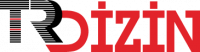
Sharpe Oranı ve Treynor Endeksi Performans Ölçülerine Dayalı Genetik Algoritma Yaklaşımı
Yıl: 2021 Cilt: 16 Sayı: 1 Sayfa Aralığı: 17 - 34 Metin Dili: Türkçe DOI: 10.29233/sdufeffd.780517 İndeks Tarihi: 29-07-2022
Sharpe Oranı ve Treynor Endeksi Performans Ölçülerine Dayalı Genetik Algoritma Yaklaşımı
Öz: Yatırımcı her zaman kendisine en yüksek faydayı sağlayacak olan portföyü oluşturmak istemektedir. Bu durum optimize edilmesi gereken portföy problemini ortaya çıkartır. Literatürde portföy optimizasyon problemi çözümünde genellikle bir klasik optimizasyon yöntemlerinden biri olan karesel programlama yöntemi kullanılmaktadır. Son yıllarda yapılan çalışmalar incelendiğinde yapay zeka algoritmalarının optimizasyon problemlerinde gösterdiği başarılardan yola çıkarak bu çalışmada genetik algoritma yaklaşımının portföy optimizasyon problemindeki başarısı ölçülmek istenmektedir. Portföy optimizasyon problemi için karesel programlama ile genetik algoritma yöntemleri portföy performans ölçüleri açısından karşılaştırılmıştır. Karşılaştırmada 2019 yılına ait BIST-30 endeksinde işlem gören hisse senetleri kullanılmıştır. Portföy performansını değerlendirmek için sharpe oranı ile treynor endeksi performans ölçüleri iki optimizasyon yönteminde de amaç fonksiyonu olarak kullanılmıştır. İki optimizasyon yönteminde de amaç; en yüksek performans ölçüsü oranına sahip portföyün belirlenmesidir. Yapılan analiz sonucunda genetik algoritma yönteminin her iki portföy performans ölçüsüne göre de optimum sonuca ulaştığı gözlemlenmiş ve treynor endeksinin sharpe oranına göre daha yüksek performans ölçüsü oranına sahip bir portföy oluşturduğu belirlenmiştir.
Anahtar Kelime: Sharpe Ratio and Treynor Index Performance Mesaurents Based A Genetic Algorithm Approach
Öz: The investor always wants to create a portfolio that will benefit him with the highest benefit. This situation presents the portfolio problem that needs to be optimized. In the literature, quadratic programming method, which is one of the classical optimization methods, is used to solve the portfolio optimization problem. In recent years, it has been observed that artificial intelligence methods give better results. In this study, genetic algorithm method was used for portfolio optimization problem. For the portfolio optimization problem, quadratic programming and genetic algorithm methods were compared in terms of portfolio performance measures. The stocks listed in the BIST-30 index of 2019 were used for comparison. Sharpe ratio and treynor index performance measures were used to evaluate portfolio performance. The goal in both optimization methods; it is the determination of the portfolio with the highest performance measure ratio. As a result of the analysis, it was observed that the genetic algorithm method reached the optimum result according to both portfolio performance measures. treynor index has been determined to form a portfolio with a higher performance measure ratio compared to the sharpe ratio.
Anahtar Kelime: Belge Türü: Makale Makale Türü: Araştırma Makalesi Erişim Türü: Erişime Açık
- [1] C. Shenoy and K.C. McCarthy, Applied Portfolio Management, John Wiley & Sons, Inc., New Jersey, 28-29, 1988.
- [2] F. K. Reilly and K. C. Brown, Investment Analysis and Portfolio Management, 2002.
- [3] H. Markowitz, “Portfolio selection,” J. Finance, 7, 77-91, 1952.
- [4] S. Canbaş, and H. Doğukanlı, Finansal Pazarlar, Adana, Karahan Kitabevi, 2007.
- [5] J.H. Holland, Adaptation in Natural and Artificial Systems: An Introductory Analysis with Application to Biology, Control, and Artificial Intelligence, Ann Arbor, MI: University of Michigan Press, 1975.
- [6] A. Diyar, T. Çetinyokuş, and M. Dağdeviren, “Portföy seçimi problemi için kds/ga yaklaşımı, ” Gazi Üniversitesi Mühendislik-Mimarlık Fakültesi Dergisi, 17(4), 2002.
- [7] K.J. Oh, T.Y. Kim, and S. Min, “Using genetic algorithm to support portfolio optimization for index fund management, ” Expert Syst. Appl., 28(2), 371-379, 2005.
- [8] D. Lin, X. Li, and M. Li., “A genetic algorithm for solving portfolio optimization problems with transaction costs and minimum transacton lots,” International Conference on Natural Computation, (3612), 808–811, 2005.
- [9] K. K. Lai, L. Yu, S. Wang, and C. Zhou, “A double-stage genetic optimization algorithm for portfolio selection,” Neural Information Processing, LNCS, 4234, 928–937, 2014.
- [10] C. M. Lin, and M. Gen, “An effective decision-based genetic algorithm approach to multiobjective portfolio optimization problem,” Appl Math. Sci., 1(5), 201-210, 2007.
- [11] S. Skolpadungket, K. Dahal, and N. Harnpornchai, “Portfolio optimization using multi-objective genetic algorithms,” in Proc. IEEE Congr. Evol. Comput. (CEC), sep., 516–523, 2007.
- [12] C.C. Lin and Y. T. Liu, “Genetic algorithms for portfolio selection problems with minimum transaction lot,” Eur. J. Ope. Res., 185(1), 393-404, 2008.
- [13] T. J. Chang, S.C. Yang and K. J. Chang, “Portfolio optimization problems in different risk measures using genetic algorithm, ” Expert Syst. Appl., 36(7), 10529-10537, 2009.
- [14] H. Soleimani, H.R. Golmakani, and M.H. Salimi, “Markowitz-based portfolio selection with minimum transaction lots, cardinality constraints and regarding sector capitalization using genetic algorithm, ” Expert Syst. Appl., 36(3/1), 5058-5063, 2009.
- [15] J. F. Chang, T.C. Wang and Y. T. Min, “Using Genetic Algorithms to construct a low-risk fund portfolio based on the Taiwan 50 Indein, ” Computational Aspects of Social Networks (CASoN), 2010 International Conference on, IEEE, 2010.
- [16] A.R. Pandari, A. Azar, and A.R. Shavazi, “Genetic algorithms for portfolio selection problems with non-linear objectives,” African Journal of Business Management, 6(20), 6209, 2012.
- [17] C.B. Kalayci, O. Ertenlice, H. Akyer and H. Aygoren, “A review on the current applications of genetic algorithms in mean-variance portfolio optimization,” Pamukkale University Journal of Engineering Sciences, 23(4), 470-476, 2017.
- [18] H. Markowitz, Portfolio Selection, Efficent Diversification of Investments, J. Wiley. 1959.
- [19] A. Ceylan and T. Korkmaz, Borsada Uygulamalı Portföy Yönetimi, Bursa, Ekin Kitabevi, 3. 1998.
- [20] A.G. Karaşin, Sermaye piyasası analizleri, Sermaye Piyasası Kurulu, 1987.
- [21] W.F. Sharpe, “Mutual fund performance,” The Journal of Business, 39(1), 119-138,1966.
- [22] E. Aksoy, Uluslararası Portföy Yönetimi, Ankara, Detay Yayıncılık, 2014.
- [23] J. Treynor, How to rate management of investment funds, 1965.
- [24] D.E. Goldberg and K. Deb, “A comparative analysis of selection schemes used in genetic algorithms,” Foundations of Genetic Algorithm, 1, 69-93, 1991.
- [25] T. Bäck, D. B. Fogel, and Z. Michalewicz, Evolutionary Computation 1: Basic Algorithms and Operators, Bristol, U.K., 2000.
- [26] M. Srinivas and L.M. Patnaik, “Genetic algorithms: A survey,” Computer, 127(6), 17-26, 1994.
- [27] D.E. Goldberg and J.H. Holland, “Genetic algorithms and machine learning,” Machine Learning, 3(2), 95-99, 1988.
- [28] G. G. Emel, Ç. Taşkın, “Genetik algoritmalar ve uygulama alanları,” Uludağ Üniversitesi İktisadi ve İdari Bilimler Fakültesi Dergisi, 21(1): 129-152, 2009.
- [29] K. De Jong, “An analysis of the behavior of a class of genetic adaptive systems,” Ph.D. dissertation, Dept. Comput. Sci., Univ. Michigan, Ann Arbor, MI, 1975.
- [30] A. Z. Çelenli Başaran, V.R. Uslu, “The portfolio optimization based on sharp performance ratio,” Turkish Journal of Forecasting, 3(1):7-14, 2019.
APA | BAŞARAN A (2021). Sharpe Oranı ve Treynor Endeksi Performans Ölçülerine Dayalı Genetik Algoritma Yaklaşımı. , 17 - 34. 10.29233/sdufeffd.780517 |
Chicago | BAŞARAN Azize Zehra ÇELENLİ Sharpe Oranı ve Treynor Endeksi Performans Ölçülerine Dayalı Genetik Algoritma Yaklaşımı. (2021): 17 - 34. 10.29233/sdufeffd.780517 |
MLA | BAŞARAN Azize Zehra ÇELENLİ Sharpe Oranı ve Treynor Endeksi Performans Ölçülerine Dayalı Genetik Algoritma Yaklaşımı. , 2021, ss.17 - 34. 10.29233/sdufeffd.780517 |
AMA | BAŞARAN A Sharpe Oranı ve Treynor Endeksi Performans Ölçülerine Dayalı Genetik Algoritma Yaklaşımı. . 2021; 17 - 34. 10.29233/sdufeffd.780517 |
Vancouver | BAŞARAN A Sharpe Oranı ve Treynor Endeksi Performans Ölçülerine Dayalı Genetik Algoritma Yaklaşımı. . 2021; 17 - 34. 10.29233/sdufeffd.780517 |
IEEE | BAŞARAN A "Sharpe Oranı ve Treynor Endeksi Performans Ölçülerine Dayalı Genetik Algoritma Yaklaşımı." , ss.17 - 34, 2021. 10.29233/sdufeffd.780517 |
ISNAD | BAŞARAN, Azize Zehra ÇELENLİ. "Sharpe Oranı ve Treynor Endeksi Performans Ölçülerine Dayalı Genetik Algoritma Yaklaşımı". (2021), 17-34. https://doi.org/10.29233/sdufeffd.780517 |
APA | BAŞARAN A (2021). Sharpe Oranı ve Treynor Endeksi Performans Ölçülerine Dayalı Genetik Algoritma Yaklaşımı. Süleyman Demirel Üniversitesi Fen Edebiyat Fakültesi Fen Dergisi, 16(1), 17 - 34. 10.29233/sdufeffd.780517 |
Chicago | BAŞARAN Azize Zehra ÇELENLİ Sharpe Oranı ve Treynor Endeksi Performans Ölçülerine Dayalı Genetik Algoritma Yaklaşımı. Süleyman Demirel Üniversitesi Fen Edebiyat Fakültesi Fen Dergisi 16, no.1 (2021): 17 - 34. 10.29233/sdufeffd.780517 |
MLA | BAŞARAN Azize Zehra ÇELENLİ Sharpe Oranı ve Treynor Endeksi Performans Ölçülerine Dayalı Genetik Algoritma Yaklaşımı. Süleyman Demirel Üniversitesi Fen Edebiyat Fakültesi Fen Dergisi, vol.16, no.1, 2021, ss.17 - 34. 10.29233/sdufeffd.780517 |
AMA | BAŞARAN A Sharpe Oranı ve Treynor Endeksi Performans Ölçülerine Dayalı Genetik Algoritma Yaklaşımı. Süleyman Demirel Üniversitesi Fen Edebiyat Fakültesi Fen Dergisi. 2021; 16(1): 17 - 34. 10.29233/sdufeffd.780517 |
Vancouver | BAŞARAN A Sharpe Oranı ve Treynor Endeksi Performans Ölçülerine Dayalı Genetik Algoritma Yaklaşımı. Süleyman Demirel Üniversitesi Fen Edebiyat Fakültesi Fen Dergisi. 2021; 16(1): 17 - 34. 10.29233/sdufeffd.780517 |
IEEE | BAŞARAN A "Sharpe Oranı ve Treynor Endeksi Performans Ölçülerine Dayalı Genetik Algoritma Yaklaşımı." Süleyman Demirel Üniversitesi Fen Edebiyat Fakültesi Fen Dergisi, 16, ss.17 - 34, 2021. 10.29233/sdufeffd.780517 |
ISNAD | BAŞARAN, Azize Zehra ÇELENLİ. "Sharpe Oranı ve Treynor Endeksi Performans Ölçülerine Dayalı Genetik Algoritma Yaklaşımı". Süleyman Demirel Üniversitesi Fen Edebiyat Fakültesi Fen Dergisi 16/1 (2021), 17-34. https://doi.org/10.29233/sdufeffd.780517 |