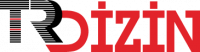
Diagnosing Diabetes with Machine Learning Techiques
Yıl: 2022 Cilt: 9 Sayı: 1 Sayfa Aralığı: 9 - 18 Metin Dili: İngilizce DOI: 10.17350/HJSE19030000250 İndeks Tarihi: 29-07-2022
Diagnosing Diabetes with Machine Learning Techiques
Öz: The rate of diabetes is rapidly increasing worldwide. Early detection of diabetes can help prevent or delay the onset of diabetes by initiating lifestyle changes and taking appropriate preventive measures. Until now, prediabetes and type 2 diabetes have proved to be early detection problems. There is a need for easy, rapid, and accurate diagnostic tools for the early diagnosis of diabetes in this context. Machine learning algorithms can help diagnose diseases early. Numerous studies are being conducted to improve the speed, performance, reliability, and accuracy of diagnosing with these methods for a particular disease. This study aims to predict whether a patient has diabetes based on diagnostic measurements in a dataset from the National Institute of Diabetes and Digestive and Kidney Diseases. Eight different variables belonging to the patients were selected as the input variable, and it was estimated whether the patient had diabetes or not.Of the 768 records examined, 500 (65.1%) were healthy, and 268 (34.9%) had diabetes. Ten different machine learning algorithms have been applied to predict diabetic status. The most successful method was the Random Forest algorithm with 90.1% accuracy.Accuracy percentages of other algorithms are also between 89% and 81%. This study describes a highly accurate machine learning prediction tool for finding patients with diabetes. The model identified in the study may be helpful for early diabetes diagnosis.
Anahtar Kelime: Belge Türü: Makale Makale Türü: Araştırma Makalesi Erişim Türü: Erişime Açık
- 1. K. G. M. M. Alberti, P. Zimmet, and J. Shaw, "International Diabetes Federation: A consensus on Type 2 diabetes prevention," Diabet. Med., vol. 24, no. 5, pp. 451–463, 2007, doi: 10.1111/j.1464-5491.2007.02157.x.
- 2. D. O. F. Diabetes, "Diagnosis and classification of diabetes mellitus," Diabetes Care, vol. 33, no. SUPPL. 1, 2010, doi: 10.2337/dc10-S062.
- 3. M. Franciosi et al., "Use of the Diabetes Risk Score for Opportunistic Screening of Undiagnosed Diabetes and Impaired Glucose Tolerance: The IGLOO (Impaired Glucose Tolerance and Long-Term Outcomes Observational) study," Diabetes Care, vol. 28, no. 5, pp. 1187–1194, May 2005, doi: 10.2337/diacare.28.5.1187.
- 4. Z. Tao, A. Shi, and J. Zhao, "Epidemiological Perspectives of Diabetes," Cell Biochem. Biophys., vol. 73, no. 1, pp. 181–185, Sep. 2015, doi: 10.1007/S12013-015-0598-4.
- 5. A. Mujumdar and V. Vaidehi, "Diabetes Prediction using Machine Learning Algorithms," Procedia Comput. Sci., vol. 165, pp. 292–299, 2019, doi: 10.1016/j.procs.2020.01.047.
- 6. P. Hossain, B. Kawar, and M. El Nahas, "Obesity and Diabetes in the Developing World — A Growing Challenge," N. Engl. J. Med., vol. 356, no. 3, pp. 213–215, 2007, doi: 10.1056/nejmp068177.
- 7. F. Mercaldo, V. Nardone, and A. Santone, "Diabetes Mellitus Affected Patients Classification and Diagnosis through Machine Learning Techniques," Procedia Comput. Sci., vol. 112, pp. 2519–2528, 2017, doi: 10.1016/j.procs.2017.08.193.
- 8. J. Tuomilehto et al., "Prevention of Type 2 Diabetes Mellitus by Changes in Lifestyle among Subjects with Impaired Glucose Tolerance," New England Journal of Medicine, vol. 344, no. 18. pp. 1343–1350, 2001, doi: 10.1056/nejm200105033441801.
- 9. J. L. Chiasson, R. G. Josse, R. Gomis, M. Hanefeld, A. Karasik, and M. Laakso, "Acarbose for prevention of type 2 diabetes mellitus: the STOP-NIDDM randomized trial," Lancet, vol. 359, no. 9323, pp. 2072–2077, Jun. 2002, doi: 10.1016/S0140-6736(02)08905-5.
- 10. A. Ramachandran, C. Snehalatha, S. Mary, B. Mukesh, A. D. Bhaskar, and V. Vijay, "The Indian Diabetes Prevention Programme shows that lifestyle modification and metformin prevent type 2 diabetes in Asian Indian subjects with impaired glucose tolerance (IDPP-1)," Diabetologia, vol. 49, no. 2, pp. 289–297, 2006, doi: 10.1007/s00125-005-0097-z.
- 11. T. Diyabet, V. Başkanı, and P. M. Temel, “DİYABET ORANI 10 YILDA YÜZDE 100 ARTTI,” pp. 10–12, 2017.
- 12. L. Parthiban and R. Subramanian, "Intelligent Heart Disease Prediction System using CANFIS and Genetic Algorithm," Int. J. Biol. Med. Sci., vol. 3, no. 3, pp. 157–160, 2008.
- 13. A. Iyer, J. S, and R. Sumbaly, "Diagnosis of Diabetes Using Classification Mining Techniques," Int. J. Data Min. Knowl. Manag. Process, vol. 5, no. 1, pp. 01–14, 2015, doi: 10.5121/ijdkp.2015.5101.
- 14. M. K. Hasan, M. A. Alam, D. Das, E. Hossain, and M. Hasan, "Diabetes prediction using ensembling of different machine learning classifiers," IEEE Access, vol. 8, pp. 76516–76531, 2020, doi: 10.1109/ACCESS.2020.2989857.
- 15. X. H. Meng, Y. X. Huang, D. P. Rao, Q. Zhang, and Q. Liu, "Comparison of three data mining models for predicting diabetes or prediabetes by risk factors," Kaohsiung J. Med. Sci., vol. 29, no. 2, pp. 93–99, 2013, doi: 10.1016/j.kjms.2012.08.016.
- 16. H. Lai, H. Huang, K. Keshavjee, A. Guergachi, and X. Gao, "Predictive models for diabetes mellitus using machine learning techniques," BMC Endocr. Disord., vol. 19, no. 1, pp. 1–9, 2019, doi: 10.1186/s12902-019-0436-6.
- 17. M. A. Sarwar, N. Kamal, W. Hamid, and M. A. Shah, "Prediction of diabetes using machine learning algorithms in healthcare," ICAC 2018 - 2018 24th IEEE Int. Conf. Autom. Comput. Improv. Product. through Autom. Comput., no. September, pp. 6–7, 2018, doi: 10.23919/IConAC.2018.8748992.
- 18. A. U. Haq et al., "Intelligent machine learning approach for effective recognition of diabetes in e-healthcare using clinical data," Sensors (Switzerland), vol. 20, no. 9, 2020, doi: 10.3390/s20092649.
- 19. M. F. Faruque, Asaduzzaman, and I. H. Sarker, "Performance Analysis of Machine Learning Techniques to Predict Diabetes Mellitus," 2nd Int. Conf. Electr. Comput. Commun. Eng. ECCE 2019, pp. 7–9, 2019, doi: 10.1109/ECACE.2019.8679365.
- 20. P. Sonar and K. Jaya Malini, "Diabetes prediction using different machine learning approaches," Proc. 3rd Int. Conf. Comput. Methodol. Commun. ICCMC 2019, no. Iccmc, pp. 367–371, 2019, doi: 10.1109/ICCMC.2019.8819841.
- 21. S. Wei, X. Zhao, and C. Miao, "A comprehensive exploration to the machine learning techniques for diabetes identification," IEEE World Forum Internet Things, WF-IoT 2018 - Proc., vol. 2018-Janua, pp. 291–295, 2018, doi: 10.1109/WF-IoT.2018.8355130.
- 22. H. Kaur and V. Kumari, "Predictive modelling and analytics for diabetes using a machine learning approach," Appl. Comput. Informatics, 2019, doi: 10.1016/j.aci.2018.12.004.
- 23. K. Sowjanya, A. Singhal, and C. Choudhary, "MobDBTest: A machine learning based system for predicting diabetes risk using mobile devices," Souvenir 2015 IEEE Int. Adv. Comput. Conf. IACC 2015, pp. 397–402, 2015, doi: 10.1109/IADCC.2015.7154738.
- 24. K. M. Orabi, Y. M. Kamal, and T. M. Rabah, "Early predictive system for diabetes mellitus disease," in Lecture Notes in Computer Science (including subseries Lecture Notes in Artificial Intelligence and Lecture Notes in Bioinformatics), 2016, vol. 9728, pp. 420–427, doi: 10.1007/978-3-319-41561-1_31.
- 25. N. Nai-Arun and R. Moungmai, "Comparison of Classifiers for the Risk of Diabetes Prediction," Procedia Comput. Sci., vol. 69, pp. 132–142, 2015, doi: 10.1016/j.procs.2015.10.014.
- 26. H. Kahramanli and N. Allahverdi, "Design of a hybrid system for the diabetes and heart diseases," Expert Syst. Appl., vol. 35, no. 1–2, pp. 82–89, 2008, doi: 10.1016/j.eswa.2007.06.004.
- 27. M. H. Zangooei, J. Habibi, and R. Alizadehsani, "Disease Diagnosis with a hybrid method SVR using NSGA-II," Neurocomputing, vol. 136, pp. 14–29, 2014, doi: 10.1016/j.neucom.2014.01.042.
- 28. Q. Zou, K. Qu, Y. Luo, D. Yin, Y. Ju, and H. Tang, "Predicting Diabetes Mellitus With Machine Learning Techniques," Front. Genet., vol. 9, no. November, pp. 1–10, 2018, doi: 10.3389/fgene.2018.00515.
- 29. V. . ACAR, E , ÖZERDEM, M , AKPOLAT, "Forecasting Diabetes Mellitus with Biometric Measurements.," Int. Arch. Med. Res., vol. 1, no. 1, pp. 28–42, 2011.
- 30. J. Tukey, "Exploratory data analysis," 1977, Accessed: Sep. 08, 2021. [Online]. Available: http://theta.edu.pl/wp-content/uploads/2012/10/exploratorydataanalysis_tukey.pdf.
- 31. R. S. Smith, J.W., Everhart, J.E., Dickson, W.C., Knowler, W.C., & Johannes, "Pima Indians Diabetes Database," Https://Www.Kaggle.Com/Uciml/Pima-Indians-Diabetes-Database, 2016. https://www.kaggle.com/uciml/pima-indians-diabetes-database (accessed Aug. 01, 2021).
- 32. "Tufte: The visual display of quantitative information - Google Akademik." https://scholar.google.com/scholar_lookup?title=The Visual Display of Quantitative Information&publication_year=2001&author=E. Tufte (accessed Sep. 08, 2021).
- 33. S. Lavalle, E. Lesser, R. Shockley, M. S. Hopkins, and N. Kruschwitz, "Big Data , Analytics and the Path From Insights to Value Big Data , Analytics and the Path From Insights to Value," no. 52205, 2011.
- 34. R. Agrawal, A. Kadadi, X. Dai, and F. Andres, "Challenges and opportunities with big data visualization," 7th Int. ACM Conf. Manag. Comput. Collect. Intell. Digit. Ecosyst. MEDES 2015, pp. 169–173, Oct. 2015, doi: 10.1145/2857218.2857256.
- 35. S. Nestorov, B. Jukić, N. Jukić, A. Sharma, and S. Rossi, "Generating insights through data preparation, visualization, and analysis: Framework for combining clustering and data visualization techniques for low-cardinality sequential data," Decis. Support Syst., vol. 125, no. March, p. 113119, 2019, doi: 10.1016/j.dss.2019.113119.
- 36. C. M. Salgado, C. Azevedo, H. Proença, and S. M. Vieira, Setting the Stage: Rationale Behind and Challenges to Health Data Analysis. 2016.
- 37. S. B. Kotsiantis and D. Kanellopoulos, "Data pre-processing for supervised leaning," Int. J. …, vol. 1, no. 2, pp. 1–7, 2006, doi: 10.1080/02331931003692557.
- 38. F. Nargesian, H. Samulowitz, U. Khurana, E. B. Khalil, and D. Turaga, "Learning feature engineering for classification," IJCAI Int. Jt. Conf. Artif. Intell., vol. 0, no. August, pp. 2529–2535, 2017, doi: 10.24963/ijcai.2017/352.
- 39. L. Breiman, "Random forests," Mach. Learn., vol. 45, no. 1, pp. 5–32, Oct. 2001, doi: 10.1023/A:1010933404324.
- 40. Ö. F. AKMEŞE, “Karın Ağrısı ile Acil Servise Başvuran Hastalarda Akut Apandisit Tanısı için Makine Öğrenmesi Yaklaşımlarının Kullanımı,” Kırıkkale University, 2020.
- 41. T. Chen and C. Guestrin, "XGBoost: A scalable tree boosting system," Proc. ACM SIGKDD Int. Conf. Knowl. Discov. Data Min., vol. 13-17-August-2016, pp. 785–794, Aug. 2016, doi: 10.1145/2939672.2939785.
- 42. J. Friedman, "Greedy Function Approximation : A Gradient Boosting Machine Author ( s ): Jerome H . Friedman Source : The Annals of Statistics , Vol . 29 , No . 5 ( Oct ., 2001 ), pp . 1189-1232 Published by : Institute of Mathematical Statistics Stable URL : http://www," Ann. Stat., vol. 29, no. 5, pp. 1189–1232, 2001.
- 43. W. Zhao, J. Li, J. Zhao, D. Zhao, J. Lu, and X. Wang, "XGB model: Research on evaporation duct height prediction based on XGBoost algorithm," Radioengineering, vol. 29, no. 1, pp. 81–93, 2020, doi: 10.13164/re.2020.0081.
- 44. G. Ke et al., "LightGBM: A Highly Efficient Gradient Boosting Decision Tree," Adv. Neural Inf. Process. Syst., vol. 30, 2017, Accessed: Nov. 28, 2021. [Online]. Available: https://github.com/Microsoft/LightGBM.
- 45. W. Cai, R. Wei, L. Xu, and X. Ding, "A method for modelling greenhouse temperature using gradient boost decision tree," Inf. Process. Agric., Sep. 2021, doi: 10.1016/J.INPA.2021.08.004.
- 46. M. Massaoudi, S. S. Refaat, I. Chihi, M. Trabelsi, F. S. Oueslati, and H. Abu-Rub, "A novel stacked generalization ensemble-based hybrid LGBM-XGB-MLP model for Short-Term Load Forecasting," Energy, vol. 214, p. 118874, Jan. 2021, doi: 10.1016/J.ENERGY.2020.118874.
- 47. K. S. Albayrak A., “VERİ MADENCİLİĞİ: KARAR AĞACI ALGORİTMALARI VE İMKB VERiLERİ ÜZERİNE BİR UYGULAMA * DATA MINING: DECISION TREE ALGORITHMS AND AN APPLICATION ON ISE DATA,” no. May, 2014.
- 48. R. E. Schapire, "Explaining AdaBoost," Empir. Inference Festschrift Honor Vladimir N. Vapnik, pp. 37–52, Jan. 2013, doi: 10.1007/978-3-642-41136-6_5.
- 49. T. K. An and M. H. Kim, "A new Diverse AdaBoost classifier," Proc. - Int. Conf. Artif. Intell. Comput. Intell. AICI 2010, vol. 1, pp. 359–363, 2010, doi: 10.1109/AICI.2010.82.
- 50. V. Vapnik, The Nature of Statistical Learning Theory. Springer science & business media, 2013.
- 51. AIZERMAN and M. A., "Theoretical Foundations of the Potential Function Method in Pattern Recognition Learning," Autom. Remote Control, vol. 25, pp. 821–837, 1964, Accessed: Nov. 27, 2021. [Online]. Available: https://ci.nii.ac.jp/naid/10021200712.
- 52. Boser Berhard E., G. I. M., and V. N. Vapnik, "A training algorithm for optimal margin classifiers," in In Proceedings of the 5th Annual ACM Workshop on Computational Learning Theory, 1992, pp. 144–152.
- 53. E. Ürük, “İstatistiksel Uygulamalarda Lojistik Regresyon Analizi,” Marmara University, 2007.
- 54. D. Soria, J. M. Garibaldi, F. Ambrogi, E. M. Biganzoli, and I. O. Ellis, "A 'non-parametric' version of the naive Bayes classifier," Knowledge-Based Syst., vol. 24, no. 6, pp. 775–784, Aug. 2011, doi: 10.1016/J.KNOSYS.2011.02.014.
- 55. "Naive Bayes Classifier in Machine Learning - Javatpoint." https://www.javatpoint.com/machine-learning-naive-bayes-classifier (accessed Nov. 29, 2021).
- 56. M. Hall, "A Decision Tree-Based Attribute Weighting Filter for Naive Bayes," Res. Dev. Intell. Syst. XXIII - Proc. AI 2006, 26th SGAI Int. Conf. Innov. Tech. Appl. Artif. Intell., pp. 59–70, Dec. 2006, doi: 10.1007/978-1-84628-663-6_5.
- 57. Gorunescu Florin, Data Mining: Concepts, Models and Techniques. Berlin: Springer Science & Business Media, 2011.
- 58. A. Dirican, “Tanı Testi̇ Performanslarının Değerlendi̇ri̇lmesi̇ ve Kıyaslanması,” Cerrahpaşa Tip Dergi̇si̇, vol. 32, no. 1, pp. 25–30, 2001.
- 59. I. Kavakiotis, O. Tsave, A. Salifoglou, N. Maglaveras, I. Vlahavas, and I. Chouvarda, "Machine Learning and Data Mining Methods in Diabetes Research," Comput. Struct. Biotechnol. J., vol. 15, pp. 104–116, 2017, doi: 10.1016/j.csbj.2016.12.005.
APA | AKMESE O (2022). Diagnosing Diabetes with Machine Learning Techiques. , 9 - 18. 10.17350/HJSE19030000250 |
Chicago | AKMESE OMER FARUK Diagnosing Diabetes with Machine Learning Techiques. (2022): 9 - 18. 10.17350/HJSE19030000250 |
MLA | AKMESE OMER FARUK Diagnosing Diabetes with Machine Learning Techiques. , 2022, ss.9 - 18. 10.17350/HJSE19030000250 |
AMA | AKMESE O Diagnosing Diabetes with Machine Learning Techiques. . 2022; 9 - 18. 10.17350/HJSE19030000250 |
Vancouver | AKMESE O Diagnosing Diabetes with Machine Learning Techiques. . 2022; 9 - 18. 10.17350/HJSE19030000250 |
IEEE | AKMESE O "Diagnosing Diabetes with Machine Learning Techiques." , ss.9 - 18, 2022. 10.17350/HJSE19030000250 |
ISNAD | AKMESE, OMER FARUK. "Diagnosing Diabetes with Machine Learning Techiques". (2022), 9-18. https://doi.org/10.17350/HJSE19030000250 |
APA | AKMESE O (2022). Diagnosing Diabetes with Machine Learning Techiques. Hittite Journal of Science and Engineering, 9(1), 9 - 18. 10.17350/HJSE19030000250 |
Chicago | AKMESE OMER FARUK Diagnosing Diabetes with Machine Learning Techiques. Hittite Journal of Science and Engineering 9, no.1 (2022): 9 - 18. 10.17350/HJSE19030000250 |
MLA | AKMESE OMER FARUK Diagnosing Diabetes with Machine Learning Techiques. Hittite Journal of Science and Engineering, vol.9, no.1, 2022, ss.9 - 18. 10.17350/HJSE19030000250 |
AMA | AKMESE O Diagnosing Diabetes with Machine Learning Techiques. Hittite Journal of Science and Engineering. 2022; 9(1): 9 - 18. 10.17350/HJSE19030000250 |
Vancouver | AKMESE O Diagnosing Diabetes with Machine Learning Techiques. Hittite Journal of Science and Engineering. 2022; 9(1): 9 - 18. 10.17350/HJSE19030000250 |
IEEE | AKMESE O "Diagnosing Diabetes with Machine Learning Techiques." Hittite Journal of Science and Engineering, 9, ss.9 - 18, 2022. 10.17350/HJSE19030000250 |
ISNAD | AKMESE, OMER FARUK. "Diagnosing Diabetes with Machine Learning Techiques". Hittite Journal of Science and Engineering 9/1 (2022), 9-18. https://doi.org/10.17350/HJSE19030000250 |