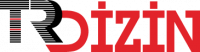
Adaptation of metaheuristic algorithms to improve training performance of an ESZSL model
Yıl: 2021 Cilt: 12 Sayı: 27 Sayfa Aralığı: 1781 - 1796 Metin Dili: İngilizce DOI: 10.3906/elk-2008-113 İndeks Tarihi: 23-06-2022
Adaptation of metaheuristic algorithms to improve training performance of an ESZSL model
Öz: Zero-shot learning (ZSL) is a recent promising learning approach that is similar to human vision systems.
ZSL essentially allows machines to categorize objects without requiring labeled training data. In principle, ZSL proposes
a novel recognition model by specifying merely the attributes of the category. Recently, several sophisticated approaches
have been introduced to address the challenges regarding this problem. Embarrassingly simple approach to zeroshot learning (ESZSL) is one of the critical of those approaches that basically proposes a simple but efficient linear
code solution. However, the performance of the ESZSL model mainly depends on parameter selection. Metaheuristic
algorithms are considered as one the most sophisticated computational intelligence paradigms that allows to approximate
optimization problems with high success. This paper addresses this problem by adapting leading metaheuristic algorithms
to automatically train the parameters of a linear ESZSL model. The model is statistically validated by performing a
series of experiments with benchmark datasets.
Anahtar Kelime: Are the Alarm Settings of the Monitors in Intensive Care Units Correct Enough?
Öz: Objective: Monitoring has vital importance in following intensive care patients. This study planned to determine whether the lower and upper limits of the alarm settings of the monitors actively used in a university hospital adult intensive care unit (ICU) were adjusted and to reveal the appropriateness of these limits. Methods: The lower and upper alarm limit settings of each monitor were photographed once. The photographs were evaluated by the anesthesiologist in the research team in terms of pulse rate, respiratory rate, systolic blood pressure, diastolic blood pressure (DBP), and oxygen saturation for lower and upper limits and appropriateness. Results: The lower and upper limits of heart rate settings were found to be adjusted in 100% of the monitors in ICUs of surgical and internal units, and the majority of them were within the appropriate value range. The alarm limits of 92% of the monitors in the ICUs of internal medicine units were found to be set, and 84% were appropriately adjusted. A total of 83 monitors in 10 ICUs were examined. The upper and lower limits of the respiratory rate were not set in any of the monitors examined. According to the results of the study, it was observed that the alarm settings of the DBP and respiratory parameters of intensive care patients had not been set. It was found that SpO2 lower limits for 3 monitors had been set in only one group of ICUs, but that none of them had been adjusted appropriately. Conclusion: Monitor alarm limits in ICUs are generally not set appropriately. According to these results, we recommend that all monitors in ICUs should be adjusted correctly to fit the clinic of the patient and lower and upper limits.
Anahtar Kelime: Yoğun Bakımlardaki Monitör Alarm Ayarları Yeterince Doğru Mu?
Öz: Amaç: Monitörizasyon, yoğun bakım (YB) hastasının takibinde hayati öneme sahiptir. Bu çalışma, bir üniversite hastanesi yetişkin YB ünitelerinde aktif olarak kullanılan monitörlerin alarm değerlerinin alt ve üst sınırlarının ayarlanıp ayarlanmadığının ve bu sınırların uygunluğunun saptanması amacıyla gerçekleştirilmiştir. Yöntemler: Her monitörün alt ve üst alarm sınır ayarları “bir kez” fotoğraflanmıştır. Fotoğraflar, araştırma ekibindeki anestezi uzmanı tarafından nabız sayısı, solunum sayısı, sistolik kan basıncı (SKB), diyastolik kan basıncı (DKB), oksijen satürasyonu “alt üst sınırları ve uygunluğu” açısından değerlendirilmiştir. Bulgular: Araştırmada, cerrahi ve dahili birimler YB ünitelerindeki monitörlerin %100’ünde nabız alt ve üst limitlerinin girildiği ve büyük çoğunluğunun uygun aralıkta olduğu saptanmıştır. Dahili birimler YB ünitelerindeki monitörlerin %92 oranında alarm limitlerinin girildiği; %84’ünün uygun olduğu belirlenmiştir. Diğer yandan; 10 YB ünitesindeki 83 monitör (veri toplama saatinde aktif kullanılan) incelenmiştir. İncelenen monitörlerin hiçbirinde solunum sayısı alt ve üst sınırları girilmemiştir. Araştırma sonucuna göre YB hastalarının DKB ve solunum parametrelerinin alarm ayarlarının yapılmadığı gözlenmiştir. Sadece bir grup YB birimlerinde 3 monitörün SpO2 alt sınırlarının girildiği ancak hiçbirinin uygun olmadığı saptanmıştır. Sonuç: YB ünitelerinde monitör alarm sınırları genellikle uygun ayarlanmamaktadır. Bu sonuçlara göre YB ünitelerinde tüm monitörlerin hastanın kliniği ve gereksinimine uygun alt ve üst sınır değerlerinin doğru ayarlanması önerilmektedir.
Anahtar Kelime: Belge Türü: Makale Makale Türü: Araştırma Makalesi Erişim Türü: Erişime Açık
- 1. Bahar M. Yoğun bakımda monitorizasyondan yararlanma. J Turk Soc Intens Care 2011;9:110-5. [Crossref]
- [1] Romera-Paredes B,Torr P. An embarrassingly simple approach to zero-shot learning. In: International Conference on Machine Learning; Lille, France; 2015. pp. 2152-2161.
- 2. Chambrin MC, Ravaux P, Calvelo-Aros D, Jaborska A, Chopin C, Boniface B. Multicentric study of monitoring alarms in the adult intensive care unit (ICU): A descriptive analysis. Intensive Care Med 1999;25(12):1360-6. [Crossref]
- [2] Li J, Jing M, Lu K, Ding Z, Zhu L et al. Leveraging the invariant side of generative zero-shot learning. In: Proceedings of the IEEE Conference on Computer Vision and Pattern Recognition; 2019. pp. 7402-7411.
- 3. Elnour S, Shankar-Hari M. The critically ill patient: Making referral to intensive care. Br J Hosp Med (Lond) 2011;72(10):M154-6. [Crossref]
- [3] Ding Z, Shao M, Fu Y. Low-rank embedded ensemble semantic dictionary for zero-shot learning. In: Proceedings of the IEEE conference on computer vision and pattern recognition; 2017. pp. 2050-2058
- 4. Ekemen S, Özen E. Yoğun bakım monitörizasyonunda standart ve güncel uygulamalar. Turkiye Klinikleri J Intensive Care-Special Topics 2015;1(3):1-9.
- [4] Zhang L, Xiang T, Gong S. Learning a deep embedding model for zero-shot learning. In: Proceedings of the IEEE Conference on Computer Vision and Pattern Recognition; 2017. pp. 2021-2030.
- 5. Kesici S. Bayrakçı B. Yoğun bakımda monitorizasyonda yenilikler. Turkiye Klinikleri J Pediatr Sci 2011;7(1):43-8.
- [5] Xian Y, Lorenz T, Schiele B, Akata Z. Feature generating networks for zero-shot learning. In: Proceedings of the IEEE conference on computer vision and pattern recognition; 2018. pp. 5542-5551.
- 6. Çağlar G. Nöro-yoğun bakım hemşireliği: Temel ilke ve uygulamalar. Turkiye Klinikleri J Neurol-Special Topics 2015;8(3):101-6000000000000000.
- [6] Mishra A, Krishna RS, Mittal A, Murthy HA. A generative model for zero shot learning using conditional variational autoencoders. In: Proceedings of the IEEE Conference on Computer Vision and Pattern Recognition Workshops; 2018. pp. 2188-2196.
- 7. Minokadeh A, Pinsky MR. Postoperative hemodynamic instability and monitoring. Curr Opin Crit Care 2016;22(4):393- 400. [Crossref]
- [7] Dietterich TG, Bakiri G. Solving multiclass learning problems via error-correcting output codes. Journal of Artificial Intelligence Research 1994; 2: pp. 263-286. doi: 10.1613/jair.105
- 8. Korkmaz Doğdu A, Dursun Ergezen F, Kol E. Yoğun bakımda hemşirelerin kullandığı hasta değerlendirme yöntemleri: teknoloji ve fiziksel muayene kullanımı. Yoğun Bakım Hemşireliği Dergisi 2019;23(2):102-6.
- [8] Bart E, Ullman S. Cross-generalization: Learning novel classes from a single example by feature replacement. In: 2005 IEEE Computer Society Conference on Computer Vision and Pattern Recognition (CVPR’05); 2005. pp. 672-679.
- 9. Demir Korkmaz F, Öden TN. Cerrahi yoğun bakım ünitelerinde hemodinamik izlem. İçinde: Özer N, editör. Yoğun Bakım Hemşireliği. 1. Baskı. Ankara: Türkiye Klinikleri; 2019.p.6-15.
- [9] Lampert CH, Nickisch H, Harmeling S. Attribute-based classification for zero-shot visual object categorization. IEEE Transactions on Pattern Analysis and Machine Intelligence 2013; (36)3: pp. 453-465. doi: 10.1109/TPAMI.2013.140
- 10. Ayhan H. Yoğun bakımda hemşirelik bilişimi. Turkiye Klinikleri J Surg Nurs-Special Topics 2016;2(1):47-52.
- [10] Krizhevsky A, Sutskever I, Hinton GE. Imagenet classification with deep convolutional neural networks. In: Advances in Neural Information Processing Systems; 2012. pp. 1097-1105.
- 11. Çekmen N. Monitörizasyon. In Frendly G, Urman RD, editors. Yoğun Bakım Cep Kitabı. Özet Bilgiler ve Güncel Notlar. Ankara: Güneş Tıp Kitabevleri; 2015.
- [11] Soysal OA, Guzel MS. An introduction to zero-shot learning: an essential review. In: 2020 International Congress on Human-Computer Interaction, Optimization and Robotic Applications (HORA); Ankara, Turkey; 2020. pp. 1-4.
- 12. Gazarian PK. Nurses’ response to frequency and types of electrocardiography alarms in a non-criticalcare setting: A descriptive study. Int J Nurs Stud 2014;51(2):190-7. [Crossref]
- [12] Kankuekul P, Kawewong A, Tangruamsub S, Hasegawa O. Online incremental attribute-based zero-shot learning. In: 2012 IEEE Conference on Computer Vision and Pattern Recognition; 2012. pp. 3657-3664.
- 13. Atzema C, Schull MJ, Borgundvaag B, Slaughter GRD, Lee CK. ALARMED: Adverse events in Low-risk patients with chest pain receiving continuous electrocardiographic monitoring in the emergency department. A pilot study. Am J Emerg Med 2006;24(1):62-7. [Crossref]
- [13] Norouzi M, Mikolov T, Bengio S, Singer Y, Shlens J et al. Zero-shot learning by convex combination of semantic embeddings. arXiv preprint arXiv:1312.5650 2013.
- 14. Kol E, İlaslan E, İnce S. Yoğun bakım ünitelerinde gürültü kaynakları ve gürültü düzeyleri. J Turk Soc Intensive Care 2015;13:122-8. [Crossref]
- [14] Jayaraman D, Grauman K. Zero-shot recognition with unreliable attributes. In: Advances in Neural Information Processing Systems; 2014. pp. 3464-3472.
- 15. Özkan S. Solunum sistemi monitörizasyonu. Turkiye Klinikleri J Anest Reanim-Special Topics 2009;2(1):1-9.
- [15] Sumbul G, Cinbis RG, Aksoy S. Fine-grained object recognition and zero-shot learning in remote sensing imagery. IEEE Transactions on Geoscience and Remote Sensing 2017; 56(2): 770-779. doi: 10.1109/TGRS.2017.2754648.
- 16. Yapıcıoğlu Yıldızbaş H. Gaz değişiminin noninvazif monitörizasyonu. Turkiye Klinikleri J Pediatr Sci 2018;14(2):209-14.
- [16] Lawrence ND, Platt JC. Learning to learn with the informative vector machine. In: Proceedings of The Twenty-first International Conference on Machine Learning; 2004. pp. 65.
- 17. Çekmen N. Noninvaziv kardiyovasküler monitörizasyon. Turkiye Klinikleri J Anest Reanim-Special Topics 2009;2(1):10-9.
- [17] Croonenborghs T, Driessens K, Bruynooghe M. Learning relational options for inductive transfer in relational reinforcement learning. In: International Conference on Inductive Logic Programming; 2007. pp. 88-97.
- 18. Gürol Arslan G. Yaşam bulguları, hemşirelik esasları insan sağlığı ve fonksiyonları. İçinde Çakırcalı E, Uysal N, editors. 7. Baskı. Ankara: Palme Yayınevi; 2015.p.329-54.
- [18] Raykar VC, Krishnapuram B, Bi J, Dundar M, Rao RB. Bayesian multiple instance learning: automatic feature selection and inductive transfer. In: Proceedings of The 25th International Conference on Machine Learning; 2008. pp. 808-815.
- 19. Arıcı M, Birdane A, Güler K, Yıldız BO, Altun B, Ertürk Ş, et al. [Turkish Hypertension Consensus Report]. Türk Kardiyol Dern Arş 2015;43(4):402-9. [Crossref]
- [19] Rückert U, Kramer S. Kernel-based inductive transfer. In: Joint European Conference on Machine Learning and Knowledge Discovery in Databases; 2008. pp. 220-233.
- 20. Imhoff M, Kuhls S. Alarm algorithms in critical care monitoring. Anesth Analg 2006;102(5):1525-37. [Crossref]
- [20] Akata Z, Perronnin F, Harchaoui Z, Schmid C. Label-embedding for attribute-based classification. In: Proceedings of the IEEE Conference on Computer Vision and Pattern Recognition; 2013. pp. 819-826.
- 21. Irwin RS, Cerra FB, Rippe JM. Intensive care medicine Volume I. 4th ed. New York: Lipincott-Raven Philadelphia;1999.p.217.
- [21] Lampert CH, Nickisch H, Harmeling S. Learning to detect unseen object classes by between-class attribute transfer. In: 2009 IEEE Conference on Computer Vision and Pattern Recognition; 2009. pp. 951-958.
- 22. Ulugöl H, Toraman F. Hemodinamik parametreler ve izlem-mortalite ve morbidite ölçekleri, yoğun bakım skorlama sistemleri. İçinde: Eti Aslan F, Olgun N, editör. Yoğun Bakım. 1. baskı. Ankara: Akademisyen Tıp Kitabevi; 2016.p.15- 24.
- [22] Patterson G, Hays J. Sun attribute database: Discovering, annotating, and recognizing scene attributes. In: 2012 IEEE Conference on Computer Vision and Pattern Recognition; 2012. pp. 2751-2758.
- [23] Farhadi A, Endres I, Hoiem D, Forsyth D. Describing objects by their attributes. In: 2009 IEEE Conference on Computer Vision and Pattern Recognition; 2009. pp. 1778-1785.
- [24] Wah C, Branson S, Welinder P, Perona P, Belongie S. The caltech-ucsd birds-200-2011 dataset; 2011.
- [25] Xian Y, Lampert CH, Schiele B, Akata Z. Zero-shot learning—A comprehensive evaluation of the good, the bad and the ugly. IEEE Transactions on Pattern Analysis and Machine Intelligence 2018; 41(9): 2251-2265. doi: 10.1109/TPAMI.2018.2857768.
- [26] Holland JH et al. Adaptation in natural and artificial systems: an introductory analysis with applications to biology, control, and artificial intelligence: MIT press, 1992.
- [27] ÖZSARI Ş, UĞUZ H, ÇAY T. Interview in land consolidation using genetic algorithm. Communications Faculty of Sciences University of Ankara Series A2-A3 2018; 60 (2): 129-146. doi: 10.1501/commua1-2_0000000119.
- [28] Kennedy J, Eberhart R. Particle swarm optimization. In: Proceedings of ICNN’95-International Conference on Neural Networks; 1995. pp. 1942-1948.
- [29] Shi Y, Eberhart RC. Parameter selection in particle swarm optimization. In: International Conference on Evolutionary Programming; 1998. pp. 591-600.
- [30] Sengupta S, Basak S, Peters RA. Particle swarm optimization: a survey of historical and recent developments with hybridization perspectives. Machine Learning and Knowledge Extraction 2019; 1(1): 157-191. doi: 10.3390/make1010010.
- [31] Güzel MS, Kara M, Beyazkılıç MS. An adaptive framework for mobile robot navigation. Adaptive Behavior 2017; 25(1): 30-39. doi: 10.1177/1059712316685875.
- [32] Neoh SC, Morad N, Marzuki A, Lim CP, Aziz ZA. A multi-resolution GA-PSO layered encoding cascade optimization model. In: Innovations in Swarm Intelligence 248th ed. Berlin, Heidelberg, Germany: Springer, 2009, pp. 121-140.
- [33] Xie B, Chen S, Liu F. Biclustering of gene expression data using PSO-GA hybrid. In: 2007 1st International Conference on Bioinformatics and Biomedical Engineering; 2007. pp. 302-305.
- [34] Robinson J, Rahmat-Samii Y. Particle swarm optimization in electromagnetics. IEEE transactions on antennas and propagation 2004; 52(2): 397-407. doi: 10.1109/TAP.2004.823969.
- [35] Gidemen G, Furat M. PID denetleyici optimizasyonu Üzerine uygulamasi. In: 2017 International Artificial Intelligence and Data Processing Symposium (IDAP); 2017. pp. 1-6.
- [36] Simon D. Evolutionary Optimization Algorithms. Hoboken, NJ, USA: John Wiley & Sons, 2013.
APA | ÖZSARI Ş, Gökce Eskin S, Guzel M, er f, Bostanci G, BOYRAZ S, AYDIN A, Kurt İ (2021). Adaptation of metaheuristic algorithms to improve training performance of an ESZSL model. , 1781 - 1796. 10.3906/elk-2008-113 |
Chicago | ÖZSARI Şifa,Gökce Eskin Serap,Guzel Mehmet,er feyza,Bostanci Gazi Erkan,BOYRAZ Sakine,AYDIN Ayhan,Kurt İbrahim Adaptation of metaheuristic algorithms to improve training performance of an ESZSL model. (2021): 1781 - 1796. 10.3906/elk-2008-113 |
MLA | ÖZSARI Şifa,Gökce Eskin Serap,Guzel Mehmet,er feyza,Bostanci Gazi Erkan,BOYRAZ Sakine,AYDIN Ayhan,Kurt İbrahim Adaptation of metaheuristic algorithms to improve training performance of an ESZSL model. , 2021, ss.1781 - 1796. 10.3906/elk-2008-113 |
AMA | ÖZSARI Ş,Gökce Eskin S,Guzel M,er f,Bostanci G,BOYRAZ S,AYDIN A,Kurt İ Adaptation of metaheuristic algorithms to improve training performance of an ESZSL model. . 2021; 1781 - 1796. 10.3906/elk-2008-113 |
Vancouver | ÖZSARI Ş,Gökce Eskin S,Guzel M,er f,Bostanci G,BOYRAZ S,AYDIN A,Kurt İ Adaptation of metaheuristic algorithms to improve training performance of an ESZSL model. . 2021; 1781 - 1796. 10.3906/elk-2008-113 |
IEEE | ÖZSARI Ş,Gökce Eskin S,Guzel M,er f,Bostanci G,BOYRAZ S,AYDIN A,Kurt İ "Adaptation of metaheuristic algorithms to improve training performance of an ESZSL model." , ss.1781 - 1796, 2021. 10.3906/elk-2008-113 |
ISNAD | ÖZSARI, Şifa vd. "Adaptation of metaheuristic algorithms to improve training performance of an ESZSL model". (2021), 1781-1796. https://doi.org/10.3906/elk-2008-113 |
APA | ÖZSARI Ş, Gökce Eskin S, Guzel M, er f, Bostanci G, BOYRAZ S, AYDIN A, Kurt İ (2021). Adaptation of metaheuristic algorithms to improve training performance of an ESZSL model. Turkish Journal of Electrical Engineering and Computer Sciences, 12(27), 1781 - 1796. 10.3906/elk-2008-113 |
Chicago | ÖZSARI Şifa,Gökce Eskin Serap,Guzel Mehmet,er feyza,Bostanci Gazi Erkan,BOYRAZ Sakine,AYDIN Ayhan,Kurt İbrahim Adaptation of metaheuristic algorithms to improve training performance of an ESZSL model. Turkish Journal of Electrical Engineering and Computer Sciences 12, no.27 (2021): 1781 - 1796. 10.3906/elk-2008-113 |
MLA | ÖZSARI Şifa,Gökce Eskin Serap,Guzel Mehmet,er feyza,Bostanci Gazi Erkan,BOYRAZ Sakine,AYDIN Ayhan,Kurt İbrahim Adaptation of metaheuristic algorithms to improve training performance of an ESZSL model. Turkish Journal of Electrical Engineering and Computer Sciences, vol.12, no.27, 2021, ss.1781 - 1796. 10.3906/elk-2008-113 |
AMA | ÖZSARI Ş,Gökce Eskin S,Guzel M,er f,Bostanci G,BOYRAZ S,AYDIN A,Kurt İ Adaptation of metaheuristic algorithms to improve training performance of an ESZSL model. Turkish Journal of Electrical Engineering and Computer Sciences. 2021; 12(27): 1781 - 1796. 10.3906/elk-2008-113 |
Vancouver | ÖZSARI Ş,Gökce Eskin S,Guzel M,er f,Bostanci G,BOYRAZ S,AYDIN A,Kurt İ Adaptation of metaheuristic algorithms to improve training performance of an ESZSL model. Turkish Journal of Electrical Engineering and Computer Sciences. 2021; 12(27): 1781 - 1796. 10.3906/elk-2008-113 |
IEEE | ÖZSARI Ş,Gökce Eskin S,Guzel M,er f,Bostanci G,BOYRAZ S,AYDIN A,Kurt İ "Adaptation of metaheuristic algorithms to improve training performance of an ESZSL model." Turkish Journal of Electrical Engineering and Computer Sciences, 12, ss.1781 - 1796, 2021. 10.3906/elk-2008-113 |
ISNAD | ÖZSARI, Şifa vd. "Adaptation of metaheuristic algorithms to improve training performance of an ESZSL model". Turkish Journal of Electrical Engineering and Computer Sciences 12/27 (2021), 1781-1796. https://doi.org/10.3906/elk-2008-113 |