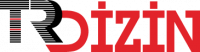
Forecasting COVID-19 Cases based on mobility
Yıl: 2020 Cilt: 8 Sayı: 2 Sayfa Aralığı: 144 - 150 Metin Dili: İngilizce İndeks Tarihi: 11-01-2022
Forecasting COVID-19 Cases based on mobility
Öz: Countries struggling to overcome the profound and devastating effects of COVID-19 have started taking steps to return to the "new normal." Any accurate forecasting can help countries and decision-makers make plans and decisions in returning to normal life. In this regard, it is needless to mention the criticality and importance of accurate forecasting. In this study, daily cases of COVID-19 are estimated based on mobility data, considering the proven human-tohuman transmission factor. The data of seven countries, namely Brazil, France, Germany, Italy, Spain, the United Kingdom (UK), and the United States of America (USA), are used to train and test the models. These countries represent around 57% of the total cases in the whole world. In this context, various machine learning algorithms are implemented to obtain accurate predictions. Unlike most studies, the predicted case numbers are evaluated against the actual values to reveal the methods' real performance and determine the most effective methods. The results indicated that it is unlikely to propose the same algorithm for forecasting COVID-19 cases for all countries. Also, mobility data can be enough the predict the COVID-19 cases in the USA.
Anahtar Kelime: Belge Türü: Makale Makale Türü: Araştırma Makalesi Erişim Türü: Erişime Açık
- [1]. World Health Organization, Coronavirus disease (COVID-19) Situation Report–139, (2020).
- [2]. El Zowalaty M.E., Järhult J.D., “From SARS to COVID-19: A previously unknown SARS-CoV-2 virus of pandemic potential infecting humans–Call for a One Health approach”, One Health, (2020), 100124.
- [3]. Findlater A., Bogoch I.I., “Human mobility and the global spread of infectious diseases: a focus on air travel”, Trends in parasitology, 34(9), (2018), 772-783.
- [4]. Alandijany T.A., Faizo A.A., Azhar E.I., “Coronavirus disease of 2019 (COVID-19) in the Gulf Cooperation Council (GCC) countries: Current status and management practices”, Journal of Infection and Public Health, 13(6), (2020), 839-842.
- [5]. Al-Tawfiq J.A., Memish Z.A., “COVID-19 in the Eastern Mediterranean Region and Saudi Arabia: prevention and therapeutic strategies”, International Journal of Antimicrobial Agents, 55(5), (2020), 105968.
- [6]. Zhu Y., et al., “The mediating effect of air quality on the association between human mobility and COVID19 infection in China”, Environmental Research, 189, (2020), 109911.
- [7]. Wang J., Du G., “COVID-19 may transmit through aerosol”. Irish Journal of Medical Science (1971 -),. 189(4), (2020), 1143-1144.
- [8]. Phan, L.T., et al., “Importation and human-to-human transmission of a novel coronavirus in Vietnam”, New England Journal of Medicine, 382(9), (2020), 872-874.
- [9]. Riou J., Althaus C.L., “Pattern of early human-tohuman transmission of Wuhan 2019 novel coronavirus (2019-nCoV), December 2019 to January 2020”, Eurosurveillance, 25(4), (2020), 2000058.
- [10]. Muhammad S., Long X., Salman M., “COVID-19 pandemic and environmental pollution: A blessing in disguise?”, Science of The Total Environment, 728, (2020), 138820.
- [11]. Kraemer M.U.G., et al., “The effect of human mobility and control measures on the COVID-19 epidemic in China”. Science, 368(6490), (2020), 493.
- [12]. Tagliazucchi E., et al., “Lessons from being challenged by COVID-19”, Chaos, Solitons & Fractals, 137, (2020), 109923.
- [13]. Yousaf M., et al., “Statistical analysis of forecasting COVID-19 for upcoming month in Pakistan”, Chaos, Solitons & Fractals, 138, (2020), 109926.
- [14]. Salgotra R., Gandomi M., Gandomi A.H., “Time Series Analysis and Forecast of the COVID-19 Pandemic in India using Genetic Programming”. Chaos, Solitons & Fractals, 138, (2020), 109945.
- [15]. Ayinde K., et al., “Modeling Nigerian Covid-19 cases: A comparative analysis of models and estimators”, Chaos, Solitons & Fractals, 138, (2020), 109911.
- [16]. Mandal M., et al., “A model based study on the dynamics of COVID-19: Prediction and control”, Chaos, Solitons & Fractals, 136, (2020), 109889.
- [17]. Parbat D., Chakraborty M., “A python based support vector regression model for prediction of COVID19 cases in India”. Chaos, Solitons & Fractals, 138, (2020), 109942.
- [18]. Chimmula V.K.R. Zhang L., “Time series forecasting of COVID-19 transmission in Canada using LSTM networks”, Chaos, Solitons & Fractals, 135, (2020), 109864.
- [19]. Fanelli D., Piazza F., “Analysis and forecast of COVID-19 spreading in China, Italy and France”, Chaos, Solitons & Fractals, 134, (2020), 109761.
- [20]. EU Open Data Portal. COVID-19 Coronavirus data 2020 [cited 2020 5/29/2020]; Available from: https://www.ecdc.europa.eu/sites/default/files/documen ts/COVID-19-geographic-disbtribution-worldwide.xlsx.
- [21]. Şahin M., “Impact of weather on COVID-19 pandemic in Turkey”, Science of The Total Environment, 728, (2020), 138810.
- [22]. Williams C.K.I., Rasmussen C.E., “Gaussian processes for machine learning”, MIT press Cambridge, MA., 2, (2006).
- [23]. Thissen U., et al., “Using support vector machines for time series prediction”, Chemometrics and Intelligent Laboratory Systems,. 69(1), (2003), 35-49.
- [24]. Moisen G.G., “Classification and Regression Trees, in Encyclopedia of Ecology, S.E. Jørgensen and B.D. Fath, Editors, Academic Press: Oxford, (2008), 582- 588.
- [25]. Wu X., et al., “Top 10 algorithms in data mining”, Knowledge and Information Systems, 14(1), (2008), 1- 37.
- [26]. Arias Velásquez R.M. Mejía Lara J.V., “Forecast and evaluation of COVID-19 spreading in USA with reduced-space Gaussian process regression”, Chaos, Solitons & Fractals, 136, (2020), 109924.
- [27]. Shahid F., Zameer A., Muneeb M., “Predictions for COVID-19 with deep learning models of LSTM, GRU and Bi-LSTM”, Chaos, Solitons & Fractals, 140, (2020), 110212.
- [28]. Tomar A., Gupta N., “Prediction for the spread of COVID-19 in India and effectiveness of preventive measures”, Science of The Total Environment, 728, (2020), 138762.
- [29]. Arora P., Kumar H., Panigrahi B.K., “Prediction and analysis of COVID-19 positive cases using deep learning models: A descriptive case study of India”, Chaos, Solitons & Fractals, 139, (2020), 110017.
- [30]. Alzahrani S.I., Aljamaan I.A., Al-Fakih E.A., “Forecasting the spread of the COVID-19 pandemic in Saudi Arabia using ARIMA prediction model under current public health interventions”, Journal of Infection and Public Health, 13(7), (2020), 914-919.
- [31]. Ribeiro M.H.D.M., et al., “Short-term forecasting COVID-19 cumulative confirmed cases: Perspectives for Brazil”, Chaos, Solitons & Fractals, 135, (2020), 109853.
- [32]. Sujath R., Chatterjee J.M., Hassanien A.E., “A machine learning forecasting model for COVID-19 pandemic in India”, Stochastic Environmental Research and Risk Assessment, (2020).
- [33]. Tuli S., et al., “Predicting the growth and trend of COVID-19 pandemic using machine learning and cloud computing”, Internet of Things, 11, (2020), 100222.
- [34]. Wang P., et al., “Prediction of epidemic trends in COVID-19 with logistic model and machine learning technics”, Chaos, Solitons & Fractals, 139, (2020), 110058.
- [35]. Liu J., et al., “Impact of meteorological factors on the COVID-19 transmission: A multi-city study in China”, Science of The Total Environment, 726, (2020), 138513.
- [36]. Goswami K., Bharali S., Hazarika J., “Projections for COVID-19 pandemic in India and effect of temperature and humidity”, Diabetes & Metabolic Syndrome: Clinical Research & Reviews, (2020).
- [37]. Li H., et al., “Air Pollution and temperature are associated with increased COVID-19 incidence: a time series study”, International Journal of Infectious Diseases, (2020).
- [38]. Wu Y., et al., “Effects of temperature and humidity on the daily new cases and new deaths of COVID-19 in 166 countries”, Science of The Total Environment, 729, (2020), 139051.
- [39]. Farzan A.N., U.S. coronavirus cases surpass 1.8 million as concern over potential spread rises with turmoil, in The Washington Post. 2020, The Washington Post.
APA | Sahin M (2020). Forecasting COVID-19 Cases based on mobility. , 144 - 150. |
Chicago | Sahin Mehmet Forecasting COVID-19 Cases based on mobility. (2020): 144 - 150. |
MLA | Sahin Mehmet Forecasting COVID-19 Cases based on mobility. , 2020, ss.144 - 150. |
AMA | Sahin M Forecasting COVID-19 Cases based on mobility. . 2020; 144 - 150. |
Vancouver | Sahin M Forecasting COVID-19 Cases based on mobility. . 2020; 144 - 150. |
IEEE | Sahin M "Forecasting COVID-19 Cases based on mobility." , ss.144 - 150, 2020. |
ISNAD | Sahin, Mehmet. "Forecasting COVID-19 Cases based on mobility". (2020), 144-150. |
APA | Sahin M (2020). Forecasting COVID-19 Cases based on mobility. Manas Journal of Engineering, 8(2), 144 - 150. |
Chicago | Sahin Mehmet Forecasting COVID-19 Cases based on mobility. Manas Journal of Engineering 8, no.2 (2020): 144 - 150. |
MLA | Sahin Mehmet Forecasting COVID-19 Cases based on mobility. Manas Journal of Engineering, vol.8, no.2, 2020, ss.144 - 150. |
AMA | Sahin M Forecasting COVID-19 Cases based on mobility. Manas Journal of Engineering. 2020; 8(2): 144 - 150. |
Vancouver | Sahin M Forecasting COVID-19 Cases based on mobility. Manas Journal of Engineering. 2020; 8(2): 144 - 150. |
IEEE | Sahin M "Forecasting COVID-19 Cases based on mobility." Manas Journal of Engineering, 8, ss.144 - 150, 2020. |
ISNAD | Sahin, Mehmet. "Forecasting COVID-19 Cases based on mobility". Manas Journal of Engineering 8/2 (2020), 144-150. |