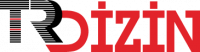
Metin Duygu sınıflandırılmasında hibrit wavelet yönteminin kullanımı
Yıl: 2021 Cilt: 36 Sayı: 2 Sayfa Aralığı: 701 - 714 Metin Dili: Türkçe DOI: 10.17341/gazimmfd.701313 İndeks Tarihi: 29-07-2022
Metin Duygu sınıflandırılmasında hibrit wavelet yönteminin kullanımı
Öz: Verilerin her geçen gün arttığı günümüzde herhangi bir metnin anlamsal ve duygusal çözümlemesi ihtiyaç duyulan konulardan biridir. Çalışmamız metinlerin sınıflandırılmasında kullanılabilecek üst anlam ilişkilerini çıkarmak ve metinlerin duygu sınıflandırmasını yapmak için yeni bir yöntem önermektedir.Bu yöntem daha önce metin analizinde çok az kullanılmış dalgacık dönüşüm yöntemidir. Çalışmamızda bu yöntemin klasik sınıflandırma algoritmaları ile birleştirilirmiş hali kullanılmaktadır. Dalgacık dönüşüm yöntemi metin içindeki anahtar kelimelerin üst anlamlarını ve temsil ettikleri ağırlıkları bulmaya yardım etmektedir. Duygu sınıflandırması probleminde, klasik yöntemler ile birlikte metin anahtar kelime vektörleri üzerinde dalgacık dönüşümü yapıldıktan sonra bulunan ağırlıkların kullanılması doğrulukları artırmıştır.
Anahtar Kelime: Document Sentiment classification using hybrid wavelet methodologies
Öz: Sentiment and semantic analysis of a text are very important issues of today because of increasing text data. Our study proposes a new method to reveal the hypernym relations (generic term) of the words in the text and to enhance the accuracy results of sentiment classification of the texts. We used wavelet transform method that has been rarely used in text analysis. In our study, the aim is to show how this method contributes the sentiment analysis classification problem. We used classical algorithms and hybrit wavelet algorithm for sentiment analysis problem. It has been observed that when wavelets are applied to classical classification algorithms, the accuracies increased approximately 5%.
Anahtar Kelime: Belge Türü: Makale Makale Türü: Araştırma Makalesi Erişim Türü: Erişime Açık
- 1. Akansu A.N. ve Smith M.J., Subband and wavelet transforms: design and applications, Springer Science & Business Media, 340, 2012. 2. Meyer Y. ve Ryan, R.D., Wavelets: Algorithms and Applications. SIAM, Philadelphia, PA, 1993.
- 3. Chan Y.T., Wavelet basics. Springer Science & Business Media, 1994.
- 4. Strang G. Ve Nguyen, T., Wavelets and Filter Banks,Wellesley-Cambridge Press, ISBN 0-9614088-7- 1 Box 812060, Wellesley MA 02181 USA, 1996.
- 5. Daubechies, I., Ten Lectures on Wavelets, Society for Industrial and Applied Mathematics, Capital City Press, Philadelphia, Pennsylvania, 1992.
- 6. Daubechies I., The wavelet transform, timefrequency localization and signal analysis. IEEE Trans. Inf. Theor., 36 (5), 961–1005, 2006.
- 7. Farkov Y. A., P. Manchanda A. H. ve Siddiqi, Construction of Wavelets Through Walsh Functions, ISBN - 978 -981-13-6370-2 (e-book), 382, 2019.
- 8. Polikar R., The story of wavelets. Physics and modern topics in mechanical and electrical engineering, 192- 197, 1999.
- 9. Siddiqi A. H., Manchanda, P. ve Kocvara, Fast waveletbased algorithms for option pricing. In Proc. world Multi conference on Systemic, Cybernetics and Informatics, 2002.
- 10. Li T., Li Q., Zhu S. ve Ogihara, M., A survey on wavelet applications in data mining. ACM SIGKDD Explorations Newsletter, 4 (2), 49-68, 2002.
- 11. Aggarwal C. C., On effective classification of strings with wavelets. In Proceedings of the eighth ACM SIGKDD international conference on Knowledge discovery and data mining, 163-172, 2002.
- 12. Xu C. ve Zhou Y. M., Wavelet-based hierarchical document categorization. In 2007 International Conference on Wavelet Analysis and Pattern Recognition IEEE, 4, 1524-1527, 2007.
- 13. Xexéo G., de Souza J., Castro P. F. ve Pinheiro W. A., Using wavelets to classify documents. In 2008 IEEE/WIC/ACM International Conference on Web Intelligence and Intelligent Agent Technology IEEE, 1, 272-278, 2008.
- 14. Mahajan A., Jat S., ve Roy S., Feature Selection for Short Text Classification using Wavelet Packet Transform. In Proceedings of the Nineteenth Conference on Computational Natural Language Learning, 321-326, 2015.
- 15. Alasmari S. F. ve Dahab, M., Sentiment detection, recognition and aspect identification. International Journal of Computer Applications, 975, 8887, 2017.
- 16. Bollen J., Mao H., ve Zeng X., Twitter mood predicts the stock market. Journal of computational science, 2 (1), 1-8, 2011.
- 17. Taboada M., Brooke J., Tofiloski M., Voll, K. ve Stede, M., Lexicon-based methods for sentiment analysis. Computational linguistics, 37 (2), 267-307, 2011.
- 18. Cambria E., Schuller B., Xia, Y. ve Havasi C., New avenues in opinion mining and sentiment analysis. IEEE Intelligent systems, 28 (2), 15-21, 2013.
- 19. Thelwall M., Buckley K., ve Paltoglou G., Sentiment strength detection for the social web. Journal of the American Society for Information Science and Technology, 63 (1), 163-173, 2012.
- 20. Kilimci Z.H., Financial Sentiment Analysis with Deep Community Models for Stock Market (DCM), Journal of the Faculty of Engineering and Architecture of Gazi University, 35 (2), 635-650, 2020.
- 21. Çoban Ö., Özyer B., ve Özyer G. T., Sentiment analysis for Turkish Twitter feeds. In 2015 23nd Signal Processing and Communications Applications Conference (SIU) IEEE, 2388-2391, 2015.
- 22. Chidambarathanu K. ve Shunmuganathan K. L., Predicting user preferences on changing trends and innovations using SVM based sentiment analysis. Cluster Computing, 1-5, 2017.
- 23. Zhang W., Kong S. X. ve Zhu Y. C., Sentiment classification and computing for online reviews by a hybrid SVM and LSA based approach. Cluster Computing, 22 (5), 12619-12632, 2019.
- 24. Akhtar M. S., Kumar A., Ghosal D., Ekbal A. ve Bhattacharyya P., A multilayer perceptron based ensemble technique for fine-grained financial sentiment analysis. In Proceedings of the 2017 Conference on Empirical Methods in Natural Language Processing, 540-546, 2017.
- 25. Alboaneen D. A., Tianfield H. ve Zhang Y., Sentiment analysis via multi-layer perceptron trained by metaheuristic optimisation. In 2017 IEEE International Conference on Big Data, 4630-4635, 2017.
- 26. Jotheeswaran J. ve Koteeswaran S., Decision tree based feature selection and multilayer perceptron for sentiment analysis. Journal of Engineering and Applied Sciences, 10 (14), 5883-5894, 2015.
- 27. Dang N. C., Moreno-García M. N. ve De la Prieta F., Sentiment analysis based on deep learning: A comparative study. Electronics, 9 (3), 483, 2020.
- 28. Taddy M., Multinomial inverse regression for text analysis. Journal of the American Statistical Association, 108 (503), 755-770, 2013.
- 29. You Q., Luo J., Jin H. ve Yang J., Cross-modality consistent regression for joint visual-textual sentiment analysis of social multimedia. In Proceedings of the Ninth ACM international conference on Web search and data mining 13-22, 2016.
- 30. Zhang Z., Zou Y. ve Gan, C., Textual sentiment analysis via three different attention convolutional neural networks and cross-modality consistent regression. Neurocomputing, 275, 1407-1415, 2018.
- 31. Akkaya C., Wiebe J. ve Mihalcea R., Subjectivity word sense disambiguation. In Proceedings of the 2009 conference on empirical methods in natural language processing 190-199, 2009.
- 32. Multi perspective question answering, Subjectivity sense annotations, http://mpqa.cs.pitt.edu/lexicons/subj_sense_ annotations/,Yayın tarihi: Ağustos 7, 2009, Erişim tarihi: Aralık 16, 2020.
- 33. Felix G, Surya K, Hagen M ve Sebastian Z. AspectBased Sentiment Analysis of Drug Reviews Applying Cross-Domain and Cross-Data Learning. In Proceedings of the 2018 International Conference on Digital Health, ACM, New York, NY, USA, 121-125, 2018.
- 34. UCI Machine Learning Repository, İlaç yorum Dataseti, https://archive.ics.uci.edu/ml/datasets/Drug+Review+D ataset+%28Drugs.com%29, Yayın tarihi: Ekim 4, 2018, Erişim tarihi: Aralık 16, 2020.
- 35. Nagesh H., Goil S. ve Choudhary A.N., UCI Machine Learning Repository, Adaptive Grids for Clustering Massive Data Sets. Department of Energy ASCI, 2020.
- 36. UCI Machine Learning Repository, Filim yorum Dataseti https://archive.ics.uci.edu/ml/datasets/Movie, Yayın tarihi: Temmuz 7, 1999, Erişim tarihi: Aralık 16, 2020.
APA | DONMEZ I, Aslan z (2021). Metin Duygu sınıflandırılmasında hibrit wavelet yönteminin kullanımı. , 701 - 714. 10.17341/gazimmfd.701313 |
Chicago | DONMEZ ILKNUR,Aslan zafer Metin Duygu sınıflandırılmasında hibrit wavelet yönteminin kullanımı. (2021): 701 - 714. 10.17341/gazimmfd.701313 |
MLA | DONMEZ ILKNUR,Aslan zafer Metin Duygu sınıflandırılmasında hibrit wavelet yönteminin kullanımı. , 2021, ss.701 - 714. 10.17341/gazimmfd.701313 |
AMA | DONMEZ I,Aslan z Metin Duygu sınıflandırılmasında hibrit wavelet yönteminin kullanımı. . 2021; 701 - 714. 10.17341/gazimmfd.701313 |
Vancouver | DONMEZ I,Aslan z Metin Duygu sınıflandırılmasında hibrit wavelet yönteminin kullanımı. . 2021; 701 - 714. 10.17341/gazimmfd.701313 |
IEEE | DONMEZ I,Aslan z "Metin Duygu sınıflandırılmasında hibrit wavelet yönteminin kullanımı." , ss.701 - 714, 2021. 10.17341/gazimmfd.701313 |
ISNAD | DONMEZ, ILKNUR - Aslan, zafer. "Metin Duygu sınıflandırılmasında hibrit wavelet yönteminin kullanımı". (2021), 701-714. https://doi.org/10.17341/gazimmfd.701313 |
APA | DONMEZ I, Aslan z (2021). Metin Duygu sınıflandırılmasında hibrit wavelet yönteminin kullanımı. Gazi Üniversitesi Mühendislik Mimarlık Fakültesi Dergisi, 36(2), 701 - 714. 10.17341/gazimmfd.701313 |
Chicago | DONMEZ ILKNUR,Aslan zafer Metin Duygu sınıflandırılmasında hibrit wavelet yönteminin kullanımı. Gazi Üniversitesi Mühendislik Mimarlık Fakültesi Dergisi 36, no.2 (2021): 701 - 714. 10.17341/gazimmfd.701313 |
MLA | DONMEZ ILKNUR,Aslan zafer Metin Duygu sınıflandırılmasında hibrit wavelet yönteminin kullanımı. Gazi Üniversitesi Mühendislik Mimarlık Fakültesi Dergisi, vol.36, no.2, 2021, ss.701 - 714. 10.17341/gazimmfd.701313 |
AMA | DONMEZ I,Aslan z Metin Duygu sınıflandırılmasında hibrit wavelet yönteminin kullanımı. Gazi Üniversitesi Mühendislik Mimarlık Fakültesi Dergisi. 2021; 36(2): 701 - 714. 10.17341/gazimmfd.701313 |
Vancouver | DONMEZ I,Aslan z Metin Duygu sınıflandırılmasında hibrit wavelet yönteminin kullanımı. Gazi Üniversitesi Mühendislik Mimarlık Fakültesi Dergisi. 2021; 36(2): 701 - 714. 10.17341/gazimmfd.701313 |
IEEE | DONMEZ I,Aslan z "Metin Duygu sınıflandırılmasında hibrit wavelet yönteminin kullanımı." Gazi Üniversitesi Mühendislik Mimarlık Fakültesi Dergisi, 36, ss.701 - 714, 2021. 10.17341/gazimmfd.701313 |
ISNAD | DONMEZ, ILKNUR - Aslan, zafer. "Metin Duygu sınıflandırılmasında hibrit wavelet yönteminin kullanımı". Gazi Üniversitesi Mühendislik Mimarlık Fakültesi Dergisi 36/2 (2021), 701-714. https://doi.org/10.17341/gazimmfd.701313 |