
- Avdan, U. ve Jovanovska, G. (2016). Algorithm for automated mapping of land surface temperature using LANDSAT 8 satellite data. Journal of Sensors, 2016, 1–8. doi: 10.1155/2016/1480307
- Aktan, Y., Isinibilir, M., Topaloğlu, B., Dede, A. ve Çardak, M. (2008). Mucilage event associated with diatoms and dinoflagellates from the Marmara Sea, Turkey. Harmful Algae News, May 2008, 36, 1-7.
- Azam F., Fonda-Umani S. ve Funari E. (1999). Significance of bacteria in the mucilage phenomenon in the northern Adriatic Sea. Ann Ist Super Sanita, 35(3), 411-9. PMID: 10721207.
- Breiman, L. (2001). Random Forests. In Machine Learning (pp. 5–32). Chapman and Hall/CRC.
- Colkesen, I. ve Kavzoglu, T. (2017). Ensemblebased canonical correlation forest (CCF) for land use and land cover classification using sentinel-2 and Landsat OLI imagery, Remote Sensing Letters, 8(11), 1082-1091. doi: 10.1080/2150704X.2017.1354262
- Copernicus Open Acess (Sentinel uydu görüntülerine erişim), (2021, 25 Mayıs). Erişim adresi: https://scihub.copernicus.eu.
- Danovaro, R., Fonda-Umani, S. ve Pusceddu, A. (2009). Climate change and the potential spreading of marine mucilage and microbial pathogens in the Mediterranean Sea. PloS one. 4. e7006. doi: 10.1371/journal.pone.0007006
- EarthExplorer (Landsat uydu görüntülerine erişim), (2021, 20 Mayıs) http://earthexplorer.usgs.gov/.
- Fu, B., Wang, Y., Campbell, A., Li, Y., Zhang, B., Yin, S., Xing, Z. ve Jin, X. (2017). Comparison of object-based and pixel-based Random Forest algorithm for wetland vegetation mapping using high spatial resolution GF-1 and SAR data. Ecological Indicators, 73, 105–117. doi: 10.1016/j.ecolind.2016.09.029
- Funari E. ve Ade P. (1999). Human health implications associated with mucilage in the northern Adriatic Sea. Ann Ist Super Sanita, 35(3), 421-5. PMID: 10721208.
- Giani, M., Savelli, F., Berto, D., Zangrando, V., Cosović, B. ve Vojvodić, V. (2005). Temporal dynamics of dissolved and particulate organic carbon in the northern Adriatic Sea in relation to the mucilage events. Science of The Total Environment, 353(1-3), 126-38. doi: 10.1016/j.scitotenv.2005.09.062
- Gigliotti, A. (2013). Extracting temporal and spatial distributions information about marine mucilage phenomenon based on Modis satellite images; a case study of the Tyrrhenian and the Adriatic Sea, 2010-2012. Master of Science in Geospatial Technologies, https://run.unl.pt/bitstream/10362/9205/1/TGE O0095.pdf
- Immitzer, M., Atzberger, C. ve Koukal, T. (2012). Tree species classification with random forest using very high spatial resolution 8-band WorldView-2 Satellite data. Remote Sensing, 4(9), 2661–2693. doi: 10.3390/rs4092661
- Jensen, J. R. (2015). Introductory digital ımage processing: A remote sensing perspective (4th. ed.). Prentice Hall Press, USA.
- Kavzoglu, T. (2017). Object-oriented random forest for high resolution land cover mapping using Quickbird-2 Imagery. Handbook of Neural Computation, 2017, 607-619.
- Kraus, R. ve Supić, N. (2015). Sea Dynamics impacts on the macroaggregates: A case study of the 1997 mucilage event in the northern Adriatic. Progress in Ocenaography, 138, 249- 267. doi: 10.1016/j.pocean.2015.06.005
- Mecozzi, M., Acquistucci, R., Noto, V., Pietrantonio, E., Amici, M. ve Cardarilli, Demetria. (2001). Characterization of mucilage aggregates in Adriatic and Tyrrhenian Sea: Structure similarities between mucilage samples and the insoluble fractions of marine humic substance. Chemosphere, 44, 709-20. doi: 10.1016/S0045-6535(00)00375-1
- Özalp, H.B. (2021). First massive mucilage event observed in deep waters of Çanakkale Strait (Dardanelles), Turkey. Journal of the Black Sea/Mediterranean Environment, 27(1), 49-66.
- Sekertekin, A. ve Bonafoni, S. (2020). Land surface temperature retrieval from Landsat 5, 7, and 8 over rural areas: Assessment of different retrieval algorithms and emissivity models and toolbox implementation. Remote Sensing, 12(2), 294. doi: 10.3390/rs12020294
- Sheykhmousa, M., Mahdianpari, M., Ghanbari, H., Mohammadimanesh, F., Ghamisi, P. ve Homayouni, S. (2020). Support vector machine versus random forest for remote sensing ımage classification: A Meta-analysis and systematic review. IEEE Journal of Selected Topics in Applied Earth Observations and Remote Sensing, 13, 6308–6325. doi: 10.1109/JSTARS.2020.3026724 SNAP (Sentinel Application Platform), (2021, 24 Haziran), https://step.esa.int/main/download/snapdownload.
- Suresh, S., Ajay Suresh, V. ve Mani, K. (2016). Estimatıon of land surface temperature of high range mountain landscape of Devıkulam Taluk using LANDSAT 8 data. International Journal of Research in Engineering and Technology, 05(01), 92–96.
- Tas, S., Kus, D. ve Yılmaz, I. N. (2020). Temporal variations in phytoplankton composition in the north-eastern Sea of Marmara: potentially toxic species and mucilage event. Mediterranean Marine Science, 21(3), 668-683. doi: 10.12681/mms.22562
- Tassan, S. (1993). An algorithm for the detection of the white-tide (“mucilage”) phenomenon in the adriatic sea using AVHRR data. Remote Sensing of Environment, 45(1), 29-42. doi: 10.1016/0034-4257(93)90079-D
- Tüfekçi, V., Balkıs, N., Beken Polat, Ç., Ediger, D. ve Mantıkçı, M. (2010). Phytoplankton composition and environmental conditions of a mucilage event in the Sea of Marmara. Turkish Journal of Biology, 34, 199-210. doi: 10.3906/biy-0812-1
- Yentur, R.E., Buyuates, Y., Ozen, O. ve Altin, A. (2013). The environmental and socioeconomical effects of a biologic problem: Mucilage. Marine Science and Technology Bulletin, 2(2), 13-15.
- Zambianchi, E., Calvitti, C., Cecamore, P., D'Amico, F., Ferulano, E. ve Lanciano, P. (1992). The mucilage phenomenon in the Northern Adriatic Sea, summer 1989: a study carried out with remote sensing techniques. Marine Coastal Eutrophication, 126, 581-598. doi: 10.1016/B978-0-444-89990-3.50053-5
APA | Kavzoglu T, COLKESEN I, Sefercik U, OZTURK M (2021). Marmara Denizi’ndeki Müsilaj Oluşumlarının Çok Zamanlı Optik ve Termal Uydu Görüntülerinden Makine Öğrenme Algoritması ile Tespiti ve Analizi. , 1 - 9. |
Chicago | Kavzoglu Taskin,COLKESEN Ismail,Sefercik Umut Gunes,OZTURK Muhammed Yusuf Marmara Denizi’ndeki Müsilaj Oluşumlarının Çok Zamanlı Optik ve Termal Uydu Görüntülerinden Makine Öğrenme Algoritması ile Tespiti ve Analizi. (2021): 1 - 9. |
MLA | Kavzoglu Taskin,COLKESEN Ismail,Sefercik Umut Gunes,OZTURK Muhammed Yusuf Marmara Denizi’ndeki Müsilaj Oluşumlarının Çok Zamanlı Optik ve Termal Uydu Görüntülerinden Makine Öğrenme Algoritması ile Tespiti ve Analizi. , 2021, ss.1 - 9. |
AMA | Kavzoglu T,COLKESEN I,Sefercik U,OZTURK M Marmara Denizi’ndeki Müsilaj Oluşumlarının Çok Zamanlı Optik ve Termal Uydu Görüntülerinden Makine Öğrenme Algoritması ile Tespiti ve Analizi. . 2021; 1 - 9. |
Vancouver | Kavzoglu T,COLKESEN I,Sefercik U,OZTURK M Marmara Denizi’ndeki Müsilaj Oluşumlarının Çok Zamanlı Optik ve Termal Uydu Görüntülerinden Makine Öğrenme Algoritması ile Tespiti ve Analizi. . 2021; 1 - 9. |
IEEE | Kavzoglu T,COLKESEN I,Sefercik U,OZTURK M "Marmara Denizi’ndeki Müsilaj Oluşumlarının Çok Zamanlı Optik ve Termal Uydu Görüntülerinden Makine Öğrenme Algoritması ile Tespiti ve Analizi." , ss.1 - 9, 2021. |
ISNAD | Kavzoglu, Taskin vd. "Marmara Denizi’ndeki Müsilaj Oluşumlarının Çok Zamanlı Optik ve Termal Uydu Görüntülerinden Makine Öğrenme Algoritması ile Tespiti ve Analizi". (2021), 1-9. |
APA | Kavzoglu T, COLKESEN I, Sefercik U, OZTURK M (2021). Marmara Denizi’ndeki Müsilaj Oluşumlarının Çok Zamanlı Optik ve Termal Uydu Görüntülerinden Makine Öğrenme Algoritması ile Tespiti ve Analizi. Harita Dergisi, 87(166), 1 - 9. |
Chicago | Kavzoglu Taskin,COLKESEN Ismail,Sefercik Umut Gunes,OZTURK Muhammed Yusuf Marmara Denizi’ndeki Müsilaj Oluşumlarının Çok Zamanlı Optik ve Termal Uydu Görüntülerinden Makine Öğrenme Algoritması ile Tespiti ve Analizi. Harita Dergisi 87, no.166 (2021): 1 - 9. |
MLA | Kavzoglu Taskin,COLKESEN Ismail,Sefercik Umut Gunes,OZTURK Muhammed Yusuf Marmara Denizi’ndeki Müsilaj Oluşumlarının Çok Zamanlı Optik ve Termal Uydu Görüntülerinden Makine Öğrenme Algoritması ile Tespiti ve Analizi. Harita Dergisi, vol.87, no.166, 2021, ss.1 - 9. |
AMA | Kavzoglu T,COLKESEN I,Sefercik U,OZTURK M Marmara Denizi’ndeki Müsilaj Oluşumlarının Çok Zamanlı Optik ve Termal Uydu Görüntülerinden Makine Öğrenme Algoritması ile Tespiti ve Analizi. Harita Dergisi. 2021; 87(166): 1 - 9. |
Vancouver | Kavzoglu T,COLKESEN I,Sefercik U,OZTURK M Marmara Denizi’ndeki Müsilaj Oluşumlarının Çok Zamanlı Optik ve Termal Uydu Görüntülerinden Makine Öğrenme Algoritması ile Tespiti ve Analizi. Harita Dergisi. 2021; 87(166): 1 - 9. |
IEEE | Kavzoglu T,COLKESEN I,Sefercik U,OZTURK M "Marmara Denizi’ndeki Müsilaj Oluşumlarının Çok Zamanlı Optik ve Termal Uydu Görüntülerinden Makine Öğrenme Algoritması ile Tespiti ve Analizi." Harita Dergisi, 87, ss.1 - 9, 2021. |
ISNAD | Kavzoglu, Taskin vd. "Marmara Denizi’ndeki Müsilaj Oluşumlarının Çok Zamanlı Optik ve Termal Uydu Görüntülerinden Makine Öğrenme Algoritması ile Tespiti ve Analizi". Harita Dergisi 87/166 (2021), 1-9. |