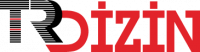
Döküman dili tanıma için içerik bağımsız yeni bir yaklaşım: Açı Örüntüler
Yıl: 2022 Cilt: 37 Sayı: 3 Sayfa Aralığı: 1277 - 1292 Metin Dili: Türkçe DOI: 10.17341/gazimmfd.844700 İndeks Tarihi: 29-07-2022
Döküman dili tanıma için içerik bağımsız yeni bir yaklaşım: Açı Örüntüler
Öz: Metin madenciliğinde dil tanıma (DT), bir belgenin veya bir kısmının yazıldığı doğal dili algılama çalışmasıdır. Bu çalışmada, karakterlerin UTF-8 değerleri arasında kalan açı bilgisini kullanan metinden yeni bir dil tanıma yaklaşımı önerilmiştir. Önerilen açı yöntemi metinlerden öznitelik çıkarımı için kullanılmıştır. Açı örüntüler yöntemi istatistiksel bir yaklaşımdır. Önerilen yaklaşımı test etmek amacıyla çeşitli şekillerde oluşturulan dört veri setinin kullanılması kararlaştırılmıştır. Elde edilen öznitelikler Rastsal Orman (RO, RF, Random Forest), Destek Vektör Makinesi (DVM, SVM, Support Vector Machine), Liner Diskriminant Analiz (LDA, Linear Discriminant Analysis), Naive Bayes (NB) ve k-en yakın komşu (Knn, k-nearest neighbors) olmak üzere farklı sınıflandırma yöntemleri kullanılmıştır. Dört farklı veri seti kümesinden belirlenen DT başarım sonuçları sırası ile %96,81, %99,39, %93,31 ve %98,60 olarak gözlenmiştir. Yapılan çalışma sonucunda ulaşılan başarım sonuçlarına göre önerilen açı örüntüler yönteminin DT uygulamasında önemli ayırt edici bilgiler verdiği belirlenmiştir.
Anahtar Kelime: A new content-free approach to identification of document language: Angle Patterns
Öz: Language identification (LI) in text mining is the study of natural language perception in which a document or a part of it is written. In this study, a new language identification approach from text using the angle information between the UTF-8 values of the characters is proposed. The proposed angle method is used for feature extraction from texts. Angle patterns method is a statistical approach. It was decided to use four data sets created in various ways to test the proposed approach. The obtained features are used with different classification methods such as RF( Random Forest), SVM (Support Vector Machine), LDA (Linear Discriminant Analysis), NB (Naive Bayes) and Knn (k-nearest neighbor). LI performance results determined from four different data set sets were observed as 96.81%, 99.39%, 93.31% and 98.60%, respectively. According to the success results obtained as a result of the study, it was determined that the proposed angle patterns method gave important distinctive information in LI application.
Anahtar Kelime: Belge Türü: Makale Makale Türü: Araştırma Makalesi Erişim Türü: Erişime Açık
- 1. Başkaya, F. & Aydin, İ., Classification of news texts by different text mining methods, In 2017 International Artificial Intelligence and Data Processing Symposium (IDAP), 1-5, 2017.
- 2. Kul, S., Natural language processıng on the way to Turkısh lecturer artıfıcıal ıntellıgence, Journal of Management Information Systems, 6 (2), 43-56, 2020.
- 3. Ong, E.J., Cooper, H., Pugeault, N., Bowden, R., Sign language recognition using sequential pattern trees, Conference on Computer Vision and Pattern Recognition, Washington-USA, 2200–2207, 16-21 Haziran, 2012.
- 4. Aksu, M. Ç., Karaman, E., Comparison of fastText and Bag of Words Word Representation Methods by Using Turkish Reviews Conducted for Touristic Places, European Journal of Science and Technology, 20, 311- 320, 2020.
- 5. Ali, C.B., Haddad, H., Slimani, Y., Empirical evaluation of compounds indexing for turkish texts, Computer Speech & Language, 56, 95-106, 2019.
- 6. Amasyali, M. F., Yıldırım, T., Automatic text categorization of news articles, Proceedings of the IEEE 12th Signal Processing and Communications Applications Conference, Kusadasi- Turkey, 224–226, 28-30 April, 2004.
- 7. Tang, B., He, H., Baggenstoss, P.M., Kay, S., A Bayesian Classification Approach Using Class-Specific Features for Text Categorization, IEEE Transactions on Knowledge and Data Engineering, 28 (6), 1602–1606, 2016.
- 8. Fragkou, P., Text segmentation for language identification in Greek forums, Procedia-Social and Behavioral Sciences, 147, 160-166, 2014.
- 9. Abainia, K., Ouamour, S., Sayoud, H., Effective language identification of forum texts based on statistical approaches, Information Processing & Management, 52 (4), 491-512, 2016.
- 10. Johnson, R., Zhang, T., Effective Use of Word Order for Text Categorization with Convolutional Neural Networks, arXiv:1412.1058v2, 2014.
- 11. Lui, M., Lau, J.H., Baldwin, T., Automatic detection and language identification of multilingual documents. Transactions of the Association for Computational Linguistics, 2, 27–40, 2014.
- 12. Cavnar, W.B., Trenkle, J.M., N-gram-based text categorization, 3rd Annual Symposium on Document Analysis and Information Retrieval, Las VegasNevadaUSA, 161–175, 11-13 April, 1994.
- 13. Kaya, Y., Ertuğrul, Ö.F., A novel feature extraction approach for text-based language identification: Binary patterns, Journal of the Faculty of Engineering and Architecture of Gazi University, 31 (4), 1085-1094, 2016.
- 14. Sarma, N., Singh, S.R., Goswami, D., Influence of social conversational features on language identification in highly multilingual online conversations, Information Processing & Management, 56 (1), 151-166, 2019.
- 15. Takçı, H., Ekinci, E., Minimal feature set in language identification and finding suitable classification method with it, Procedia Technology, 1, 444–448, 2012.
- 16. Gamallo, P., Pichel, J.R., Alegria, I., From language identification to language distance, Physica A: Statistical Mechanics and its Applications, 484, 152- 162, 2017.
- 17. Takcı, H., Soğukpınar, İ., Letter based text scoring method for language identification, International Conference on Advances in Information Systems, İzmir-Türkiye, 283-290, 20-22 October, 2004.
- 18. Evans, D.A., Grefenstette, G.T., Tong X., Method of identifying the language of a textual passage using short word and/or n-gram comparisons, U.S. Patent No: US7359851, Washington, DC: U.S. Patent and Trademark Office, 15 April, 2008.
- 19. Popescu, M., Dinu, L.P., Kernel methods and string kernels for authorship identification: The federalist papers case, International Conference on Recent Advances in Natural Language Processing (RANLP07), Borovets-Bulgaria, 27-29 September, 2007.
- 20. Popescu, M., Grozea, C., Kernel methods and string kernels for authorship analysis Notebook for PAN at CLEF, Conference and Labs of the Evaluation Forum, Rome-Italy, 17-20 September, 2012.
- 21. Popescu, M., Ionescu, R.T., The Story of the Characters, the DNA and the Native Language, Eighth Workshop on Innovative Use of NLP for Building Educational Applications, Atlanta-GA-USA, 270–278, 13 June, 2013.
- 22. Ahmed, B., Cha, S.H., Tappert, C., Language identification from text using n-gram based cumulative frequency addition, Proceedings of Student/Faculty Research Day, CSIS, Pace University, 12.1-12.8, 7 May, 2004.
- 23. Gary, F. Simons and Charles, D. Fennig, editors. Ethnologue: Languages of the World, Twentieth Edition. SIL International, Dallas, USA, 2017.
- 24. Acı, Ç., Çırak, A., Turkish News Articles Categorization Using Convolutional Neural Networks and Word2Vec, Journal of Information Technologies, 12 (3), 219-228, 2019.
- 25. Öztürk, Ö., Abidin, D., Özacar, T., Using classification algorithms for Turkish music makam recognition, Selcuk University Journal of Engineering, Science and Technology, 6 (3), 377-393, 2018.
- 26. Basile, A., Dwyer, G., Medvedeva, M., Rawee, J., Haagsma, H., & Nissim, M., N-gram: New groningen author-profiling model, arXiv preprint arXiv:1707.03764, 2017.
- 27. Tohma, K., Kutlu, Y., Challenges Encountered in Turkish Natural Language Processing Studies, Natural and Engineering Sciences, 5 (3), 204-211 , 2020.
- 28. Tian, J., Suontausta, J., Scalable neural network based language identification from written text, In 2003 IEEE International Conference on Acoustics, Speech, and Signal Processing, Proceedings.(ICASSP'03), 1, I-48, 2003.
- 29. Özcan T., Baştürk A., ERUSLR: A new Turkish sign language dataset and its recognition using hyperparameter optimization aided convolutional neural network, Journal of Gazi University Faculty of Engineering and Architecture, 36 (1), 527-542, 2020.
- 30. Kuncan F., Kaya Y., Kuncan, M., New approaches based on local binary patterns for gender identification from sensor signals, Journal of the Faculty of Engineering and Architecture of Gazi University, 34 (4), 2173-2185, 2019.
- 31. Li, G., Li, J., Ju, Z., Sun, Y., & Kong, J., A novel feature extraction method for machine learning based on surface electromyography from healthy brain, Neural Computing and Applications, 31 (12), 9013-9022, 2019.
- 32. Kuncan, M., Kaplan, K., Minaz, M. R., Kaya, Y., & Ertunc, H. M., A novel feature extraction method for bearing fault classification with one dimensional ternary patterns, ISA transactions, 100, 346-357, 2020.
- 33. Gumaei, A., Hassan, M. M., Hassan, M. R., Alelaiwi, A., & Fortino, G., A hybrid feature extraction method with regularized extreme learning machine for brain tumor classification, IEEE Access, 7, 36266-36273, 2019.
- 34. Takçı, H., Güngör, T., A high performance centroidbased classification approach for language identification, Pattern Recognition Letters, 33 (16), 2077-2084, 2012.
- 35. Xiao, D., Li, Y. K., Zhang, H., Sun, Y., Tian, H., Wu, H., & Wang, H., ERNIE-Gram: Pre-Training with Explicitly N-Gram Masked Language Modeling for Natural Language Understanding, arXiv preprint arXiv:2010.12148, 2020.
- 36. Suzuki, I., Mikami, Y., Ohsato A., Chubachi, Y., A language and character set determination method based on N-gram statistics, ACM Transactions on Asian Language Information Processing, 1 (3), 269-278, 2002.
- 37. Castro, D.W., Souza, E., Vitório, D., Santos, D., Oliveira, A. L., Smoothed n-gram based models for tweet language identification: A case study of the brazilian and european portuguese national varieties, Applied Soft Computing, 61, 1160-1172, 2017.
- 38. Zheng, L., Liang, B., Sign language recognition using depth images, 14th International Conference on Control, Automation, Robotics and Vision (ICARCV), PhuketThailand, 1-6, 13-15 Kasım, 2016.
- 39. Zhang, X., Zhao, J., LeCun, Y., Character-level Convolutional Networks for Text Classification, Advances in Neural Information Processing Systems, Curran Associates Inc., 649-657, 2015.
- 40. Güven Z., Dı̇rı̇ B., Çakaloğlu T., Comparison of n-stage Latent Dirichlet Allocation versus other topic modeling methods for emotion analysis, Journal of the Faculty of Engineering and Architecture of Gazi University, 35 (4), 2135-2145, 2020.
- 41. Durmuş G., Soğukpınar İ., A novel approach for analyzing buffer overflow vulnerabilities in binary executables by using machine learning techniques, Journal of the Faculty of Engineering and Architecture of Gazi University, 34 (4), 1695-1704, 2019.
- 42. Yücesoy E., Nabiyev V.V., Determination of a speaker’s age and gender with an SVM classifier based on GMM supervectors, Journal of the Faculty of Engineering and Architecture of Gazi University, 31 (3), 501-509, 2016.
- 43. Poutsma, A., Applying Monte Carlo techniques to language identification, In: Proceedings of Computational Linguistics in the Netherlands, 2001.
- 44. Binas, A., Markovian Time Series Models for Language Identification, Project Report, Available: http://www.cs.toronto.edu/ abinas/csc2515report.pdf (online), 2005.
- 45. Xafopoulos, A., Kotropoulos, C., Almpanidis, G., Pitas, I., Language identification in web documents using discrete HMMs, Pattern Recognition, 37 (3), 583-594, 2004.
- 46. Li, Q., Chen, Y.P., Personalized text snippet extraction using statistical language models, Pattern Recognition, 43 (1), 378-386, 2010.
- 47. Sibun, P., Reynar, J.C., Language identification: examining the issues, In: Proc.5th Symposium on Document Analysis and Information Retrieval, Las Vegas-Nevada-USA, 125–135, 15-17 April, 1996.
- 48. Song, Y., Dai, L., Wang, R.., An automatic language identification method based on subspace analysis, IEEE International Conference on Multimedia and Expo, New York-NY-USA, 598-601, 28 Jun - 03 Jul, 2009.
- 49. Takci H., Diagnosis of breast cancer by the help of centroid based classifiers, Journal of the Faculty of Engineering and Architecture of Gazi University, 31 (2), 323-330, 2016.
- 50. Sagiroglu, S., Yavanoglu, U., & Guven, E.N., Web based machine learning for language identification and translation. In Sixth International Conference on Machine Learning and Applications (ICMLA 2007), 280-285, 2007.
- 51. Selamat, A., Ng, C.C., Arabic script web page language identifications using decision tree neural networks, Pattern Recognition, 44 (1), 133-144, 2011.
- 52. Köklü M., Kahramanlı H., Allahverdi N., A new accurate and efficient approach to extract classification rules, Journal of the Faculty of Engineering and Architecture of Gazi University, 29 (3), 477-486, 2014.
- 53. Jo, T., Normalized table-matching algorithm as approach to text categorization, Soft Computing, 19 (4), 839–849, 2015.
- 54. Tan S., An effective refinement strategy for KNN text classifier, Expert Systems with Applications, 30 (2), 290-298, 2006.
- 55. Murthy, K.N., Kumar, G.B., Language identification from small text samples, Journal of Quantitative Linguistics, 13 (01), 57-80, 2006.
- 56. Jiang, C., Coenen, F., Sanderson, R., Zito, M., Text classification using graph mining-based feature extraction, Knowledge-Based Systems, 23 (4), 302- 308, 2010.
- 57. Botha, G.R., Barnard, E., Factors that affect the accuracy of text-based language identification, Computer Speech & Language, 26 (5), 307-320, 2012.
- 58. Hayta, Ş.B., Takçı, H., Eminli M., Language Identification Based on n-Gram Feature Extraction Method by Using Classifiers, IU-Journal of Electrical & Electronics Engineering, 13 (2), 1629-1639, 2013.
- 59. Yavanoğlu U., Sağıroğlu Ş., Automatic web based language identification and translation system, Journal of the Faculty of Engineering and Architecture of Gazi University, 25 (3), 483-494, 2010.
- 60. Singh, A.K., Study of some distance measures for language and encoding identification, In Proceedings of the Workshop on Linguistic Distances, 63-72, 2006.
- 61. Gottron, T., Lipka, N., A comparison of language identification approaches on short, query-style texts, In European Conference on Information Retrieval, Springer, Berlin, Heidelberg, 611-614, March, 2010.
- 62. Baldwin, T., Lui, M., Language identification: The long and the short of the matter, In Human language technologies: The 2010 annual conference of the North American Chapter of the Association for Computational Linguistics, 229-237, June, 2010.
- 63. Tromp, E., Pechenizkiy, M., Graph-based n-gram language identification on short texts, In Proc. 20th Machine Learning conference of Belgium and The Netherlands, 27-34, May, 2011.
- 64. Hakkinen, J., & Tian, J., N-gram and decision tree based language identification for written words, In IEEE Workshop on Automatic Speech Recognition and Understanding, ASRU'01, 335-338, 2001.
- 65. Carreras, X., Chao, I., Padró, L., Padró, M., FreeLing: An Open-Source Suite of Language Analyzers, In LREC, 239-242, May, 2004.
- 66. Zhai, L.F., Siu, M., Yang, X., Gish, H., Discriminatively trained language models using support vector machines for language identification, In: IEEE Odyssey 2006: The Speaker and Language Recognition Workshop, 1–6, 2006.
- 67. Ljubesic, N., Mikelic, N., Boras, D., Language indentification: How to distinguish similar languages?, In 2007 29th International Conference on Information Technology Interfaces, 541-546, June, 2007.
- 68. Martin, T., The WiLI benchmark dataset for written language identification, https:// arxiv. org/ pdf / 1801 . 07779 . pdf, 2020.
APA | NOYAN T, KUNCAN F, Tekin R, KAYA Y (2022). Döküman dili tanıma için içerik bağımsız yeni bir yaklaşım: Açı Örüntüler. , 1277 - 1292. 10.17341/gazimmfd.844700 |
Chicago | NOYAN Tuba,KUNCAN Fatma,Tekin Ramazan,KAYA YILMAZ Döküman dili tanıma için içerik bağımsız yeni bir yaklaşım: Açı Örüntüler. (2022): 1277 - 1292. 10.17341/gazimmfd.844700 |
MLA | NOYAN Tuba,KUNCAN Fatma,Tekin Ramazan,KAYA YILMAZ Döküman dili tanıma için içerik bağımsız yeni bir yaklaşım: Açı Örüntüler. , 2022, ss.1277 - 1292. 10.17341/gazimmfd.844700 |
AMA | NOYAN T,KUNCAN F,Tekin R,KAYA Y Döküman dili tanıma için içerik bağımsız yeni bir yaklaşım: Açı Örüntüler. . 2022; 1277 - 1292. 10.17341/gazimmfd.844700 |
Vancouver | NOYAN T,KUNCAN F,Tekin R,KAYA Y Döküman dili tanıma için içerik bağımsız yeni bir yaklaşım: Açı Örüntüler. . 2022; 1277 - 1292. 10.17341/gazimmfd.844700 |
IEEE | NOYAN T,KUNCAN F,Tekin R,KAYA Y "Döküman dili tanıma için içerik bağımsız yeni bir yaklaşım: Açı Örüntüler." , ss.1277 - 1292, 2022. 10.17341/gazimmfd.844700 |
ISNAD | NOYAN, Tuba vd. "Döküman dili tanıma için içerik bağımsız yeni bir yaklaşım: Açı Örüntüler". (2022), 1277-1292. https://doi.org/10.17341/gazimmfd.844700 |
APA | NOYAN T, KUNCAN F, Tekin R, KAYA Y (2022). Döküman dili tanıma için içerik bağımsız yeni bir yaklaşım: Açı Örüntüler. Gazi Üniversitesi Mühendislik Mimarlık Fakültesi Dergisi, 37(3), 1277 - 1292. 10.17341/gazimmfd.844700 |
Chicago | NOYAN Tuba,KUNCAN Fatma,Tekin Ramazan,KAYA YILMAZ Döküman dili tanıma için içerik bağımsız yeni bir yaklaşım: Açı Örüntüler. Gazi Üniversitesi Mühendislik Mimarlık Fakültesi Dergisi 37, no.3 (2022): 1277 - 1292. 10.17341/gazimmfd.844700 |
MLA | NOYAN Tuba,KUNCAN Fatma,Tekin Ramazan,KAYA YILMAZ Döküman dili tanıma için içerik bağımsız yeni bir yaklaşım: Açı Örüntüler. Gazi Üniversitesi Mühendislik Mimarlık Fakültesi Dergisi, vol.37, no.3, 2022, ss.1277 - 1292. 10.17341/gazimmfd.844700 |
AMA | NOYAN T,KUNCAN F,Tekin R,KAYA Y Döküman dili tanıma için içerik bağımsız yeni bir yaklaşım: Açı Örüntüler. Gazi Üniversitesi Mühendislik Mimarlık Fakültesi Dergisi. 2022; 37(3): 1277 - 1292. 10.17341/gazimmfd.844700 |
Vancouver | NOYAN T,KUNCAN F,Tekin R,KAYA Y Döküman dili tanıma için içerik bağımsız yeni bir yaklaşım: Açı Örüntüler. Gazi Üniversitesi Mühendislik Mimarlık Fakültesi Dergisi. 2022; 37(3): 1277 - 1292. 10.17341/gazimmfd.844700 |
IEEE | NOYAN T,KUNCAN F,Tekin R,KAYA Y "Döküman dili tanıma için içerik bağımsız yeni bir yaklaşım: Açı Örüntüler." Gazi Üniversitesi Mühendislik Mimarlık Fakültesi Dergisi, 37, ss.1277 - 1292, 2022. 10.17341/gazimmfd.844700 |
ISNAD | NOYAN, Tuba vd. "Döküman dili tanıma için içerik bağımsız yeni bir yaklaşım: Açı Örüntüler". Gazi Üniversitesi Mühendislik Mimarlık Fakültesi Dergisi 37/3 (2022), 1277-1292. https://doi.org/10.17341/gazimmfd.844700 |