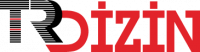
Öznitelik Seçme Yöntemlerinin Makine Öğrenmesi Tabanlı Saldırı Tespit Sistemi Performansına Etkileri
Yıl: 2021 Cilt: 12 Sayı: 5 Sayfa Aralığı: 743 - 755 Metin Dili: Türkçe DOI: 10.24012/dumf.1051340 İndeks Tarihi: 29-07-2022
Öznitelik Seçme Yöntemlerinin Makine Öğrenmesi Tabanlı Saldırı Tespit Sistemi Performansına Etkileri
Öz: Artan İnternet tabanlı teknolojilerin kullanımı insanlara ve kurumlara önemli avantajlar sağlamanın yanı sıra bir takım dezavantajları da beraberinde getirmiştir. Bunlardan en önemlisi siber saldırılardır. Siber saldırıların çeşitlenmesi ve artmasıyla, büyük miktarlara ulaşan kritik verilerin silme, değiştirilme, ifşa edilme gibi eylemlere karşı korunması her geçen gün daha zor hale gelmektedir. Bu sebeple bilgi sistemlerinin güvenliğinin sağlanması amaçlı geliştirilen araçlardan biri olan Saldırı Tespit Sistemleri çok önemli yere sahip bir çalışma alanı olmuştur. Bu çalışmada, CSE-CIC-IDS2018 veri kümesi üzerinde literatürde önerilen çeşitli öznitelik seçim yöntemleri ve makine öğrenmesi teknikleri kullanılarak, öznitelik seçiminin Saldırı Tespit Sistemi başarım ve performansı üzerindeki etkisi incelenmiştir. Orijinal veri kümesini temsil edebilecek en iyi alt kümeyi belirlemek için Ki-Kare Testi, Spearman‘ın Sıralama Korelasyon Katsayısı ve Özyinelemeli Öznitelik Eliminasyonu yöntemleri kullanılmıştır. Yeni veri kümeleri Adaptif Yükseltme, Karar Ağacı, Lojistik Regresyon, Çok Katmanlı Algılayıcı, Ekstra Ağaçlar, Pasif-Agresif ve Gradyan Artırma makine öğrenmesi yöntemleri ile sınıflandırılarak performans sonuçlarının karşılaştırmalı bir analizi yapılmıştır. Performansların objektif değerlendirilebilmesi için K-Fold kullanılmıştır. K-Fold işleminin hesaplama ve zaman yönünden maliyetli olması sebebiyle paralleştirme uygulanarak işlem süresi düşürülmüştür. Elde edilen deneysel sonuçlara göre Ki-Kare Testi ve Spearman’ın Sıralama Korelasyon Katsayısı öznitelik seçim yöntemleri veri boyutunun indirgenmesinden dolayı işlem yükünü azaltarak işlem süresini %45 oranında kısaltmış fakat hata oranını sırasıyla %14,46 ve %10,52 artırmıştır. Ayrica, Özyinelemeli Öznitelik Eliminasyonu yönteminin uygun ayar parametreleri kullanıldığında, işlem süresini %38 oranında kısaltması ile birlikte sistemin hata oranını da %2,95’e kadar düşürdüğü görülmüştür.
Anahtar Kelime: Effects of Feature Selection Methods on Machine Learning Based Intrusion Detection System Performance
Öz: The increasing use of the Internet-based technologies has brought along some disadvantages as well as providing significant advantages to people and institutions. The most important of these disadvantages is cyber-attacks. With the variety and increase of cyber-attacks, it becomes more and more difficult to protect large amounts of critical data against actions such as deletion, modification and disclosure. For this reason, Intrusion Detection Systems, one of the tools developed to ensure the security of information systems, has become a very important study area. In this study, the effect of feature selection on Intrusion Detection System performance and success, was investigated. The study was developed on the CSE-CIC-IDS2018 dataset by using various feature selection methods and machine learning techniques suggested in the literature. Chi-Square Test, Spearman's Ranking Correlation Coefficient and Recursive Feature Elimination methods were used to determine the best subset that could represent the original dataset. The new datasets created with the features determined by each feature selection method were classified using Adaptive Boosting, Decision Tree, Logistic Regression, Multilayer Perceptron, Extra Trees, PassiveAggressive and Gradient Boosting machine learning methods, and a comparative analysis of the obtained performance results was made. K-Fold was used to evaluate the performances objectively. Since the KFold process is costly in terms of computation and time, the processing time is reduced by applying parallelization. According to the experimental results obtained, Chi-Square Test and Spearman's Ranking Correlation Coefficient feature selection methods reduced the processing load due to the reduction of the data size and shortened the processing time by 45%, but increased the error rate by 14.46% and 10.52% respectively. On the other hand, it has been observed that the Recursive Feature Elimination method reduces the processing time by 38% and the error rate of the system up to 2.95% when appropriate setting parameters are used.
Anahtar Kelime: Belge Türü: Makale Makale Türü: Araştırma Makalesi Erişim Türü: Erişime Açık
- [1] Kim, K., Aminanto, M.E., Tanuwidjaja, H.C. (2018). Network Intrusion Detection Using Deep Learning SpringerBriefs on Cyber Security Systems and Networks.
- [2] Mishra, P., Varadharajan, V., Tupakula, U., Pilli, E.S. (2018). A Detailed Investigation and Analysis of Using Machine Learning Technique for Intrusion Detection. IEEE, 2018.
- [3] Gao, X., Shan, C., Hu, C., Niu, Z., Liu, Z. (2019). An Adaptive Ensemble Machine Learning Model for Intrusion Detection. IEEE, 2019.
- [4] Sharafaldin, I., Lashkari A.H., Ghorbani A. (2018). Toward Generating a New Intrusion Detection Dataset and Intrusion Traffic Characterizaion. ICISSP.
- [5] Zhou, Y., Cheng, G., Jiang, S., Dai, M. (2019). An Efficient Intrusion Detection System Based on Feature Selection and Ensemble Classifier.
- [6] Sommer, R., Paxson, V. (2010). Outside the Closed World: On Using Machine Learning for Network Intrusion Detection”, IEEE Symposium on security and Privacy.
- [7] Aljawarneh, S., Aldawairi, M., Yassein, M. B. (2018) Anomaly-based Intrusion Detection System Through Feature Selection Analysis and Build Hybrid Efficient Model. Journal of Computational Science.
- [8] Wankhede, S., Kshirsagar, D. (2018). DoS Attack Detection Using Machine Learning and Neural Network. 2018 Fourth International Conference on Computing Communication Control and Automation (ICCUBEA), Pune, India, pp. 1-5. Conference on Information Systems Security and Privacy (ICISSP), Portugal.
- [9] Zhou, Q., Pezaros, D., (2019). Evaluation of Machine Learning Classifier for Zero-Day Intrusion detectionAn Analysis on CIC AWS 2018 Dataset. School of Computing Science, University of Glasgow.
- [10] Kanimozhi, V., Jacob, T.P. (2019). Artificial Intelligence based Network Intrusion Detection with Hyper-Parameter Optimization Tuning on The Realistic Cyber Dataset CSE-CIC-IDS2018 Using Cloud Computing”. International Conference on Communication and Signal Processing.
- [11] Yulianto, A, Sukarno, P., Suwastika, N. A. (2017). Improving AdaBoost-based Intrusion Detection System (IDS) Performance on CIC IDS 2017 Dataset. Journal of Physics: Conference Series, 1192.
- [12] Wani, A.R., Rana, Q. P., Saxena, U., Pandey, N. (2019). Analysis and Detection of DDoS Attacks on Cloud Computing Environment using Machine Learning Techniques," 2019 Amity International Conference on Artificial Intelligence (AICAI), Dubai, United Arab Emirates, pp. 870-875.
- [13] McKay, R., Pendleton, B., Britt, J., Nakhavanit, B. (2019). Machine Learning Algorithms on Botnet Traffic: Ensemble and Simple Algorithms. The International Conference on Compute and Data Analysis 2019 (ICCDA).
- [14] Kanimozhi, V., Jacob, T.P. (2019). Calibration of Various Optimized Machine Learning Classifiers in Network Intrusion Detection System on the Realistic Cyber Dataset CSE-CIC-IDS2018 Using Cloud Computing. International Journal of Engineering AppliedSciencesandTechnology,2019 Vol.4, Issue 6, ISSN No. 2455-2143, Pages 209-213.
- [15] Ferrag, M.A., Maglaras, L. (2019). DeliveryCoin: An IDS and Blockchain-Based Delivery Framework for Drone-Delivered Services. Computers 2019, 8, 58.
- [16] Atay, R., Odabaş, D. E., Pehlivanoğlu, M. K. (2019). İki Seviyeli Hibrit Makine Öğrenmesi Yöntemi ile Saldırı Tespiti. Dergipark, 258-272.
- [17] De Lima Filho, F.S., Silveira, F.A.F., De Medeiros Brito Junior, A., Vargas-Solar, G., Silveira, L.F. (2019). Smart Detection: An Online Approach for DoS/DDoS Attack Detection Using Machine Learning,” Security and Communication Networks, vol. 2019, Article ID 1574749, 15 pages.
- [18] Fitni, Q.R.S., Ramli, K. (2020). Implementation of ensemble learning and feature selection for performance improvements in anomaly-based intrusion detection systems", Proc. IEEE Int. Conf. Ind. 4.0 Artif. Intell. Commun. Technol. (IAICT), pp. 118-124.
- [19] Cil, A. E., Yildiz, K., Buldu, A. (2021). Detection of DDoS attacks with feed forward based deep neural network model. Expert Systems with Applications, 169, 114520.
- [20] Arslan, R. S. (2021). FastTrafficAnalyzer: An Efficient Method for Intrusion Detection Systems to Analyze Network Traffic. Dicle Üniversitesi Mühendislik Fakültesi Mühendislik Dergisi, 12(4), 565-572.
- [21] Emhan, Ö., Akın, M. (2019). Filtreleme tabanlı öznitelik seçme yöntemlerinin anomali tabanlı ağ saldırısı tespit sistemlerine etkisi. Dicle Üniversitesi Mühendislik Fakültesi Mühendislik Dergisi, 10(2), 549-559.
- [22] Thomas, R., Pavithran, D. (2018). A Survey of Intrusion Detection Models based on NSL-KDD Data Set. 2018 Fifth HCT Information Technology Trends (ITT), Dubai, United Arab Emirates, 286-291.
- [23] Athmaja, S., Hanumanthappa, M., Kavitha, V. (2017). A Survey of Machine Learning Algorithms for Big Data Analytics. 2017 International Conference on Innovations in Information, Communication Coimbatore, 1-4.
- [24] Sahingoz, O, Çebi, C, Bulut, F, Fırat, H, Karataş, G. (2019). Saldırı Tespit Sistemlerinde Makine Öğrenmesi Modellerinin Karşılaştırılması. Erzincan Üniversitesi Fen Bilimleri Enstitüsü Dergisi 12 (2019): 1513-1525
- [25] Amrita, M.A. (2013) Performance Analysis of Different Feature Selection Methods in Intrusion Detection. Int J Sci Technol Res 2(6):225–231
- [26] CSE-CIC-IDS-2018 dataset from University of NewBrunswick, available online: https://www.unb.ca/cic/datasets/ids-2018.html
- [27] CICFlowMeter: Network Traffic Flow Analyzer,http://netflowmeter.ca/netflowmeter.html, Accessed 28 July 2018.
- [28] Saeys, Y., Inza, I., Larranaga, P. (2007). A Review of Feature Selection Techniques in Bioinformatics, Bioinformatics, 23(19), 2507-2517.
- [29] Bisyron, W., Kalamullah, R., Hendri, M. (2018). Implementation and Analysis of Combined Machine Learning Method for Intrusion Detection System. International Journal of Communication Networks and Information Security.
- [30] Zhang W.Y., Wei Z.W, Wang, B.H., Han, X.P. (2016). Measuring Mixing Patterns in Complex Neteorks by Spearman Rank Correlation Coefficient, Physica A 451.
- [31] Solomatine, D.P., Shrestha, D.L. (2004). AdaBoost. RT: A Boosting Algorithm for Regression Problems, Neural Networks, Vol 2, 1163 – 1168.
- [32] Bauer, E., Kohavi, R. (1999). An Empirical Comparison of Voting Classification Algorithms: Bagging, Boosting, and Variants, Machine Learning., Volume 36, Issue 1, pp 105-139.
- [33] Geurts, P., Ernst, D., Wehenkel, L. (2006). Extremely randomized trees. Machine learning 63(1): 3-42.
- [34] Çatal, Ç., Özyılmaz, L. (2005). Analysis of Multiple Myeloma Gene Expression Data by Multilayer Perceptron, National Conference on Biomedical Engineering.
- [35] Sokolova, M., Lapalme, G. (2009). A Systematics Analysis of Performance Measures for Classification Tasks. Information processing and management.
- [36] Scikit-learn.org. scikit-learn: machine learning in Python — scikit-learn 1.0.1 documentation. [online] https://scikit-learn.org/stable/, 2021.
- [37] NumPy. Numpy documentation. [online] https://numpy.org/, 2021.
- [38] Pandas. Pandas Python Data Analysis Library documentation. [online] https://pandas.pydata.org/, 2021.
APA | Emanet Ş, Karatas Baydogmus G, Demir O (2021). Öznitelik Seçme Yöntemlerinin Makine Öğrenmesi Tabanlı Saldırı Tespit Sistemi Performansına Etkileri. , 743 - 755. 10.24012/dumf.1051340 |
Chicago | Emanet Şura,Karatas Baydogmus Gozde,Demir Onder Öznitelik Seçme Yöntemlerinin Makine Öğrenmesi Tabanlı Saldırı Tespit Sistemi Performansına Etkileri. (2021): 743 - 755. 10.24012/dumf.1051340 |
MLA | Emanet Şura,Karatas Baydogmus Gozde,Demir Onder Öznitelik Seçme Yöntemlerinin Makine Öğrenmesi Tabanlı Saldırı Tespit Sistemi Performansına Etkileri. , 2021, ss.743 - 755. 10.24012/dumf.1051340 |
AMA | Emanet Ş,Karatas Baydogmus G,Demir O Öznitelik Seçme Yöntemlerinin Makine Öğrenmesi Tabanlı Saldırı Tespit Sistemi Performansına Etkileri. . 2021; 743 - 755. 10.24012/dumf.1051340 |
Vancouver | Emanet Ş,Karatas Baydogmus G,Demir O Öznitelik Seçme Yöntemlerinin Makine Öğrenmesi Tabanlı Saldırı Tespit Sistemi Performansına Etkileri. . 2021; 743 - 755. 10.24012/dumf.1051340 |
IEEE | Emanet Ş,Karatas Baydogmus G,Demir O "Öznitelik Seçme Yöntemlerinin Makine Öğrenmesi Tabanlı Saldırı Tespit Sistemi Performansına Etkileri." , ss.743 - 755, 2021. 10.24012/dumf.1051340 |
ISNAD | Emanet, Şura vd. "Öznitelik Seçme Yöntemlerinin Makine Öğrenmesi Tabanlı Saldırı Tespit Sistemi Performansına Etkileri". (2021), 743-755. https://doi.org/10.24012/dumf.1051340 |
APA | Emanet Ş, Karatas Baydogmus G, Demir O (2021). Öznitelik Seçme Yöntemlerinin Makine Öğrenmesi Tabanlı Saldırı Tespit Sistemi Performansına Etkileri. Dicle Üniversitesi Mühendislik Fakültesi Mühendislik Dergisi, 12(5), 743 - 755. 10.24012/dumf.1051340 |
Chicago | Emanet Şura,Karatas Baydogmus Gozde,Demir Onder Öznitelik Seçme Yöntemlerinin Makine Öğrenmesi Tabanlı Saldırı Tespit Sistemi Performansına Etkileri. Dicle Üniversitesi Mühendislik Fakültesi Mühendislik Dergisi 12, no.5 (2021): 743 - 755. 10.24012/dumf.1051340 |
MLA | Emanet Şura,Karatas Baydogmus Gozde,Demir Onder Öznitelik Seçme Yöntemlerinin Makine Öğrenmesi Tabanlı Saldırı Tespit Sistemi Performansına Etkileri. Dicle Üniversitesi Mühendislik Fakültesi Mühendislik Dergisi, vol.12, no.5, 2021, ss.743 - 755. 10.24012/dumf.1051340 |
AMA | Emanet Ş,Karatas Baydogmus G,Demir O Öznitelik Seçme Yöntemlerinin Makine Öğrenmesi Tabanlı Saldırı Tespit Sistemi Performansına Etkileri. Dicle Üniversitesi Mühendislik Fakültesi Mühendislik Dergisi. 2021; 12(5): 743 - 755. 10.24012/dumf.1051340 |
Vancouver | Emanet Ş,Karatas Baydogmus G,Demir O Öznitelik Seçme Yöntemlerinin Makine Öğrenmesi Tabanlı Saldırı Tespit Sistemi Performansına Etkileri. Dicle Üniversitesi Mühendislik Fakültesi Mühendislik Dergisi. 2021; 12(5): 743 - 755. 10.24012/dumf.1051340 |
IEEE | Emanet Ş,Karatas Baydogmus G,Demir O "Öznitelik Seçme Yöntemlerinin Makine Öğrenmesi Tabanlı Saldırı Tespit Sistemi Performansına Etkileri." Dicle Üniversitesi Mühendislik Fakültesi Mühendislik Dergisi, 12, ss.743 - 755, 2021. 10.24012/dumf.1051340 |
ISNAD | Emanet, Şura vd. "Öznitelik Seçme Yöntemlerinin Makine Öğrenmesi Tabanlı Saldırı Tespit Sistemi Performansına Etkileri". Dicle Üniversitesi Mühendislik Fakültesi Mühendislik Dergisi 12/5 (2021), 743-755. https://doi.org/10.24012/dumf.1051340 |