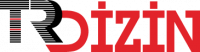
Lactation Milk Yield Prediction with Possibilistic Logistic Regression Analysis
Yıl: 2021 Cilt: 27 Sayı: 5 Sayfa Aralığı: 547 - 557 Metin Dili: İngilizce DOI: 10.9775/kvfd.2020.25171 İndeks Tarihi: 23-01-2022
Lactation Milk Yield Prediction with Possibilistic Logistic Regression Analysis
Öz: The logistic regression is a popular method to model the probability of a categorical outcome given as a dependent variable. However, the possibilistic logistic regression can be preferred instead of classical logistic regression when the dependent variable has uncertainity. The aim of this study is to use the possibilistic logistic regression on animal husbandry examining the theoretical foundations of the method based on fuzzy logic approach. A total of 90 cows were enrolled in the study and the average milk yield in 305 days was predicted by animal’s weight, breed of the animal, age in lactation, number of milkings per day and the milking seasons of cows belonging to different breeds. The Mean Degree of Memberships (MDM) and the Mean of Squared Error (MSE) indices were calculated to decide the goodness of fit of the model. The index values were found as MDM=0.896 and MSE=4.871, respectively. It was shown that the model is fit and is succesfull to predict the average milk yield. It can be concluded that the model can provide the businesses on lactation milk yield production an efficient and accurate prediction results.
Anahtar Kelime: Possibilistic Lojistik Regresyon Analizi İle Laktasyon Süt Verimi Tahmini
Öz: Lojistik regresyon analizi, bağımlı değişken olarak verilen özelliğin kategorilerini tahmin etmek için kullanılır. Ancak, bağımlı değişken belirsiz olduğunda klasik lojistik rgeresyon yerine posibilistik lojistik regresyon yöntemi tercih edilebilir. Bu çalışmanın amacı, süt sığırcılığında teorik altyapısı ile birlikte bulanık mantık yaklaşımı temelli posibilistik lojistik regresyon yöntemini kullanmaktır. Çalışmaya toplam 90 inekten elde edilen bilgiler dahil edildi ve hayvanın ağırlığı, hayvanın ırkı, hayvanın laktasyon yaşı, günlük sağım sayısı ve sağım mevsimi bilgileri kullanılarak ortalama süt verimi tahmin edildi. Modelin yeterliliğine karar verebilmek için ortalama üyelik derecesi (MDM) ve hata kareler ortalaması (MSE) indeks değerleri hesaplandı. İndeks değerleri sırasıyla MDM=0.896 ve MSE=4.871 olarak hesaplandı. Bu değerlere göre modelin uyumunun iyi olduğuna karar verildi. Bulgular, modelin laktasyon süt verimini tahminlemede etkin ve güvenilir sonuçlara sahip olduğunu göstermektedir.
Anahtar Kelime: Belge Türü: Makale Makale Türü: Araştırma Makalesi Erişim Türü: Erişime Açık
- 1. Zadeh LA: Fuzzy sets as a basis for a theory of possibility. Fuzzy Sets Syst, 100 (1): 9-34, 1999. DOI: 10.1016/S0165-0114(99)80004-9
- 2. Kim IK, Lee WJ, Yoon JH, Choi SH: Fuzzy regression model using trapezoidal fuzzy numbers for re-auction data. Int J Fuzzy Log Intell Syst, 16 (1): 72-80, 2016. DOI: 10.5391/IJFIS.2016.16.1.72
- 3. Shakouri H, Nadimi GR: A novel fuzzy linear regression model based on a non-equality possibility index and optimum uncertainty. Appl Soft Comput, 9 (2): 590-598, 2009. DOI: 10.1016/j.asoc.2008.08.005
- 4. Namdari M, Yoon JH, Abadi A, Taheri SM, Choi SH: Fuzzy logistic regression with least absolute deviations estimators. Soft Comput, 19 (4): 909-917, 2015. DOI: 10.1007/s00500-014-1418-2
- 5. Chang YHO, Ayyub BM: Fuzyy regression methotds: A comparative assessment. Fuzzy Set Syst, 119 (2): 187-203, 2001. DOI: 10.1016/s0165- 0114(99)00091-3
- 6. Sohn SY, Kim DH, Yoon JH: Technology credit scoring model with fuzzy logistic regression. Appl Soft Comput, 43, 150-158, 2016. DOI: 10.1016/j.asoc.2016.02.025
- 7. Tanaka H, Uejima S, Asai K: Linear regression analysis with fuzzy model. IEEE Trans Syst Man Cybern, 12 (6): 903-907, 1982.
- 8. Atalik G, Senturk S: A new approach for parameter estimation in fuzzy regression. Iran J Fuzzy Syst, 15 (1): 91-102, 2018. DOI: 10.22111/ ijfs.2018.3580
- 9. Mustafa S, Asghar S, Hanif M: Fuzzy logistic regression based on least square approach and trapezoidal membership function. Iran J Fuzzy Syst, 15 (6): 97-106, 2018. DOI: 10.22111/IJFS.2018.4369
- 10. Taheri SM, Salmani F, Abadi A, Majd HA: A transition model for fuzzy correlated longitudinal responses. J Intell Fuzzy Syst, 30 (3): 1265- 1273, 2016.
- 11. Abdalla HA, El-Sayed AA, Hamed R: Fuzzy multinomial logistic regression analysis: A multi-objective programming approach. AIP Conf Proc, 1842:030017 2017. DOI: 10.1063/1.4982855
- 12. Nasrabadi MM, Nasrabadi E: A mathematical-programming approach to fuzzy linear regression analysis. Appl Math Comput, 155 (3): 873-881, 2004. DOI: 10.1016/j.amc.2003.07.031
- 13. Nagar P, Srivastava S: Adaptive fuzzy regression model for the prediction of dichotomous response variables using cancer data: A case study. J Appl Math Stat Inform, 4 (2): 183-191, 2008.
- 14. Namdari M, Abadi A, Taheri S M, Rezaei M, Kalantari M, Omidvar N: Effect of folic acid on appetite in children: Ordinal logistic and fuzzy logistic regressions. Nutrition, 30 (3): 274-278, 2014. DOI: 10.1016/j. nut.2013.08.008
- 15. Pourahmad S, Ayatollahi SMT, Taheri SM: Fuzzy logistic regression: A new possibilistic model and its application in clinical vague status. Iran J Fuzzy Syst, 8 (1): 1-17, 2011. DOI: 10.22111/IJFS.2011.232
- 16. Salmani F, Taheri SM, Yoon JH, Abadi A, Majd HA, Abbaszadeh A: Logistic regression for fuzzy covariates: modeling, inference, and applications. Int J Fuzzy Syst, 19 (5): 1635-1644, 2017. DOI: 10.1007/ s40815-016-0258-x
- 17. Gao Y, Lu Q: A fuzzy logistic regression model based on the least squares estimation. Comp Appl Math, 37 (3): 3562-3579, 2018. DOI: 10.1007/s40314-017-0531-0
- 18. Modarres M, Nasrabadi E, Nasrabadi MM: Fuzzy linear regression models with least square errors. Appl Math Comput, 163 (2): 977-989, 2005. DOI: 10.1016/j.amc.2004.05.004
- 19. Ghoshray S: Fuzzy linear regression analysis by symmetric triangular. Proceedings of IEEE International Conference on Intelligent Engineering Systems, 307-313, 1997. DOI: 10.1109/INES.1997.632436
- 20. Tanaka H, Guo P: Possibilistic data analysis for operations research. Studies in Fuzziness and Soft Computing Heidelberg, 194 page, PhysicaVerlag, New York. 1999.
- 21. Kao C, Chyu CL: A fuzzy linear regression model with better explanatory power. Fuzzy Sets Syst, 126 (3): 401-409, 2002. DOI: 10.1016/ s0165-0114(01)00069-0
- 22. Khan UT, Valeo C: A new fuzzy linear regression approach for dissolved oxygen prediction. Hydrolog Sci J, 60 (6): 1096-1119, 2015. DOI: 10.1080/02626667.2014.900558
- 23. Pourahmad S, Ayatollahi SMT, Taheri SM, Agahi ZH: Fuzzy logistic regression based on the least squares approach with application in clinical studies. Comput Math Appl, 62 (9): 3353-3365, 2011. DOI: 10.1016/j. camwa.2011.08.050
- 24. Yen KK, Ghoshray S, Roig G: Linear regression model using triangular fuzzy number coefficients. Fuzzy Sets Syst, 106 (2): 167-177, 1999. DOI: 10.1016/s0165-0114(97)00269-8
- 25. Chen LH, Chang CJ: Approaches to select suitable subset of explanatory variables for establishing fuzzy regression models. J Intell Fuzzy Syst, 34 (1): 437-457, 2018. DOI: 10.3233/JIFS-17206
- 26. Hosseinzadeh E, Hassanpour H, Arefi M, Aman M: A weighted goal programming approach to fuzzy linear regression with quasi type-2 fuzzy input-output data. TWMS J App Eng Math, 6 (2): 193-212, 2016. DOI: 10.1142/S0217595909002420
- 27. Tanak HA, Watada J: Possibilistic linear systems and their application to the linear regression model. Fuzzy Sets Syst,27 (3): 275-289, 1988. DOI: 10.1016/0165-0114(88)90054-1
- 28. Namdari M, Taheri SM, Abadi A, Rezaei M, Kalantari N: Possibilistic logistic regression for fuzzy categorical response data. 2013 IEEE International Conference on Fuzzy Systems (FUZZ-IEEE), 1-6, 2013. DOI: 10.1109/FUZZIEEE.2013.6622457
- 29. Tanaka H: Fuzzy data analysis by possibilistic linear models. Fuzzy Sets Syst, 24 (3): 363-375, 1987. DOI: 10.1016/0165-0114(87)90033-9
- 30. Memmedova N, Keskin İ: Oestrus detection by fuzzy logic model using trait activity in cows. Kafkas Univ Vet Fak Derg, 17 (6): 1003-1008, 2011. DOI: 10.9775/kvfd.2011.4960
- 31. Akıllı A, Atıl H, Takma Ç, Ayyılmaz T: Fuzzy logic-based decision support system for dairy cattle. Kafkas Univ Vet Fak Derg, 22 (1): 13-19, 2016. DOI: 10.9775/kvfd.2015.13516
- 32. Cihan P, Gökçe E, Kalıpsız O: A review of machine learning applications in veterinary field. Kafkas Univ Vet Fak Derg, 23 (4): 673-680, 2017. DOI: 10.9775/kvfd.2016.172816
APA | TOPUZ D (2021). Lactation Milk Yield Prediction with Possibilistic Logistic Regression Analysis. , 547 - 557. 10.9775/kvfd.2020.25171 |
Chicago | TOPUZ Derviş Lactation Milk Yield Prediction with Possibilistic Logistic Regression Analysis. (2021): 547 - 557. 10.9775/kvfd.2020.25171 |
MLA | TOPUZ Derviş Lactation Milk Yield Prediction with Possibilistic Logistic Regression Analysis. , 2021, ss.547 - 557. 10.9775/kvfd.2020.25171 |
AMA | TOPUZ D Lactation Milk Yield Prediction with Possibilistic Logistic Regression Analysis. . 2021; 547 - 557. 10.9775/kvfd.2020.25171 |
Vancouver | TOPUZ D Lactation Milk Yield Prediction with Possibilistic Logistic Regression Analysis. . 2021; 547 - 557. 10.9775/kvfd.2020.25171 |
IEEE | TOPUZ D "Lactation Milk Yield Prediction with Possibilistic Logistic Regression Analysis." , ss.547 - 557, 2021. 10.9775/kvfd.2020.25171 |
ISNAD | TOPUZ, Derviş. "Lactation Milk Yield Prediction with Possibilistic Logistic Regression Analysis". (2021), 547-557. https://doi.org/10.9775/kvfd.2020.25171 |
APA | TOPUZ D (2021). Lactation Milk Yield Prediction with Possibilistic Logistic Regression Analysis. Kafkas Üniversitesi Veteriner Fakültesi Dergisi, 27(5), 547 - 557. 10.9775/kvfd.2020.25171 |
Chicago | TOPUZ Derviş Lactation Milk Yield Prediction with Possibilistic Logistic Regression Analysis. Kafkas Üniversitesi Veteriner Fakültesi Dergisi 27, no.5 (2021): 547 - 557. 10.9775/kvfd.2020.25171 |
MLA | TOPUZ Derviş Lactation Milk Yield Prediction with Possibilistic Logistic Regression Analysis. Kafkas Üniversitesi Veteriner Fakültesi Dergisi, vol.27, no.5, 2021, ss.547 - 557. 10.9775/kvfd.2020.25171 |
AMA | TOPUZ D Lactation Milk Yield Prediction with Possibilistic Logistic Regression Analysis. Kafkas Üniversitesi Veteriner Fakültesi Dergisi. 2021; 27(5): 547 - 557. 10.9775/kvfd.2020.25171 |
Vancouver | TOPUZ D Lactation Milk Yield Prediction with Possibilistic Logistic Regression Analysis. Kafkas Üniversitesi Veteriner Fakültesi Dergisi. 2021; 27(5): 547 - 557. 10.9775/kvfd.2020.25171 |
IEEE | TOPUZ D "Lactation Milk Yield Prediction with Possibilistic Logistic Regression Analysis." Kafkas Üniversitesi Veteriner Fakültesi Dergisi, 27, ss.547 - 557, 2021. 10.9775/kvfd.2020.25171 |
ISNAD | TOPUZ, Derviş. "Lactation Milk Yield Prediction with Possibilistic Logistic Regression Analysis". Kafkas Üniversitesi Veteriner Fakültesi Dergisi 27/5 (2021), 547-557. https://doi.org/10.9775/kvfd.2020.25171 |