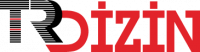
Sentinel 2 Uydu Görüntülerinden Bazı Bitki Türlerinin Makine Öğrenmesi ile Belirlenmesi
Yıl: 2021 Cilt: 9 Sayı: 1 Sayfa Aralığı: 189 - 200 Metin Dili: Türkçe DOI: 10.33202/comuagri.842202 İndeks Tarihi: 31-01-2022
Sentinel 2 Uydu Görüntülerinden Bazı Bitki Türlerinin Makine Öğrenmesi ile Belirlenmesi
Öz: Uydu görüntülerinden bitkisel ürünlerin sınıflandırılması tarım alanlarının yönetimi, gıda güvenliğinin sağlanması ve tarımsal politikaların oluşturulması için oldukça önemli bilgiler sağlar. Bitki türleri genel olarak uydu görüntülerinden hesaplanan vejetasyon indekslerine dayalı olarak veya çeşitli görüntü sınıflandırma teknikleri ile tahmin edilmektedir. Fakat bu yaklaşımlarda farklı bitkilerin benzer fenelojik ve spektral özelliklere sahip olması nedeniyle başarı oranı düşüktür. Bu nedenle bitki türlerinin uydu görüntüleri ile sınıflandırılması işleminde yeni, hassas ve başarılı bir yaklaşıma ihtiyaç duyulmaktadır. Bu çalışmanın amacı Rassal Orman (RO), Destek Vektör Makinesi (DVM) ve K-En Yakın Komşu (K-NN) makine öğrenme algoritmaları kullanılarak uydu görüntülerinden bitki türlerinin sınıflandırılmasıdır. Çalışma kapsamında 2020 yılı Gökhöyük Tarım İşletmesi Müdürlüğü’ne ait bitkisel üretim sahasında yetiştirilen bitkilerin sınıflandırılmasında zaman serisi biçiminde Sentinel 2 uydu görüntüleri kullanılmıştır. Çalışmadan elde edilen sonuçlara göre en başarılı sınıflandırma (%95.3) RO ile hesaplanırken en düşük başarı DVM ile elde edilmiştir (%75.9). K-NN ile yapılan sınıflandırma başarısı ise %91.8 olarak hesaplanmıştır.
Anahtar Kelime: Determination of Some Plant Species from Sentinel 2 Satellite Images by Machine Learning
Öz: Crop classification provides important information for agricultural management, ensuring food security and developing agricultural policy. Generally, crop species are estimated based on vegetation indexes calculated from satellite images or by using various image classification techniques. However, this approach has low success rate due to different crop species have similar phenological and spectral features. For this reason, a new, accurate and robust approach is needed. The aim of this study was to classify crop from satellite image by using Random Forest (RO), Support Vector Machines (DVM) and K-Nearest Neighbors (K-NN) machine learning algorithms. Within the scope of the study, Sentinel 2 satellite images were used in time series format for the crop classification of grown in the field of Gökhöyük Agricultural Enterprise Directorate in 2020. According to the result of this study, while the most successful classification result obtained from RO, the lowest success was obtained from DVM. Classification success with K-NN was calculated as 91.8%.
Anahtar Kelime: Belge Türü: Makale Makale Türü: Araştırma Makalesi Erişim Türü: Erişime Açık
- Baker, C. 1987. Changes in financial markets and their effects on agriculture. Federal Reserve Bank of St. Louis Review.
- Breiman L. 2001. Random forests,machine learning. Kluwer Academic Publishers. 45 (1): 5-32.
- Chakhar, A., Hernández-López, D., Ballesteros, R., Moreno, M. A. 2021. Improving the accuracy of multiple algorithms for crop classification by integrating sentinel-1 observations with sentinel-2 data. Remote Sensing. 13(2), 243.
- Chan, J. C., Paelinckx, D. 2008. Evaluation of random forest and adaboost tree-based ensemble classification and spectral band selection for ecotope mapping using airborne hyperspectral imagery. Remote Sensing of Environment. 112 (6): 2999-3011.
- Doğan, Z., Arslan, S., Berkman, A. N. 2015. Türkiye'de tarım sektörünün iktisadi gelişimi ve sorunları: tarihsel bir bakış. Academic Review of Economics & Administrative Sciences. 8(1): 1308-4208.
- Freund, Y., R. E. Schapire. 1997. A decision-theoretic generalization of on-line learning and an application to boosting. Journal of Computer and System Sciences. 55: 119–139.
- Hawryło, P., Bednarz, B., Wężyk, P., Szostak, M., 2018. Estimating defoliation of scots pine stands using machine learning methods and vegetation indices of sentinel-2. Eur. J. Remote Sens. 51: 194–204.
- Homer, C, Huang, C., Yang, L., Wylie, B., Coan, M. 2004. Development of a 2001 national land-cover database for the United States. Photogrammetric Engineering. 70(7): 829.
- Hooda, R. S., Yadav, M., Kalubarme, M. H. 2006. Wheat production estimation using remote sensing data: An Indian experience. Workshop Proceedings: Remote Sensing Support to Crop Yield Forecast and Area Estimates, Stresa, Italy. 30 Nov.–1 Dec. 2006.
- Huang, C., L. S. Davis, J. R. G. Townshend. 2002. An Assessment of support vector machines for land cover classification. International Journal of Remote Sensing. 23: 725– 749.
- Jay, S., Lawrence, R., Repasky, K., Keith, C. 2009. Invasive species mapping using low cost hyperspectral imagery. ASPRS 2009 Annual Conference. Baltimore, Maryland.
- Kumar, P., Gupta, D. K., Mishra, V. N., Prasad, R. 2015. Comparison of support vector machine, artificial neural network, and spectral angle mapper algorithms for crop classification using LISS IV data. International Journal of Remote Sensing. 36(6): 1604-1617.
- Li, L., Zheng, X., Zhao, K., Li, X., Meng, Z., Su, C. 2020. Potential evaluation of high spatial resolution multi-spectral ımages based on unmanned aerial vehicle in accurate recognition of crop types. Journal of the Indian Society of Remote Sensing.
- Maxwell, A. E., Warner, T. A., Fang, F. 2018. Implementation of machine-learning classification in remote sensing: An applied review. IJRS. 39(9): 2784-2817
- MGM, 2020. Meteoroloji Genel Müdürlüğü, Mevbis Sistemi.
- Mountrakis, G., Im, J., Ogole, C. 2011. Support vector machines in remote sensing: A review. ISPRS Journal of Photogrammetry and Remote Sensing. 66(3): 247-259.
- Ok, A. O., Akar, Ö., Güngör, O. 2012. Evaluation of random forest method for agricultural crop classification. European Journal of Remote Sensing. 45(1): 421-432.
- Pal, M., Foody, G. M. 2010. Feature selection for classification of hyperspectral data by SVM. IEEE Transactions on Geoscience and Remote Sensing. 48(5): 2297-2307.
- Pal, M., Foody, G. M. 2012. Evaluation of SVM, RVM and SMLR for accurate image classification with limited ground data. IEEE Journal of Selected Topics in Applied Earth Observations and Remote Sensing. 5(5): 1344-1355.
- Pal, M., Mather, P. M. 2003. An assessment of the effectiveness of decision tree methods for land cover classification. Remote sensing of environment. 86(4): 554-565.
- Pal, M., Mather, P. M. 2015. Support vector machines for classification in remote sensing. International journal of remote sensing. 26(5): 1007-1011.
- Pedregosa, F., Varoquaux, G., Gramfort, A., Michel, V., Thirion, B., Grisel, O., Blondel, M., Prettenhofer, P., Weiss, R., Dubourg, V. 2011. Scikit-learn: machine learning in Python. Journal of machine Learning research. 12: 2825-2830.
- Prasad, A. M., Iverson, L. R., Liaw, A. 2006. Newer classification and regression tree techniques: bagging and random forests for ecological prediction. Ecosystems. 9: 181-199.
- Scikit-learn. 2020. Scikit-learn: Machine learning in Python. https://scikit-learn.org
- Trier, Ø. D., Salberg, A. B., Kermit, M., Rudjord, Ø., Gobakken, T., Næsset, E., Aarsten, D. 2018. Tree species classification in Norway from airborne hyperspectral and airborne laser scanning data. European Journal of Remote Sensing. 51(1), 336-351.
- Uzundumlu, A. S. 2012. Tarım sektörünün ülke ekonomisindeki yeri ve önemi. Alinteri Journal of Agriculture Sciences. 22(1):1.
- Vapnik, V. N. 1998. Statistical learning theory. New York: Wiley.
- Waske, B., Braun, M. 2009. Classifier ensembles for land cover mapping using multiemporal SAR imagery. ISPRS Journal of Photogrammetry and Remote Sensing. 64: 450–457.
- Watts, J. D., Lawrence, R. L. 2008. Merging random forest classification with an object- oriented approach for analysis of agricultural lands. The International Archives of the Photogrammetry, Remote Sensing and Spatial Information Sciences. XXXVII(B7):1.
- Yang, C., Everitt, J. H., Murden, D. 2011. Evaluating high resolution SPOT 5 satellite imagery for crop identification. Computers and Electronics in Agriculture. 75 (2): 347.
- Zhang, H., Kang, J., Xu, X., Zhang, L. 2020. Accessing the temporal and spectral features in crop type mapping using multi-temporal Sentinel-2 imagery: A case study of Yi’an County, Heilongjiang province, China. Computers and Electronics in Agriculture. 176(05618).
APA | Tunca E, KOKSAL E (2021). Sentinel 2 Uydu Görüntülerinden Bazı Bitki Türlerinin Makine Öğrenmesi ile Belirlenmesi. , 189 - 200. 10.33202/comuagri.842202 |
Chicago | Tunca Emre,KOKSAL EYÜP SELIM Sentinel 2 Uydu Görüntülerinden Bazı Bitki Türlerinin Makine Öğrenmesi ile Belirlenmesi. (2021): 189 - 200. 10.33202/comuagri.842202 |
MLA | Tunca Emre,KOKSAL EYÜP SELIM Sentinel 2 Uydu Görüntülerinden Bazı Bitki Türlerinin Makine Öğrenmesi ile Belirlenmesi. , 2021, ss.189 - 200. 10.33202/comuagri.842202 |
AMA | Tunca E,KOKSAL E Sentinel 2 Uydu Görüntülerinden Bazı Bitki Türlerinin Makine Öğrenmesi ile Belirlenmesi. . 2021; 189 - 200. 10.33202/comuagri.842202 |
Vancouver | Tunca E,KOKSAL E Sentinel 2 Uydu Görüntülerinden Bazı Bitki Türlerinin Makine Öğrenmesi ile Belirlenmesi. . 2021; 189 - 200. 10.33202/comuagri.842202 |
IEEE | Tunca E,KOKSAL E "Sentinel 2 Uydu Görüntülerinden Bazı Bitki Türlerinin Makine Öğrenmesi ile Belirlenmesi." , ss.189 - 200, 2021. 10.33202/comuagri.842202 |
ISNAD | Tunca, Emre - KOKSAL, EYÜP SELIM. "Sentinel 2 Uydu Görüntülerinden Bazı Bitki Türlerinin Makine Öğrenmesi ile Belirlenmesi". (2021), 189-200. https://doi.org/10.33202/comuagri.842202 |
APA | Tunca E, KOKSAL E (2021). Sentinel 2 Uydu Görüntülerinden Bazı Bitki Türlerinin Makine Öğrenmesi ile Belirlenmesi. ÇOMÜ Ziraat Fakültesi Dergisi, 9(1), 189 - 200. 10.33202/comuagri.842202 |
Chicago | Tunca Emre,KOKSAL EYÜP SELIM Sentinel 2 Uydu Görüntülerinden Bazı Bitki Türlerinin Makine Öğrenmesi ile Belirlenmesi. ÇOMÜ Ziraat Fakültesi Dergisi 9, no.1 (2021): 189 - 200. 10.33202/comuagri.842202 |
MLA | Tunca Emre,KOKSAL EYÜP SELIM Sentinel 2 Uydu Görüntülerinden Bazı Bitki Türlerinin Makine Öğrenmesi ile Belirlenmesi. ÇOMÜ Ziraat Fakültesi Dergisi, vol.9, no.1, 2021, ss.189 - 200. 10.33202/comuagri.842202 |
AMA | Tunca E,KOKSAL E Sentinel 2 Uydu Görüntülerinden Bazı Bitki Türlerinin Makine Öğrenmesi ile Belirlenmesi. ÇOMÜ Ziraat Fakültesi Dergisi. 2021; 9(1): 189 - 200. 10.33202/comuagri.842202 |
Vancouver | Tunca E,KOKSAL E Sentinel 2 Uydu Görüntülerinden Bazı Bitki Türlerinin Makine Öğrenmesi ile Belirlenmesi. ÇOMÜ Ziraat Fakültesi Dergisi. 2021; 9(1): 189 - 200. 10.33202/comuagri.842202 |
IEEE | Tunca E,KOKSAL E "Sentinel 2 Uydu Görüntülerinden Bazı Bitki Türlerinin Makine Öğrenmesi ile Belirlenmesi." ÇOMÜ Ziraat Fakültesi Dergisi, 9, ss.189 - 200, 2021. 10.33202/comuagri.842202 |
ISNAD | Tunca, Emre - KOKSAL, EYÜP SELIM. "Sentinel 2 Uydu Görüntülerinden Bazı Bitki Türlerinin Makine Öğrenmesi ile Belirlenmesi". ÇOMÜ Ziraat Fakültesi Dergisi 9/1 (2021), 189-200. https://doi.org/10.33202/comuagri.842202 |