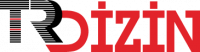
Long-term traffic flow estimation: a hybrid approach using location-based traffic characteristic
Yıl: 2022 Cilt: 30 Sayı: 3 Sayfa Aralığı: 562 - 578 Metin Dili: İngilizce DOI: 10.3906/elk-2104-28 İndeks Tarihi: 01-07-2022
Long-term traffic flow estimation: a hybrid approach using location-based traffic characteristic
Öz: Traffic speed estimation plays a key role in various situations, ranging from individual’s trip planning to urban
traffic management. Despite many studies on short-term prediction, there is only a limited number of studies focusing on
long-term prediction and only a couple of them does go beyond 24 h. On the contrary, this study presents a novel hybrid
architecture using location-based traffic characteristic for traffic speed estimation up to 7 days. In this architecture,
the introduced mean filtering estimation (MFE) model and long short-term memory (LSTM) neural network are jointly
utilized for minimizing the error for traffic flow estimation. Both MFE and LSTM utilizes the speed data, collected
from roadside sensors in İstanbul, of previous weeks that have the same weekday and the same time with target time
to be predicted. Results in this study indicate that the use of MFE gives lower error rates for locations with low traffic
complexity while LSTM outperforms MFE model for locations with high traffic complexity. Thanks to the introduced
MFE and the proposed hybrid architecture, we are able to predict the speed data of a given location with an error of
lower than +/- 10 km/h.
Anahtar Kelime: Belge Türü: Makale Makale Türü: Araştırma Makalesi Erişim Türü: Erişime Açık
- [1] Wang J, Chen R, He Z. Traffic speed prediction for urban transportation network: A path based deep learning approach. Transportation Research Part C: Emerging Technologies 2019; 100: 372–385. doi: 10.1016/j.trc.2019.02.002
- [2] Wang Z, Su X, Ding Z. Long-term traffic prediction based on lstm encoder-decoder architecture. IEEE Transactions on Intelligent Transportation Systems 2020;1–11. doi: 10.1109/TITS.2020.2995546
- [3] Jeong YS, Byon YJ, Castro-Neto MM, Easa SM. Supervised weighting-online learning algorithm for short-term traffic flow prediction. IEEE Transactions on Intelligent Transportation Systems 2013; 14 (4): 1700-1707. doi: 10.1109/TITS.2013.2267735
- [4] Chang H, Lee Y, Yoon B, Baek S. Dynamic near-term traffic flow prediction: system-oriented approach based on past experiences. IET intelligent transport systems 2012; 6(3): 292-305. doi: 10.1049/iet-its.2011.0123
- [5] Van Lint JWC, Hoogendoorn SP, van Zuylen HJ. Accurate freeway travel time prediction with state-space neural networks under missing data. Transportation Research Part C: Emerging Technologies 2005; 13 (5-6): 347-369. doi: doi.org/10.1016/j.trc.2005.03.001
- [6] Zhong M, Sharma S, Lingras P. Short-term traffic prediction on different types of roads with genetically designed regression and time delay neural network models. Journal of computing in civil engineering 2005; 19 (1): 94-103.
- [7] Kumar K, Parida M, Katiyar V. K. Short term traffic flow prediction for a non urban highway using artificial neural network. Procedia-Social and Behavioral Sciences 2013; 104, 755-764. doi: 10.1016/j.sbspro.2013.11.170
- [8] Lv Y, Duan Y, Kang W, Li Z, Wang, FY. Traffic flow prediction with big data: a deep learning approach. IEEE Transactions on Intelligent Transportation Systems 2014; 16 (2): 865-873. doi: 10.1109/TITS.2014.2345663
- [9] Wu Y, Tan H, Qin L, Ran B, Jiang Z. A hybrid deep learning based traffic flow prediction method and its understanding. Transportation Research Part C: Emerging Technologies 2018; 90:166–180. doi: 10.1016/j.t<c.2018.03.001
- [10] Zhao J, Gao Y, Bai Z, Wang H, Lu S. Traffic speed prediction under non-recurrent congestion: Based on LSTM method and BeiDou navigation satellite system data. IEEE Intelligent Transportation Systems Magazine 2019; 11 (2): 70-81. doi: 10.1109/MITS.2019.2903431
- [11] Yufang L, Mingnuo C, Wanzhong Z. Investigating long-term vehicle speed prediction based on BP-LSTM algorithms. IET Intelligent Transport Systems 2019; 13 (8): 1281-1290. doi: 10.1049/iet-its.2018.5593
- [12] Bui KHN, Cho J, Yi H. Spatial-temporal graph neural network for traffic forecasting: An overview and open research issues. Applied Intelligence 2021; 1-12. doi: 10.1007/s10489-021-02587-w
- [13] Zhao Z, Chen W, Wu X, Chen PC, Liu J. LSTM network: a deep learning approach for short-term traffic forecast. IET Intelligent Transport Systems 2017; 11 (2): 68-75. doi: doi.org/10.1049/iet-its.2016.0208
- [14] Lu Z, Lv W, Cao Y, Xie Z, Peng H et al. LSTM variants meet graph neural networks for road speed prediction. Neurocomputing 2020; 400: 34-45. doi: 10.1016/j.neucom.2020.03.031
- [15] Habtemichael FG, Cetin M. Short-term traffic flow rate forecasting based on identifying similar traffic patterns. Transportation research Part C: emerging technologies 2016; 66: 61-78. doi: 10.1016/j.trc.2015.08.017
- [16] Li R, Hu Y, Liang Q. T2F-LSTM Method for Long-Term Traffic Volume Prediction. IEEE Transactions on Fuzzy Systems 2020; 28 (12): 3256-3264. doi: 10.1109/TFUZZ.2020.2986995
- [17] Bogaerts T, Masegosa AD , Angarita-Zapata JS, Onieva E, Hellinckx P. A graph cnn-lstm neural network for short and long-term traffic forecasting based on trajectory data. Transportation Research PartC: Emerging Technologies 2020; 112:62–77. doi: 10.1016/j.trc.2020.01.010
- [18] Chen W, An J, Li R, Fu L, Xie G et al. A novel fuzzy deep-learning approach to traffic flow prediction with uncertain spatial–temporal data features. Future generation computer systems 2018; 89: 78-88. doi: 10.1016/j.future.2018.06.021
- [19] Zang D, Ling J, Wei Z, Tang K, Cheng J. Long-term traffic speed prediction based on multiscale spatio-temporal feature learning network. IEEE Transactions on Intelligent Transportation Systems 2018; 20 (10):3700–3709. doi: 10.1109/TITS.2018.2878068
- [20] Qu L, Li W, Li W, Ma D, Wang Y. Daily long-term traffic flow forecasting based on a deep neural network. Expert systems with applications 2019; 121:304–312 doi: 10.1016/j.eswa.2018.12.031
- [21] Li Y, Chai S, Ma Z, Wang G. A Hybrid Deep Learning Framework for Long-Term Traffic Flow Prediction. IEEE Access 2021; 9: 11264-11271. doi: 10.1109/ACCESS.2021.3050836
- [22] He Z, Chow CY, Zhang JD. STCNN: A spatio-temporal convolutional neural network for long-term traffic prediction. In: 2019 20th IEEE International Conference on Mobile Data Management (MDM); 2019 pp. 226-233.
- [23] Peng H, Bobade SU, Cotterell ME, Miller JA. Forecasting traffic flow: Short term, long term, and when it rains. In: Springer, Cham International Conference on Big Data 2018; pp. 57-71.
- [24] Belhadi A, Djenouri Y, Djenouri D, Lin DJCW. A recurrent neural network for urban long-term traffic flow forecasting. APPLIED INTELLIGENCE 2020; 50, 3252-3265. doi: 10.1007/s10489-020-01716-1
- [25] Simunek M, Smutny Z. Traffic information enrichment: creating long-term traffic speed prediction ensemble model for better navigation through waypoints. Applied Sciences 2021; 11 (1): 315. doi: 10.3390/app11010315
- [26] Guo YR, Wang XM, Zhang H, Jim GJ. Traffic flow prediction method of diversion area in peak hours based on double flow graph convolution network. Advances in Transportation Studies 2021;
- [27] Wang J, Zhu W, Sun Y, Tian C. An effective dynamic spatiotemporal framework with external features information for traffic prediction. Applied Intelligence 2021; 51 (6): 3159-3173. doi: 10.1007/s10489-020-02043-1
- [28] Ünlü R. Cost-oriented LSTM methods for possible expansion of control charting signals. Computers & Industrial Engineering 2021; 154, 107163. doi: 10.1016/j.cie.2021.107163
- [29] Smola AJ, Schölkopf B. A tutorial on support vector regression. Statistics and computing 2004; 14 (3): 199-222. doi: 10.1023/B:STCO.0000035301.49549.88
- [30] Ahn J, Ko E, Kim EY. Highway traffic flow prediction using support vector regression and Bayesian classifier. In: IEEE International conference on big data and smart computing (BigComp); 2016. pp. 239-244. doi: 10.1109/BIGCOMP.2016.7425919
APA | Ayar T, Atlinar F, Guvensan A, Turkmen H (2022). Long-term traffic flow estimation: a hybrid approach using location-based traffic characteristic. , 562 - 578. 10.3906/elk-2104-28 |
Chicago | Ayar Tuğberk,Atlinar Ferhat,Guvensan Amac,Turkmen H. Irem Long-term traffic flow estimation: a hybrid approach using location-based traffic characteristic. (2022): 562 - 578. 10.3906/elk-2104-28 |
MLA | Ayar Tuğberk,Atlinar Ferhat,Guvensan Amac,Turkmen H. Irem Long-term traffic flow estimation: a hybrid approach using location-based traffic characteristic. , 2022, ss.562 - 578. 10.3906/elk-2104-28 |
AMA | Ayar T,Atlinar F,Guvensan A,Turkmen H Long-term traffic flow estimation: a hybrid approach using location-based traffic characteristic. . 2022; 562 - 578. 10.3906/elk-2104-28 |
Vancouver | Ayar T,Atlinar F,Guvensan A,Turkmen H Long-term traffic flow estimation: a hybrid approach using location-based traffic characteristic. . 2022; 562 - 578. 10.3906/elk-2104-28 |
IEEE | Ayar T,Atlinar F,Guvensan A,Turkmen H "Long-term traffic flow estimation: a hybrid approach using location-based traffic characteristic." , ss.562 - 578, 2022. 10.3906/elk-2104-28 |
ISNAD | Ayar, Tuğberk vd. "Long-term traffic flow estimation: a hybrid approach using location-based traffic characteristic". (2022), 562-578. https://doi.org/10.3906/elk-2104-28 |
APA | Ayar T, Atlinar F, Guvensan A, Turkmen H (2022). Long-term traffic flow estimation: a hybrid approach using location-based traffic characteristic. Turkish Journal of Electrical Engineering and Computer Sciences, 30(3), 562 - 578. 10.3906/elk-2104-28 |
Chicago | Ayar Tuğberk,Atlinar Ferhat,Guvensan Amac,Turkmen H. Irem Long-term traffic flow estimation: a hybrid approach using location-based traffic characteristic. Turkish Journal of Electrical Engineering and Computer Sciences 30, no.3 (2022): 562 - 578. 10.3906/elk-2104-28 |
MLA | Ayar Tuğberk,Atlinar Ferhat,Guvensan Amac,Turkmen H. Irem Long-term traffic flow estimation: a hybrid approach using location-based traffic characteristic. Turkish Journal of Electrical Engineering and Computer Sciences, vol.30, no.3, 2022, ss.562 - 578. 10.3906/elk-2104-28 |
AMA | Ayar T,Atlinar F,Guvensan A,Turkmen H Long-term traffic flow estimation: a hybrid approach using location-based traffic characteristic. Turkish Journal of Electrical Engineering and Computer Sciences. 2022; 30(3): 562 - 578. 10.3906/elk-2104-28 |
Vancouver | Ayar T,Atlinar F,Guvensan A,Turkmen H Long-term traffic flow estimation: a hybrid approach using location-based traffic characteristic. Turkish Journal of Electrical Engineering and Computer Sciences. 2022; 30(3): 562 - 578. 10.3906/elk-2104-28 |
IEEE | Ayar T,Atlinar F,Guvensan A,Turkmen H "Long-term traffic flow estimation: a hybrid approach using location-based traffic characteristic." Turkish Journal of Electrical Engineering and Computer Sciences, 30, ss.562 - 578, 2022. 10.3906/elk-2104-28 |
ISNAD | Ayar, Tuğberk vd. "Long-term traffic flow estimation: a hybrid approach using location-based traffic characteristic". Turkish Journal of Electrical Engineering and Computer Sciences 30/3 (2022), 562-578. https://doi.org/10.3906/elk-2104-28 |