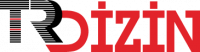
FastGTWR: Hızlı coğrafi ve zamansal ağırlıklı regresyon yaklaşımı
Yıl: 2021 Cilt: 36 Sayı: 2 Sayfa Aralığı: 715 - 726 Metin Dili: Türkçe DOI: 10.17341/gazimmfd.757131 İndeks Tarihi: 29-07-2022
FastGTWR: Hızlı coğrafi ve zamansal ağırlıklı regresyon yaklaşımı
Öz: Mekânsal analizler günümüzde önemli hale gelmiştir ve çok farklı uygulama alanlarında kullanılmaktadır. Yaygın olarak kullanılan konum temelli analiz yöntemlerinden biri olan Coğrafi Ağırlıklı Regresyon (Geographically Weighted Regression - GWR) coğrafya üzerindeki değişen ilişkileri modellemek için kullanılan bir yerel mekânsal regresyon tekniğidir. Coğrafi ve Zamansal Ağırlıklı Regresyon (Geographically and Temporal Weighted Regression - GTWR) ise GWR yaklaşımının verideki zamansal ilişkileri göz önüne almasıyla geliştirilen bir yaklaşımdır. Veri kümesinde mekân-zamansal heterojenliğin olduğu durumlarda GTWR yaklaşımı GWR yaklaşımına göre daha iyi modeller üretmesine rağmen mekânzamansal modellerin karmaşıklığı göz önüne alındığında algoritma zaman karmaşıklığı artmaktadır. Bu nedenle, literatürde bulunan GTWR algoritmaları sınırlı sayıdaki veri üzerinde çalışabilmektedir. Bu çalışmada, GTWR algoritmasının hızını arttırmak ve dolayısı ile veri boyutu kısıtlamasının üstesinden gelmek için hızlı bir GTWR algoritması olan FastGTWR yaklaşımı önerilmiştir. Önerilen FastGTWR yaklaşımının performansı gerçek ve sentetik veriler kullanılarak klasik GWR ve GTWR yaklaşımlarının performanslarıyla karşılaştırılmıştır. Deneysel sonuçlar önerilen FastGTWR yaklaşımının GWR ve GTWR yaklaşımlarına göre daha hızlı çalıştığını ortaya koymuştur.
Anahtar Kelime: FastGTWR: A fast geographically and temporally weighted regression approach
Öz: Spatial analysis has become more important today and is used in several application domains. Geographically Weighted Regression (GWR) method, which is one of the widely used spatial analysis methods, is the local spatial regression technique used to model the changing relationships on geography. Geographically and Temporal Weighted Regression (GTWR) is an approach developed by including temporal relations into the GWR approach. Although the GTWR approach produces much better models than the GWR approach in the dataset containing spatial-temporal heterogeneity, there are still challenges given the complexity of spatialtemporal approaches. Because of this reason, in the literature, GTWR algorithms can able to handle limited number of data. In this study, we proposed the FastGTWR approach to reduce the algorithmic complexity of GTWR approach and overcome data size restriction. The proposed FastGTWR approach was run on real and synthetic dataset. The performance of the proposed FastGTWR approach was compared with the performances of the classical GWR and GTWR approaches. Experimental results showed that the proposed FastGTWR approach works faster than the GWR and GTWR approaches.
Anahtar Kelime: Belge Türü: Makale Makale Türü: Araştırma Makalesi Erişim Türü: Erişime Açık
- 1. Perera, C., Zaslavsky, A., Christen, P., Georgakopoulos, D., Sensing as a service model for smart cities supported by internet of things, Transactions on Emerging Telecommunications Technologies, 25 (1), 81-93, 2014.
- 2. Prasad, A. V., Exploring the convergence of big data and the Internet of Things, IGI Global, 2017.
- 3. Fotheringham, A. S., Brunsdon, C. ve Charlton, M., Geographically weighted regression: the analysis of spatially varying relationships, John Wiley & Sons, 2003.
- 4. Huang, B., Wu, B., Barry, M., Geographically and temporally weighted regression for modeling spatiotemporal variation in house prices, International Journal of Geographical Information Science, 24 (3), 383-401, 2010.
- 5. Fotheringham, A. S., Crespo, R., Yao, J., Geographical and temporal weighted regression (GTWR), Geographical Analysis, 47 (4), 431-452, 2015.
- 6. Ma, X., Zhang, J., Ding, C., Wang, Y., A geographically and temporally weighted regression model to explore the spatiotemporal influence of built environment on transit ridership, Computers, Environment and Urban Systems, 70, 113-124, 2018.
- 7. Li, Z., Fotheringham, A. S., Li, W., Oshan, T., Fast Geographically Weighted Regression (FastGWR): a scalable algorithm to investigate spatial process heterogeneity in millions of observations, International Journal of Geographical Information Science, 33 (1), 155-175, 2019.
- 8. Taşyürek, M., Çelik, M., RNN-GWR: A geographically weighted regression approach for frequently updated data, Neurocomputing, 399, 258-270, 2020.
- 9. Li, Z., Fotheringham, A. S., Computational improvements to multi-scale geographically weighted regression, International Journal of Geographical Information Science, 1-20, 2020.
- 10. Oshan, T. M., Li, Z., Kang, W., Wolf, L. J., Fotheringham, A. S., mgwr: A Python implementation of multiscale geographically weighted regression for investigating process spatial heterogeneity and scale. ISPRS International Journal of Geo-Information, 8 (6), 269, 2019.
- 11. Guo, Y., Tang, Q., Gong, D. Y., Zhang, Z., Estimating ground-level PM2. 5 concentrations in Beijing using a satellite-based geographically and temporally weighted regression model, Remote Sensing of Environment, 198, 140-149, 2017.
- 12. He, Q., Huang, B., Satellite-based high-resolution PM2. 5 estimation over the Beijing-Tianjin-Hebei region of China using an improved geographically and temporally weighted regression model, Environmental Pollution, 236, 1027-1037, 2018.
- 13. Leong, Y. Y., Yue, J. C., A modification to geographically weighted regression, International Journal of Health Geographics, 16 (1), 11, 2017.
- 14. Zou, B., Pu, Q., Bilal, M., Weng, Q., Zhai, L., Nichol, J. E., High-resolution satellite mapping of fine particulates based on geographically weighted regression, IEEE Geoscience and Remote Sensing Letters, 13 (4), 495- 499, 2016.
- 15. Chu, H. J., Kong, S. J., Chang, C. H., Spatio-temporal water quality mapping from satellite images using geographically and temporally weighted regression, International Journal of Applied Earth Observation And Geoinformation, 65, 1-11, 2018.
- 16. Harris, R., Singleton, A., Grose, D., Brunsdon, C., Longley, P., Grid enabling geographically weighted regression: a case study of participation in higher education in England, Transactions in GIS, 14 (1), 43- 61, 2010.
- 17. Tran, H. T., Nguyen, H. T., Tran, V. T., Large-scale geographically weighted regression on Spark, In 2016 Eighth International Conference on Knowledge and Systems Engineering (KSE), IEEE, 127-132, 2016.
- 18. Pozdnoukhov, A., Kaiser, C., Scalable local regression for spatial analytics, In Proceedings of the 19th ACM SIGSPATIAL International Conference on Advances in Geographic Information Systems, 361-364, 2011.
- 19. Stergiou, C., Psannis, K. E., Kim, B. G., Gupta, B., Secure integration of IoT and cloud computing. Future Generation Computer Systems, 78, 964-975, 2018.
- 20. Yıldırım G., Tatar Y., Remote user supported IoT-WSN Laboratory and testbed platform: FiratWSN, Journal of the Faculty of Engineering and Architecture of Gazi University, 34 (4), 1831-1846, 2019.
- 21. Shekhar, S., Vatsavai, R.R., Çelik, M., Spatial and spatiotemporal data mining: Recent advances, Data Mining: Next Generation Challenges and Future Directions, 1-34, 2009.
- 22. Çelik M., Dadaser-Çelik, F., Dokuz A.S., Discovery of hydrometeorological patterns, Turkısh Journal Of Electrical Engineering and Computer Sciences, 22, 840- 857, 2014.
- 23. Türker A., Göleç A., Aktepe A., Ersöz S., İpek M., Çağıl G., A real-time system design using data mining for estimation of delayed orders and application, Journal of the Faculty of Engineering and Architecture of Gazi University, 35 (2), 709-724, 2019.
- 24. Tan, P. N., Steinbach, M. Karpatne, A. ve Kumar, V., Introduction to data mining. Pearson, 2018.
- 25. Çelik, M., Kazar, B. M., Shekhar, S. ve Boley, D., Parameter Estimation for the Spatial Autoregression Model: A Rigorous Approach, Proceedings of the Second NASA Data Mining Workshop: Issues and Applications in Earth Science, Pasadena, A.B.D., 2006.
- 26. Kazar, B. M. ve Çelik, M., Spatial autoregression (SAR) model: Parameter estimation techniques, Springer Briefs in Computer Science, ISBN:978-1461418412, Springer, March 2012.
- 27. Başar G., Mıstıkoğlu S., Determination of the optimum welding parameters for ultimate tensile strength and hardness in friction stir welding of Cu/Al plates using Taguchi method, Journal of the Faculty of Engineering and Architecture of Gazi University, 34 (3), 1595-1608, 2019.
- 28. Lu, B., Charlton, M., Brunsdon, C., Harris, P., The Minkowski approach for choosing the distance metric in geographically weighted regression, International Journal of Geographical Information Science, 30 (2), 351-368, 2016.
- 29. Fotheringham, A. S., Oshan, T. M., Geographically weighted regression and multicollinearity: dispelling the myth, Journal of Geographical Systems, 18 (4), 303- 329, 2016.
- 30. Lu, B., Yang, W., Ge, Y., Harris, P., Improvements to the calibration of a geographically weighted regression with parameter-specific distance metrics and bandwidths, Computers, Environment and Urban Systems, 71, 41-57, 2018.
- 31. Fotheringham, A. S., Yang, W., Kang, W., Multiscale geographically weighted regression (MGWR), Annals of the American Association of Geographers, 107 (6), 1247-1265, 2017.
- 32. Da Silva, A. R. , De Oliveira Lima, A., Geographically weighted beta regression, Spatial Statistics, 21, 279-303, 2017.
- 33. Gollini, I., Lu, B., Charlton, M., Brunsdon, C., Harris, P., GWmodel: an R package for exploring spatial heterogeneity using geographically weighted models, arXiv preprint arXiv:1306.0413, 2013.
- 34. Guo, L., Ma, Z., Zhang, L., Comparison of bandwidth selection in application of geographically weighted regression: a case study, Canadian Journal of Forest Research, 38 (9), 2526-2534, 2008.
- 35. Hadayeghi, A., Shalaby, A. S., Persaud, B. N., Development of planning level transportation safety tools using Geographically Weighted Poisson Regression, Accident Analysis & Prevention, 42 (2), 676-688, 2010.
- 36. Bidanset, P. E., Lombard, J. R., The effect of kernel and bandwidth specification in geographically weighted regression models on the accuracy and uniformity of mass real estate appraisal, Journal of Property Tax Assessment & Administration, 11 (3), 5-14, 2014.
- 37. Kauermann, G., Opsomer, J. D., Generalized crossvalidation for bandwidth selection of backfitting estimates in generalized additive models, Journal of Computational and Graphical Statistics, 13 (1), 66-89, 2004.
- 38. Zougab, N., Adjabi, S., Kokonendji, C. C., Bayesian estimation of adaptive bandwidth matrices in multivariate kernel density estimation, Computational Statistics & Data Analysis, 75, 28-38, 2014.
- 39. Taşyürek, M., Çelik, M., Hava Sıcaklık Değerlerinin Coğrafi ve Rakım Ağırlıklı Regresyon Yöntemi ile Tahmin Edilmesi, Avrupa Bilim ve Teknoloji Dergisi, 81-86, 2020.
APA | Taşyürek M, Celik M (2021). FastGTWR: Hızlı coğrafi ve zamansal ağırlıklı regresyon yaklaşımı. , 715 - 726. 10.17341/gazimmfd.757131 |
Chicago | Taşyürek Murat,Celik Mete FastGTWR: Hızlı coğrafi ve zamansal ağırlıklı regresyon yaklaşımı. (2021): 715 - 726. 10.17341/gazimmfd.757131 |
MLA | Taşyürek Murat,Celik Mete FastGTWR: Hızlı coğrafi ve zamansal ağırlıklı regresyon yaklaşımı. , 2021, ss.715 - 726. 10.17341/gazimmfd.757131 |
AMA | Taşyürek M,Celik M FastGTWR: Hızlı coğrafi ve zamansal ağırlıklı regresyon yaklaşımı. . 2021; 715 - 726. 10.17341/gazimmfd.757131 |
Vancouver | Taşyürek M,Celik M FastGTWR: Hızlı coğrafi ve zamansal ağırlıklı regresyon yaklaşımı. . 2021; 715 - 726. 10.17341/gazimmfd.757131 |
IEEE | Taşyürek M,Celik M "FastGTWR: Hızlı coğrafi ve zamansal ağırlıklı regresyon yaklaşımı." , ss.715 - 726, 2021. 10.17341/gazimmfd.757131 |
ISNAD | Taşyürek, Murat - Celik, Mete. "FastGTWR: Hızlı coğrafi ve zamansal ağırlıklı regresyon yaklaşımı". (2021), 715-726. https://doi.org/10.17341/gazimmfd.757131 |
APA | Taşyürek M, Celik M (2021). FastGTWR: Hızlı coğrafi ve zamansal ağırlıklı regresyon yaklaşımı. Gazi Üniversitesi Mühendislik Mimarlık Fakültesi Dergisi, 36(2), 715 - 726. 10.17341/gazimmfd.757131 |
Chicago | Taşyürek Murat,Celik Mete FastGTWR: Hızlı coğrafi ve zamansal ağırlıklı regresyon yaklaşımı. Gazi Üniversitesi Mühendislik Mimarlık Fakültesi Dergisi 36, no.2 (2021): 715 - 726. 10.17341/gazimmfd.757131 |
MLA | Taşyürek Murat,Celik Mete FastGTWR: Hızlı coğrafi ve zamansal ağırlıklı regresyon yaklaşımı. Gazi Üniversitesi Mühendislik Mimarlık Fakültesi Dergisi, vol.36, no.2, 2021, ss.715 - 726. 10.17341/gazimmfd.757131 |
AMA | Taşyürek M,Celik M FastGTWR: Hızlı coğrafi ve zamansal ağırlıklı regresyon yaklaşımı. Gazi Üniversitesi Mühendislik Mimarlık Fakültesi Dergisi. 2021; 36(2): 715 - 726. 10.17341/gazimmfd.757131 |
Vancouver | Taşyürek M,Celik M FastGTWR: Hızlı coğrafi ve zamansal ağırlıklı regresyon yaklaşımı. Gazi Üniversitesi Mühendislik Mimarlık Fakültesi Dergisi. 2021; 36(2): 715 - 726. 10.17341/gazimmfd.757131 |
IEEE | Taşyürek M,Celik M "FastGTWR: Hızlı coğrafi ve zamansal ağırlıklı regresyon yaklaşımı." Gazi Üniversitesi Mühendislik Mimarlık Fakültesi Dergisi, 36, ss.715 - 726, 2021. 10.17341/gazimmfd.757131 |
ISNAD | Taşyürek, Murat - Celik, Mete. "FastGTWR: Hızlı coğrafi ve zamansal ağırlıklı regresyon yaklaşımı". Gazi Üniversitesi Mühendislik Mimarlık Fakültesi Dergisi 36/2 (2021), 715-726. https://doi.org/10.17341/gazimmfd.757131 |