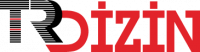
Prediction of Response to Neoadjuvant Chemoradiotherapy with Machine Learning in Rectal Cancer: A Pilot Study
Yıl: 2021 Cilt: 36 Sayı: 4 Sayfa Aralığı: 459 - 467 Metin Dili: İngilizce DOI: 10.5505/tjo.2021.2843 İndeks Tarihi: 15-06-2022
Prediction of Response to Neoadjuvant Chemoradiotherapy with Machine Learning in Rectal Cancer: A Pilot Study
Öz: OBJECTIVE
In locally advanced rectal cancer, trimodality therapy comprising chemoradiotherapy, total mesorectal
excision, and chemotherapy (CT) are accepted as standard treatment. However, standard “one-size-fitsall” therapy based on the TNM staging system may not be suitable for every patient. In cases with a good
response, less invasive surgical treatments, such as sphincter-sparing local excision or the watch-andwait approach may be more appropriate due to their lower recurrence rates. Therefore, it is very important to predict these cases and plan treatment accordingly to ensure effective personalized treatment.
Machine learning can successfully predict these cases. Aim: The aim of the study was to predict the
response to neoadjuvant chemoradiotherapy with machine learning in locally advanced rectal cancer.
METHODS
The study included 125 rectal cancer cases who underwent neoadjuvant radiotherapy (RT)±CT between
2010 and 2020, and the cases with a good response (grade 0-1) according to the Modified Ryan classification were predicted using machine learning. A total of 26 variables were evaluated. After determining
key variables, the dataset was divided into training/test sets at 80%/20%. Logistic regression, artificial
neural network-multilayer perceptron classifier, XGBoost, support vector classification, random forest, and Gaussian Naive Bayes algorithms used to establish a prediction model. In the prediction of the
group with a good response, 173 cases were created and evaluated with the synthetic minority oversampling technique method.
RESULTS
Of the 125 cases, 15 had a complete response and 33 had a good response (Modified Ryan grades 0 and
1). Six algorithms were tested in terms of their ability to predict a good response. Key variables for this
prediction were found to be tumor localization, RT break time, age, gender, Karnofsky Performance
Scale score, body mass index, pre- and post-treatment carcinoembryonic antigen levels, pre-treatment
hemoglobin and neutrophil-to-lymphocyte ratio and platelet-to-lymphocyte ratio, radiological T and N
stages, perineural and lymphatic invasion, tumor grade, radiological metastatic lymph node region, RT
dose and technique, and presence and scheme of concurrent CT. The algorithm that showed the best
performance was determined as logistic regression with an accuracy rate of 84% (CI: 0.69-0.98), sensitivity of 83%, and specificity of 85%.
CONCLUSION
It is very important to predict the cases with a good response and plan treatment accordingly to ensure
effective personalized treatment. Machine learning can successfully predict these cases.
Keywords: Artificial intelligence; machine learning; neoadjuvant chemoradiotherapy; prediction of treatment response; rectal cancer.
Anahtar Kelime: Belge Türü: Makale Makale Türü: Araştırma Makalesi Erişim Türü: Erişime Açık
- 1. National Comprehensive Cancer Network. NCCN Guidelines. Rectal Cancer, Version 1. Available at: National Comprehensive Cancer Network; 2021. Available from: http://www.nccn.org. Accessed Sep 1, 2021.
- 2. Meyer P, Noblet V, Mazzara C, Lallement A. Survey on deep learning for radiotherapy. Comput Biol Med 2018;98:126–46.
- 3. LeCun Y, Bengio Y, Hinton G. Deep learning. Nature 2015;521(7553):436–44.
- 4. Jarrett D, Stride E, Vallis K, Gooding MJ. Applications and limitations of machine learning in radiation oncology. Br J Radiol 2019;92(1100):20190001.
- 5. Bibault JE, Giraud P, Burgun A. Big data and machine learning in radiation oncology: State of the art and future prospects. Cancer Lett 2016;382(1):110–7.
- 6. Sauer R, Liersch T, Merkel S, Fietkau R, Hohenberger W, Hess C, et al. Preoperative versus postoperative chemoradiotherapy for locally advanced rectal cancer: Results of the German CAO/ARO/AIO-94 randomized phase III trial after a median follow-up of 11 years. J Clin Oncol 2012;30(16):1926–33.
- 7. Gérard JP, Azria D, Gourgou-Bourgade S, MartelLaffay I, Hennequin C, Etienne PL, et al. Comparison of two neoadjuvant chemoradiotherapy regimens for locally advanced rectal cancer: Results of the phase III trial ACCORD 12/0405-prodige 2. J Clin Oncol 2010;28(10):1638–44.
- 8. Borschitz T, Wachtlin D, Möhler M, Schmidberger H, Junginger T. Neoadjuvant chemoradiation and local excision for T2-3 rectal cancer. Ann Surg Oncol 2008;15(3):712–20.
- 9. Renehan AG, Malcomson L, Emsley R, Gollins S, Maw A, Myint AS, et al. Watch-and-wait approach versus surgical resection after chemoradiotherapy for patients with rectal cancer (the OnCoRe project): A propensity-score matched cohort analysis. Lancet Oncol 2016;17(2):174–83.
- 10.Ludwig KA. Sphincter-sparing resection for rectal cancer. Clin Colon Rectal Surg 2007;20(3):203–12.
- 11.Marijnen CA. Organ preservation in rectal cancer: Have all questions been answered? Lancet Oncol 2015;16(1):e13–22.
- 12.Weiser MR. AJCC 8th edition: Colorectal cancer. Ann Surg Oncol 2018;25(6):1454–5.
- 13.Ryan R, Gibbons D, Hyland JM, Treanor D, White A, Mulcahy HE, et al. Pathological response following long-course neoadjuvant chemoradiotherapy for locally advanced rectal cancer. Histopathology 2005;47(2):141–6.
- 14.Hastie T, Tibshirani R, Friedman J. The Elements of Statistical Learning: Data Mining, Inference, and Prediction. New York: Springer; 2009.
- 15.Chen T, Guestrin C. Xgboost: A Scalable Tree Boosting System. In: KDD 16: Proceedings of the 22nd ACM SIGKDD International Conference on Knowledge Discovery and Data Mining; 2016. p. 785–94.
- 16.Ben-Hur A, Horn D, Siegelmann HT, Vapnik V. Support vector clustering. J Mac Learn Res 2001;2:125–37.
- 17.Masetic Z, Subasi A. Congestive heart failure detection using random forest classifier. Comput Methods Programs Biomed 2016;130:54–64.
- 18.Jahromi AH, Taheri M. A non-parametric Mixture of Gaussian Naive Bayes Classifiers Based on Local Independent Features. In: 2017 Artificial Intelligence and Signal Processing Conference (AISP). IEEE; 2017. p. 209–12.
- 19.Celik O, Osmanoglu UO. Comparing to techniques used in customer churn analysis. J Multidiscip Res Dev 2019;4(1):30–8.
- 20.Galkin F, Aliper A, Putin E, Kuznetsov I, Gladyshev VN, Zhavoronkov A. Human microbiome aging clocks based on deep learning and tandem of permutation feature importance and accumulated local effects. EBioMedicine 2019;45:1–2.
- 21.Kohavi R. A Study of Cross-validation and Bootstrap for Accuracy Estimation and Model Selection. Proceedings of the 14th International Joint Conference on Artificial Intelligence. San Mateo, CA: Morgan Kaufmann; 1995. p. 1137–43.
- 22.Sun Y, Kamel MS, Wong AK, Wang Y. Cost-sensitive boosting for classification of imbalanced data. Pattern Recognit 2007;40(1):3358–78.
- 23.Han H, Wang WY, Mao BH. Borderline-SMOTE: A New Over-sampling Method in Imbalanced Data Sets Learning. In: Huang DS, Zhang XP, Huang GB, editors. Advances in Intelligent Computing ICIC 2005. Lecture Notes in Computer Science; Berlin: Springer, 2014. p. 878–87.
- 24.Sanghera P, Wong DW, McConkey CC, Geh JI, Hartley A. Chemoradiotherapy for rectal cancer: An updated analysis of factors affecting pathological response. Clin Oncol (R Coll Radiol) 2008;20(2):176–83.
- 25.Shayesteh SP, Alikhassi A, Esfahani AF, Miraie M, Geramifar P, Bitarafan-Rajabi A, et al. Neo-adjuvant chemoradiotherapy response prediction using MRI based ensemble learning method in rectal cancer patients. Phys Med 2019;62:111–9.
- 26.Cai D, Huang ZH, Yu HC, Wang XL, Bai LL, Tang GN, et al. Prognostic value of preoperative carcinoembryonic antigen/tumor size in rectal cancer. World J Gastroenterol 2019;25(33):4945–58.
- 27.Bacha D, Talbi G, Ben Slama S, Lahmar A, Gharbi L, Bouraoui S. Predictive factors for histological response to neo-adjuvant treatment in rectal cancers. Tunis Med 2019;97(11):1284–90.
- 28.Huang CM, Huang MY, Huang CW, Tsai HL, Su WC, Chang WC, et al. Machine learning for predicting pathological complete response in patients with locally advanced rectal cancer after neoadjuvant chemoradiotherapy. Sci Rep 2020;10:12555.
- 29.Shi L, Zhang Y, Nie K, Sun X, Niu T, Yue N, et al. Machine learning for prediction of chemoradiation therapy response in rectal cancer using pre-treatment and mid-radiation multi-parametric MRI. Magn Reson Imaging 2019;61:33–40.
- 30.Nie K, Shi L, Chen Q, Hu X, Jabbour SK, Yue N, et al. Rectal cancer: Assessment of neoadjuvant chemoradiation outcome based on radiomics of multiparametric MRI. Clin Cancer Res 2016;22(21):5256–64.
- 31.Fu J, Zhong X, Li N, Van Dams R, Lewis J, Sung K, et al. Deep learning-based radiomic features for improving neoadjuvant chemoradiation response prediction in locally advanced rectal cancer. Phys Med Biol 2020;65(7):075001.
- 32.Bibault JE, Giraud P, Housset M, Durdux C, Taieb J, Berger A, et al. Deep learning and radiomics predict complete response after neo-adjuvant chemoradiation for locally advanced rectal cancer. Sci Rep 2018;8(1):16914.
APA | Yakar M, Etiz D, BADAK B, Çelik Ö, KUTRI D, Ozen A, Yılmaz E (2021). Prediction of Response to Neoadjuvant Chemoradiotherapy with Machine Learning in Rectal Cancer: A Pilot Study. , 459 - 467. 10.5505/tjo.2021.2843 |
Chicago | Yakar Melek,Etiz Durmuş,BADAK Bartu,Çelik Özer,KUTRI DENIZ,Ozen Alaattin,Yılmaz Evrim Prediction of Response to Neoadjuvant Chemoradiotherapy with Machine Learning in Rectal Cancer: A Pilot Study. (2021): 459 - 467. 10.5505/tjo.2021.2843 |
MLA | Yakar Melek,Etiz Durmuş,BADAK Bartu,Çelik Özer,KUTRI DENIZ,Ozen Alaattin,Yılmaz Evrim Prediction of Response to Neoadjuvant Chemoradiotherapy with Machine Learning in Rectal Cancer: A Pilot Study. , 2021, ss.459 - 467. 10.5505/tjo.2021.2843 |
AMA | Yakar M,Etiz D,BADAK B,Çelik Ö,KUTRI D,Ozen A,Yılmaz E Prediction of Response to Neoadjuvant Chemoradiotherapy with Machine Learning in Rectal Cancer: A Pilot Study. . 2021; 459 - 467. 10.5505/tjo.2021.2843 |
Vancouver | Yakar M,Etiz D,BADAK B,Çelik Ö,KUTRI D,Ozen A,Yılmaz E Prediction of Response to Neoadjuvant Chemoradiotherapy with Machine Learning in Rectal Cancer: A Pilot Study. . 2021; 459 - 467. 10.5505/tjo.2021.2843 |
IEEE | Yakar M,Etiz D,BADAK B,Çelik Ö,KUTRI D,Ozen A,Yılmaz E "Prediction of Response to Neoadjuvant Chemoradiotherapy with Machine Learning in Rectal Cancer: A Pilot Study." , ss.459 - 467, 2021. 10.5505/tjo.2021.2843 |
ISNAD | Yakar, Melek vd. "Prediction of Response to Neoadjuvant Chemoradiotherapy with Machine Learning in Rectal Cancer: A Pilot Study". (2021), 459-467. https://doi.org/10.5505/tjo.2021.2843 |
APA | Yakar M, Etiz D, BADAK B, Çelik Ö, KUTRI D, Ozen A, Yılmaz E (2021). Prediction of Response to Neoadjuvant Chemoradiotherapy with Machine Learning in Rectal Cancer: A Pilot Study. Türk Onkoloji Dergisi, 36(4), 459 - 467. 10.5505/tjo.2021.2843 |
Chicago | Yakar Melek,Etiz Durmuş,BADAK Bartu,Çelik Özer,KUTRI DENIZ,Ozen Alaattin,Yılmaz Evrim Prediction of Response to Neoadjuvant Chemoradiotherapy with Machine Learning in Rectal Cancer: A Pilot Study. Türk Onkoloji Dergisi 36, no.4 (2021): 459 - 467. 10.5505/tjo.2021.2843 |
MLA | Yakar Melek,Etiz Durmuş,BADAK Bartu,Çelik Özer,KUTRI DENIZ,Ozen Alaattin,Yılmaz Evrim Prediction of Response to Neoadjuvant Chemoradiotherapy with Machine Learning in Rectal Cancer: A Pilot Study. Türk Onkoloji Dergisi, vol.36, no.4, 2021, ss.459 - 467. 10.5505/tjo.2021.2843 |
AMA | Yakar M,Etiz D,BADAK B,Çelik Ö,KUTRI D,Ozen A,Yılmaz E Prediction of Response to Neoadjuvant Chemoradiotherapy with Machine Learning in Rectal Cancer: A Pilot Study. Türk Onkoloji Dergisi. 2021; 36(4): 459 - 467. 10.5505/tjo.2021.2843 |
Vancouver | Yakar M,Etiz D,BADAK B,Çelik Ö,KUTRI D,Ozen A,Yılmaz E Prediction of Response to Neoadjuvant Chemoradiotherapy with Machine Learning in Rectal Cancer: A Pilot Study. Türk Onkoloji Dergisi. 2021; 36(4): 459 - 467. 10.5505/tjo.2021.2843 |
IEEE | Yakar M,Etiz D,BADAK B,Çelik Ö,KUTRI D,Ozen A,Yılmaz E "Prediction of Response to Neoadjuvant Chemoradiotherapy with Machine Learning in Rectal Cancer: A Pilot Study." Türk Onkoloji Dergisi, 36, ss.459 - 467, 2021. 10.5505/tjo.2021.2843 |
ISNAD | Yakar, Melek vd. "Prediction of Response to Neoadjuvant Chemoradiotherapy with Machine Learning in Rectal Cancer: A Pilot Study". Türk Onkoloji Dergisi 36/4 (2021), 459-467. https://doi.org/10.5505/tjo.2021.2843 |