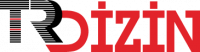
Use of Hybrid Clustering and Scattering Parameters for Liquid Classification
Yıl: 2022 Cilt: 22 Sayı: 2 Sayfa Aralığı: 237 - 243 Metin Dili: İngilizce DOI: 10.54614/electrica.2022.21160 İndeks Tarihi: 03-07-2022
Use of Hybrid Clustering and Scattering Parameters for Liquid Classification
Öz: With the advancement of technology, the use of machine learning techniques has increased. The need for the prevention of terrorist attacks has brought upon the
use of machine learning techniques to explosive detection. Flammable liquids such as alcohol are easily available and widely used in various terrorist attacks. In this
study, a new microwave measurement system is developed and a hybrid clustering approach is proposed to classify liquids. With the proposed measurement system,
the reflection coefficient (S11 parameter) of liquids in bottles is measured at room temperature and these measurements are used as inputs by the proposed clustering
algorithm. The results obtained using the proposed clustering algorithm are compared with the results obtained using a set of well-known clustering algorithms,
that is, K-means, hierarchical clustering, farthest first, and fuzzy C-means, in order to make a fair comparison. The results show that the proposed clustering algorithm
provides 100% accuracy and is superior to the well-known algorithms used in this study. The results will enable us to manufacture a low-cost liquid scanner for railway
stations and shopping malls as well as small airports. The proposed liquid scanner’s design was completed, and the manufacturing phase has been started.
Anahtar Kelime: Belge Türü: Makale Makale Türü: Araştırma Makalesi Erişim Türü: Erişime Açık
- 1. E. Davicioni, and Z. Haddad, “Thyroid cancer diagnostics,” Google Patents, 2018.
- 2. K. Wang, X. Qi, H. Liu, and J. Song, “Deep belief network based k-means cluster approach for short-term wind power forecasting,” Energy, vol. 165, pp. 840–852, 2018.
- 3. W. Zhang, Z. Lian, and C. Huang, “Evaluation of unsupervised clustering methods on hyperspectral image data sets,” in IEEE International Conference on Progress in Informatics and Computing (PIC), 2018, pp. 117–121.
- 4. S. N. H. Sheikh Abdullah et al., “Round randomized learning vector quantization for brain tumor imaging,” Comp. Math. Methods Med., vol. 2016, 8603609, 2016.
- 5. B. R. Shivakumar, and S. V. Rajashekararadhya, “Classification of Landsat 8 imagery using Kohonen’s self organizing maps and learning vector quantization,” in Adv. Commun. Signal Process. VLSI Embedded Syst. Berlin: Springer, 2020, pp. 445–462.
- 6. J. Lee et al., “Classification of diesel and gasoline dual-fuel combustion modes by the analysis of heat release rate shapes in a compression ignition engine,” Fuel, vol. 209, pp. 587–597, 2017.
- 7. I. Barnett, and M. Zhang, “Discrimination of brands of gasoline by using DART-MS and chemometrics,” Forensic Chem., vol. 10, pp. 58–66, 2018.
- 8. V. D. Baquiran, J. A. M. Bate, M. J. B. Sembrano, J. F. Villaverde, and G. V. Magwili, “Determination of methanol, ethanol, and ethylene glycol content in coconut wine using PCA and SIMCA,” in Proc. 2020 12th Int. Conf. Comput. Automat. Eng., 2020, pp. 150–154.
- 9. J. Wang, M. Chang, W. He, X. Lu, S. Fei, and G. Lu, “Optimization of electronic nose sensor array for tea aroma detecting based on correlation coefficient and cluster analysis,” Sens. Actuators B, p. 128068, 2020.
- 10. J. A. Ardila, F. L. F. Soares, M. A. dos Santos Farias, and R. L. Carneiro, “Characterization of gasoline by Raman spectroscopy with chemometric analysis,” Anal. Lett., vol. 50, no. 7, pp. 1126–1138, 2017.
- 11. J. Dadson, S. Pandam, and N. Asiedu, “Modeling the characteristics and quantification of adulterants in gasoline using FTIR spectroscopy and chemometric calibrations,” Cogent Chem., vol. 4, no. 1, p. 1482637, 2018.
- 12. I. M. A. G. Wirasuta et al., “A rapid method for screening and determination test of methanol content in ethanol-based products using portable Raman spectroscopy,” Forensic Chem., vol. 16, p. 100190, 2019.
- 13. F. Mabood et al., “Detection and estimation of Super premium 95 gasoline adulteration with Premium 91 gasoline using new NIR spectroscopy combined with multivariate methods,” Fuel, vol. 197, pp. 388–396, 2017.
- 14. T. Hayasaka et al., “An electronic nose using a single graphene FET and machine learning for water, methanol, and ethanol,” Microsyst. Nanoeng., vol. 6, no. 1, p. 50, 2020.
- 15. P. Orachorn, N. Chankow, and S. Srisatit, “An alternative method for screening liquid in bottles at airports using low energy X-ray transmission technique,” in Radiation environment and medicine: Covering a broad scope of topics relevant to environmental and medical radiation research, vol. 8, no. 2, pp. 77–84, 2019.
- 16. H. Chen, Z. Hu, P. Wang, W. Xu, and Y. Hou, “Application of spectral droplet analysis method in flammable liquids identification,” in 2019 Int. Conf. Optical Instruments Tech.: Optical Sensors Appl., International Society for Optics and Photonics, 2020, vol. 11436, p. 1143609.
- 17. P. Skládal, “Smart bioelectronic tongues for food and drinks control,” TrAC Trends Anal. Chem., vol. 127, p. 115887, 2020.
- 18. A. Gorji-Chakespari, A. M. Nikbakht, F. Sefidkon, M. Ghasemi-Varnamkhasti, J. Brezmes, and E. Llobet, “Performance comparison of fuzzy ARTMAP and LDA in qualitative classification of Iranian Rosa damascena essential oils by an electronic nose,” Sensors (Basel), vol. 16, no. 5, p. 636, 2016.
- 19. L. Guo, and A. Abbosh, “Stroke localization and classification using microwave tomography with k‐means clustering and support vector machine,” Bioelectromagnetics, vol. 39, no. 4, pp. 312–324, 2018.
- 20. Z. Zhang, Y. Yu, X. Li, F. Hui, X. Cheng, and Z. Chen, “Arctic sea ice classification using microwave Scatterometer and radiometer data during 2002–2017,” IEEE Trans. Geosci. Remote Sens., vol. 57, no. 8, pp. 5319–5328, 2019.
- 21. Z. Zhang, F. Hui, X. Cheng, and Z. Chen, “Arctic Sea ice classification using microwave scatterometer and radiometer data,” AGUFM, vol. 2018, p. C31E-1573, 2018.
- 22. B. Saçlı et al., “Microwave dielectric property based classification of renal calculi: Application of a kNN algorithm,” Comput. Biol. Med., vol. 112, p. 103366, 2019.
- 23. S. P. Rana et al., “Machine learning approaches for automated lesion detection in microwave breast imaging clinical data,” Sci. Rep., vol. 9, no. 1, 10510, 2019.
- 24. J. Van den Broek, S. Abegg, S. E. Pratsinis, and A. T. Güntner, “Highly selective detection of methanol over ethanol by a handheld gas sensor,” Nat. Commun., vol. 10, no. 1, p. 4220, 2019.
- 25. J. V. Ashurst, and T. M. Nappe, “Methanol toxicity,” StatPearls Publishing, Treasure Island (FL), PMID: 29489213, 2019.
APA | Efeoğlu E, TUNA G (2022). Use of Hybrid Clustering and Scattering Parameters for Liquid Classification. , 237 - 243. 10.54614/electrica.2022.21160 |
Chicago | Efeoğlu Ebru,TUNA Gürkan Use of Hybrid Clustering and Scattering Parameters for Liquid Classification. (2022): 237 - 243. 10.54614/electrica.2022.21160 |
MLA | Efeoğlu Ebru,TUNA Gürkan Use of Hybrid Clustering and Scattering Parameters for Liquid Classification. , 2022, ss.237 - 243. 10.54614/electrica.2022.21160 |
AMA | Efeoğlu E,TUNA G Use of Hybrid Clustering and Scattering Parameters for Liquid Classification. . 2022; 237 - 243. 10.54614/electrica.2022.21160 |
Vancouver | Efeoğlu E,TUNA G Use of Hybrid Clustering and Scattering Parameters for Liquid Classification. . 2022; 237 - 243. 10.54614/electrica.2022.21160 |
IEEE | Efeoğlu E,TUNA G "Use of Hybrid Clustering and Scattering Parameters for Liquid Classification." , ss.237 - 243, 2022. 10.54614/electrica.2022.21160 |
ISNAD | Efeoğlu, Ebru - TUNA, Gürkan. "Use of Hybrid Clustering and Scattering Parameters for Liquid Classification". (2022), 237-243. https://doi.org/10.54614/electrica.2022.21160 |
APA | Efeoğlu E, TUNA G (2022). Use of Hybrid Clustering and Scattering Parameters for Liquid Classification. Electrica, 22(2), 237 - 243. 10.54614/electrica.2022.21160 |
Chicago | Efeoğlu Ebru,TUNA Gürkan Use of Hybrid Clustering and Scattering Parameters for Liquid Classification. Electrica 22, no.2 (2022): 237 - 243. 10.54614/electrica.2022.21160 |
MLA | Efeoğlu Ebru,TUNA Gürkan Use of Hybrid Clustering and Scattering Parameters for Liquid Classification. Electrica, vol.22, no.2, 2022, ss.237 - 243. 10.54614/electrica.2022.21160 |
AMA | Efeoğlu E,TUNA G Use of Hybrid Clustering and Scattering Parameters for Liquid Classification. Electrica. 2022; 22(2): 237 - 243. 10.54614/electrica.2022.21160 |
Vancouver | Efeoğlu E,TUNA G Use of Hybrid Clustering and Scattering Parameters for Liquid Classification. Electrica. 2022; 22(2): 237 - 243. 10.54614/electrica.2022.21160 |
IEEE | Efeoğlu E,TUNA G "Use of Hybrid Clustering and Scattering Parameters for Liquid Classification." Electrica, 22, ss.237 - 243, 2022. 10.54614/electrica.2022.21160 |
ISNAD | Efeoğlu, Ebru - TUNA, Gürkan. "Use of Hybrid Clustering and Scattering Parameters for Liquid Classification". Electrica 22/2 (2022), 237-243. https://doi.org/10.54614/electrica.2022.21160 |