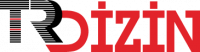
Supervised Learning-Aided Control of a DC-DC Power Converter in Wind Energy Conversion Systems
Yıl: 2021 Cilt: 33 Sayı: 0 Sayfa Aralığı: 47 - 56 Metin Dili: İngilizce DOI: 10.7240/jeps.897076 İndeks Tarihi: 29-07-2022
Supervised Learning-Aided Control of a DC-DC Power Converter in Wind Energy Conversion Systems
Öz: Over the last decades, to adopt high penetration of renewable energy sources (RESs) in electrical energy systems, distributed energy resources (DERs) have become prominent. Due to easy attainability status of small wind turbines (WTs), wind energy conversion systems (WECSs) are feasible applications for small customers, especially in windy areas. The next decade is likely to witness a considerable rise in DERs. In this context, WECSs are preferred broadly, thus harvesting wind energy into electrical energy effectively is a substantial issue. WTs can be got involved in the grid-connected or autonomous mode with a variety of topologies. In this paper, we examine to control of DC-DC boost converter of a WECS with the help of artificial intelligence (AI)-aided PI controller based on supervised learning method. Regarding the proposed method, artificial neural networks (ANNs) as a subset of AI are utilized. To test and ensure the applicability of the proposed control method, a small WECS with a permanent magnet synchronous generator (PMSG) connected a DC bus was implemented in MATLAB/Simulink environment. The proposed ANN scheme has reached a high accuracy rate with an overall mean squared error (MSE) equal to 7.4e-08. The results present that dynamic response and less complexity with a high accuracy rate have been obtained under study. The main target of this study is to reduce the number of sensors in the control layer. Thus, a cost-effective and more reliable structure is obtained with fewer sensor requirements.
Anahtar Kelime: Rüzgâr Enerjisi Dönüşüm Sistemlerinde bir DC-DC Güç Dönüştürücüsünün Denetimli Öğrenme Destekli Kontrolü
Öz: Son on yıllarda, elektrik enerjisi sistemlerinde yenilenebilir enerji kaynaklarının yüksek oranda nüfuzunu yaygınlaştırmak
için dağıtılmış enerji kaynakları öne çıkmıştır. Küçük rüzgâr türbinlerinin kolay erişilebilirlik durumu nedeniyle, rüzgâr
enerjisi dönüşüm sistemleri özellikle rüzgârlı alanlarda küçük müşteriler için elverişli uygulamalardır. Önümüzdeki on yıl
muhtemelen dağıtık enerji kaynakları önemli bir artışa tanık olacaktır. Bu bağlamda, rüzgâr enerji dönüşüm sistemleri yaygın
olarak tercih edilmektedir, bu nedenle rüzgâr enerjisinin elektrik enerjisine etkin bir şekilde dönüştürülmesi önemli bir
konudur. Rüzgâr türbinleri çeşitli topolojilerle şebekeye bağlı veya otonom modda dâhil edilebilirler. Bu makalede, denetimli
öğrenme yöntemine dayalı yapay zekâ destekli PI denetleyicisi yardımıyla bir rüzgâr enerji dönüşüm sistemindeki yükselten
DC-DC güç dönüştürücüsünün kontrolünü incelemekteyiz. Önerilen yöntemle ilgili olarak, yapay zekânın bir alt kümesi
olarak yapay sinir ağları kullanılmaktadır. Önerilen kontrol yönteminin uygulanabilirliğini test etmek ve doğrulamak için,
MATLAB/Simulink ortamında bir DC baraya sabit mıknatıslı senkron generatör ile küçük bir rüzgar enerji dönüşüm sistem
uygulanmıştır. Önerilen YSA şeması, 7.4e-08'e eşit toplam ortalama karesel hata (MSE) ile yüksek bir doğruluk oranına
ulaşmıştır. Sonuçlar, çalışma kapsamında dinamik yanıtın ve daha az karmaşıklığın yüksek doğruluk ile elde edildiğini
göstermektedir. Bu çalışmanın ana hedefi, kontrol katmanındaki sensör sayısını azaltmaktır. Böylece daha az sensör
gereksinimi ile uygun maliyetli ve daha güvenilir bir yapı elde edilmektedir.
Anahtar Kelime: Belge Türü: Makale Makale Türü: Araştırma Makalesi Erişim Türü: Erişime Açık
- 1] Kolar, J. W., Biela, J., Waffler S., Friedli, T. & Badstuebner, U., (2011). Performance trends and limitations of power electronic systems. 2010 6th International Conference on Integrated Power Electronics Systems, Nuremberg, pp. 1-20.
- [2] Melicio, R., Mendes, V. M. F. & Catalao, J. P. S., (2010). Power converter topologies for wind energy conversion systems: Integrated modeling, control strategy and performance simulation. Renewable Energy, 35 (10), pp. 2165-2174.
- [3] Hannan, M. A., et al. (2019). Power electronics contribution to renewable energy conversion addressing emission reduction: Applications, issues, and recommendations. Applied Energy, 251, p. 113404.
- [4] Soliman, M. A., Hasanien, H. M., Azazi, H. Z., El-Kholy, E. E., & Mahmoud, S. A., (2019). An Adaptive Fuzzy Logic Control Strategy for Performance Enhancement of a Grid- Connected PMSG-Based Wind Turbine. IEEE Transactions on Industrial Informatics, 15 (6), pp. 3163-3173.
- [5] Zhang, Y., Wang, Z., Wang, H., & Blaabjerg, F., (2020). Artificial Intelligence-Aided Thermal Model Considering Cross-Coupling Effects. IEEE Transactions on Power Electronics, 35 (10), pp. 9998-10002.
- [6] Bayhan, S., Demirbaş, S., & Abu-Rub, H., (2016). Fuzzy-PI-based sensorless frequency and voltage controller for doubly fed induction generator connected to a DC microgrid. IET Renewable Power Generation, 10 (8), pp. 1069-1077.
- [7] Mesbahi, A., Aljarhizi, Y., Hassoune, A., Khafallah, M., & Alibrahmi, E., (2020). Boost Converter implementation for Wind Generation System based on a variable speed PMSG," 2020 1st International Conference on Innovative Research in Applied Science, Engineering and Technology (IRASET), Meknes, Morocco, pp. 1-6.
- [8] Deng, X. et al. (2019). Sensorless effective wind speed estimation method based on unknown input disturbance observer and extreme learning machine. Energy, 186 (115790).
- [9] Wei, C., Zhang, Z., Qiao, W., & Qu, L., (2016). An Adaptive Network-Based Reinforcement Learning Method for MPPT Control of PMSG Wind Energy Conversion Systems. IEEE Transactions on Power Electronics, 31 (11), pp. 7837-7848.
- [10] Chatri, C., & Ouassaid, M., (2018). Sensorless Control of the PMSG in WECS Using Artificial Neural Network and Sliding Mode Observer. 2018 International Symposium on Advanced Electrical and Communication Technologies (ISAECT), Rabat, Morocco, pp. 1-6.
- [11] Jday, M., & Haggège, J., (2017). Modeling and neural networks based control of power converters associated with a wind turbine. 2017 International Conference on Green Energy Conversion Systems (GECS), Hammamet, Tunisia, pp. 1-7.
- [12] Adineh, B., Habibi, M. R., Akpolat, A. N., & Blaabjerg, F., (2021). Sensorless Voltage Estimation for Total Harmonic Distortion Calculation using Artificial Neural Networks in Microgrids. IEEE Transactions on Circuits and Systems II: Express Briefs, 68 (7), pp. 2583- 2587.
- [13] Akpolat, A. N., Dursun, E., & Kuzucuoğlu, A. E., (2020). AI-Aided Control of a Power Converter in Wind Energy Conversion System. 2020 Innovations in Intelligent Systems and Applications Conference (ASYU), Istanbul, Turkey, pp. 1-6, doi: 10.1109/ASYU50717.2020.9259877.
- [14] Samara, S., & Natsheh, E., (2019). Intelligent Real-Time Photovoltaic Panel Monitoring System Using Artificial Neural Networks. IEEE Access, 7, pp. 50287-50299.
- [15] Akpolat, A. N., Habibi, M. R., Dursun, E., Kuzucuoğlu, A. E., Yang, Y., Dragičević, T., & Blaabjerg, F., (2021). Sensorless Control of DC Microgrid Based on Artificial Intelligence. IEEE Transactions on Energy Conversion, 36 (3), pp. 2319-2329.
- [16] Egea-Àlvarez, A., Aragüés-Peñalba, M., Gomis-Bellmunt, O., Rull-Duran, J., & Sudrià- Andreu, A., (2016). Sensorless control of a power converter for a cluster of small wind turbines. IET Renewable Power Generation, 10 (5), pp. 721-728.
- [17] Teiar, H., Chaoui, H., & Sicard, P., (2015). Almost parameter-free sensorless control of PMSM. IECON 2015 - 41st Annual Conference of the IEEE Industrial Electronics Society, Yokohama, pp. 004667-004671.
- [18] Syskakis. T., & Ordonez, M., (2019). MPPT for Small Wind Turbines: Zero-Oscillation Sensorless Strategy. 2019 IEEE 10th International Symposium on Power Electronics for Distributed Generation Systems (PEDG), Xi'an, China, pp. 1060-1065.
- [19] Akpolat, A. N., Habibi, M. R., Baghaee, H. R. Dursun, E., Kuzucuoğlu, A. E., Yang, Y., Dragičević, T., & Blaabjerg, F., (2021). Dynamic Stabilization of DC Microgrids using ANN-Based Model Predictive Control. IEEE Transactions on Energy Conversion, Early Access, doi: 10.1109/TEC.2021.3118664.
- [20] Khan, M. A., Haque, A., Kurukuru, V. S. B., & Saad, M., (2020). Advanced Control Strategy with Voltage Sag Classification for Single- Phase Grid-Connected Photovoltaic System," IEEE Trans. Emerg. Sel. Topics Ind. Electron., Early Access, doi: 10.1109/JESTIE.2020.3041704.
- [21] Rosato, A., Panella, M., Araneo, R., & Andreotti, A., (2019). A Neural Network Based Prediction System of Distributed Generation for the Management of Microgrids. IEEE Trans. Ind. Appl., 55 (6), pp. 7092-7102.
- [22] Chaouachi, A., Kamel, R. M., Andoulsi, R., & Nagasaka, K., (2013). Multiobjective Intelligent Energy Management for a Microgrid. IEEE Trans. Ind. Electron., 60 (4), pp. 1688-1699.
- [23] Peyghami, S., Dragicevic, T., & F. Blaabjerg., (2021). Intelligent long-term performance analysis in power electronics systems. Sci. Rep., 11 (1), pp. 1–18.
- [24] Habibi, M. R., Baghaee, H. R., Blaabjerg, F., & Dragičević, T., (2021). Secure Control of DC Microgrids for Instant Detection and Mitigation of Cyber-Attacks Based on Artificial Intelligence. Early Access, IEEE Systems Journal, doi: 10.1109/JSYST.2021.3119355.
- [25] Farooq, Z., Zaman, T. M., Khan, A., Nasimullah, Muyeen, S. M., & Ibeas A., (2019). Artificial Neural Network Based Adaptive Control of Single Phase Dual Active Bridge With Finite Time Disturbance Compensation. IEEE Access, 7, pp. 112229- 112239.
- [26] Li, X., Zhang, X., Lin, F., & Blaabjerg, F., (2021). Artificial-intelligence based design (AI-D) for circuit parameters of power converters. IEEE Trans. Ind. Electron., Early Access, doi: 10.1109/TIE.2021.3088377.
- [27] Wang, T. He, X., & Deng, T., (2019). Neural networks for power management optimal strategy in hybrid microgrid. Neural Comput & Applic 31, pp. 2635–2647.
- [28] Onar, O. C., & Khaligh, A., (2015). Alternative Energy in Power Electronics. Chapter 2 - Energy Sources, 1st ed., UK: Butterworth- Heinemann, Elsevier, 2015, pp. 81-154.
- [29] Heier, S., (2014). Wind Energy Conversion System. Grid Integration of Wind Energy: Onshore and Offshore Conversion Systems, 3rd ed., Germany: John Wiley & Sons, pp. 31-117.
- [30] Akpolat, A.N., (2021). Management And Control of Distributed Energy Generation Systems via Artificial Intelligence Techniques. Doktora Tezi, Marmara Üniversitesi, Türkiye, pp. 37-38.
- [31] Piccinini, G., (2004). The first computational theory of mind and brain: A close look at McCulloch and Pitts’s Logical Calculus of Ideas Immanent in Nervous Activity. Synthese, 141, pp. 75–215.
- [32] Rathnayaka, R. M. K. T., & Seneviratna, D. M. K. N., (2019). A Novel Hybrid Back Propagation Neural Network Approach for Time Series Forecasting Under the Volatility. Communications in Computer and Information Science, Singapore.
APA | AKPOLAT A, Dursun E, Kuzucuoglu A (2021). Supervised Learning-Aided Control of a DC-DC Power Converter in Wind Energy Conversion Systems. , 47 - 56. 10.7240/jeps.897076 |
Chicago | AKPOLAT Alper Nabi,Dursun Erkan,Kuzucuoglu Ahmet Emin Supervised Learning-Aided Control of a DC-DC Power Converter in Wind Energy Conversion Systems. (2021): 47 - 56. 10.7240/jeps.897076 |
MLA | AKPOLAT Alper Nabi,Dursun Erkan,Kuzucuoglu Ahmet Emin Supervised Learning-Aided Control of a DC-DC Power Converter in Wind Energy Conversion Systems. , 2021, ss.47 - 56. 10.7240/jeps.897076 |
AMA | AKPOLAT A,Dursun E,Kuzucuoglu A Supervised Learning-Aided Control of a DC-DC Power Converter in Wind Energy Conversion Systems. . 2021; 47 - 56. 10.7240/jeps.897076 |
Vancouver | AKPOLAT A,Dursun E,Kuzucuoglu A Supervised Learning-Aided Control of a DC-DC Power Converter in Wind Energy Conversion Systems. . 2021; 47 - 56. 10.7240/jeps.897076 |
IEEE | AKPOLAT A,Dursun E,Kuzucuoglu A "Supervised Learning-Aided Control of a DC-DC Power Converter in Wind Energy Conversion Systems." , ss.47 - 56, 2021. 10.7240/jeps.897076 |
ISNAD | AKPOLAT, Alper Nabi vd. "Supervised Learning-Aided Control of a DC-DC Power Converter in Wind Energy Conversion Systems". (2021), 47-56. https://doi.org/10.7240/jeps.897076 |
APA | AKPOLAT A, Dursun E, Kuzucuoglu A (2021). Supervised Learning-Aided Control of a DC-DC Power Converter in Wind Energy Conversion Systems. International journal of advances in engineering and pure sciences (Online), 33(0), 47 - 56. 10.7240/jeps.897076 |
Chicago | AKPOLAT Alper Nabi,Dursun Erkan,Kuzucuoglu Ahmet Emin Supervised Learning-Aided Control of a DC-DC Power Converter in Wind Energy Conversion Systems. International journal of advances in engineering and pure sciences (Online) 33, no.0 (2021): 47 - 56. 10.7240/jeps.897076 |
MLA | AKPOLAT Alper Nabi,Dursun Erkan,Kuzucuoglu Ahmet Emin Supervised Learning-Aided Control of a DC-DC Power Converter in Wind Energy Conversion Systems. International journal of advances in engineering and pure sciences (Online), vol.33, no.0, 2021, ss.47 - 56. 10.7240/jeps.897076 |
AMA | AKPOLAT A,Dursun E,Kuzucuoglu A Supervised Learning-Aided Control of a DC-DC Power Converter in Wind Energy Conversion Systems. International journal of advances in engineering and pure sciences (Online). 2021; 33(0): 47 - 56. 10.7240/jeps.897076 |
Vancouver | AKPOLAT A,Dursun E,Kuzucuoglu A Supervised Learning-Aided Control of a DC-DC Power Converter in Wind Energy Conversion Systems. International journal of advances in engineering and pure sciences (Online). 2021; 33(0): 47 - 56. 10.7240/jeps.897076 |
IEEE | AKPOLAT A,Dursun E,Kuzucuoglu A "Supervised Learning-Aided Control of a DC-DC Power Converter in Wind Energy Conversion Systems." International journal of advances in engineering and pure sciences (Online), 33, ss.47 - 56, 2021. 10.7240/jeps.897076 |
ISNAD | AKPOLAT, Alper Nabi vd. "Supervised Learning-Aided Control of a DC-DC Power Converter in Wind Energy Conversion Systems". International journal of advances in engineering and pure sciences (Online) 33/0 (2021), 47-56. https://doi.org/10.7240/jeps.897076 |