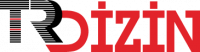
EEG Sinyallerinin Sınıflandırılmasında Evrimsel Öznitelik Seçim Metotlarının Kullanılması
Yıl: 2021 Cilt: 33 Sayı: 2 Sayfa Aralığı: 171 - 179 Metin Dili: Türkçe DOI: 10.7240/jeps.729478 İndeks Tarihi: 29-07-2022
EEG Sinyallerinin Sınıflandırılmasında Evrimsel Öznitelik Seçim Metotlarının Kullanılması
Öz: Elektroensefalografi beyindeki elektriksel akımın ölçülmesi ile elde edilen sinyallerdir. Bu sinyallerin sınıflandırılmasıözellikle beyin sinyalleri ile ilgili rahatsızlıkların teşhis, tanı ve tedavisine katkı sağladığı için önemlidir. Bu çalışmada bualanda epilepsi hastalığının tanısı için en çok kullanılan veri kümesi olan Bonn Üniversitesi veri kümesi kullanılmıştır. Beşfarklı denekten alınan sinyallerden oluşan bu veri kümesinden anlamlı sonuçlar elde edebilmek için öncelikle veri temizleme,öznitelik çıkarma ve öznitelik seçme yöntemleri kullanılmıştır. Daha sonra bu yöntemler sınıflandırma başarısına katkılarıaçısından kıyaslanmıştır. İlk olarak filtrelenen veriden Ayrık Dalgacık Dönüşümü metodu ile istatistiksel özellikler çıkarılmış,ardından Diferansiyel Evrim Algoritması kullanılarak en iyi sınıflandırma sonucunu veren öznitelik alt kümesi seçilmiştir.Seçilen özniteliklere sahip veri kümesinin sınıflandırma başarısı Destek Vektör Makineleri ile test edilmiştir. Kullanılanyöntem ile bazı sınıfların ayrılmasında literatürdeki benzer çalışmalardan daha iyi sonuçlar elde edilmiştir. Bazı ikili ve üçlükümelerin sınıflandırılmasında sırasıyla 0,98 ve 0,94 doğruluk oranları elde edilmiştir.
Anahtar Kelime: Using Evolutionary Feature Selection Methods in Classification of EEG Signals
Öz: Electroencephalography signals are obtained by measuring the electrical current in the brain. The classification of these signals are especially important, as they contribute to the diagnosis, and treatment of disorders related to brain signals. In this study, the data set of the University of Bonn, which is the most widely used data set for the diagnosis of epilepsy, was used in this field. In order to obtain meaningful results from this data set consisting of signals from five different subjects, firstly, data filtering, feature extraction and feature selection methods have been used first. Later, these methods were then compared in terms of their contribution to classification success. First, statistical properties were extracted from the filtered data by the Discrete Wavelet Transform method, and then the subset of the features that gave the best classification result was selected using the Differential Evolution Algorithm. The classification success of the data set with the selected features has been tested with the Support Vector Machines. With the method used, better results were obtained than similar studies in separating some classes. In the classification of some double and triple sets, accuracy rates of 0.98 and 0.94, respectively, were obtained.
Anahtar Kelime: Belge Türü: Makale Makale Türü: Araştırma Makalesi Erişim Türü: Erişime Açık
- [1] Andrzejak, R. (2005). BONN UNIVERCITY EEG time series download page. (10 2019) tarihinde, http://epileptologiebonn.de/cms/front_content.php?idcat=193&l ang=3 adresinden alındı.
- [2] Zhang, G., Yang, L., Li, B., Lu, Y., Liu, Q., Zhao, W., & ... & Che, W. (2020). MNL-Network: A Multi-Scale Non-local Network for Epilepsy Detection From EEG Signals. Frontiers in Neuroscience, 14.
- [3] Lu, D., & Triesch, J. (2019). Residual deep convolutional neural network for eeg signal classification in epilepsy. arXiv preprint arXiv:1903.08100,.
- [4] Kaya, D., Türk, M., & Kaya, T. (2018). En Yakın Komşu Algoritması Kullanılarak EEG Sinyallerine Boyut Azaltmanın Etkilerinin İncelenmesi. El-Cezeri Journal of Science and Engineering, s. 5.2: 591-595.
- [5] Physio Bank. (2018). 11 12, 2018 tarihinde (http://physi onet.org/cgi-bin/atm/ATM adresinden alındı.
- [6] Ramakrishnan, S., & Murugavel, A. M. (2019). Epileptic seizure detection using fuzzy-rules-based sub-band specific features and layered multi-class SVM. Pattern Analysis and Applications, 16.
- [7] Lasefr, Z. A. (2017). Epilepsy seizure detection using EEG signals. In 2017 IEEE 8th Annual Ubiquitous Computing, Electronics and Mobile Communication Conference (UEMCON) (pp. 162-167),IEEE. IEEE.
- [8] Or Rashid, M. M., & Ahmad, M. (2017). Epileptic Seizure Classification using Statistical Features of EEG Signal. In 2017 International Conference on Electrical, Computer and Communication Engineering (ECCE) (pp. 308-312). IEEE.
- [9] Ahmadi, A. S. (2017). A New Method for Epileptic Seizure Classification in EEG Using Adapted Wavelet Packets. İstanbul, Turkey: IEEE.
- [10] Baig, M. Z., Aslam, N., Shum, H. P., & Zhang, L. (2017). Differential evolution algorithm as a tool for optimal feature subset selection in motor imagery EEG. Expert Systems with Applications, 2017, 90: 184-195.
- [11] Intelligent Data Analysis Group Klaus-Robert Müller, B. B.-U. (2004, Aralık 12). BCI competition III dataset IVa. 12 10, 2020 tarihinde http://www.bbci.de/competition/iii/desc_IVa. html adresinden alındı
- [12] Ahammad, N. F. (2014). Detection of epileptic seizure event and onset using EEG. BioMed research international 2014, 2014(7).
- [13] Salem, O., Naseem, A., & Mehaoua, A. (2014). Epileptic Seizure Detection From EEG Signal using Discrete Wavelet Transform and Ant Colony classifier Communications (ICC). IEEE International Conference on. IEEE, 2014, 3529-3534.
- [14] Mahajan, K., Vargantwar, M. R., & Rajput, S. M. (2011). Classification of EEG using PCA, ICA and Neural Network. International Journal of Engineering and Advanced Technology, 1(80-83).
- [15] Siuly, S., Li, Y., & Zhang, Y. (2016, 11). EEG signal analysis and classification. IEEE Transactions on Neural Systems and Rehabilitaiton Engineering, s. 141-4.
- [16] Subasi, A. (2007). EEG signal classification using wavelet feature extraction and a mixture of expert model. Expert Systems with Applications, s. Cilt 4, 1084-1093.
- [17] Ojala, T., Pietikäinen, M., & Mäenpää, T. (2000). Gray scale and rotation invariant texture classification with local binary patterns. European Conference on Computer Vision. Springer, Berlin, Heidelberg, s. 404-420.
- [18] Burçin, K., & Vasif, N. V. (2011). Down syndrome recognition using local binary patterns and statistical evaluation of the system. Expert Systems with Applications. 38 2011, Cilt 7, s. 8690-8695.
- [19] Kaya, Y., Uyar, M., Tekin, R., & Yıldırım, S. (2014). 1D-local binary pattern based feature extraction for classification of epileptic EEG signals. Applied Mathematics and Computation,, s. 243: 209-219.
- [20] Tiwari, A. K., Pachori, R. B., Kanhangad, V., & Panigrahi, B. K. (2016). Automated diagnosis of epilepsy using key-point-based local binary pattern of EEG signals. IEEE journal of biomedical and health informatics,, s. 21(4), 888-896.
- [21] Price, K., Storn, R. M., & Lampinen, J. A. (2006). Differential Evolution A Practical Approach to Global Optimization. Springer Science & Business Media.
- [22] Fisher, R. A. (1936). The use of multiple measurements in taxonomic problems. Annals of eugenics, 7(2), 179-188.
- [23] Aronszajn, N. (1950). Theory of reproducing kernels. . Transactions of the American mathematical society, 68(3), 337-404.
- [24] Vapnik, V., & Lerner, A. Y. (1963). Recognition of patterns with help of generalized portraits. . Avtomat. i Telemekh, 24(6), 774-780.
- [25] Boser, B. E., Guyon, I. M., & Vapnik, V. N. (1992, July). A training algorithm for optimal margin classifiers. . In Proceedings of the fifth annual workshop on Computational learning theory (pp. 144-152).
- [26] Cortes, C., & Vapnik, V. (1995). Support-vector networks. Machine learning, 20(3), 273-297.
APA | ABBASOĞLU F, GÜLCÜ A, Baspinar U (2021). EEG Sinyallerinin Sınıflandırılmasında Evrimsel Öznitelik Seçim Metotlarının Kullanılması. , 171 - 179. 10.7240/jeps.729478 |
Chicago | ABBASOĞLU FERDA,GÜLCÜ AYLA,Baspinar Ulvi EEG Sinyallerinin Sınıflandırılmasında Evrimsel Öznitelik Seçim Metotlarının Kullanılması. (2021): 171 - 179. 10.7240/jeps.729478 |
MLA | ABBASOĞLU FERDA,GÜLCÜ AYLA,Baspinar Ulvi EEG Sinyallerinin Sınıflandırılmasında Evrimsel Öznitelik Seçim Metotlarının Kullanılması. , 2021, ss.171 - 179. 10.7240/jeps.729478 |
AMA | ABBASOĞLU F,GÜLCÜ A,Baspinar U EEG Sinyallerinin Sınıflandırılmasında Evrimsel Öznitelik Seçim Metotlarının Kullanılması. . 2021; 171 - 179. 10.7240/jeps.729478 |
Vancouver | ABBASOĞLU F,GÜLCÜ A,Baspinar U EEG Sinyallerinin Sınıflandırılmasında Evrimsel Öznitelik Seçim Metotlarının Kullanılması. . 2021; 171 - 179. 10.7240/jeps.729478 |
IEEE | ABBASOĞLU F,GÜLCÜ A,Baspinar U "EEG Sinyallerinin Sınıflandırılmasında Evrimsel Öznitelik Seçim Metotlarının Kullanılması." , ss.171 - 179, 2021. 10.7240/jeps.729478 |
ISNAD | ABBASOĞLU, FERDA vd. "EEG Sinyallerinin Sınıflandırılmasında Evrimsel Öznitelik Seçim Metotlarının Kullanılması". (2021), 171-179. https://doi.org/10.7240/jeps.729478 |
APA | ABBASOĞLU F, GÜLCÜ A, Baspinar U (2021). EEG Sinyallerinin Sınıflandırılmasında Evrimsel Öznitelik Seçim Metotlarının Kullanılması. International journal of advances in engineering and pure sciences (Online), 33(2), 171 - 179. 10.7240/jeps.729478 |
Chicago | ABBASOĞLU FERDA,GÜLCÜ AYLA,Baspinar Ulvi EEG Sinyallerinin Sınıflandırılmasında Evrimsel Öznitelik Seçim Metotlarının Kullanılması. International journal of advances in engineering and pure sciences (Online) 33, no.2 (2021): 171 - 179. 10.7240/jeps.729478 |
MLA | ABBASOĞLU FERDA,GÜLCÜ AYLA,Baspinar Ulvi EEG Sinyallerinin Sınıflandırılmasında Evrimsel Öznitelik Seçim Metotlarının Kullanılması. International journal of advances in engineering and pure sciences (Online), vol.33, no.2, 2021, ss.171 - 179. 10.7240/jeps.729478 |
AMA | ABBASOĞLU F,GÜLCÜ A,Baspinar U EEG Sinyallerinin Sınıflandırılmasında Evrimsel Öznitelik Seçim Metotlarının Kullanılması. International journal of advances in engineering and pure sciences (Online). 2021; 33(2): 171 - 179. 10.7240/jeps.729478 |
Vancouver | ABBASOĞLU F,GÜLCÜ A,Baspinar U EEG Sinyallerinin Sınıflandırılmasında Evrimsel Öznitelik Seçim Metotlarının Kullanılması. International journal of advances in engineering and pure sciences (Online). 2021; 33(2): 171 - 179. 10.7240/jeps.729478 |
IEEE | ABBASOĞLU F,GÜLCÜ A,Baspinar U "EEG Sinyallerinin Sınıflandırılmasında Evrimsel Öznitelik Seçim Metotlarının Kullanılması." International journal of advances in engineering and pure sciences (Online), 33, ss.171 - 179, 2021. 10.7240/jeps.729478 |
ISNAD | ABBASOĞLU, FERDA vd. "EEG Sinyallerinin Sınıflandırılmasında Evrimsel Öznitelik Seçim Metotlarının Kullanılması". International journal of advances in engineering and pure sciences (Online) 33/2 (2021), 171-179. https://doi.org/10.7240/jeps.729478 |