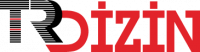
Detection of amyotrophic lateral sclerosis disease by variational mode decomposition and convolution neural network methods from event-related potential signals
Yıl: 2021 Cilt: 29 Sayı: Özel sayı 1 Sayfa Aralığı: 2840 - 2854 Metin Dili: İngilizce DOI: 10.3906/elk-2105-86 İndeks Tarihi: 29-06-2022
Detection of amyotrophic lateral sclerosis disease by variational mode decomposition and convolution neural network methods from event-related potential signals
Öz: Amyotrophic lateral sclerosis (ALS), also known as motor neuron disease, is a neurological disease that occurs
as a result of damage to the nerves in the brain and restriction of muscle movements. Electroencephalography (EEG) is
the most common method used in brain imaging to study neurological disorders. Diagnosis of neurological disorders such
as ALS, Parkinson’s, attention deficit hyperactivity disorder is important in biomedical studies. In recent years, deep
learning (DL) models have been started to be applied in the literature for the diagnosis of these diseases. In this study,
event-related potentials (ERPs) were obtained from EEG signals obtained as a result of visual stimuli from ALS patients
and healthy controls. As a new method, variational mode decomposition (VMD) is applied to the produced ERP signals
and the signals are decomposed into subbands. In addition, empirical mode decomposition (EMD), one of the popular
decomposition methods in the literature, was also analyzed, and ERP signals were divided into subbands and compared
with the VMD method. Subband signals were classified in two stages with the one-dimensional convolutional neural
network (1D CNN) model, which is one of the DL techniques proposed in the study. Accuracy, sensitivity, specificity, and
F1-Score measurements were obtained using 5- and 10-fold cross-validation to evaluate classifier performance. In the first
stage of classification, only VMD and EMD subband signals were used and 92.95% classification accuracy was obtained
by the VMD method. In the second stage, VMD, EMD subband signals, and original ERP signals were all classified
together with the VMD+ERP model achieving the maximum classification accuracy rate of 90.42%. It is thought that
the results of the study will contribute to the diagnosis of similar neurological disorders such as ALS, attention studies
based on visual stimuli, and the development of brain-computer interface (BCI) systems using the method applied to
the proposed ERP signals.
Anahtar Kelime: Belge Türü: Makale Makale Türü: Araştırma Makalesi Erişim Türü: Erişime Açık
- [1] Al-Chalabi A, Hardiman O, Kiernan MC, Chiò A, Rix-Brooks B et al. Amyotrophic lateral sclerosis: moving towards a new classification system. The Lancet Neurology 2016; 15 (11): 1182-1194. doi: 10.1016/S1474-4422(16)30199-5
- [2] Mir N, Sarirete A, Hejres J, Al Omairi M. Use of EEG technology with based brain-computer interface to address amyotrophic lateral sclerosis—ALS. In: The International Research & Innovation Forum 2019; Springer, Cham: pp. 433-439. doi: 10.1007/978-3-030-30809-4_39
- [3] Oliveira ASB, Pereira RDB. Amyotrophic lateral sclerosis (ALS): three letters that change the people’s life. For ever. Arquivos de neuro-psiquiatria 2009; 67(3A): 750-782. doi: 10.1590/S0004-282X2009000400040
- [4] Arico P. Mental States monitoring through passive brain-computer interface systems. PhD, University of Bologna, Italy: 2014.
- [5] Zhang K, Robinson N, Lee SW, Guan C. Adaptive transfer learning for EEG motor imagery classification with deep Convolutional Neural Network. Neural Networks 2021; 136: 1-10.
- [6] Sanei S, Chambers JA. EEG Signal Processing. Hoboken, NJ, USA: John Wiley & Sons, 2013.
- [7] Luck SJ, Kappenman SE (editors). The Oxford Handbook of Event-Related Potential Components. Oxford University Press, 2011.
- [8] Sutton S, Braren M, Zubin J, John, ER. Evoked-potential correlates of stimulus uncertainty. Science 1965; 150 (3700): 1187-1188. doi: 10.1126 / science.150.3700.1187
- [9] Güven A, Altınkaynak M, Dolu N, İzzetoğlu M, Pektaş F et al. Combining functional near-infrared spectroscopy and EEG measurements for the diagnosis of attention-deficit hyperactivity disorder. Neural Computing and Applications 2019: 1-14. doi: 10.1007/s00521-019-04294-7
- [10] Orhanbulucu F, Latifoğlu F, Baş A. K-Ortalamalar Kümeleme Yöntemi Kullanılarak ALS Hastalarında Dikkatin Olaya İlişkin Potansiyel Sinyalleri İle İncelenmesi. Avrupa Bilim ve Teknoloji Dergisi (EJOSAT) 2020: 239-244 (in Turkish).
- [11] Orekhova EV, Stroganova TA. Arousal and attention reorienting in autism spectrum disorders: evidence from auditory event-related potentials. Front Hum Neurosci 2014; 8: 1–17. doi: 10.3389/fnhum.2014.00034
- [12] Rupom AI, Patwary AB. P300 speller based ALS detection using daubechies wavelet transform in electroencephalograph. In: 2019 International Conference on Electrical, Computer and Communication Engineering (ECCE) 2019; IEEE: pp. 1-5.
- [13] McCane LM, Heckman SM,McFarland DJ, Townsend G, Mak JN et al. P300-based brain-computer interface (BCI) event-related potentials (ERPs): People with amyotrophic lateral sclerosis (ALS) vs. age-matched controls. Clinical Neurophysiology 2015; 126 (11): 2124-2131. doi: 10.1016/j.clinph.2015.01.013
- [14] Singh BK, Tikka SK, Singh LK. Investigation of quantitative electroencephalography markers for schizophrenia diagnosis using variational mode decomposition. In: 2021 International Conference on Emerging Smart Computing and Informatics (ESCI) 2021; IEEE: pp. 466-470.
- [15] Rahman MM, Bhuiyan MIH, Das AB. Classification of focal and non-focal EEG signals in VMD-DWT domain using ensemble stacking. Biomedical Signal Processing and Control 2019; 50: 72-82.
- [16] Williams N, Nasuto SJ, Saddy JD. Evaluation of empirical mode decomposition for event-related potential analysis. EURASIP Journal on Advances in Signal Processing 2011; 1-11.
- [17] Taran S, Bajaj V. Clustering variational mode decomposition for identification of focal EEG signals. IEEE sensors letters 2018; 2 (4): 1-4.
- [18] Ullal A, Pachori RB. EEG signal classification using variational mode decomposition. arXiv preprint 2020; arXiv:2003: 12690
- [19] Uyulan Ç, Ergüzel TT, Tarhan N. Elektroensefalografi tabanlı sinyallerin analizinde derin öğrenme algoritmalarının kullanılması. JNBS 2019; 108 (in Turkish). doi: 10.5455/JNBS.1553607558
- [20] Gautam R, Sharma M. Prevalence and diagnosis of neurological disorders using different deep learning techniques: a meta-analysis. Journal of medical systems 2020; 44 (2): 1-24. doi: 10.1007/s10916-019-1519-7
- [21] Sengur A, Akbulut Y, Guo Y, Bajaj V. Classification of amyotrophic lateral sclerosis disease based on convolutional neural network and reinforcement sample learning algorithm. Health information science and systems 2017; 5(1): 1-7. doi: 10.1007/s13755-017-0029-6
- [22] Rusiya P, Chaudhari NS. Amyotrophic lateral sclerosis EEG classification using deep neural network And TLBO. In: Proceedings of the International Conference on Innovative Computing & Communications (ICICC) 2020. doi: 10.2139/ssrn.3565002
- [23] Pandey P, Seeja KR. Subject independent emotion recognition from EEG using VMD and deep learning. Journal of King Saud University-Computer and Information Sciences 2019.
- [24] Kshirsagar GB, Londhe ND. Deep convolutional neural network based character detection in devanagari script input based P300 speller. In: 2017 International Conference on Electrical, Electronics, Communication, Computer, and Optimization Techniques (ICEECCOT), IEEE; 2017: pp. 507-511.
- [25] Cecotti H, Graser A. Convolutional neural networks for P300 detection with application to brain-computer interfaces. IEEE transactions on pattern analysis and machine intelligence 2010; 33 (3): 433-445.
- [26] Vahid A, Bluschke A, Roessner V, Stober S, Beste C. Deep learning based on event-related EEG differentiates children with ADHD from healthy controls. Journal of Clinical Medicine 2019; 8 (7): 1055. doi: 10.3390/jcm8071055
- [27] Murugappan M, Alshuaib W, Bourisly AK, Khare SK, Sruthi S et al. Tunable Q wavelet transform based emotion classification in Parkinson’s disease using Electroencephalography. Plos one 2020; 15 (11): e0242014. doi: 10.1371/journal.pone.0242014
- [28] Oh SL, Vicnesh J, Ciaccio EJ, Yuvaraj R, Acharya UR. Deep convolutional neural network model for automated diagnosis of schizophrenia using EEG signals. Applied Sciences 2019; 9 (14): 2870. doi: 10.3390/app9142870
- [29] Craik A, He Y, Contreras-Vidal JL. Deep learning for electroencephalogram (EEG) classification tasks: a review. Journal of Neural Engineering 2019; 16(3), 031001. doi: 10.1088/1741-2552/ab0ab5
- [30] Riccio A, Simione L, Schettini F, Pizzimenti A, Inghilleri M et al. Attention and P300-based BCI performance in people with amyotrophic lateral sclerosis. Frontiers in Human Neuroscience 2013; 7: 732. doi: 10.3389/fnhum.2013.00732
- [31] Farwell LA, Donchin E. Talking off the top of your head: toward a mental prosthesis utilizing event-related brain potentials. Electroencephalography and Clinical Neurophysiology 1988; 70 (6): 510-523. doi: 10.1016/0013- 4694(88)90149-6
- [32] Aricò P, Aloise F, Schettini F, Salinari S, Mattia D et al. Influence of P300 latency jitter on event related potentialbased brain–computer interface performance. Journal of Neural Engineering 2014; 11 (3): 035008.
- [33] Akiyama M, Tero A, Kawasaki M, Nishiura Y, Yamaguchi Y. Theta-alpha EEG phase distributions in the frontal area for dissociation of visual and auditory working memory. Scientific Reports 2017; 7: 42776. doi: 10.1038/srep42776 (2017).
- [34] Huang NE, Shen Z, Long SR, Wu MC, Shih HH et al. The empirical mode decomposition and the Hilbert spectrum for nonlinear and non-stationary time series analysis. Proceedings of the Royal Society of London. Series A: Mathematical, Physical and Engineering Sciences 1998; 454 (1971):903-995.
- [35] Dragomiretskiy K, Zosso D. Variational mode decomposition. IEEE Transactions on Signal Processing 2013; 62 (3): 531-544.
- [36] Rahman MM, Bhuiyan MIH, Das AB. Classification of focal and non-focal EEG signals in VMD-DWT domain using ensemble stacking. Biomedical Signal Processing and Control 2019; 50: pp. 72-82. doi: 10.1016/j.bspc.2019.01.012
- [37] LeCun Y, Bottou L, Bengio Y, Haffner P. Gradient-based learning applied to document recognition. Proceedings of the IEEE 1998; 86 (11): 2278-2324.
- [38] Freer D, Yang GZ. Data augmentation for self-paced motor imagery classification with C-LSTM. Journal of Neural Engineering 2020; 17 (1): 016041. doi: 10.1088/1741-2552/ab57c0
- [39] Hossin M, Sulaiman MN. A review on evaluation metrics for data classification evaluations. International Journal of Data Mining & Knowledge Management Process 2015; 5 (2): 1. doi: 10.5121/ijdkp.2015.5201
- [40] Albawi S,Mohammed TA,Al-Zawi S. Understanding of a convolutional neural network. 2017 International Conference on Engineering and Technology (ICET), 2017: pp. 1-6.
- [41] Latifoğlu F, İleri R, Demirci E. Assessment of dyslexic children with EOG signals: Determining retrieving words/rereading and skipping lines using convolutional neural networks. Chaos, Solitons & Fractals 2021; 145: 110721. doi: 10.1016/j.chaos.2021.110721
- [42] Mishra VK, Bajaj V,Kumar A. Classification of normal, ALS, and myopathy EMG signals using ELM classifier. I:n 2016 2nd international conference on advances in electrical, electronics, information, communication and bioinformatics (AEEICB) 2016; IEEE: 455-459.
- [43] Mishra VK, Bajaj V, Kumar A, Singh GK. Analysis of ALS and normal EMG signals based on empirical mode decomposition. IET Science, Measurement & Technology 2016; 10 (8): 963-971.
APA | Latifoğlu F, ORHANBULUCU F, ILERI R (2021). Detection of amyotrophic lateral sclerosis disease by variational mode decomposition and convolution neural network methods from event-related potential signals. , 2840 - 2854. 10.3906/elk-2105-86 |
Chicago | Latifoğlu Fatma,ORHANBULUCU FIRAT,ILERI Ramis Detection of amyotrophic lateral sclerosis disease by variational mode decomposition and convolution neural network methods from event-related potential signals. (2021): 2840 - 2854. 10.3906/elk-2105-86 |
MLA | Latifoğlu Fatma,ORHANBULUCU FIRAT,ILERI Ramis Detection of amyotrophic lateral sclerosis disease by variational mode decomposition and convolution neural network methods from event-related potential signals. , 2021, ss.2840 - 2854. 10.3906/elk-2105-86 |
AMA | Latifoğlu F,ORHANBULUCU F,ILERI R Detection of amyotrophic lateral sclerosis disease by variational mode decomposition and convolution neural network methods from event-related potential signals. . 2021; 2840 - 2854. 10.3906/elk-2105-86 |
Vancouver | Latifoğlu F,ORHANBULUCU F,ILERI R Detection of amyotrophic lateral sclerosis disease by variational mode decomposition and convolution neural network methods from event-related potential signals. . 2021; 2840 - 2854. 10.3906/elk-2105-86 |
IEEE | Latifoğlu F,ORHANBULUCU F,ILERI R "Detection of amyotrophic lateral sclerosis disease by variational mode decomposition and convolution neural network methods from event-related potential signals." , ss.2840 - 2854, 2021. 10.3906/elk-2105-86 |
ISNAD | Latifoğlu, Fatma vd. "Detection of amyotrophic lateral sclerosis disease by variational mode decomposition and convolution neural network methods from event-related potential signals". (2021), 2840-2854. https://doi.org/10.3906/elk-2105-86 |
APA | Latifoğlu F, ORHANBULUCU F, ILERI R (2021). Detection of amyotrophic lateral sclerosis disease by variational mode decomposition and convolution neural network methods from event-related potential signals. Turkish Journal of Electrical Engineering and Computer Sciences, 29(Özel sayı 1), 2840 - 2854. 10.3906/elk-2105-86 |
Chicago | Latifoğlu Fatma,ORHANBULUCU FIRAT,ILERI Ramis Detection of amyotrophic lateral sclerosis disease by variational mode decomposition and convolution neural network methods from event-related potential signals. Turkish Journal of Electrical Engineering and Computer Sciences 29, no.Özel sayı 1 (2021): 2840 - 2854. 10.3906/elk-2105-86 |
MLA | Latifoğlu Fatma,ORHANBULUCU FIRAT,ILERI Ramis Detection of amyotrophic lateral sclerosis disease by variational mode decomposition and convolution neural network methods from event-related potential signals. Turkish Journal of Electrical Engineering and Computer Sciences, vol.29, no.Özel sayı 1, 2021, ss.2840 - 2854. 10.3906/elk-2105-86 |
AMA | Latifoğlu F,ORHANBULUCU F,ILERI R Detection of amyotrophic lateral sclerosis disease by variational mode decomposition and convolution neural network methods from event-related potential signals. Turkish Journal of Electrical Engineering and Computer Sciences. 2021; 29(Özel sayı 1): 2840 - 2854. 10.3906/elk-2105-86 |
Vancouver | Latifoğlu F,ORHANBULUCU F,ILERI R Detection of amyotrophic lateral sclerosis disease by variational mode decomposition and convolution neural network methods from event-related potential signals. Turkish Journal of Electrical Engineering and Computer Sciences. 2021; 29(Özel sayı 1): 2840 - 2854. 10.3906/elk-2105-86 |
IEEE | Latifoğlu F,ORHANBULUCU F,ILERI R "Detection of amyotrophic lateral sclerosis disease by variational mode decomposition and convolution neural network methods from event-related potential signals." Turkish Journal of Electrical Engineering and Computer Sciences, 29, ss.2840 - 2854, 2021. 10.3906/elk-2105-86 |
ISNAD | Latifoğlu, Fatma vd. "Detection of amyotrophic lateral sclerosis disease by variational mode decomposition and convolution neural network methods from event-related potential signals". Turkish Journal of Electrical Engineering and Computer Sciences 29/Özel sayı 1 (2021), 2840-2854. https://doi.org/10.3906/elk-2105-86 |