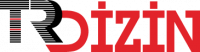
ALTERNATIVE ROBUST ESTIMATORS FOR PARAMETERS OF THE LINEAR REGRESSION MODEL
Yıl: 2022 Cilt: 10 Sayı: 1 Sayfa Aralığı: 35 - 58 Metin Dili: İngilizce DOI: 10.20290/estubtdb.887201 İndeks Tarihi: 20-06-2022
ALTERNATIVE ROBUST ESTIMATORS FOR PARAMETERS OF THE LINEAR REGRESSION MODEL
Öz: This paper considers parameter estimation of the linear regression model with Ramsay-Novick (RN) distributed errors, focusing on its use to aid robustness. Positioning within the class of heavy-tailed distributions, RN distribution can be defined as the modification of unbounded influence function of a non-robust density so that it has more resistance to outliers.
Potential use of this robust density has been assessed in Bayesian settings on real data examples and there is a lack of performance assessment for finite samples in the classical approach. Therefore, this study explores its robustness properties when used as error distribution compared to normal and other alternating heavy-tailed distributions like Laplace and Studentt. An extensive simulation study was conducted for this purpose under different settings of sample size, model parameters and outlier percentages. An efficient data generation of RN distribution through random-walk Metropolis algorithm is here also suggested. The results were supported by a real world application on famously known as Brownlee’s stack loss plant data.
Anahtar Kelime: Belge Türü: Makale Makale Türü: Araştırma Makalesi Erişim Türü: Erişime Açık
- Lange KL, Little RJA, Taylor JMG. Robust statistical modelling using the t distribution. J Americ Statist Assoc 1989; 84: 881-896.
- Shi J, Chen K, Song W. Robust errors-in-variables linear regression via Laplace distribution.Statist Prob Lett 2013; 84: 113-120.
- Lange K, Sinsheimer JS. Normal/Independent Distributions and Their Applications in Robust Regression. J Comput Graph Statist 1993; 2: 175-198.
- Albert J, Delampady M, Polasek W. A class of distributions for robustness studies. J Statist. Plann Inf 1991; 28: 291-304.
- Butler RJ, McDonald JB, Nelson RD, White SB. Robust and partially adaptive estimation of regression models. Rev. Econom. Statist. 1990; 72: 321-327.
- Taylor J, Verbyla A. Joint modelling of location and scale parameters of the t distribution. Stat. Model 2004; 4: 91-112.
- Bolfarine H, Arellano-Valle RB. Robust modelling in measurement error models using the tdistribution. REBRAPE 1994; 8: 67-84.
- Tiku ML, Islam MQ, Selcuk AS. Non-normal regression, II: Symmetric distributions. Comm Stat Theor Meth 2001; 30: 1021-1045.
- Alcantara IC, Cysneiros FJA. Linear regression models with slash-elliptical errors. Comput. Stat. Data Anal. 2013; 64: 153-164.
- Smith VK, Hall TH. A comparison of maximum likelihood versus BLUE estimators. Rev. Econ. Stat. 1972; 54: 186-190
- Ramsay JO, Novick MR. PLU robust Bayesian decision theory: point estimation. J Americ Statist Assoc 1980; 75: 901-907.
- West M. Outlier Models and Prior Distributions in Bayesian Linear Regression. J Royal Statist Soc 1984; 46: 431-439.
- Chen Y, Fournier D. Impacts of atypical data on Bayesian inference and robust Bayesian approach in fisheries. Can J Fish Aquat Sci 1999; 56: 1525-1533.
- Passarin K. Robust Bayesian Estimation. Varese: Italy, 2004.
- Kaya M, Çankaya E, Arslan O. Robust bayesian regression analysis using Ramsay-Novick distributed errors with Student-t prior. Commun Fac Sci Univ Ank Ser A1 Math Stat 2019; 68: 602-618.
- Draper NR, Smith H. Applied Regression Analysis. J Wiley New York 1996; 337: 204-215.
- Daniel C, Wood FS. Fitting Equations to Data. 2nd ed. New York, Wiley, 1971.
- Andrews DF. A robust method for multiple linear regression. Technometrics 1974; 16: 523-531.
- Denby L, Mallows CL. Two diagnostic displays for robust regression analysis. Technometrics1977; 19: 1-13.
- Andrews DF, Pregibon D. Finding the outliers that matter. J Roy Statist Soc B 1978; 40: 85-93.
- Cook RD. Influential observations in linear regression. J Americ Statist Assoc 1979; 74: 169-174.
- Dempster AP, Gasko-Green M. New tools for residuals analysis. Ann Statist. 1981; 9: 945-959.
- Atkinson AC. Regression diagnostics, transformations and constructed variables. J Roy StatistSoc B 1982a; 44: 1-36.
- Atkinson AC. Robust and diagnostic regression analyses. Comm Stat Theor Meth 1982b; 11:2559-2571.
- Rey WJJ. Introduction to Robust and Quasi-Robust Statistical Methods. Springer-Verlag Berlin, 1983.
- Carroll RJ, Ruppert D. Transformations in regression: A robust analysis. Technometrics 1985; 27: 1-12.
- Li G. Robust regression. In: David C, Hoaglin FM, John WT, editors. Exploring Data Tables, Trends, and Shapes. New York: J Wiley, 1985, pp. 281-343.
- Preece DA. Illustrative examples: illustrative of what?. Stat 1986; 33:35.
- R Development Core Team R. A language and environment for statistical computing. R Foundation for Statistical Computing, Vienna, Austria, 2013
- Rao CR. Unified theory of least squares. Comm in Statist 1973; 1:1-8.
- Zellner A. Bayesian and non-Bayesian analysis on the regression model with multivariate Student-t error terms. J Americ Statist Assoc, 1976; 71:400-405.
- Amemiya T. Advanced econometrics. Cambridge, Massachusetts, 1985.
- Geer S. Least squares estimators. Encyclop Statististics in the Behaviour Science Wiley, 2005.
- Rousseeuw PJ, Leroy AM. Robust Regression & Outlier Detection. J Wiley New York 1987; 76-78.
- Huber PJ. Robust statistics. J Wiley New York, 1981.
- Maronna RA, Yohai VJ. Asymptotic behavior of M-estimates for the linear model. Ann Statist 1979; 7: 258-268.
- Bloomfield P, Staiger W. Least Absolute Deviations Theory-Applications and Algorithms. Birkhauser Boston Mass, 1983.
- Yohai VJ. High breakdown point and high efficiency robust estimates for regression Univ. Washington, 1985.
- Metropolis N, Rosenbluth AW, Rosenbluth MN, Teller AH, Teller E. Equation of state calculations by fast computing machines. J Chem Phys 1953; 21: 1087-1092.
- Hastings WK. Monte Carlo sampling methods using Markov chains and their applications. Biometrika 1970; 57: 97-109.
- Roberts GO, Rosenthal JS. Optimal Scaling for Various Metropolis-Hastings Algorithms. Statist Scienc 2001; 16: 351–367.
- Kohavi R. A Study of Cross-Validation and Bootstrap for Accuracy Estimation and Model Selection. Appears in the International Joint Conference on Artificial Intelligence(IJCAI), 1995.
- Hastie T, Tibshirani R, Friedman J. The Elements of Statistical Learning. New York, NY, USA:1.10. Springer series in statistics, 2001.
- Arlot S, Celisse A. A survey of cross-validation procedures for model selection. Stat. Surv. 2010; 4: 40-79.
- James G, Witten D, Hastie T, Tibshirani R. An Introduction to Statistical Learning. Springer, 2013.
- Brownlee KA. Statistical Theory and Methodology in Science and Engineering. J Wiley New York, 1960. pp. 491-500.
- Dodge Y. The guinea pig of multiple regression. Robust Statistics, Data Analysis, and Computer Intensive Methods (Lecture Notes in Statistics) Springer-Verlag New York 1996; 109: 91-118
- Aitkin M, Wilson GT. Mixture models, outliers, and the EM algorithm. Technometrics 1980; 22: 325-331.
- Ruppert D, Carroll RJ. Trimmed least squares estimation in the linear model. J Americ Statist Assoc, 1980; 75: 828-838.
- Antoch J, Jureckova J. Trimmed least squares estimator resistant to leverage points. CSQ 1985; 4:329-339.
- Rey WJJ. M-Estimators in Robust Regression, a Case Study. M.B.L.E. Researc Lab Brussels, 1977.
- Birkes D, Dodge Y. Alternative Methods of Regression. J Wiley New York 1993; 177-179.
- Osborne MR. Finite Algorithms in Optimization and Data Analysis. J Wiley New York, 1985; 267-270.
- Hettmansperger TP, McKean JW. A robust alternative based on ranks to least squares in analyzing linear models. Technometrics 1977; 19: 275-284.
- Chambers RL, Heathcote CR. On the estimation of slope and the identification of outliers in linear regression. Biometrika 1981; 68: 21-33.
- Wiens DP. A note of the computation of robust, bounded influence estimates and test statistics in regression. Comput Statist Data Analy 1992; 13: 211-220.
- Tichavsky P. Algorithms for and geometrical characterization of solutions in the LMS and the LTS linear regression. CSQ 1991; 2: 139-151.
- Moberg TF, Ramberg JS, Randles RH. An adaptative multiple regression procedure based on Mestimators. Technometrics 1980; 22: 213-224.
- Welsh AH. The trimmed mean in the linear model. Ann Statist 1987; 15: 20-36
APA | ALTUNTAŞ M, CANKAYA E, Arslan O (2022). ALTERNATIVE ROBUST ESTIMATORS FOR PARAMETERS OF THE LINEAR REGRESSION MODEL . , 35 - 58. 10.20290/estubtdb.887201 |
Chicago | ALTUNTAŞ Mutlu,CANKAYA EMEL,Arslan Olcay ALTERNATIVE ROBUST ESTIMATORS FOR PARAMETERS OF THE LINEAR REGRESSION MODEL . (2022): 35 - 58. 10.20290/estubtdb.887201 |
MLA | ALTUNTAŞ Mutlu,CANKAYA EMEL,Arslan Olcay ALTERNATIVE ROBUST ESTIMATORS FOR PARAMETERS OF THE LINEAR REGRESSION MODEL . , 2022, ss.35 - 58. 10.20290/estubtdb.887201 |
AMA | ALTUNTAŞ M,CANKAYA E,Arslan O ALTERNATIVE ROBUST ESTIMATORS FOR PARAMETERS OF THE LINEAR REGRESSION MODEL . . 2022; 35 - 58. 10.20290/estubtdb.887201 |
Vancouver | ALTUNTAŞ M,CANKAYA E,Arslan O ALTERNATIVE ROBUST ESTIMATORS FOR PARAMETERS OF THE LINEAR REGRESSION MODEL . . 2022; 35 - 58. 10.20290/estubtdb.887201 |
IEEE | ALTUNTAŞ M,CANKAYA E,Arslan O "ALTERNATIVE ROBUST ESTIMATORS FOR PARAMETERS OF THE LINEAR REGRESSION MODEL ." , ss.35 - 58, 2022. 10.20290/estubtdb.887201 |
ISNAD | ALTUNTAŞ, Mutlu vd. "ALTERNATIVE ROBUST ESTIMATORS FOR PARAMETERS OF THE LINEAR REGRESSION MODEL ". (2022), 35-58. https://doi.org/10.20290/estubtdb.887201 |
APA | ALTUNTAŞ M, CANKAYA E, Arslan O (2022). ALTERNATIVE ROBUST ESTIMATORS FOR PARAMETERS OF THE LINEAR REGRESSION MODEL . Eskişehir Teknik Üniversitesi Bilim ve Teknoloji Dergisi b- Teorik Bilimler, 10(1), 35 - 58. 10.20290/estubtdb.887201 |
Chicago | ALTUNTAŞ Mutlu,CANKAYA EMEL,Arslan Olcay ALTERNATIVE ROBUST ESTIMATORS FOR PARAMETERS OF THE LINEAR REGRESSION MODEL . Eskişehir Teknik Üniversitesi Bilim ve Teknoloji Dergisi b- Teorik Bilimler 10, no.1 (2022): 35 - 58. 10.20290/estubtdb.887201 |
MLA | ALTUNTAŞ Mutlu,CANKAYA EMEL,Arslan Olcay ALTERNATIVE ROBUST ESTIMATORS FOR PARAMETERS OF THE LINEAR REGRESSION MODEL . Eskişehir Teknik Üniversitesi Bilim ve Teknoloji Dergisi b- Teorik Bilimler, vol.10, no.1, 2022, ss.35 - 58. 10.20290/estubtdb.887201 |
AMA | ALTUNTAŞ M,CANKAYA E,Arslan O ALTERNATIVE ROBUST ESTIMATORS FOR PARAMETERS OF THE LINEAR REGRESSION MODEL . Eskişehir Teknik Üniversitesi Bilim ve Teknoloji Dergisi b- Teorik Bilimler. 2022; 10(1): 35 - 58. 10.20290/estubtdb.887201 |
Vancouver | ALTUNTAŞ M,CANKAYA E,Arslan O ALTERNATIVE ROBUST ESTIMATORS FOR PARAMETERS OF THE LINEAR REGRESSION MODEL . Eskişehir Teknik Üniversitesi Bilim ve Teknoloji Dergisi b- Teorik Bilimler. 2022; 10(1): 35 - 58. 10.20290/estubtdb.887201 |
IEEE | ALTUNTAŞ M,CANKAYA E,Arslan O "ALTERNATIVE ROBUST ESTIMATORS FOR PARAMETERS OF THE LINEAR REGRESSION MODEL ." Eskişehir Teknik Üniversitesi Bilim ve Teknoloji Dergisi b- Teorik Bilimler, 10, ss.35 - 58, 2022. 10.20290/estubtdb.887201 |
ISNAD | ALTUNTAŞ, Mutlu vd. "ALTERNATIVE ROBUST ESTIMATORS FOR PARAMETERS OF THE LINEAR REGRESSION MODEL ". Eskişehir Teknik Üniversitesi Bilim ve Teknoloji Dergisi b- Teorik Bilimler 10/1 (2022), 35-58. https://doi.org/10.20290/estubtdb.887201 |