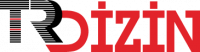
Doğrusal genelleştirilmiş ağırlıklı n-den k-çıkışlı F sistemin güvenilirlik analizi
Yıl: 2021 Cilt: 14 Sayı: 1 Sayfa Aralığı: 1 - 13 Metin Dili: Türkçe İndeks Tarihi: 30-05-2022
Doğrusal genelleştirilmiş ağırlıklı n-den k-çıkışlı F sistemin güvenilirlik analizi
Öz: Belirli sayıdaki bileşenlerin paralel bağlanmasıyla oluşan yapı modül olarak adlandırılır. N tane modülün
dairesel veya doğrusal sıralanmasıyla genelleştirilmiş n-den k-çıkışlı sistem elde edilir. Bu çalışmada,
doğrusal genelleştirilmiş ağırlıklı n-den k-çıkışlı F sistem önerilmiştir. Bu sistemin güvenilirliği ve sistem
imzası elde edilmiştir. Ağırlıklı sistemlerde her bir bileşenin sisteme katkısı farklı olduğundan bu katkı
bileşen ağırlığı olarak ifade edilir. Sistemdeki her bir bileşenin kendine ait ağırlığı ve çalışma olasılığı vardır.
Modülü oluşturan bileşenlerin toplam ağırlığına modül ağırlığı denir. Ardışık arızalı modüllerin toplam
ağırlığı en az k olduğunda veya sistemdeki arızalı bileşenlerin toplam ağırlığı en az τ olduğunda doğrusal
genelleştirilmiş ağırlıklı n-den k-çıkışlı F sistem arızalanır.
Anahtar Kelime: Reliability analysis of linear generalized weighted k-out-of-n F system
Öz: The structure formed by connecting a certain number of components in parallel is called a module. A
generalized k-out-of-n F system consists of a sequence of N ordered modules in a line or circle. In this study,
linear generalized weighted k-out-of-n F system is proposed. The reliability and system signature of this system
have been obtained. Since the coefficient of each component is different in weighted systems, this additive is
expressed as component weight. Each component in the system has its own weight and working probability.
The total weight of all components in the module is called the module weight. The linear generalized weighted
k-out-of-n F system fails when the total weight of consecutive failed modules is at least k or the total weight of
the failed components in the system is at least τ.
Anahtar Kelime: Belge Türü: Makale Makale Türü: Araştırma Makalesi Erişim Türü: Erişime Açık
- [1] Guolo, C. Varin. (2014). Beta Regression For Time Series Analysis Of Bounded Data, With Application To Canada Google R Flu Trends, The Annals of Applied Statistics, Institute of Mathematical Statistics, Vol. 8, No. 1, 74–88, Doi: 10.1214/13-AOAS684
- [2] S. Arasu, M. Jeevananthan, N. Thamaraiselvan, B. Janarthanan. (2014). Performances of data mining techniques in forecasting stock index – evidence from India and US, J.Natn.Sci.Foundation Sri Lanka 42 (2): 177–191, DOI: http://dx.doi.org/10.4038/jnsfsr.v42i2.6989
- [3] Friedman, J. (1991). Multivariate Adaptive Regression Splines. The Annals of Statistics, 19(1), 1-67. Retrieved April 7, 2021, from http://www.jstor.org/stable/2241837
- [4] J. R. Leathwick, D. Rowe, J. Richardson, J. Elith, T. Hastie. (2005). Using multivariate adaptive regression splines to predict the distributions of New Zealand’s freshwater diadromous fish, Freshwater Biology 50, 2034–2052, doi:10.1111/j.1365-2427.2005.01448.x
- [5] M. M. Al-Idrisi. (1991). Use of Regression and Triple Exponential Smoothing Models for Forecasting Share Prices of Saudi Companies, JKAU: Econ. & Adm. vol. 4, pp. 3-25 (1411 A.H. / 1991 A.D.)
- [6] M. C. A. Neto, G. Tavares, V. M. O. Alves, G. D. C. Cavalcanti, T. I. Ren. (2010). Improving financial time series prediction using exogenous series and neural networks committees, The 2010 International Joint Conference on Neural Networks (IJCNN), Barcelona, 2010, pp. 1-8., doi: 10.1109/IJCNN.2010.5596911
- [7] Gelper, S., Fried, R. and Croux, C. (2010), Robust forecasting with exponential and Holt–Winters smoothing. J. Forecast., 29: 285-300. https://doi.org/10.1002/for.1125
- [8] McKenzie, E. (n.d.). General exponential smoothing and the equivalent arma process. Journal of Forecasting, 3(3), 333–344. https://doi.org/10.1002/for.3980030312
- [9] O. Kisi, K. S. Parmar. (2016) Application of least square support vector machine and multivariate adaptive regression spline models in long term prediction of river water pollution. J Hydrol 534:104–112]
- [10] O. Hamidi, L. Tapak, H. Abbasi, Z. Maryanaji. (2017). Application of random forest time series, support vector regression and multivariate adaptive regression splines models in prediction of snowfall (a case study of Alvand in the middle Zagros, Iran). Theoretical and Applied Climatology. 134. 1-8. 10.1007/s00704-017- 2300-9.
- [11] L. J. Kao, C. C. Chiu. (2020). Application of integrated recurrent neural network with multivariate adaptive regression splines on SPC-EPC process, Journal of Manufacturing Systems, Vol. 57, Pages 109-118, ISSN 0278-6125, doi.org/10.1016/j.jmsy.2020.07.020.
- [12] Grillenzoni, M. Fornaciari. (2019). On-line peak detection in medical time series with adaptive regression methods. Econometrics and Statistics, Vol. 10, Pages 134-150, ISSN 2452-3062, doi.org/10.1016/j.ecosta.2018.07.002.
- [13] S. Lee, S. Lee, M. Moon. (2020). Hybrid change point detection for time series via support vector regression and CUSUM method. Applied Soft Computing, Vol. 89, 106101, ISSN 1568-4946, doi.org/10.1016/j.asoc.2020.106101.
- [14] G. R. Mode, K. A. Hoque. (2020). Adversarial Examples in Deep Learning for Multivariate Time Series Regression. eprint=2009.11911, arXiv, cs. LG
- [15] Y. Okkaoglu, Y. Akdi, , E. Golveren, M. Yucel. (2020). Estimation and forecasting of PM10 air pollution in Ankara via time series and harmonic regressions. International Journal of Environmental Science and Technology. (doi:10.1007/s13762-020-02705-0)
- [16] S. Jiang. (2019)."Combining Deep Neural Networks and Classical Time Series Regression Models for Forecasting Patient Flows in Hong Kong," in IEEE Access, vol. 7, pp. 118965-118974, doi: 10.1109/ACCESS.2019.2936550.
- [17] I. Ilic, B. Gorgulu, M. Cevik, M. G. Baydogan. (2020). Explainable boosted linear regression for time series forecasting. eprint=2009.09110, arXiv, cs.LG
- [18] Hjort, Jan & Suomi, Juuso & Käyhkö, Jukka. (2011). Spatial prediction of urban–rural temperatures using statistical methods. Theoretical and Applied Climatology. 106. 139-152. 10.1007/s00704-011-0425-9.
- [14] K. K. Kamalja, 2017, Reliability computing method for generalized k-out-of-n system, Journal of Computational and Applied Mathematics, 323, 111–122.
- [15] C. Kan, 2018, A Note on circular m-consecutive-k-out-of-n: F systems, Trends and Perspectives in Linear Statistical Inference, M. Tez, D. Rosen, (eds.), Springer International Publishing, Cham, s. 131–142.
- [16] J. S. Wu, R. J. Chen, 1994, An Algorithm for computing the reliability of weighted-k-out-of-n systems, IEEE Transactions on Reliability, 43(2), 327–328.
- [17] Y. Higashiyama, 2001, A factored reliability formula for weighted-k-out-of-n system, Asia-Pacific Journal of Operational Research, 18(1), 61–66.
- [18] S. Eryilmaz, G. Y. Tutuncu, 2009, Reliability evaluation of linear consecutive-weighted-k-out-of-n:F system, Asia-Pacific Journal of Operational Research, 26(6), 805–816.
- [19] F. Özbey, G. Gökdere, 2021, Analysis of linear consecutive-2-out-of-n: F repairable system with different failure rate, Bitlis Eren Üniversitesi Fen Bilimleri Dergisi, 10(1), 91–99.
- [20] F. J. Samaniego, 1985, On closure of the IFR class under formation of coherent systems, IEEE Transactions on Reliability, 34(1), 69–72.
- [21] S. Eryilmaz, 2010, Review of recent advances in reliability of consecutive k-out-of-n and related systems, Proceedings of the Institution of Mechanical Engineers, Part O: Journal of Risk and Reliability, 224(3), 225–237.
- [22] S. Eryilmaz, A. Tuncel, 2015, Computing the signature of a generalized k-out-of-n system, IEEE Transactions on Reliability, 64(2), 766–771.
- [23] P. J. Boland, 2001, Signatures of indirect majority systems, Journal of Applied Probability, 38(2), 597–603.
APA | Özbey F, Gökdere G (2021). Doğrusal genelleştirilmiş ağırlıklı n-den k-çıkışlı F sistemin güvenilirlik analizi. , 1 - 13. |
Chicago | Özbey Fahrettin,Gökdere Gökhan Doğrusal genelleştirilmiş ağırlıklı n-den k-çıkışlı F sistemin güvenilirlik analizi. (2021): 1 - 13. |
MLA | Özbey Fahrettin,Gökdere Gökhan Doğrusal genelleştirilmiş ağırlıklı n-den k-çıkışlı F sistemin güvenilirlik analizi. , 2021, ss.1 - 13. |
AMA | Özbey F,Gökdere G Doğrusal genelleştirilmiş ağırlıklı n-den k-çıkışlı F sistemin güvenilirlik analizi. . 2021; 1 - 13. |
Vancouver | Özbey F,Gökdere G Doğrusal genelleştirilmiş ağırlıklı n-den k-çıkışlı F sistemin güvenilirlik analizi. . 2021; 1 - 13. |
IEEE | Özbey F,Gökdere G "Doğrusal genelleştirilmiş ağırlıklı n-den k-çıkışlı F sistemin güvenilirlik analizi." , ss.1 - 13, 2021. |
ISNAD | Özbey, Fahrettin - Gökdere, Gökhan. "Doğrusal genelleştirilmiş ağırlıklı n-den k-çıkışlı F sistemin güvenilirlik analizi". (2021), 1-13. |
APA | Özbey F, Gökdere G (2021). Doğrusal genelleştirilmiş ağırlıklı n-den k-çıkışlı F sistemin güvenilirlik analizi. İstatistikçiler Dergisi: İstatistik ve Aktüerya, 14(1), 1 - 13. |
Chicago | Özbey Fahrettin,Gökdere Gökhan Doğrusal genelleştirilmiş ağırlıklı n-den k-çıkışlı F sistemin güvenilirlik analizi. İstatistikçiler Dergisi: İstatistik ve Aktüerya 14, no.1 (2021): 1 - 13. |
MLA | Özbey Fahrettin,Gökdere Gökhan Doğrusal genelleştirilmiş ağırlıklı n-den k-çıkışlı F sistemin güvenilirlik analizi. İstatistikçiler Dergisi: İstatistik ve Aktüerya, vol.14, no.1, 2021, ss.1 - 13. |
AMA | Özbey F,Gökdere G Doğrusal genelleştirilmiş ağırlıklı n-den k-çıkışlı F sistemin güvenilirlik analizi. İstatistikçiler Dergisi: İstatistik ve Aktüerya. 2021; 14(1): 1 - 13. |
Vancouver | Özbey F,Gökdere G Doğrusal genelleştirilmiş ağırlıklı n-den k-çıkışlı F sistemin güvenilirlik analizi. İstatistikçiler Dergisi: İstatistik ve Aktüerya. 2021; 14(1): 1 - 13. |
IEEE | Özbey F,Gökdere G "Doğrusal genelleştirilmiş ağırlıklı n-den k-çıkışlı F sistemin güvenilirlik analizi." İstatistikçiler Dergisi: İstatistik ve Aktüerya, 14, ss.1 - 13, 2021. |
ISNAD | Özbey, Fahrettin - Gökdere, Gökhan. "Doğrusal genelleştirilmiş ağırlıklı n-den k-çıkışlı F sistemin güvenilirlik analizi". İstatistikçiler Dergisi: İstatistik ve Aktüerya 14/1 (2021), 1-13. |