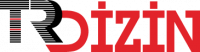
Predicting Health Spending in Turkey Using theGPR, SVR, and DT Models
Yıl: 2021 Cilt: 5 Sayı: 1 Sayfa Aralığı: 155 - 166 Metin Dili: İngilizce DOI: 10.26650/acin.882660 İndeks Tarihi: 25-11-2021
Predicting Health Spending in Turkey Using theGPR, SVR, and DT Models
Öz: Rising healthcare costs for countries and the long-term maintainability of this situation are at the center ofthe political agenda. The steady increase in health spending puts pressure on government budgets, healthcare,and personal patient financing. Policymakers would like to plan reforms to reduce these costs to adapt toproblems that may arise. This has led planners to decision support systems and forecasting models. In thispaper, three machine learnings algoritms, namely Support Vector Regression (SVR), Decision TreeRegression (DT), and Gaussian Process Regression (GPR) are employed to design a forecasting model forHealth Spendings (HS) of Turkey considering various determinants. Gross domestic product per capita,urban population rate, unemployment rate, population ages 65 and above, the life expectancy, the physicians’rate, and the total number of hospital beds are used as inputs. The data set consists of 30 years between 1990- 2019, which splits as training and test sets. Developed models were compared considering performancemetrics, and the most accurate model was identified. The coefficient of determinations (R2 ) for SVR, GPR,and DT models are 0.9929, 0.9989, and 0.9611 in the training phase, 0.9536, 0.8944, and 0.1166 in the testingstage, respectively. Therefore, the SVR model has accurate prediction results with the highest R2and the leastroot mean square error values in the testing phase. The study showed that the proposed SVR model reducedRMSE value by 32.02% and 39.66% compared to the GPR and DT models, respectively. Consequently, theHealth Spendings of Turkey can be predicted by employing SVR with high accuracy.
Anahtar Kelime: Türkiye Sağlık Harcamalarının GPR, SVR ve DT Modelleri ile Tahmini
Öz: Ülkeler için artan sağlık maliyetleri ve bu durumun uzun vadeli sürdürülebilirliği siyasi gündeminmerkezinde yer almaktadır. Sağlık harcamalarındaki sürekli artış, hükümet bütçeleri, sağlık hizmetleri vekişisel hasta finansmanı üzerinde baskı oluşturmaktadır. Politika yapıcılar, ortaya çıkabilecek sorunlarauyum sağlamak ve bu maliyetleri düşürmek için reformlar planlamak isterler. Bu durum, planlayıcıları karardestek sistemlerine ve tahmin modellerine yönlendirmiştir. Bu çalışmada, Türkiye’nin Sağlık Harcaması(HS) için çeşitli belirleyicileri dikkate alan bir tahmin modeli tasarlamak amacıyla Destek Vektör Regresyonu(SVR), Regresyon Ağacı (DT) ve Gauss Süreç Regresyonu (GPR) olmak üzere üç makine öğrenmealgoritması kullanılmıştır. Kişi başına gayri safi yurtiçi hasıla, kentsel nüfus oranı, işsizlik oranı, 65 yaş veüstü nüfus, ortalama yaşam süresi, hekim oranı ve toplam hastane yatak sayısı girdi değişkenleri olarakbelirlenmiştir. Veri seti eğitim ve test verisi olarak ayrılmış ve 1990-2019 yılları arası 30 yılı kapsamaktadır.Geliştirilen modeller performans ölçütleri dikkate alınarak karşılaştırılmış ve en iyi model belirlenmiştir.SVR, GPR ve DT modelleri için belirleme katsayısı (R2 ) eğitim aşamasında sırasıyla 0.9929, 0.9989 ve0.9611, test aşamasında sırasıyla 0.9536, 0.8944 ve 0.1166’dır. Ayrıca, SVR modeli, test aşamasında enyüksek R2ve en düşük kök ortalama kare hatası değerleri ile en iyi tahmin sonuçlarına sahiptir. Çalışma,önerilen SVR modelinin RMSE değerini diğer GPR ve DT modellerine kıyasla sırasıyla % 32.02 ve % 39.66azalttığını göstermiştir. Sonuç olarak, Türkiye’nin sağlık harcamaları SVR modeli kullanılarak yüksekdoğrulukta tahmin edilebilir.
Anahtar Kelime: Belge Türü: Makale Makale Türü: Araştırma Makalesi Erişim Türü: Erişime Açık
- Akca, N., Sonmez, S., Yilmaz, A., 2017. Determinants of health expenditure in OECD countries: A decision tree model. Pakistan J. Med. Sci. 33, 1490–1494. https://doi.org/10.12669/pjms.336.13300
- Aliyu, A.A., Amadu, L., 2017. Urbanization, cities, and health: The challenges to Nigeria - A review. Ann. Afr. Med. 16, 149–158. https://doi.org/10.4103/ aam.aam_1_17
- Anderson, G.F., Frogner, B.K., 2008. Health spending in OECD countries: Obtaining value per dollar. Health Aff. 27, 1718–1727. https://doi.org/10.1377/ hlthaff.27.6.1718
- Bauer, G., Davies, J.K., Pelikan, J., Consortium, on behalf of the E.T.W.G. and T.E., 2006. The EUHPID Health Development Model for the classification of public health indicators. Health Promot. Int. 21, 153–159. https://doi.org/10.1093/heapro/dak002
- Biadgilign, S., Ayenew, H.Y., Shumetie, A., Chitekwe, S., Tolla, A., Haile, D., Gebreyesus, S.H., Deribew, A., Gebre, B., 2019. Good governance, public health expenditures, urbanization and child undernutrition Nexus in Ethiopia: An ecological analysis. BMC Health Serv. Res. 19, 1–10. https://doi. org/10.1186/s12913-018-3822-2
- Boyce, T., Brown, C., 2019. Economic and social impacts and benefits of health systems. World Heal. Organ. 56.
- Ceylan, Z., 2020. Investigation the insights between health expenditures and air quality. Int. J. Glob. Warm. 20, 203–215. https://doi.org/10.1504/ IJGW.2020.106594
- Ceylan, Z., Atalan, A., 2020. Estimation of healthcare expenditure per capita of Turkey using artificial intelligence techniques with genetic algorithmbased feature selection. J. Forecast. 279–290. https://doi.org/10.1002/for.2747
- Cinaroglu, S., 2020. Modelling unbalanced catastrophic health expenditure data by using machine-learning methods. Intell. Syst. Accounting, Financ. Manag. 27, 168–181. https://doi.org/10.1002/isaf.1483
- Cinaroglu, Songül, 2017. Sağlık Harcamasının Tahmininde Makine Öğrenmesi Regresyon Yöntemlerinin Karşılaştırılması. Uludağ Univ. J. Fac. Eng. 22, 179–200. https://doi.org/10.17482/uumfd.338805
- Cinaroglu, Songul, 2017. International Journal of Economics and Financial Issues A Fresh Look at Out-of-Pocket Health Expenditures after More than a Decade Health Reform Experience in Turkey: A Data Mining Application. Int. J. Econ. Financ. Issues 7, 33–40.
- Crémieux, P.Y., Ouellette, P., Pilon, C., 1999. Health care spending as determinants of health outcomes. Health Econ. 8, 627–639. https://doi.org/10.1002/ (SICI)1099-1050(199911)8:7<627::AID-HEC474>3.0.CO;2-8
- Dalbokova, D., Krzyzanowski, M., 2002. Environmental health indicators: Development of a methodology for the WHO European Region. Stat. J. UN. Econ. Comm. Eur. 19, 93–103. https://doi.org/10.3233/SJU-2002-191-209
- Di Matteo, L., 2005. The macro determinants of health expenditure in the United States and Canada: Assessing the impact of income, age distribution and time. Health Policy (New. York). 71, 23–42. https://doi.org/10.1016/j.healthpol.2004.05.007
- Dritsakis, N., Klazoglou, P., 2019. Time Series Analysis using ARIMA Models: An Approach to Forecasting Health Expenditure in USA. Econ. Internazionale / Int. Econ. 72, 77–106.
- Durmaz, A., Pabuçcu, H., 2020. Kamu Sağlık Harcamaları - İşgücü Verimliliği İlişkisi: Sinirsel Bulanık Yaklaşım 8, 1375–1382.
- Efe, B., Efe, O.F., 2016. An Application of Value Analysis for Lean Healthcare Management in an Emergency Department. Int. J. Comput. Intell. Syst. 9, 689–697. https://doi.org/https://doi.org/10.1080/18756891.2016.1204117
- Guleryuz, D., Ozden, E., 2020. The Prediction of Brent Crude Oil Trend Using LSTM and Facebook Prophet. Eur. J. Sci. Technol. 1–9. https://doi. org/10.31590/ejosat.759302
- Häkkinen, U., Martikainen, P., Noro, A., Nihtilä, E., Peltola, M., 2008. Aging, health expenditure, proximity to death, and income in Finland. Heal. Econ. Policy Law 3, 165–195. https://doi.org/10.1017/S174413310800443X
- Jakovljevic, M., Timofeyev, Y., Ranabhat, C.L., Fernandes, P.O., Teixeira, J.P., Rancic, N., Reshetnikov, V., 2020b. Real GDP growth rates and healthcare spending - Comparison between the G7 and the EM7 countries. Global. Health 16, 1–13. https://doi.org/10.1186/s12992-020-00590-3
- Jia, H., Jiang, H., Yu, J., Zhang, J., Cao, P., Yu, X., 2021. Total Health Expenditure and Its Driving Factors in China: A Gray Theory Analysis. Healthcare 9. https://doi.org/10.3390/healthcare9020207
- Maksimović, G., Jović, S., Jovanović, R., Aničić, O., 2017. Management of health care expenditure by soft computing methodology. Phys. A Stat. Mech. its Appl. 465, 370–373. https://doi.org/10.1016/j.physa.2016.08.035
- Martín, J.J.M., del Amo González, M.P.L., García, M.D.C., 2011. Review of the literature on the determinants of healthcare expenditure. Appl. Econ. 43, 19–46. https://doi.org/10.1080/00036841003689754
- Ministry of Development, 2014. Onuncu Kalkınma Planı 2014-2018 Sağlık Hizmetlerinin Etkinliğinin Artırılması ve Mali Sürdürülebilirlik Özel İhtisas Komisyonu Raporu 2023 (In Turkish)
- Mladenović, I., Milovančević, M., Sokolov Mladenović, S., Marjanović, V., Petković, B., 2016. Analyzing and management of health care expenditure and gross domestic product (GDP) growth rate by adaptive neuro-fuzzy technique. Comput. Human Behav. 64, 524–530. https://doi.org/10.1016/j. chb.2016.07.052
- OECD, 2021. Health Resources (indicators) [WWW Document]. URL https://data.oecd.org/health.htm (accessed 2.1.21).
- Özcan, T., Tüysüz, F., 2018. Healthcare expenditure prediction in Turkey by using genetic algorithm based grey forecasting models, International Series in Operations Research and Management Science. https://doi.org/10.1007/978-3-319-65455-3_7
- Papanicolas, I., Woskie, L.R., Orlander, D., John Orav, E., Jha, A.K., 2019. The relationship between health spending and social spending in high-income countries: How does the US compare? Health Aff. 38, 1–9. https://doi.org/10.1377/hlthaff.2018.05187
- Pekel, E., Akkoyunlu, M.C., Akkoyunlu, M.T., Pusat, S., 2020. Decision tree regression model to predict low-rank coal moisture content during convective drying process. Int. J. Coal Prep. Util. 40, 505–512. https://doi.org/10.1080/19392699.2020.1737527
- Quan, Q., Hao, Z., Xifeng, H., Jingchun, L., 2020. Research on water temperature prediction based on improved support vector regression. Neural Comput. Appl. 4. https://doi.org/10.1007/s00521-020-04836-4
- Ram, M., Akbar Haghdoost, A., Hossein Mehrolhassani, M., Abolhallaje, M., Dehnavieh, R., Najafi, B., Akbar Fazaeli, A., 2019. Forecastting health expennditures in Iran using the ARIMA.
- Rana, R.H., Alam, K., Gow, J., 2020. Health expenditure and gross domestic product: causality analysis by income level. Int. J. Heal. Econ. Manag. 20, 55–77. https://doi.org/10.1007/s10754-019-09270-1
- Samah, I.H.A., Rashid, I.M.A., Husain, WAFW, Ibrahim, S., Hamzah, H., Amlus, M.H., 2020. The impact of healthcare expenditure and healthcare sector growth on CO2 emission using dynamic panel data system GMM estimation model during COVID 19 crisis. Int. J. Energy Econ. Policy 10, 235–241. https://doi.org/10.32479/ijeep.9769
- Shi, L., Smit, E., Luck, J., 2020. Panel Survey Estimation of the Impact of Urbanization in China: Does Level of Urbanization Affect Healthcare Expenditure, Utilization or Healthcare Seeking Behavior? Chinese Econ. 0, 1–12. https://doi.org/10.1080/10971475.2020.1848472
- TURKSTAT, 2020. Turkish Statistical Institute [WWW Document]. URL http://www.tuik.gov.tr/Start.do (accessed 6.13.20).
- Wang, Y., Li, J., Gu, J., Zhou, Z., Wang, Z., 2015. Artificial neural networks for infectious diarrhea prediction using meteorological factors in Shanghai (China). Appl. Soft Comput. J. 35, 280–290. https://doi.org/10.1016/j.asoc.2015.05.047
- Worldbank, 2021. World Bank Open Data [WWW Document]. URL https://data.worldbank.org/
- WHO, 2018. Delivering quality health services, World Health Organization, World Bank Group, OECD.
- Zhang, C., Wei, H., Zhao, X., Liu, T., Zhang, K., 2016. A Gaussian process regression based hybrid approach for short-term wind speed prediction. Energy Convers. Manag. 126, 1084–1092. https://doi.org/10.1016/j.enconman.2016.08.086
APA | GULERYUZ D (2021). Predicting Health Spending in Turkey Using theGPR, SVR, and DT Models. , 155 - 166. 10.26650/acin.882660 |
Chicago | GULERYUZ DIDEM Predicting Health Spending in Turkey Using theGPR, SVR, and DT Models. (2021): 155 - 166. 10.26650/acin.882660 |
MLA | GULERYUZ DIDEM Predicting Health Spending in Turkey Using theGPR, SVR, and DT Models. , 2021, ss.155 - 166. 10.26650/acin.882660 |
AMA | GULERYUZ D Predicting Health Spending in Turkey Using theGPR, SVR, and DT Models. . 2021; 155 - 166. 10.26650/acin.882660 |
Vancouver | GULERYUZ D Predicting Health Spending in Turkey Using theGPR, SVR, and DT Models. . 2021; 155 - 166. 10.26650/acin.882660 |
IEEE | GULERYUZ D "Predicting Health Spending in Turkey Using theGPR, SVR, and DT Models." , ss.155 - 166, 2021. 10.26650/acin.882660 |
ISNAD | GULERYUZ, DIDEM. "Predicting Health Spending in Turkey Using theGPR, SVR, and DT Models". (2021), 155-166. https://doi.org/10.26650/acin.882660 |
APA | GULERYUZ D (2021). Predicting Health Spending in Turkey Using theGPR, SVR, and DT Models. Acta Infologica, 5(1), 155 - 166. 10.26650/acin.882660 |
Chicago | GULERYUZ DIDEM Predicting Health Spending in Turkey Using theGPR, SVR, and DT Models. Acta Infologica 5, no.1 (2021): 155 - 166. 10.26650/acin.882660 |
MLA | GULERYUZ DIDEM Predicting Health Spending in Turkey Using theGPR, SVR, and DT Models. Acta Infologica, vol.5, no.1, 2021, ss.155 - 166. 10.26650/acin.882660 |
AMA | GULERYUZ D Predicting Health Spending in Turkey Using theGPR, SVR, and DT Models. Acta Infologica. 2021; 5(1): 155 - 166. 10.26650/acin.882660 |
Vancouver | GULERYUZ D Predicting Health Spending in Turkey Using theGPR, SVR, and DT Models. Acta Infologica. 2021; 5(1): 155 - 166. 10.26650/acin.882660 |
IEEE | GULERYUZ D "Predicting Health Spending in Turkey Using theGPR, SVR, and DT Models." Acta Infologica, 5, ss.155 - 166, 2021. 10.26650/acin.882660 |
ISNAD | GULERYUZ, DIDEM. "Predicting Health Spending in Turkey Using theGPR, SVR, and DT Models". Acta Infologica 5/1 (2021), 155-166. https://doi.org/10.26650/acin.882660 |