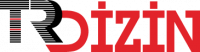
Kanser hastalığı ile ilgili paylaşımlar için Dirichlet ayrımı ile gizli konu modelleme
Yıl: 2021 Cilt: 36 Sayı: 4 Sayfa Aralığı: 2183 - 2196 Metin Dili: Türkçe DOI: 10.17341/gazimmfd.734730 İndeks Tarihi: 29-07-2022
Kanser hastalığı ile ilgili paylaşımlar için Dirichlet ayrımı ile gizli konu modelleme
Öz: Sosyal medya ortamlarında, kullanıcılar yaşadıkları olaylar ile ilgili edindikleri tecrübeleri paylaşmaktadır. Kişiler başlarından geçen bir olayı, yeni gördükleri bir şehri, okudukları kitabı vb. paylaşarak aynı belirli konular hakkında diğer kişilere deneyimlerini aktarmaktadır. Sosyal medya kullanan bireylerin konuştuğu konulardan biri de sağlık problemleri ve bu konudaki deneyimlerin paylaşılmasıdır. Sağlık problemi yaşayan bazı bireyler, geçirdikleri hastalıkları, gördüğü tedavileri ve sonuçlarını, her bir evresinde kazandıkları tecrübeleri sosyal ortamlarda yazarak paylaşmaktadır. Bu paylaşımlar gerek bilgilendirici gerekse hastalıkla mücadelede moral/motivasyon için diğer hastalar açısından önem arz etmektedir. Paylaşım sayısının fazla olması, hastalıkların çeşitliği ve veri miktarının büyüklüğü nedeniyle insan tarafından manuel olarak yorumlanması imkânsız hale gelmektedir. Bu çalışmada, Reddit sosyal platformu üzerinden, kanser hastalığı ile ilgili paylaşımlar toplanarak bu veriler üzerinde çalışılmıştır. Bu paylaşımlar üzerinden yapay zekâ tabanlı konu modelleme algoritmalarından “Gizli Dirichlet Ayrımı (GDA)” algoritması ile konuşulan başlıca konu başlıkları bulunmuştur. Konu başlıklarının konuşulan konu ile ilişkisi incelenmiş ve içerik analizi yapılmıştır. Kanser hastalığı ile ilgili paylaşımlar içerisinde en fazla konuşulan içeriklerin belirlenmesi hedeflenmiştir. Ayrıca t-SNE tekniği kullanılarak konuların birbiri arasındaki ilişkisi incelenmiştir. GDA algoritması ile modelleme sonucunda elde edilen konu başlıklarında bulunan kelimelerin yapılan tutarlılık testinde uyumlu olduğu görülmüştür.
Anahtar Kelime: Topic modeling with latent Dirichlet allocation for cancer disease posts
Öz: In social media platforms, users share their experiences about the events they have experienced. People talk about a recent event, a city they have just seen, a book they read, etc. They post their experiences with other people about the same specific issues. One of the topics that users often talk about is health problems and sharing their experiences on this subject. Individuals with health problems can share their illnesses, treatments and results, and the experiences they have gained at each stage in social media platforms. These shares are important for other patients, both for informative and for morale / motivation in combating the disease. Manual analysis of the posts by human beings becomes impossible due to reasons such as the high number of posts, the variety of diseases and the amount of data. In this study, posts about cancer disease were collected on the Reddit social platform and these data were studied. The main topics discussed with the “Latent Dirichlet Allocation (LDA)” algorithm, one of the artificial intelligence-based topic modeling algorithms, were found through these posts. The relationship of the subject headings with the spoken subject was examined and content analysis was made. It is aimed to determine the most talked about contents among the posts about cancer disease. In addition, the relationship between the subjects was examined using the tSNE technique. It was observed that the words in the topics obtained as a result of modeling with the LDA algorithm were compatible in the coherence test.
Anahtar Kelime: Belge Türü: Makale Makale Türü: Araştırma Makalesi Erişim Türü: Erişime Açık
- 1. Bhat, M. R., Kundroo, M. A., Tarray, T. A., ve Agarwal, B., Deep LDA: A new way to topic model, Journal of Information and Optimization Sciences, 41 (3), 823- 834, 2019.
- 2. Alexa. Alexa Top 500 Global Sites. http://alexa.com/topsites/. Erişim Tarihi Şubat 10 2020.
- 3. Blei, D. M., Ng, A. Y., ve Jordan, M. I., Latent Dirichlet Allocation, Journal of Machine Learning Research, 3, 993-1022, 2003.
- 4. Dan Foresee, F. ve Hagan, M. T., Gauss-Newton approximation to Bayesian learning, Proceedings of International Conference on Neural Networks (ICNN’97), Houston-USA, 1930-1935, 12 Haziran, 1997.
- 5. Okon, E., Rachakonda, V., Hong, H. J., Callison-Burch, C., ve Lipoff, J., Natural Language Processing of Reddit Data to Evaluate Dermatology Patient Experiences and Therapeutics, Journal of the American Academy of Dermatology, 83 (3), 803-808, 2020.
- 6. Ding, S., Li, Z., Liu, X., Huang, H., ve Yang, S., Diabetic complication prediction using a similarityenhanced latent Dirichlet allocation model, Information Sciences, 499, 12–24, 2019.
- 7. Chen, C. ve Ren, J., Forum latent Dirichlet allocation for user interest discovery, Knowledge-Based Systems, 126, 1–7, 2017.
- 8. Bastani, K., Namavari, H., ve Shaffer, J., Latent Dirichlet allocation (LDA) for topic modeling of the CFPB consumer complaints, Expert Systems with Applications, 127, 256–271, 2019.
- 9. Wang, W., Feng, Y., ve Dai, W., Topic analysis of online reviews for two competitive products using latent Dirichlet allocation, Electronic Commerce Research and Applications, 29, 142–156, May, 2018.
- 10. Hagen, L., Content analysis of e-petitions with topic modeling: How to train and evaluate LDA models?, Information Processing & Management, 54 (6), 1292– 1307, 2018.
- 11. Griffiths, T. L. ve Steyvers, M., Finding scientific topics, Proceedings of the National Academy of Sciences, 101 (1), 5228–5235, 2004.
- 12. Wang, Y., Agichtein, E., ve Benzi, M., TM-LDA: efficient online modeling of latent topic transitions in social media, KDD '12: Proceedings of the 18th ACM SIGKDD international conference on Knowledge discovery and data mining, Beijing- China, 123-131, 12- 16 Ağustos, 2012.
- 13. Xu, Z., Ru, L., Xiang, L., ve Yang, Q., Discovering User Interest on Twitter with a Modified Author-Topic Model, 2011 IEEE/WIC/ACM International Conferences on Web Intelligence and Intelligent Agent Technology, Lyon-Fransa, 422-429, 22-27 Ağustos 2011.
- 14. Xu, H., Zhang, F., ve Wang, W., Implicit feature identification in Chinese reviews using explicit topic mining model, Knowledge-Based Systems, 76, 166– 175, 2015.
- 15. Güven Z. A., Diri B., Çakaloğlu T., Comparison of nstage Latent Dirichlet Allocation versus other topic modeling methods for emotion analysis, Journal of the Faculty of Engineering and Architecture of Gazi University, 35 (4), 2135-2145, 2020.
- 16. Lu, Y., Mei, Q., ve Zhai, C., Investigating task performance of probabilistic topic models: an empirical study of PLSA and LDA, Information Retrieval, 14 (2), 178–203, 2011.
- 17. Blei, D. M., Probabilistic topic models, Communications of the ACM, 55 (4), 77-86, 2012.
- 18. Landauer, T. K. ve Dutnais, S. T., a Solution to platos problem: The latent semantic analysis theory of acquisition, induction, and representation of knowledge, Psychological review,104 (2), 211–240, 1997.
- 19. Lee, D. D. ve Seung, H. S., Algorithms for non-negative matrix Factorization, 14th Annual Neural Information Processing Systems Conference, NIPS 2000 , DenverUSA, 556–562, 27 Kasım-2 Aralık, 2000.
- 20. Stevens, K., Kegelmeyer, P., Andrzejewski, D., ve Buttler, D., Exploring Topic Coherence over Many Models and Many Topics, Proceedings of the 2012 Joint Conference on Empirical Methods in Natural Language Processing and Computational Natural Language Learning, Jeju Island, Korea, 952–961, 5 Temmuz, 2012.
- 21. Wallach, H. M., Mimno, D., ve McCallum, A., Rethinking LDA: Why priors matter, Advances in Neural Information Processing Systems 22-Proceedings of the 2009 Conference, Vancouver- Kanada 1973– 1981, 7-10 Aralık, 2009.
- 22. Reddit, http://www.reddit.com, Erişim Tarihi 10 Ocak 2020
- 23. PRAW, http://praw.readthedocs.io, Erişim Tarihi 17 Eylül 2019.
- 24. Nltk, http://www.nltk.org, Erişim Tarihi 17 Eylül 2019.
- 25. Joachims, T., A probabilistic analysis of the Rocchio algorithm with TFIDF for text categorixation, Proceedings of ICML97, San Francisco-USA,143-151, 8-12 Temmuz, 1997.
- 26. Lee, D. D. ve Seung, H. S., Learning the parts of objects by non-negative matrix factorization, Nature, 401, (6755), 788–791, 1999.
- 27. Altıntaş, V., Topal, K., ve Albayrak, M., Sosyal Medya Platformu Üzerinde Gizli Anlam Analizi, European Journal of Science and Technology, 16, 863–869, 2019.
- 28. Röder, M., Both, A., ve Hinneburg, A., Exploring the Space of Topic Coherence Measures, Proceedings of the Eighth ACM International Conference on Web Search and Data Mining, New York-USA 399–408, 2-6 Şubat, 2015.
- 29. Maaten, L. van der ve Hinton, G., Visualizing Data using t-SNE, Journal of Machine Learning Research, 9, 2579–2605, 2008.
- 30. Sievert, C. ve Shirley, K., LDAvis: A method for visualizing and interpreting topics, Proceedings of the Workshop on Interactive Language Learning, Visualization, and Interfaces, Maryland-USA 63–70, 2014.
- 31. Hidayatullah, A. F., Ma’arif, M. R., Road traffic topic modeling on Twitter using latent Dirichlet allocation, International Conference on Sustainable Information Engineering and Technology (SIET17), Batu, Indonesia 47-52, 24-25 Kasım, 2017.
APA | ALTINTAS V, Albayrak M, Topal K (2021). Kanser hastalığı ile ilgili paylaşımlar için Dirichlet ayrımı ile gizli konu modelleme. , 2183 - 2196. 10.17341/gazimmfd.734730 |
Chicago | ALTINTAS VOLKAN,Albayrak Mehmet,Topal Kamil Kanser hastalığı ile ilgili paylaşımlar için Dirichlet ayrımı ile gizli konu modelleme. (2021): 2183 - 2196. 10.17341/gazimmfd.734730 |
MLA | ALTINTAS VOLKAN,Albayrak Mehmet,Topal Kamil Kanser hastalığı ile ilgili paylaşımlar için Dirichlet ayrımı ile gizli konu modelleme. , 2021, ss.2183 - 2196. 10.17341/gazimmfd.734730 |
AMA | ALTINTAS V,Albayrak M,Topal K Kanser hastalığı ile ilgili paylaşımlar için Dirichlet ayrımı ile gizli konu modelleme. . 2021; 2183 - 2196. 10.17341/gazimmfd.734730 |
Vancouver | ALTINTAS V,Albayrak M,Topal K Kanser hastalığı ile ilgili paylaşımlar için Dirichlet ayrımı ile gizli konu modelleme. . 2021; 2183 - 2196. 10.17341/gazimmfd.734730 |
IEEE | ALTINTAS V,Albayrak M,Topal K "Kanser hastalığı ile ilgili paylaşımlar için Dirichlet ayrımı ile gizli konu modelleme." , ss.2183 - 2196, 2021. 10.17341/gazimmfd.734730 |
ISNAD | ALTINTAS, VOLKAN vd. "Kanser hastalığı ile ilgili paylaşımlar için Dirichlet ayrımı ile gizli konu modelleme". (2021), 2183-2196. https://doi.org/10.17341/gazimmfd.734730 |
APA | ALTINTAS V, Albayrak M, Topal K (2021). Kanser hastalığı ile ilgili paylaşımlar için Dirichlet ayrımı ile gizli konu modelleme. Gazi Üniversitesi Mühendislik Mimarlık Fakültesi Dergisi, 36(4), 2183 - 2196. 10.17341/gazimmfd.734730 |
Chicago | ALTINTAS VOLKAN,Albayrak Mehmet,Topal Kamil Kanser hastalığı ile ilgili paylaşımlar için Dirichlet ayrımı ile gizli konu modelleme. Gazi Üniversitesi Mühendislik Mimarlık Fakültesi Dergisi 36, no.4 (2021): 2183 - 2196. 10.17341/gazimmfd.734730 |
MLA | ALTINTAS VOLKAN,Albayrak Mehmet,Topal Kamil Kanser hastalığı ile ilgili paylaşımlar için Dirichlet ayrımı ile gizli konu modelleme. Gazi Üniversitesi Mühendislik Mimarlık Fakültesi Dergisi, vol.36, no.4, 2021, ss.2183 - 2196. 10.17341/gazimmfd.734730 |
AMA | ALTINTAS V,Albayrak M,Topal K Kanser hastalığı ile ilgili paylaşımlar için Dirichlet ayrımı ile gizli konu modelleme. Gazi Üniversitesi Mühendislik Mimarlık Fakültesi Dergisi. 2021; 36(4): 2183 - 2196. 10.17341/gazimmfd.734730 |
Vancouver | ALTINTAS V,Albayrak M,Topal K Kanser hastalığı ile ilgili paylaşımlar için Dirichlet ayrımı ile gizli konu modelleme. Gazi Üniversitesi Mühendislik Mimarlık Fakültesi Dergisi. 2021; 36(4): 2183 - 2196. 10.17341/gazimmfd.734730 |
IEEE | ALTINTAS V,Albayrak M,Topal K "Kanser hastalığı ile ilgili paylaşımlar için Dirichlet ayrımı ile gizli konu modelleme." Gazi Üniversitesi Mühendislik Mimarlık Fakültesi Dergisi, 36, ss.2183 - 2196, 2021. 10.17341/gazimmfd.734730 |
ISNAD | ALTINTAS, VOLKAN vd. "Kanser hastalığı ile ilgili paylaşımlar için Dirichlet ayrımı ile gizli konu modelleme". Gazi Üniversitesi Mühendislik Mimarlık Fakültesi Dergisi 36/4 (2021), 2183-2196. https://doi.org/10.17341/gazimmfd.734730 |