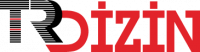
Artificial Intelligence Based Video Processing Methods for Predicting COVID-19: Observational Study
Yıl: 2022 Cilt: 14 Sayı: 1 Sayfa Aralığı: 22 - 31 Metin Dili: İngilizce DOI: 10.5336/biostatic.2022-88805 İndeks Tarihi: 09-06-2022
Artificial Intelligence Based Video Processing Methods for Predicting COVID-19: Observational Study
Öz: Objective: The aim of this study is to develop a
high-performance model and web-based clinical decision
making method to successfully distinguish and classify COVID19 from bacterial pneumonia, viral pneumonia and healthy
controls with lung ultrasound (LUS) videos using appropriate
video processing techniques and artificial intelligence (AI)
methods development of the support system. Material and
Methods: In this study, the open source LUS video dataset at
https://github.com/jannisborn/covid19_ultrasound was used. The
dataset includes 32 healthy controls, 24 COVID-19, 24 bacterial
pneumonia and 12 viral pneumonia class videos. In the video
processing stage, 300 image frames were taken from the videos in
each class. The images were divided into 80% (960) training and
20% (240) test datasets. In the modeling phase, the convolutional
neural network (CNN) method, one of the deep neural network
architectures in the keras library, was used. Accuracy, sensitivity,
specificity, precision, Matthews’ correlation coefficient and F1
score criteria are given to evaluate the performance of the model.
A web-based system has been developed that can successfully
detect COVID-19 using the, with the help of the AI-based model,
Python Flask Library. Results: The accuracy in the test dataset
was calculated as 93.39% for healthy control, COVID-19 and
viral pneumonia, and 95.07% for bacterial pneumonia.
Conclusion: According to the performance criteria values
obtained with the video processing-based CNN model, it can be
said that the developed system gives very successful predictions
in the diagnosis of COVID-19, bacterial pneumonia and viral
pneumonia.
Anahtar Kelime: COVID-19’u Tahmin Etmek için Yapay Zekâ Tabanlı Video İşleme Yöntemleri: Gözlemsel Çalışma
Öz: Amaç: Bu çalışmanın amacı, uygun video işleme teknikleri
ve yapay zekâ yöntemleri kullanılarak akciğer ultrason videoları ile
COVID19’u bakteriyel pnömoni, viral pnömoni ve sağlıklı
kontrollerden başarılı bir şekilde ayırt ederek sınıflandırmak için
yüksek performansa sahip bir modelin ve web tabanlı klinik karar
destek sisteminin geliştirilmesidir. Gereç ve Yöntemler: Bu
çalışmada, https://github.com/jannisborn/covid19_ultrasound
adresindeki açık kaynaklı akciğer ultrason video veri seti
kullanılmıştır. Veri setinde bulunan videoların 32’si sağlıklı
kontrol, 24’ü COVID-19, 24’ü bakteriyel pnömoni ve 12’si viral
pnömoni şeklinde klinik olarak sınıflandırılmıştır. Video işleme
aşamasında, her bir sınıftaki videolardan 300 görüntü karesi
alınmıştır. Görüntülerin %80’i (960) eğitim ve %20’si (240) test
veri seti olarak bölünmüştür. Modelleme aşamasında, keras
kütüphanesinde bulunan derin sinir ağları mimarilerinden evrişimli
sinir ağları CNN yöntemi kullanılmıştır. Oluşturulan modelin
performansını değerlendirmek için doğruluk, duyarlılık, seçicilik,
kesinlik, Matthews’in korelasyon katsayısı ve F1 skoru ölçütleri
verilmiştir. Bunlara ek olarak oluşturulan yapay zekâ tabanlı model
ile, Python Flask Kütüphanesi kullanılarak COVID-19’U başarılı
bir şekilde tespit edebilen web tabanlı bir sistem geliştirilmiştir.
Bulgular: Test veri setinde doğruluk sağlıklı kontrol, COVID-19
ve viral pnömoni için %93,39 ve bakteriyel pnömoni için ise
%95,07 olarak hesaplanmıştır. Sonuç: Önerilen video işleme
tabanlı CNN modeli ile elde edilen performans ölçütlerine göre
geliştirilen sistemin COVID-19, bakteriyel pnömoni ve viral
pnömoni tanısında oldukça başarılı tahminler verdiği ve klinik karar
destek amacıyla kullanılabileceği söylenebilir.
Anahtar Kelime: Belge Türü: Makale Makale Türü: Araştırma Makalesi Erişim Türü: Erişime Açık
- 1. Yağın FH, Güldoğan E, Ucuzal H, Çolak C. A Computer-assisted diagnosis tool for classifying COVID-19 based on chest X-Ray images. Konuralp Medical Journal. 2021;13(S1):438-45. [Crossref]
- 2. Muhammad G, Shamim Hossain M. COVID-19 and non-COVID-19 classification using multi-layers fusion from lung ultrasound images. Inf Fusion. 2021;72:80-8. [Crossref] [PubMed] [PMC]
- 3. Hassantabar S, Stefano N, Ghanakota V, Ferrari A, Nicola GN, Bruno R, et al. Coviddeep: Sars-cov-2/covid-19 test based on wearable medical sensors and efficient neural networks. arXiv preprint arXiv. 2020;200710497. [Crossref]
- 4. Ai T, Yang Z, Hou H, Zhan C, Chen C, Lv W, et al. Correlation of chest CT and RT-PCR testing for coronavirus disease 2019 (COVID-19) in China: A report of 1014 cases. Radiology. 2020;296(2):E32-E40. [Crossref] [PubMed] [PMC]
- 5. Chung M, Bernheim A, Mei X, Zhang N, Huang M, Zeng X, et al. CT imaging features of 2019 novel coronavirus (2019-nCoV). Radiology. 2020;295(1):202-7. [Crossref] [PubMed] [PMC]
- 6. Roy S, Menapace W, Oei S, Luijten B, Fini E, Saltori C, et al. Deep learning for classification and localization of COVID-19 markers in point-of-care lung ultrasound. IEEE Trans Med Imaging. 2020;39(8):2676-87. [Crossref] [PubMed]
- 7. Mahmud T, Rahman MA, Fattah SA. CovXNet: A multi-dilation convolutional neural network for automatic COVID-19 and other pneumonia detection from chest X-ray images with transferable multi-receptive feature optimization. Comput Biol Med. 2020;122:103869. [Crossref] [PubMed] [PMC]
- 8. Fan L, Li D, Xue H, Zhang L, Liu Z, Zhang B, et al. Progress and prospect on imaging diagnosis of COVID-19. Chin J Acad Radiol. 2020:1-10. [Crossref] [PubMed] [PMC]
- 9. Amatya Y, Rupp J, Russell FM, Saunders J, Bales B, House DR. Diagnostic use of lung ultrasound compared to chest radiograph for suspected pneumonia in a resource-limited setting. Int J Emerg Med. 2018;11(1):8. [Crossref] [PubMed] [PMC]
- 10. Born J, Wiedemann N, Brändle G, Buhre C, Rieck B, Borgwardt K. Accelerating covid-19 differential diagnosis with explainable ultrasound image analysis. arXiv preprint arXiv. 2020;200906116. [Crossref]
- 11. Yağın FH, Güldoğan E, Çolak C. A web-based software for the calculation of theoretical probability distributions. The Journal of Cognitive Systems. 2021;6(1):44-50. [Crossref]
- 12. Yağın B, Yağın FH, Gözükara Bag HG, Çolak C. A web-based software for reporting guidelines of scientific researches. The Journal of Cognitive Systems. 2021;6(1):39-43. [Crossref]
- 13. Yağın FH, Yağın B, Çolak C. An interactive web-based software for epidemiological research designs. Middle Black Sea Journal of Health Science. 2021;7(1):122-31. [Crossref]
- 14. Yılmaz R, Yağın FH. Early detection of coronary heart disease based on machine learning methods. Medical Records. 2022;4(1):1-6. [Link]
- 15. Yılmaz R, Yağın FH. A comparative study for the prediction of heart attack risk and associated factors using MLP and RBF neural networks. The Journal of Cognitive Systems. 2021;6(2):51-4. [Link]
- 16. Perçin İ, Yağın FH, Güldoğan E, Yoloğlu S. ARM: An interactive web software for association rules mining and an application in medicine. 2019 International Artificial Intelligence and Data Processing Symposium (IDAP); IEEE. 2019. [Crossref]
- 17. Perçin İ, Yağın FH, Arslan AK, Çolak C. An interactive web tool for classification problems based on machine learning algorithms using java programming language: data classification software. 2019 3rd International Symposium on Multidisciplinary Studies and Innovative Technologies (ISMSIT); IEEE. 2019. [Crossref]
- 18. Yagin FH, Kucukkakcali Z, Cicek IB, Gozukara Bag HG. The effects of variable selection and dimension reduction methods on the classification model in the small round blue cell tumor dataset. Middle Black Sea Journal of Health Science. 2021;7(3):390-6. [Crossref]
- 19. Yagin FH, Cicek IB, Kucukakcali Z. Classification of stroke with gradient boosting tree using smote-based oversampling method. Medicine. 2021;10(4):1510-5. [Crossref]
- 20. Kang Z, Li X, Zhou S. Recommendation of low-dose CT in the detection and management of COVID-2019. Eur Radiol. 2020;30(8):4356-7. [Crossref] [PubMed] [PMC]
- 21. Ahmad F, Farooq A, Ghani MU. Deep ensemble model for classification of novel coronavirus in chest X-ray images. Comput Intell Neurosci. 2021;2021:8890226. [Crossref] [PubMed] [PMC]
- 22. Ismael AM, Şengür A. Deep learning approaches for COVID-19 detection based on chest X-ray images. Expert Syst Appl. 2021;164:114054. [Crossref] [PubMed] [PMC]
- 23. Abbas A, Abdelsamea MM, Gaber MM. Classification of COVID-19 in chest X-ray images using DeTraC deep convolutional neural network. Appl Intell (Dordr). 2020:1-11. [Crossref] [PubMed] [PMC]
- 24. Hu Z, Liu Z, Dong Y, Liu J, Huang B, Liu A, et al. Evaluation of lung involvement in COVID-19 pneumonia based on ultrasound images. Biomed Eng Online. 2021;20(1):27. [Crossref] [PubMed] [PMC]
- 25. Born J, Brändle G, Cossio M, Disdier M, Goulet J, Roulin J, et al. POCOVID-Net: automatic detection of COVID-19 from a new lung ultrasound imaging dataset (POCUS). arXiv preprint arXiv. 2020;200412084. [Link]
APA | Yağın B, GÜLDOĞAN E, ÇOLAK C (2022). Artificial Intelligence Based Video Processing Methods for Predicting COVID-19: Observational Study. , 22 - 31. 10.5336/biostatic.2022-88805 |
Chicago | Yağın Burak,GÜLDOĞAN Emek,ÇOLAK Cemil Artificial Intelligence Based Video Processing Methods for Predicting COVID-19: Observational Study. (2022): 22 - 31. 10.5336/biostatic.2022-88805 |
MLA | Yağın Burak,GÜLDOĞAN Emek,ÇOLAK Cemil Artificial Intelligence Based Video Processing Methods for Predicting COVID-19: Observational Study. , 2022, ss.22 - 31. 10.5336/biostatic.2022-88805 |
AMA | Yağın B,GÜLDOĞAN E,ÇOLAK C Artificial Intelligence Based Video Processing Methods for Predicting COVID-19: Observational Study. . 2022; 22 - 31. 10.5336/biostatic.2022-88805 |
Vancouver | Yağın B,GÜLDOĞAN E,ÇOLAK C Artificial Intelligence Based Video Processing Methods for Predicting COVID-19: Observational Study. . 2022; 22 - 31. 10.5336/biostatic.2022-88805 |
IEEE | Yağın B,GÜLDOĞAN E,ÇOLAK C "Artificial Intelligence Based Video Processing Methods for Predicting COVID-19: Observational Study." , ss.22 - 31, 2022. 10.5336/biostatic.2022-88805 |
ISNAD | Yağın, Burak vd. "Artificial Intelligence Based Video Processing Methods for Predicting COVID-19: Observational Study". (2022), 22-31. https://doi.org/10.5336/biostatic.2022-88805 |
APA | Yağın B, GÜLDOĞAN E, ÇOLAK C (2022). Artificial Intelligence Based Video Processing Methods for Predicting COVID-19: Observational Study. Türkiye Klinikleri Biyoistatistik Dergisi, 14(1), 22 - 31. 10.5336/biostatic.2022-88805 |
Chicago | Yağın Burak,GÜLDOĞAN Emek,ÇOLAK Cemil Artificial Intelligence Based Video Processing Methods for Predicting COVID-19: Observational Study. Türkiye Klinikleri Biyoistatistik Dergisi 14, no.1 (2022): 22 - 31. 10.5336/biostatic.2022-88805 |
MLA | Yağın Burak,GÜLDOĞAN Emek,ÇOLAK Cemil Artificial Intelligence Based Video Processing Methods for Predicting COVID-19: Observational Study. Türkiye Klinikleri Biyoistatistik Dergisi, vol.14, no.1, 2022, ss.22 - 31. 10.5336/biostatic.2022-88805 |
AMA | Yağın B,GÜLDOĞAN E,ÇOLAK C Artificial Intelligence Based Video Processing Methods for Predicting COVID-19: Observational Study. Türkiye Klinikleri Biyoistatistik Dergisi. 2022; 14(1): 22 - 31. 10.5336/biostatic.2022-88805 |
Vancouver | Yağın B,GÜLDOĞAN E,ÇOLAK C Artificial Intelligence Based Video Processing Methods for Predicting COVID-19: Observational Study. Türkiye Klinikleri Biyoistatistik Dergisi. 2022; 14(1): 22 - 31. 10.5336/biostatic.2022-88805 |
IEEE | Yağın B,GÜLDOĞAN E,ÇOLAK C "Artificial Intelligence Based Video Processing Methods for Predicting COVID-19: Observational Study." Türkiye Klinikleri Biyoistatistik Dergisi, 14, ss.22 - 31, 2022. 10.5336/biostatic.2022-88805 |
ISNAD | Yağın, Burak vd. "Artificial Intelligence Based Video Processing Methods for Predicting COVID-19: Observational Study". Türkiye Klinikleri Biyoistatistik Dergisi 14/1 (2022), 22-31. https://doi.org/10.5336/biostatic.2022-88805 |