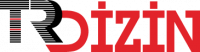
Comparison of Bayesian Regularized Neural Network, Random Forest Regression, Support Vector Regression and Multivariate Adaptive Regression Splines Algorithms to Predict Body Weight from Biometrical Measurements in Th alli Sheep
Yıl: 2022 Cilt: 28 Sayı: 3 Sayfa Aralığı: 411 - 419 Metin Dili: İngilizce DOI: 10.9775/kvfd.2022.27164 İndeks Tarihi: 07-07-2022
Comparison of Bayesian Regularized Neural Network, Random Forest Regression, Support Vector Regression and Multivariate Adaptive Regression Splines Algorithms to Predict Body Weight from Biometrical Measurements in Th alli Sheep
Öz: In this study, it is aimed to compare several data mining and artificial neural network algorithms to predict body weight from biometric
measurements for the Th alli sheep breed. For this purpose, the prediction capabilities of Bayesian Regularized Neural Network (BRNN), Support
Vector Regression (SVR), Random Forest Regression (RFR) and Multivariate Adaptive Regression Splines (MARS) algorithms were comparatively
investigated. To measure the predictive performances of the evaluated algorithms, body measurements such as body length, heart girth, ear length,
ear width, head width, head length, withers height, rump length, rump width neck length, neck width of Th alli sheep were used for predicting
the body weight. In this context, 270 female Th alli sheep were used to predict body weight. Model comparison criteria such as root-mean square
error (RMSE), standard deviation ratio (SDR), performance index (PI), global relative approximation error (RAE), mean absolute percentage error
(MAPE), Pearson’s correlation coefficient (r), determination of coefficient (R2) and Akaike’s information criteria (AIC) were used to compare all
algorithms. In conclusion, the MARS algorithm can be recommended to enable breeders to obtain an elite population of Th alli sheep breed.
Anahtar Kelime: Belge Türü: Makale Makale Türü: Araştırma Makalesi Erişim Türü: Erişime Açık
- 1. FAO: FAOSTAT, 2019. http://www.fao.org/faostat/en/#data/QL; Accessed: 11.03.2021.
- 2. Tariq MM, Bajwa MA, Jawasreh K, Awan MA, Abbas F, Waheed A, Rafeeq M, Wadood A, Khan KU, Rashid N, Atique MA, Bukhari FA: Characterization of four indigenous sheep breeds of Balochistan, Pakistan by random amplified polymorphic DNAs. Afr J Biotechnol, 11, 2581-2586, 2012. DOI: 10.5897/AJB11.3196
- 3. Celik S, Eyduran E, Karadas K, Tariq MM: Comparison of predictive performance of data mining algorithms in predicting body weight in Mengali rams of Pakistan. Braz J Vet Res Anim Sci, 46 (11): 863-872, 2017. DOI: 10.1590/S1806-92902017001100005
- 4. Zhang AL, Wu BP, Wuyun CT, Jiang DX, Xuan EC, Ma FY: Algorithm of sheep body dimension measurement and its applications based on image analysis. Comput Electron Agric, 153, 33-45, 2018. DOI: 10.1016/j. compag.2018.07.033
- 5. Sabbioni A, Beretti V, Superchi P, Ablondi M: Body weight estimation from body measures in Cornigliese sheep breed. Ital J Anim Sci, 19 (1): 25- 30, 2020. DOI: 10.1080/1828051X.2019.1689189
- 6. Faraz A, Tirink C, Eyduran E, Waheed A, Tauqir NA, Nabeel MS, Tariq MM: Prediction of live body weight based on body measurements in Thalli sheep under tropical conditions of Pakistan using CART and MARS. Trop Anim Health Prod, 53 (2): 1-12, 2021. DOI: 10.1007/s11250-021-02748-6
- 7. Cam MA, Olfaz M, Soydan E: Body measurements reflect body weights and carcass yields in Karayaka sheep. Asian J Anim Vet Adv, 5 (2): 120-127, 2010. DOI: 10.3923/ajava.2010.120.127
- 8. Ghotbaldini H, Mohammadabadi M, Nezamabadi-Pour H, Babenko OI, Bushtruk MV, Tkachenko SV: Predicting breeding value of body weight at 6-month age using Artificial Neural Networks in Kermani sheep breed. Acta Sci Anim Sci, 41, 2019. DOI: 10.4025/actascianimsci.v41i1.45282
- 9. Olfaz M, Tirink C, Onder H: Use of CART and CHAID algorithms in Karayaka sheep breeding. Kafkas Univ Vet Fak Derg, 25 (1): 105-110, 2019. DOI: 10.9775/kvfd.2018.20388
- 10. Aytekin I, Eyduran E, Karadas K, Aksahan R, Keskin I: Prediction of fattening final live weight from some body measurements and fattening period in young bulls of crossbred and exotic breeds using MARS data mining algorithm. Pak J Zool, 50 (1): 189-195, 2018. DOI: 10.17582/journal. pjz/2018.50.1.189.195
- 11. Weber VAM, de Lima Weber F, da Silva Oliveira A, Astolfi G, Menezes GV, de Andrade Porto JV, Rezende FPC, de Moraes PH, Matsubara ET, Mateus RG, de Araújo TLAC, da Silva LOC, de Queiroz EQA, de Abreu UGP, da Costa Gomes R, Pistori H: Cattle weight estimation using active contour models and regression trees Bagging. Comput Electron Agric, 179, 105804, 2020. DOI: 10.1016/j.compag.2020.105804
- 12. Salawu EO, Abdulraheem M, Shoyombo A, Adepeju A, Davies S, Akinsola O, Nwagu B: Using artificial neural network to predict body weights of rabbits. Open J Anim Sci, 4, 182-186, 2014. DOI: 10.4236/ojas. 2014.44023
- 13. Celik S, Yilmaz O: Prediction of body weight of Turkish tazi dogs using data mining Techniques: Classification and Regression Tree (CART) and multivariate adaptive regression splines (MARS), Pak J Zool, 50 (2): 575- 583, 2018. DOI: 10.17582/journal.pjz/2018.50.2.575.583
- 14. Fatih A, Celik S, Eyduran E, Tirink C, Tariq MM, Sheikh IS, Faraz A, Waheed A: Use of MARS algorithm for predicting mature weight of different camel (Camelus dromedarius) breeds reared in Pakistan and morphological characterization via cluster analysis. Trop Anim Health Prod, 53 (1): 1-14, 2021. DOI: 10.1007/s11250-021-02633-2
- 15. Topal M, Macit M: Prediction of body weight from body measurements in morkaraman sheep. J Appl Anim Res, 25 (2): 97-100, 2004. DOI: 10.1080/ 09712119.2004.9706484
- 16. Huma ZE, Iqbal F: Predicting the body weight of Balochi sheep using a machine learning approach. Turk J Vet Anim Sci, 43 (4): 500-506, 2019. DOI: 10.3906/vet-1812-23
- 17. Hussain A, Akhtar P, Ali S, Younas M, Yaqoob M, Babar ME, Kaved K, Shakoor A: Factors influencing body weights at different ages in Thalli sheep. J Anim Plant Sci, 23 (1): 1-6, 2013.
- 18. Hussain A, Akhtar P, Ali S, Javed K, Younas M, Shakoor A, Waheed U: Genetic analysis of post-weaning growth traits of Thalli sheep under tropical conditions. Trop Anim Health Prod, 46, 1527-1531, 2014. DOI: 10.1007/ s11250-014-0670-x
- 19. R Core Team: A language and environment for statistical computing. R Foundation for Statistical Computing, Vienna, Austria, 2020. https:// www.R-project.org/; Accessed: 11.03.2021.
- 20. Revelle W: Psych: Procedures for Personality and Psychological Research, Northwestern University, Evanston, Illinois, USA, 2020. https://CRAN.Rproject. org/package=psych Version = 2.0.12; Accessed: 11.03.2021.
- 21. Wei T, Simko V: R package “corrplot”: Visualization of a Correlation Matrix (Version 0.84), 2017. https://github.com/taiyun/corrplot; Accessed: 11.03.2021.
- 22. Kuhn M: Caret: Classification and Regression Training. R package version 6.0-86, 2020. https://CRAN.R-project.org/package=caret; Accessed: 11.03.2021.
- 23. Liaw A, Wiener M: Classification and regression by random forest. R News, 2 (3): 18-22, 2002.
- 24. Meyer D, Dimitriadou E, Hornik K, Weingessel A, Leisch F: e1071: Misc Functions of the Department of Statistics, Probability Theory Group (Formerly: E1071), TU Wien R package version 1.7-4, 2020. https:// CRAN.R-project.org/package=e1071; Accessed: 11.03.2021.
- 25. Eyduran E: ehaGoF: Calculates Goodness of Fit Statistics. R package version 0.1.1., 2020. https://CRAN.R-project.org/package=ehaGoF; Accessed: 11.03.2021.
- 26. Pérez-Rodríguez P, Gianola D, Weigel KA, Rosa GJM, Crossa J: Technical Note: An R package for fitting Bayesian regularized neural networks with applications in animal breeding. J Anim Sci, 91 (8): 3522- 3531, 2013. DOI: 10.2527/jas.2012-6162
- 27. Kayri M: Predictive abilities of bayesian regularization and Levenberg– Marquardt algorithms in artificial neural networks: A comparative empirical study on social data. Math Comput Appl, 21(2): 20, 2016. DOI: 10.3390/ mca21020020
- 28. MacKay DJC: A practical Bayesian framework for backpropagation networks. Neural Comput, 4, 448-472, 1992. DOI: 10.1162/neco.1992.4.3.448
- 29. Foresee FD, Hagan MT: Gauss-Newton approximation to Bayesian learning. Proceedings of International Conference on Neural Networks, 3, 1930-1935, 1997. DOI: 10.1109/ICNN.1997.614194
- 30. Nguyen D, Widrow B: Improving the learning speed of 2-layer neural networks by choosing initial values of the adaptive weights. 1990 IJCNN International Joint Conference on Neural Network, 3, 21-26, 1990. DOI: 10.1109/IJCNN.1990.137819
- 31. Dolezel P, Skrabane P, Gago L: Weight initialization possibilities for feedforward neural network with linear saturated activation functions. IFAC-PapersOnLine, 49 (25): 49-54, 2016. DOI: 10.1016/j.ifacol.2016.12.009
- 32. Linan MN, Gerardo B, Medina R: Modified weight initialization in the self-organizing map using Nguyen-Widrow initialization algorithm. J Phys Conf Ser, 1235:012055, 2019. DOI: 10.1088/1742-6596/1235/1/012055
- 33. Nguyen QT, Fouchereau R, Frénod E, Gerard C, Sincholle V: Comparison of forecast models of production of dairy cows combining animal and diet parameters. Comput Electron Agric, 170, 105258, 2020. DOI: 10.1016/j.compag.2020.105258
- 34. Smola AJ, Schölkopf B: A tutorial on support vector regression. Stat Comput, 14, 199-222, 2004. DOI: 10.1023/B:STCO.0000035301.49549.88
- 35. Kavaklioglu K: Modeling and prediction of Turkey’s electricity consumption using Support Vector Regression. Appl Energy, 88, 368-375, 2011. DOI: 10.1016/j.apenergy.2010.07.021
- 36. Alonso J, Castañón ÁR, Bahamonde A: Support Vector Regression to predict carcass weight in beef cattle in advance of the slaughter. Comput Electron Agric, 91, 116-120, 2013. DOI: 10.1016/j.compag.2012.08.009
- 37. Patel AK, Chatterjee S, Gorai AK: Development of a machine vision system using the support vector machine regression (SVR) algorithm for the online prediction of iron ore grades. 2017 Fifteenth IAPR International Conference on Machine Vision Applications 12, 197-210, 2017. DOI: 10.23919/MVA.2017.7986823
- 38. Breiman L: Random forests. Mach Learn, 45 (1): 5-32, 2001. DOI: 10.1023/A:1010933404324
- 39. Rodriguez-Galiano V, Mendes MP, Garcia-Soldado MJ, Chica-Olmo M, Riberio L: Predictive modeling of groundwater nitrate pollution using Random Forest and multisource variables related to intrinsic and specific vulnerability: A case study in an agricultural setting (Southern Spain). Sci Total Environ, 476-477, 189-206, 2014. DOI: 10.1016/j.scitotenv.2014.01.001
- 40. Wang L, Zhou X, Zhu X, Dong Z, Guo W: Estimation of biomass in wheat using random forest regression algorithm and remote sensing data. Crop J, 4, 212-219, 2016. DOI: 10.1016/j.cj.2016.01.008
- 41. Akin M, Eyduran E, Niedz RP, Reed BM: Developing hazelnut tissue culture free of ion confounding. Plant Cell Tissue Organ Cult, 130 (3): 483- 494, 2017. DOI: 10.1007/s11240-017-1238-z
- 42. Akin M, Eyduran E, Reed BM: Use of RSM and CHAID data mining algorithm for predicting mineral nutrition of hazelnut. Plant Cell Tissue Organ Cult, 128 (2): 303-316, 2017. DOI: 10.1007/s11240-016-1110-6
- 43. Kovalchuk IY, Mukhitdinova Z, Turdiyev T, Madiyeva G, Akin M, Eyduran E, Reed BM: Modeling some mineral nutrient requirements for micropropagated wild apricot shoot cultures. Plant Cell Tissue Organ Cult, 129, 325-335, 2017. DOI: 10.1007/s11240-017-1180-0
- 44. Friedman JH: Multivariate adaptive regression splines. Ann Stat, 19 (1): 1-67, 1991. DOI: 10.1214/aos/1176347963
- 45. Aksoy A, Erturk YE, Eyduran E, Tariq MM: Utility of MARS algorithm for describing non-genetic factors affecting pasture revenue of Morkaraman breed and Romanov x Morkaraman F1 crossbred sheep under semi intensive conditions. Pak J Zool, 51(1): 235-240, 2019. DOI: 10.17582/journal. pjz/2019.51.1.235.240
- 46. Akin M, Eyduran SP, Eyduran E, Reed BM: Analysis of macro nutrient related growth responses using multivariate adaptive regression splines. Plant Cell Tissue Organ Cult, 140, 661-670, 2020. DOI: 10.1007/s11240-019- 01763-8
- 47. Arthur CK, Temeng VA, Ziggah YY: Multivariate Adaptive Regression Splines (MARS) approach to blast-induced ground vibration prediction. Int J Min Reclam Environ, 34 (3): 198-222, 2020. DOI: 10.1080/17480930. 2019.1577940
- 48. Tatliyer A: The Effects of raising type on performances of some data mining algorithms in lambs. KSU J Agric Nat, 23(3), 772-780, 2020. DOI: 10.18016/ksutarimdoga.vi.651232
- 49. Ali M, Eyduran E, Tariq MM, Tirink C, Abbas F, Bajwa MA, Baloch MH, Nizamani AH, Waheed A, Awan MA, Shah SH, Ahmad Z, Jan S: Comparison of artificial neural network and decision tree algorithms used for predicting live weight at post weaning period from some biometrical characteristics in Harnai sheep. Pak J Zool, 47, 1579-1585, 2015.
- 50. Eyduran E, Zaborski D, Waheed A, Celik S, Karadas K, Grzesiak W: Comparison of the predictive capabilities of several data mining algorithms and multiple linear regression in the prediction of body weight by means of body measurements in the indigenous Beetal goat of Pakistan. Pak J Zool, 49 (1): 257-265, 2017.
APA | TIRINK C (2022). Comparison of Bayesian Regularized Neural Network, Random Forest Regression, Support Vector Regression and Multivariate Adaptive Regression Splines Algorithms to Predict Body Weight from Biometrical Measurements in Th alli Sheep. , 411 - 419. 10.9775/kvfd.2022.27164 |
Chicago | TIRINK CEM Comparison of Bayesian Regularized Neural Network, Random Forest Regression, Support Vector Regression and Multivariate Adaptive Regression Splines Algorithms to Predict Body Weight from Biometrical Measurements in Th alli Sheep. (2022): 411 - 419. 10.9775/kvfd.2022.27164 |
MLA | TIRINK CEM Comparison of Bayesian Regularized Neural Network, Random Forest Regression, Support Vector Regression and Multivariate Adaptive Regression Splines Algorithms to Predict Body Weight from Biometrical Measurements in Th alli Sheep. , 2022, ss.411 - 419. 10.9775/kvfd.2022.27164 |
AMA | TIRINK C Comparison of Bayesian Regularized Neural Network, Random Forest Regression, Support Vector Regression and Multivariate Adaptive Regression Splines Algorithms to Predict Body Weight from Biometrical Measurements in Th alli Sheep. . 2022; 411 - 419. 10.9775/kvfd.2022.27164 |
Vancouver | TIRINK C Comparison of Bayesian Regularized Neural Network, Random Forest Regression, Support Vector Regression and Multivariate Adaptive Regression Splines Algorithms to Predict Body Weight from Biometrical Measurements in Th alli Sheep. . 2022; 411 - 419. 10.9775/kvfd.2022.27164 |
IEEE | TIRINK C "Comparison of Bayesian Regularized Neural Network, Random Forest Regression, Support Vector Regression and Multivariate Adaptive Regression Splines Algorithms to Predict Body Weight from Biometrical Measurements in Th alli Sheep." , ss.411 - 419, 2022. 10.9775/kvfd.2022.27164 |
ISNAD | TIRINK, CEM. "Comparison of Bayesian Regularized Neural Network, Random Forest Regression, Support Vector Regression and Multivariate Adaptive Regression Splines Algorithms to Predict Body Weight from Biometrical Measurements in Th alli Sheep". (2022), 411-419. https://doi.org/10.9775/kvfd.2022.27164 |
APA | TIRINK C (2022). Comparison of Bayesian Regularized Neural Network, Random Forest Regression, Support Vector Regression and Multivariate Adaptive Regression Splines Algorithms to Predict Body Weight from Biometrical Measurements in Th alli Sheep. Kafkas Üniversitesi Veteriner Fakültesi Dergisi, 28(3), 411 - 419. 10.9775/kvfd.2022.27164 |
Chicago | TIRINK CEM Comparison of Bayesian Regularized Neural Network, Random Forest Regression, Support Vector Regression and Multivariate Adaptive Regression Splines Algorithms to Predict Body Weight from Biometrical Measurements in Th alli Sheep. Kafkas Üniversitesi Veteriner Fakültesi Dergisi 28, no.3 (2022): 411 - 419. 10.9775/kvfd.2022.27164 |
MLA | TIRINK CEM Comparison of Bayesian Regularized Neural Network, Random Forest Regression, Support Vector Regression and Multivariate Adaptive Regression Splines Algorithms to Predict Body Weight from Biometrical Measurements in Th alli Sheep. Kafkas Üniversitesi Veteriner Fakültesi Dergisi, vol.28, no.3, 2022, ss.411 - 419. 10.9775/kvfd.2022.27164 |
AMA | TIRINK C Comparison of Bayesian Regularized Neural Network, Random Forest Regression, Support Vector Regression and Multivariate Adaptive Regression Splines Algorithms to Predict Body Weight from Biometrical Measurements in Th alli Sheep. Kafkas Üniversitesi Veteriner Fakültesi Dergisi. 2022; 28(3): 411 - 419. 10.9775/kvfd.2022.27164 |
Vancouver | TIRINK C Comparison of Bayesian Regularized Neural Network, Random Forest Regression, Support Vector Regression and Multivariate Adaptive Regression Splines Algorithms to Predict Body Weight from Biometrical Measurements in Th alli Sheep. Kafkas Üniversitesi Veteriner Fakültesi Dergisi. 2022; 28(3): 411 - 419. 10.9775/kvfd.2022.27164 |
IEEE | TIRINK C "Comparison of Bayesian Regularized Neural Network, Random Forest Regression, Support Vector Regression and Multivariate Adaptive Regression Splines Algorithms to Predict Body Weight from Biometrical Measurements in Th alli Sheep." Kafkas Üniversitesi Veteriner Fakültesi Dergisi, 28, ss.411 - 419, 2022. 10.9775/kvfd.2022.27164 |
ISNAD | TIRINK, CEM. "Comparison of Bayesian Regularized Neural Network, Random Forest Regression, Support Vector Regression and Multivariate Adaptive Regression Splines Algorithms to Predict Body Weight from Biometrical Measurements in Th alli Sheep". Kafkas Üniversitesi Veteriner Fakültesi Dergisi 28/3 (2022), 411-419. https://doi.org/10.9775/kvfd.2022.27164 |