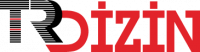
Medikal Görüntü İşlemede Derin Öğrenme Uygulamaları
Yıl: 2021 Cilt: 5 Sayı: 2 Sayfa Aralığı: 459 - 474 Metin Dili: Türkçe DOI: 10.26650/acin.927561 İndeks Tarihi: 16-06-2022
Medikal Görüntü İşlemede Derin Öğrenme Uygulamaları
Öz: Manyetik rezonans görüntüleme (MRI), bilgisayarlı tomografi (BT), pozitron emisyon tomografisi (PET),
mamografi, ultrason ve röntgen gibi tıbbi görüntüleme teknikleri uzun yıllardan beri hastalıkların teşhisi,
tanısı ve tedavisi için kullanılmıştır. Ancak hastalıkların daha erken teşhisi, uzmanların yoğunluğunu
azaltma, çakışan uzman görüşlerini karara bağlama gibi nedenlerle, bu alanda makine öğrenmesi
yöntemlerinden yararlanılmaktadır. Veri miktarının artması ile makine öğrenmesi yöntemleri görüntü
işleme alanında yetersiz kalmış, gelişen matematiksel modeller ve donanımsal cihazlar sayesinde derin
öğrenme bu alanda kendine geniş bir yer edinmiştir. Bu çalışmada derin öğrenme yöntemlerinin medikal
görüntü işleme alanında uygulanması incelenmiştir. Segmentasyon, sınıflandırma ve hastalık teşhisi,
görüntü oluşturma, dönüştürme ve iyileştirme alanlarında yapılan çalışmalardan oldukça güncel örnekler
sunulmuş, yapılan çalışmalarda kullanılan algoritmalar kısaca açıklanmıştır. Ayrıca BraTS 2020 veri seti
üzerinde derin öğrenme ile beyin tümör segmentasyonu gerçekleştirme denenmiş, sonuç olarak %86 dice
benzerlik oranı ve %80 hassasiyet değeri elde edilmiştir. Bu çalışmanın medikal görüntüler üzerinde derin
öğrenme yöntemleri ile yapılacak farklı çalışmalara yol gösterecek bir kaynak olması hedeflenmiştir.
Anahtar Kelime: Deep Learning Applications in Medical Image Processing
Öz: Medical imaging techniques such as magnetic resonance imaging (MRI), computed tomography (CT),
positron emission tomography (PET), mammography, ultrasound and x-ray have been used for the diagnosis
and treatment of diseases for many years. However, machine learning methods are used in this field for
reasons such as earlier diagnosis of diseases, reduce the workload of doctors, and adjudicate conflicting
expert opinions. With the increase in the amount of data, machine learning methods have remained
insufficient in the field of image processing. Thanks to the developing mathematical models and hardware
devices, deep learning has taken a wide place in this field. In this study, the application of deep learning
methods in the field of medical image processing has been examined. Very recent examples are presented
from studies in the fields of segmentation, classification and disease diagnosis, image generation, image
transformation and image enhancement. The algorithms used in the studies are briefly explained. In addition,
brain tumor segmentation with deep learning was tried on the BraTS 2020 dataset, and as a result, a dice
similarity rate of 86% and a sensitivity value of 80% were obtained. Our aim is for this study to guide
different studies on medical images with deep learning methods and serve as a basic resource in this field.
Anahtar Kelime: Belge Türü: Makale Makale Türü: Derleme Erişim Türü: Erişime Açık
- Alom, M. Z., Yakopcic, C., Hasan, M., Taha, T. M., & Asari, V. K. (2019). Recurrent residual U-Net for medical image segmentation. Journal of Medical Imaging, 6(1), 014006.
- Alyafeai, Z., & Ghouti, L. (2020). A fully-automated deep learning pipeline for cervical cancer classification. Expert Systems with Applications, 141, 112951.
- Angelov, P., & Almeida Soares, E. (2020). SARS-CoV-2 CT-scan dataset: A large dataset of real patients CT scans for SARS-CoV-2 identification. MedRxiv.
- Armanious, K., Jiang, C., Fischer, M., Küstner, T., Hepp, T., Nikolaou, K., ... & Yang, B. (2020). MedGAN: Medical image translation using GANs. Computerized Medical Imaging and Graphics, 79, 101684.
- Arvidsson, I., Overgaard, N. C., Marginean, F. E., Krzyzanowska, A., Bjartell, A., Åström, K., & Heyden, A. (2018, April). Generalization of prostate cancer classification for multiple sites using deep learning. In 2018 IEEE 15th International Symposium on Biomedical Imaging (ISBI 2018) (pp. 191-194). IEEE.
- Bakas, S., Reyes, M., Jakab, A., Bauer, S., Rempfler, M., Crimi, A., ... & Eaton-Rosen, Z. (2018). Identifying the best machine learning algorithms for brain tumor segmentation, progression assessment, and overall survival prediction in the BRATS challenge. arXiv preprint arXiv:1811.02629.
- Bera, S., & Biswas, P. K. (2021). Noise Conscious Training of Non Local Neural Network Powered by Self Attentive Spectral Normalized Markovian Patch GAN for Low Dose CT Denoising. IEEE Transactions on Medical Imaging.
- Bowyer, K., Kopans, D., Kegelmeyer, W. P., Moore, R., Sallam, M., Chang, K., & Woods, K. (1996, June). The digital database for screening mammography. In Third international workshop on digital mammography (Vol. 58, p. 27).
- Brosch, T., Tam, R., & Alzheimer’s Disease Neuroimaging Initiative. (2013, September). Manifold learning of brain MRIs by deep learning. In International Conference on Medical Image Computing and Computer-Assisted Intervention (pp. 633-640). Springer, Berlin, Heidelberg.
- Chu, C., Zhmoginov, A., & Sandler, M. (2017). Cyclegan, a master of steganography. arXiv preprint arXiv:1712.02950.
- Cirillo, M. D., Abramian, D., & Eklund, A. (2020). Vox2Vox: 3D-GAN for brain tumour segmentation. arXiv preprint arXiv:2003.13653.
- Clark, K., Vendt, B., Smith, K., Freymann, J., Kirby, J., Koppel, P., ... & Prior, F. (2013). The Cancer Imaging Archive (TCIA): maintaining and operating a public information repository. Journal of digital imaging, 26(6), 1045-1057.
- Çiçek, Ö., Abdulkadir, A., Lienkamp, S. S., Brox, T., & Ronneberger, O. (2016, October). 3D U-Net: learning dense volumetric segmentation from sparse annotation. In International conference on medical image computing and computer-assisted intervention (pp. 424-432). Springer, Cham.
- Díaz-Pernas, F. J., Martínez-Zarzuela, M., Antón-Rodríguez, M., & González-Ortega, D. (2021, February). A deep learning approach for brain tumor classification and segmentation using a multiscale convolutional neural network. In Healthcare (Vol. 9, No. 2, p. 153). Multidisciplinary Digital Publishing Institute.
- Dorj, U. O., Lee, K. K., Choi, J. Y., & Lee, M. (2018). The skin cancer classification using deep convolutional neural network. Multimedia Tools and Applications, 77(8), 9909-9924.
- El Kader Isselmou, A., Xu, G., Zhang, S., Saminu, S., & Javaid, I. (2019, July). Deep learning algorithm for brain tumor detection and analysis using MR brain images. In Proceedings of the 2019 International Conference on Intelligent Medicine and Health (pp. 28-32).
- Esteva, A., Kuprel, B., Novoa, R. A., Ko, J., Swetter, S. M., Blau, H. M., & Thrun, S. (2017). Dermatologist-level classification of skin cancer with deep neural networks. nature, 542(7639), 115-118.
- Farag, A., Lu, L., Roth, H. R., Liu, J., Turkbey, E., & Summers, R. M. (2017). Automatic pancreas segmentation using coarse-to-fine Superpixel labeling. In Deep learning and convolutional neural networks for medical image computing (pp. 279-302). Springer, Cham.
- Farooq, M., & Hafeez, A. (2020). Covid-resnet: A deep learning framework for screening of covid19 from radiographs. arXiv preprint arXiv:2003.14395.
- Gargeya, R., & Leng, T. (2017). Automated identification of diabetic retinopathy using deep learning. Ophthalmology, 124(7), 962-969.
- Gómez-Valverde, J. J., Antón, A., Fatti, G., Liefers, B., Herranz, A., Santos, A., ... & Ledesma-Carbayo, M. J. (2019). Automatic glaucoma classification using color fundus images based on convolutional neural networks and transfer learning. Biomedical optics express, 10(2), 892-913.
- Goodfellow, I., Pouget-Abadie, J., Mirza, M., Xu, B., Warde-Farley, D., Ozair, S., ... & Bengio, Y. (2014). Generative adversarial nets. Advances in neural information processing systems, 27.
- Gulshan, V., Peng, L., Coram, M., Stumpe, M. C., Wu, D., Narayanaswamy, A., ... & Webster, D. R. (2016). Development and validation of a deep learning algorithm for detection of diabetic retinopathy in retinal fundus photographs. Jama, 316(22), 2402-2410.
- Han, C., Hayashi, H., Rundo, L., Araki, R., Shimoda, W., Muramatsu, S., ... & Nakayama, H. (2018, April). GAN-based synthetic brain MR image generation. In 2018 IEEE 15th International Symposium on Biomedical Imaging (ISBI 2018) (pp. 734-738). IEEE.
- Hemdan, E. E. D., Shouman, M. A., & Karar, M. E. (2020). Covidx-net: A framework of deep learning classifiers to diagnose covid-19 in x-ray images. arXiv preprint arXiv:2003.11055.
- Heuvelmans, M. A., van Ooijen, P. M., Ather, S., Silva, C. F., Han, D., Heussel, C. P., ... & Oudkerk, M. (2021). Lung cancer prediction by Deep Learning to identify benign lung nodules. Lung cancer, 154, 1-4.
- Hu, K., Gan, Q., Zhang, Y., Deng, S., Xiao, F., Huang, W., ... & Gao, X. (2019). Brain tumor segmentation using multi-cascaded convolutional neural networks and conditional random field. IEEE Access, 7, 92615-92629.
- Hussain, E., Mahanta, L. B., Das, C. R., & Talukdar, R. K. (2020). A comprehensive study on the multi-class cervical cancer diagnostic prediction on pap smear images using a fusion-based decision from ensemble deep convolutional neural network. Tissue and Cell, 65, 101347.
- Hüseyin, Ü. Z. E. N., & HANBAY, K. Yaya özellik tanıma için LM filtre temelli derin evrişimsel sinir ağı. Politeknik Dergisi, 23(3), 605-613.
- Ibtehaz, N., & Rahman, M. S. (2020). MultiResUNet: Rethinking the U-Net architecture for multimodal biomedical image segmentation. Neural Networks, 121, 74-87.
- Isola, P., Zhu, J. Y., Zhou, T., & Efros, A. A. (2017). Image-to-image translation with conditional adversarial networks. In Proceedings of the IEEE conference on computer vision and pattern recognition (pp. 1125-1134).
- Jifara, W., Jiang, F., Rho, S., Cheng, M., & Liu, S. (2019). Medical image denoising using convolutional neural network: a residual learning approach. The Journal of Supercomputing, 75(2), 704-718.
- Jinnai, S., Yamazaki, N., Hirano, Y., Sugawara, Y., Ohe, Y., & Hamamoto, R. (2020). The development of a skin cancer classification system for pigmented skin lesions using deep learning. Biomolecules, 10(8), 1123.
- Jung, M. M., van den Berg, B., Postma, E., & Huijbers, W. (2018). Inferring PET from MRI with pix2pix. In Benelux Conference on Artificial Intelligence (Vol. 9).
- Khan, S., Islam, N., Jan, Z., Din, I. U., & Rodrigues, J. J. C. (2019). A novel deep learning based framework for the detection and classification of breast cancer using transfer learning. Pattern Recognition Letters, 125, 1-6.
- Krizhevsky, A., Sutskever, I., & Hinton, G. E. (2017). ImageNet classification with deep convolutional neural networks. Communications of the ACM, 60(6), 84-90.
- Lakshmanaprabu, S. K., Mohanty, S. N., Shankar, K., Arunkumar, N., & Ramirez, G. (2019). Optimal deep learning model for classification of lung cancer on CT images. Future Generation Computer Systems, 92, 374-382.
- Li, C., Zhang, J., Zhao, X., Kulwa, F., Li, Z., Xu, H., & Li, H. (2021). MRFU-Net: A Multiple Receptive Field U-Net for Environmental Microorganism Image Segmentation. In Information Technology in Biomedicine (pp. 27-40). Springer, Cham.
- Li, M., Shen, S., Gao, W., Hsu, W., & Cong, J. (2018). Computed tomography image enhancement using 3D convolutional neural network. In Deep Learning in Medical Image Analysis and Multimodal Learning for Clinical Decision Support (pp. 291-299). Springer, Cham.
- Li, W., Li, Y., Qin, W., Liang, X., Xu, J., Xiong, J., & Xie, Y. (2020). Magnetic resonance image (MRI) synthesis from brain computed tomography (CT) images based on deep learning methods for magnetic resonance (MR)-guided radiotherapy. Quantitative imaging in medicine and surgery, 10(6), 1223.
- Litjens, G., Kooi, T., Bejnordi, B. E., Setio, A. A. A., Ciompi, F., Ghafoorian, M., ... & Sánchez, C. I. (2017). A survey on deep learning in medical image analysis. Medical image analysis, 42, 60-88.
- Long, J., Shelhamer, E., & Darrell, T. (2015). Fully convolutional networks for semantic segmentation. In Proceedings of the IEEE conference on computer vision and pattern recognition (pp. 3431-3440).
- Menze, B. H., Jakab, A., Bauer, S., Kalpathy-Cramer, J., Farahani, K., Kirby, J., ... & Van Leemput, K. (2014). The multimodal brain tumor image segmentation benchmark (BRATS). IEEE transactions on medical imaging, 34(10), 1993-2024.
- Nawaz, M., Sewissy, A. A., & Soliman, T. H. A. (2018). Multi-class breast cancer classification using deep learning convolutional neural network. Int. J. Adv. Comput. Sci. Appl, 9(6), 316-332.
- Nema, S., Dudhane, A., Murala, S., & Naidu, S. (2020). RescueNet: An unpaired GAN for brain tumor segmentation. Biomedical Signal Processing and Control, 55, 101641.
- Nie, D., Trullo, R., Lian, J., Wang, L., Petitjean, C., Ruan, S., ... & Shen, D. (2018). Medical image synthesis with deep convolutional adversarial networks. IEEE Transactions on Biomedical Engineering, 65(12), 2720-2730.
- Nie, D., Wang, L., Gao, Y., & Shen, D. (2016, April). Fully convolutional networks for multi-modality isointense infant brain image segmentation. In 2016 IEEE 13Th international symposium on biomedical imaging (ISBI) (pp. 1342-1345). IEEE.
- Pan, X., Yang, D., Li, L., Liu, Z., Yang, H., Cao, Z., ... & Chen, Y. (2018). Cell detection in pathology and microscopy images with multi-scale fully convolutional neural networks. World Wide Web, 21(6), 1721-1743.
- Pervan, N., & Keleş, Y. (2019). Derin öğrenme yaklaşımları kullanarak Türkçe metinlerden anlamsal çıkarım yapma (Doctoral dissertation).
- Plis, S. M., Hjelm, D. R., Salakhutdinov, R., Allen, E. A., Bockholt, H. J., Long, J. D., ... & Calhoun, V. D. (2014). Deep learning for neuroimaging: a validation study. Frontiers in neuroscience, 8, 229.
- Qiao, Z., Qian, Z., Tang, H., Gong, G., Yin, Y., Huang, C., & Fan, W. (2020, December). CorGAN: Context aware Recurrent Generative Adversarial Network for Medical Image Generation. In 2020 IEEE International Conference on Bioinformatics and Biomedicine (BIBM) (pp. 1100-1103). IEEE.
- Qu, L., Zhang, Y., Wang, S., Yap, P. T., & Shen, D. (2020). Synthesized 7T MRI from 3T MRI via deep learning in spatial and wavelet domains. Medical image analysis, 62, 101663.
- Radford, A., Metz, L., & Chintala, S. (2015). Unsupervised representation learning with deep convolutional generative adversarial networks. arXiv preprint arXiv:1511.06434.
- Rajaraman, S., & Antani, S. (2020). Training deep learning algorithms with weakly labeled pneumonia chest X-ray data for COVID-19 detection. medRxiv.
- Raudonis, V., Paulauskaite-Taraseviciene, A., & Sutiene, K. (2021). Fast multi-focus fusion based on deep learning for early-stage embryo image enhancement. Sensors, 21(3), 863.
- Ronneberger, O., Fischer, P., & Brox, T. (2015, October). U-net: Convolutional networks for biomedical image segmentation. In International Conference on Medical image computing and computer-assisted intervention (pp. 234-241). Springer, Cham.
- Roy, S., Menapace, W., Oei, S., Luijten, B., Fini, E., Saltori, C., ... & Demi, L. (2020). Deep learning for classification and localization of COVID-19 markers in point-of-care lung ultrasound. IEEE transactions on medical imaging, 39(8), 2676-2687.
- Saiz, F. A., & Barandiaran, I. (2020). COVID-19 Detection in Chest X-ray Images using a Deep Learning Approach. Int. J. Interact. Multim. Artif. Intell., 6(2), 1-4.
- Suk, H. I., & Shen, D. (2013, September). Deep learning-based feature representation for AD/MCI classification. In International Conference on Medical Image Computing and Computer-Assisted Intervention (pp. 583-590). Springer, Berlin, Heidelberg.
- Suk, H. I., Lee, S. W., Shen, D., & Alzheimer’s Disease Neuroimaging Initiative. (2014). Hierarchical feature representation and multimodal fusion with deep learning for AD/MCI diagnosis. NeuroImage, 101, 569-582.
- Sun, J., Darbehani, F., Zaidi, M., & Wang, B. (2020, October). Saunet: Shape attentive u-net for interpretable medical image segmentation. In International Conference on Medical Image Computing and Computer-Assisted Intervention (pp. 797-806). Springer, Cham.
- Sun, J., Peng, Y., Guo, Y., & Li, D. (2021). Segmentation of the multimodal brain tumor image used the multi-pathway architecture method based on 3D FCN. Neurocomputing, 423, 34-45.
- Sun, W., Zheng, B., & Qian, W. (2016, March). Computer aided lung cancer diagnosis with deep learning algorithms. In Medical imaging 2016: computeraided diagnosis (Vol. 9785, p. 97850Z). International Society for Optics and Photonics.
- Tan, Z. (2019). Derin öğrenme yardımıyla araç sınıflandırma (Master’s thesis, Fırat Üniversitesi, Fen Bilimleri Enstitüsü).
- Tong, X., Wei, J., Sun, B., Su, S., Zuo, Z., & Wu, P. (2021). ASCU-Net: Attention Gate, Spatial and Channel Attention U-Net for Skin Lesion Segmentation. Diagnostics, 11(3), 501.
- Ucuzal, H., YAŞAR, Ş., & Çolak, C. (2019, October). Classification of brain tumor types by deep learning with convolutional neural network on magnetic resonance images using a developed web-based interface. In 2019 3rd International Symposium on Multidisciplinary Studies and Innovative Technologies (ISMSIT) (pp. 1-5). IEEE.
- Umut, K. A. Y. A., YILMAZ, A., & Dikmen, Y. (2019). Sağlık alanında kullanılan derin öğrenme yöntemleri. Avrupa Bilim ve Teknoloji Dergisi, (16), 792-808.
- Vaid, S., Kalantar, R., & Bhandari, M. (2020). Deep learning COVID-19 detection bias: accuracy through artificial intelligence. International Orthopaedics, 44, 1539-1542.
- Waghmare, V. K., & Kolekar, M. H. (2021). Brain Tumor Classification Using Deep Learning. In Internet of Things for Healthcare Technologies (pp. 155-175). Springer, Singapore.
- Wang, L., Lin, Z. Q., & Wong, A. (2020). Covid-net: A tailored deep convolutional neural network design for detection of covid-19 cases from chest x-ray images. Scientific Reports, 10(1), 1-12.
- Wang, S., Kang, B., Ma, J., Zeng, X., Xiao, M., Guo, J., ... & Xu, B. (2021). A deep learning algorithm using CT images to screen for Corona Virus Disease (COVID-19). European radiology, 1-9.
- Wang, X., Peng, Y., Lu, L., Lu, Z., Bagheri, M., & Summers, R. M. (2017). Chestx-ray8: Hospital-scale chest x-ray database and benchmarks on weaklysupervised classification and localization of common thorax diseases. In Proceedings of the IEEE conference on computer vision and pattern recognition (pp. 2097-2106).
- Weng, Y., Zhou, T., Li, Y., & Qiu, X. (2019). Nas-unet: Neural architecture search for medical image segmentation. IEEE Access, 7, 44247-44257.
- Wu, M., Yan, C., Liu, H., Liu, Q., & Yin, Y. (2018). Automatic classification of cervical cancer from cytological images by using convolutional neural network. Bioscience reports, 38(6), BSR20181769.
- Xia, X., Togneri, R., Sohel, F., & Huang, D. (2018). Auxiliary classifier generative adversarial network with soft labels in imbalanced acoustic event detection. IEEE Transactions on Multimedia, 21(6), 1359-1371.
- Yamashita, K., & Markov, K. (2020, June). Medical image enhancement using super resolution methods. In International Conference on Computational Science (pp. 496-508). Springer, Cham.
- Yang, S., Jiang, L., Cao, Z., Wang, L., Cao, J., Feng, R., ... & Shan, F. (2020). Deep learning for detecting corona virus disease 2019 (COVID-19) on highresolution computed tomography: a pilot study. Annals of Translational Medicine, 8(7).
- Yang, X., He, X., Zhao, J., Zhang, Y., Zhang, S., & Xie, P. (2020). COVID-CT-dataset: a CT scan dataset about COVID-19. arXiv preprint arXiv:2003.13865.
- Yao, S., Tan, J., Chen, Y., & Gu, Y. (2021). A weighted feature transfer gan for medical image synthesis. Machine Vision and Applications, 32(1), 1-11.
- Zheng, J., Lin, D., Gao, Z., Wang, S., He, M., & Fan, J. (2020). Deep learning assisted efficient AdaBoost algorithm for breast cancer detection and early diagnosis. IEEE Access, 8, 96946-96954.
- Zheng, Y., Liu, D., Georgescu, B., Xu, D., & Comaniciu, D. (2017). Deep learning based automatic segmentation of pathological kidney in CT: local versus global image context. In Deep learning and convolutional neural networks for medical image computing (pp. 241-255). Springer, Cham.
- Zhou, X., Takayama, R., Wang, S., Hara, T., & Fujita, H. (2017). Deep learning of the sectional appearances of 3D CT images for anatomical structure segmentation based on an FCN voting method. Medical physics, 44(10), 5221-5233.
- Zhou, Z., Siddiquee, M. M. R., Tajbakhsh, N., & Liang, J. (2018). Unet++: A nested u-net architecture for medical image segmentation. In Deep learning in medical image analysis and multimodal learning for clinical decision support (pp. 3-11). Springer, Cham.
APA | Eker A, DURU N (2021). Medikal Görüntü İşlemede Derin Öğrenme Uygulamaları. , 459 - 474. 10.26650/acin.927561 |
Chicago | Eker Ayse Gul,DURU NEVCIHAN Medikal Görüntü İşlemede Derin Öğrenme Uygulamaları. (2021): 459 - 474. 10.26650/acin.927561 |
MLA | Eker Ayse Gul,DURU NEVCIHAN Medikal Görüntü İşlemede Derin Öğrenme Uygulamaları. , 2021, ss.459 - 474. 10.26650/acin.927561 |
AMA | Eker A,DURU N Medikal Görüntü İşlemede Derin Öğrenme Uygulamaları. . 2021; 459 - 474. 10.26650/acin.927561 |
Vancouver | Eker A,DURU N Medikal Görüntü İşlemede Derin Öğrenme Uygulamaları. . 2021; 459 - 474. 10.26650/acin.927561 |
IEEE | Eker A,DURU N "Medikal Görüntü İşlemede Derin Öğrenme Uygulamaları." , ss.459 - 474, 2021. 10.26650/acin.927561 |
ISNAD | Eker, Ayse Gul - DURU, NEVCIHAN. "Medikal Görüntü İşlemede Derin Öğrenme Uygulamaları". (2021), 459-474. https://doi.org/10.26650/acin.927561 |
APA | Eker A, DURU N (2021). Medikal Görüntü İşlemede Derin Öğrenme Uygulamaları. Acta Infologica, 5(2), 459 - 474. 10.26650/acin.927561 |
Chicago | Eker Ayse Gul,DURU NEVCIHAN Medikal Görüntü İşlemede Derin Öğrenme Uygulamaları. Acta Infologica 5, no.2 (2021): 459 - 474. 10.26650/acin.927561 |
MLA | Eker Ayse Gul,DURU NEVCIHAN Medikal Görüntü İşlemede Derin Öğrenme Uygulamaları. Acta Infologica, vol.5, no.2, 2021, ss.459 - 474. 10.26650/acin.927561 |
AMA | Eker A,DURU N Medikal Görüntü İşlemede Derin Öğrenme Uygulamaları. Acta Infologica. 2021; 5(2): 459 - 474. 10.26650/acin.927561 |
Vancouver | Eker A,DURU N Medikal Görüntü İşlemede Derin Öğrenme Uygulamaları. Acta Infologica. 2021; 5(2): 459 - 474. 10.26650/acin.927561 |
IEEE | Eker A,DURU N "Medikal Görüntü İşlemede Derin Öğrenme Uygulamaları." Acta Infologica, 5, ss.459 - 474, 2021. 10.26650/acin.927561 |
ISNAD | Eker, Ayse Gul - DURU, NEVCIHAN. "Medikal Görüntü İşlemede Derin Öğrenme Uygulamaları". Acta Infologica 5/2 (2021), 459-474. https://doi.org/10.26650/acin.927561 |