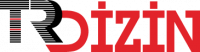
Diagnostic Performance of Machine Learning Models Based on 18 F-FDG PET/CT Radiomic Features in the Classification of Solitary Pulmonary Nodules
Yıl: 2022 Cilt: 31 Sayı: 2 Sayfa Aralığı: 82 - 88 Metin Dili: İngilizce DOI: 10.4274/mirt.galenos.2021.43760 İndeks Tarihi: 20-07-2022
Diagnostic Performance of Machine Learning Models Based on 18 F-FDG PET/CT Radiomic Features in the Classification of Solitary Pulmonary Nodules
Öz: Objectives: This study aimed to evaluate the ability of 18fluorine-fluorodeoxyglucose (18F-FDG) positron emission tomography/computed
tomography (PET/CT) radiomic features combined with machine learning methods to distinguish between benign and malignant solitary
pulmonary nodules (SPN).
Methods: Data of 48 patients with SPN detected on18F-FDG PET/CT scan were evaluated retrospectively. The texture feature extraction from PET/
CT images was performed using an open-source application (LIFEx). Deep learning and classical machine learning algorithms were used to build
the models. Final diagnosis was confirmed by pathology and follow-up was accepted as the reference. The performances of the models were
assessed by the following metrics: Sensitivity, specificity, accuracy, and area under the receiver operator characteristic curve (AUC).
Results: The predictive models provided reasonable performance for the differential diagnosis of SPNs (AUCs ~0.81). The accuracy and AUC of
the radiomic models were similar to the visual interpretation. However, when compared to the conventional evaluation, the sensitivity of the deep
learning model (88% vs. 83%) and specificity of the classic learning model were higher (86% vs. 79%).
Conclusion: Machine learning based on 18F-FDG PET/CT texture features can contribute to the conventional evaluation to distinguish between
benign and malignant lung nodules
Anahtar Kelime: Soliter Pulmoner Nodüllerin Sınıflandırılmasında 18 F-FDG PET/BT Radyomik Özelliklerine Dayalı Makine Öğrenme Modellerinin Tanısal Performansı
Öz: Amaç: Bu çalışmada, 18flor-florodeoksiglukoz (18F-FDG) pozitron emisyon tomografisi/bilgisayarlı tomografi (PET/BT) radyomik özelliklerinin makine
öğrenme yöntemleriyle birleştirilmesinin benign ve malign soliter pulmoner nodülleri (SPN) ayırt etme yeteneğini değerlendirmeyi amaçladık.
Yöntem: 18F-FDG PET/BT taramasında SPN saptanan 48 hastanın verileri geriye dönük olarak değerlendirildi. PET/BT görüntülerinden doku özelliği
çıkarımı, açık kaynaklı bir uygulama (LIFEx) kullanılarak yapıldı. Modelleri oluşturmak için derin öğrenme ve klasik makine öğrenme algoritmalarıkullanıldı. Patoloji ve izlem ile kesinleşen tanı referans olarak kabul edildi. Modellerin performansları şu metriklerle değerlendirildi: Duyarlılık,
özgüllük, doğruluk ve alıcı operatör özellikleri eğrisi altındaki alan (EAA).
Bulgular: Tahmine dayalı modeller, SPN’lerin ayırıcı tanısı için makul performans sağlandı (EAA’ler ~0,81). Radyomik modellerin doğruluğu ve
EAA’sı görsel yorumlamaya benzerdi. Ancak geleneksel değerlendirme ile karşılaştırıldığında, derin öğrenme modelinin duyarlılığı (%88’e karşı
%83) ve klasik öğrenme modelinin özgüllüğü (%86’ya karşı %79) daha yüksekti.
Sonuç: 18F-FDG PET/BT doku özelliklerine dayalı makine öğrenimi, iyi huylu ve kötü huylu akciğer nodüllerini ayırt etmek için geleneksel
değerlendirmeye katkıda bulunabili
Anahtar Kelime: Belge Türü: Makale Makale Türü: Araştırma Makalesi Erişim Türü: Erişime Açık
- 1. Siegel RL, Miller KD, Jemal A. Cancer statistics, 2019. CA Cancer J Clin 2019;69:7-34.
- 2. Nasim F, Ost DE. Management of the solitary pulmonary nodule. Curr Opin Pulm Med 2019;25:344-353.
- 3. Bai C, Choi CM, Chu CM, Anantham D, Chung-Man Ho J, Khan AZ, Lee JM, Li SY, Saenghirunvattana S, Yim A. Evaluation of pulmonary nodules: clinical practice consensus guidelines for Asia. Chest 2016;150:877-893.
- 4. MacMahon H, Naidich DP, Goo JM, Lee KS, Leung ANC, Mayo JR, Mehta AC, Ohno Y, Powell CA, Prokop M, Rubin GD, Schaefer-Prokop CM, Travis WD, Van Schil PE, Bankier AA. Guidelines for management of incidental pulmonary nodules detected on CT images: from the Fleischner Society 2017. Radiology 2017;284:228-243.
- 5. Jia Y, Gong W, Zhang Z, Tu G, Li J, Xiong F, Hou H, Zhang Y, Wu M, Zhang L. Comparing the diagnostic value of 18F-FDG-PET/CT versus CT for differentiating benign and malignant solitary pulmonary nodules: a meta-analysis. J Thorac Dis 2019;11:2082-2098.
- 6. Divisi D, Barone M, Bertolaccini L, Zaccagna G, Gabriele F, Crisci R. Diagnostic performance of fluorine-18 fluorodeoxyglucose positron emission tomography in the management of solitary pulmonary nodule: a meta-analysis. J Thorac Dis 2018;10(Suppl 7):S779-S789.
- 7. Zwanenburg A. Radiomics in nuclear medicine: robustness, reproducibility, standardization, and how to avoid data analysis traps and replication crisis. Eur J Nucl Med Mol Imaging 2019;46:2638-2655.
- 8. Mayerhoefer ME, Materka A, Langs G, Häggström I, Szczypiński P, Gibbs P, Cook G. Introduction to Radiomics. J Nucl Med 2020;61:488-495.
- 9. Hatt M, Cheze Le Rest C, Antonorsi N, Tixier F, Tankyevych O, Jaouen V, Lucia F, Bourbonne V, Schick U, Badic B, Visvikis D. Radiomics in PET/ CT: current status and future AI-based evolutions. Semin Nucl Med 2021;51:126-133.
- 10. Piñeiro-Fiel M, Moscoso A, Pubul V, Ruibal Á, Silva-Rodríguez J, Aguiar P. A Systematic review of pet textural analysis and radiomics in cancer. Diagnostics (Basel) 2021;11:380.
- 11. Bianconi F, Palumbo I, Spanu A, Nuvoli S, Fravolini ML, Palumbo B. PET/ CT radiomics in lung cancer: an overview. Appl Sci 2020;10:1718.
- 12. Manafi-Farid R, Karamzade-Ziarati N, Vali R, Mottaghy FM, Beheshti M. 2-[(18)F]FDG PET/CT radiomics in lung cancer: an overview of the technical aspect and its emerging role in management of the disease. Methods 2021;188:84-97.
- 13. Krarup MMK, Krokos G, Subesinghe M, Nair A, Fischer BM. Artificial intelligence for the characterization of pulmonary nodules, lung tumors and mediastinal nodes on PET/CT. Semin Nucl Med 2021;51:143-156.
- 14. Avanzo M, Stancanello J, Pirrone G, Sartor G. Radiomics and deep learning in lung cancer. Strahlenther Onkol 2020;196:879-887.
- 15. Chen S, Harmon S, Perk T, Li X, Chen M, Li Y, Jeraj R. Diagnostic classification of solitary pulmonary nodules using dual time 18F-FDG PET/CT image texture features in granuloma-endemic regions. Sci Rep 2017;7:9370.
- 16. Chen S, Harmon S, Perk T, Li X, Chen M, Li Y, Jeraj R. Using neighborhood gray tone difference matrix texture features on dual time point PET/ CT images to differentiate malignant from benign FDG-avid solitary pulmonary nodules. Cancer Imaging 2019;19:56.
- 17. Zhang J, Ma G, Cheng J, Song S, Zhang Y, Shi LQ. Diagnostic classification of solitary pulmonary nodules using support vector machine model based on 2-[18F]fluoro-2-deoxy-D-glucose PET/computed tomography texture features. Nucl Med Commun 2020;41:560-566.
- 18. Palumbo B, Bianconi F, Palumbo I, Fravolini ML, Minestrini M, Nuvoli S, Stazza ML, Rondini M, Spanu A. Value of shape and texture features from 18F-FDG PET/CT to discriminate between benign and malignant solitary pulmonary nodules: an experimental evaluation. Diagnostics (Basel) 2020;10:696.
- 19. Zhou Y, Ma XL, Zhang T, Wang J, Zhang T, Tian R. Use of radiomics based on 18F-FDG PET/CT and machine learning methods to aid clinical decision-making in the classification of solitary pulmonary lesions: an innovative approach. Eur J Nucl Med Mol Imaging 2021;48:2904-2913.
- 20. Cruickshank A, Stieler G, Ameer F. Evaluation of the solitary pulmonary nodule. Intern Med J 2019;49:306-315.
- 21. Nioche C, Orlhac F, Boughdad S, Reuzé S, Goya-Outi J, Robert C, Pellot- Barakat C, Soussan M, Frouin F, Buvat I. LIFEx: a freeware for radiomic feature calculation in multimodality imaging to accelerate advances in the characterization of tumor heterogeneity. Cancer Res 2018;78:4786- 4789.
- 22. Jeon H, Oh S. Hybrid-recursive feature elimination for efficient feature selection. Applied Sciences 2020;10:3211.
- 23. Taralli S, Scolozzi V, Foti M, Ricciardi S, Forcione AR, Cardillo G, Calcagni ML. 18F-FDG PET/CT diagnostic performance in solitary and multiple pulmonary nodules detected in patients with previous cancer history: reports of 182 nodules. Eur J Nucl Med Mol Imaging 2019;46:429-436.
- 24. Deppen SA, Blume JD, Kensinger CD, Morgan AM, Aldrich MC, Massion PP, Walker RC, McPheeters ML, Putnam JB Jr, Grogan EL. Accuracy of FDG-PET to diagnose lung cancer in areas with infectious lung disease: a meta-analysis. JAMA 2014;312:1227-1236.
- 25. Du D, Gu J, Chen X, Lv W, Feng Q, Rahmim A, Wu H, Lu L. Integration of PET/CT radiomics and semantic features for differentiation between active pulmonary tuberculosis and lung cancer. Mol Imaging Biol 2021;23:287-298.
- 26. Hu Y, Zhao X, Zhang J, Han J, Dai M. Value of 18F-FDG PET/CT radiomic features to distinguish solitary lung adenocarcinoma from tuberculosis. Eur J Nucl Med Mol Imaging 2021;48:231-240.
- 27. Nakajo M, Jinguji M, Aoki M, Tani A, Sato M, Yoshiura T. The clinical value of texture analysis of dual-time-point 18F-FDG-PET/CT imaging to differentiate between 18F-FDG-avid benign and malignant pulmonary lesions. Eur Radiol 2020;30:1759-1769.
- 28. Teramoto A, Tsujimoto M, Inoue T, Tsukamoto T, Imaizumi K, Toyama H, Saito K, Fujita H. Automated classification of pulmonary nodules through a retrospective analysis of conventional CT and two-phase PET images in patients undergoing biopsy. Asia Ocean J Nucl Med Biol 2019;7:29-37.
- 29. Vaidya M, Creach KM, Frye J, Dehdashti F, Bradley JD, El Naqa I. Combined PET/CT image characteristics for radiotherapy tumor response in lung cancer. Radiother Oncol 2012;102:239-245.
- 30. Oliver JA, Budzevich M, Zhang GG, Dilling TJ, Latifi K, Moros EG. Variability of image features computed from conventional and respiratory-gated PET/CT images of lung cancer. Transl Oncol 2015;8:524-534.
- 31. Grootjans W, Tixier F, van der Vos CS, Vriens D, Le Rest CC, Bussink J, Oyen WJ, de Geus-Oei LF, Visvikis D, Visser EP. The Impact of optimal respiratory gating and image noise on evaluation of intratumor heterogeneity on 18F-FDG PET imaging of lung cancer. J Nucl Med 2016;57:1692-1698.
- 32. Lambin P, Leijenaar RTH, Deist TM, Peerlings J, de Jong EEC, van Timmeren J, Sanduleanu S, Larue RTHM, Even AJG, Jochems A, van Wijk Y, Woodruff H, van Soest J, Lustberg T, Roelofs E, van Elmpt W, Dekker A, Mottaghy FM, Wildberger JE, Walsh S. Radiomics: the bridge between medical imaging and personalized medicine. Nat Rev Clin Oncol 2017;14:749-762.
- 33. Wang H, Zhou Z, Li Y, Chen Z, Lu P, Wang W, Liu W, Yu L. Comparison of machine learning methods for classifying mediastinal lymph node metastasis of non-small cell lung cancer from 18F-FDG PET/CT images. EJNMMI Res 2017;7:11
APA | Salihoğlu Y, USLU ERDEMİR R, PÜREN B, OZDEMİR S, UYULAN Ç, ERGÜZEL T, TEKİN H (2022). Diagnostic Performance of Machine Learning Models Based on 18 F-FDG PET/CT Radiomic Features in the Classification of Solitary Pulmonary Nodules. , 82 - 88. 10.4274/mirt.galenos.2021.43760 |
Chicago | Salihoğlu Yavuz Sami,USLU ERDEMİR Rabiye,PÜREN Büşra Aydur,OZDEMİR Semra,UYULAN Çağlar,ERGÜZEL Türker Tekin,TEKİN Hüseyin Ozan Diagnostic Performance of Machine Learning Models Based on 18 F-FDG PET/CT Radiomic Features in the Classification of Solitary Pulmonary Nodules. (2022): 82 - 88. 10.4274/mirt.galenos.2021.43760 |
MLA | Salihoğlu Yavuz Sami,USLU ERDEMİR Rabiye,PÜREN Büşra Aydur,OZDEMİR Semra,UYULAN Çağlar,ERGÜZEL Türker Tekin,TEKİN Hüseyin Ozan Diagnostic Performance of Machine Learning Models Based on 18 F-FDG PET/CT Radiomic Features in the Classification of Solitary Pulmonary Nodules. , 2022, ss.82 - 88. 10.4274/mirt.galenos.2021.43760 |
AMA | Salihoğlu Y,USLU ERDEMİR R,PÜREN B,OZDEMİR S,UYULAN Ç,ERGÜZEL T,TEKİN H Diagnostic Performance of Machine Learning Models Based on 18 F-FDG PET/CT Radiomic Features in the Classification of Solitary Pulmonary Nodules. . 2022; 82 - 88. 10.4274/mirt.galenos.2021.43760 |
Vancouver | Salihoğlu Y,USLU ERDEMİR R,PÜREN B,OZDEMİR S,UYULAN Ç,ERGÜZEL T,TEKİN H Diagnostic Performance of Machine Learning Models Based on 18 F-FDG PET/CT Radiomic Features in the Classification of Solitary Pulmonary Nodules. . 2022; 82 - 88. 10.4274/mirt.galenos.2021.43760 |
IEEE | Salihoğlu Y,USLU ERDEMİR R,PÜREN B,OZDEMİR S,UYULAN Ç,ERGÜZEL T,TEKİN H "Diagnostic Performance of Machine Learning Models Based on 18 F-FDG PET/CT Radiomic Features in the Classification of Solitary Pulmonary Nodules." , ss.82 - 88, 2022. 10.4274/mirt.galenos.2021.43760 |
ISNAD | Salihoğlu, Yavuz Sami vd. "Diagnostic Performance of Machine Learning Models Based on 18 F-FDG PET/CT Radiomic Features in the Classification of Solitary Pulmonary Nodules". (2022), 82-88. https://doi.org/10.4274/mirt.galenos.2021.43760 |
APA | Salihoğlu Y, USLU ERDEMİR R, PÜREN B, OZDEMİR S, UYULAN Ç, ERGÜZEL T, TEKİN H (2022). Diagnostic Performance of Machine Learning Models Based on 18 F-FDG PET/CT Radiomic Features in the Classification of Solitary Pulmonary Nodules. Molecular Imaging and Radionuclide Therapy, 31(2), 82 - 88. 10.4274/mirt.galenos.2021.43760 |
Chicago | Salihoğlu Yavuz Sami,USLU ERDEMİR Rabiye,PÜREN Büşra Aydur,OZDEMİR Semra,UYULAN Çağlar,ERGÜZEL Türker Tekin,TEKİN Hüseyin Ozan Diagnostic Performance of Machine Learning Models Based on 18 F-FDG PET/CT Radiomic Features in the Classification of Solitary Pulmonary Nodules. Molecular Imaging and Radionuclide Therapy 31, no.2 (2022): 82 - 88. 10.4274/mirt.galenos.2021.43760 |
MLA | Salihoğlu Yavuz Sami,USLU ERDEMİR Rabiye,PÜREN Büşra Aydur,OZDEMİR Semra,UYULAN Çağlar,ERGÜZEL Türker Tekin,TEKİN Hüseyin Ozan Diagnostic Performance of Machine Learning Models Based on 18 F-FDG PET/CT Radiomic Features in the Classification of Solitary Pulmonary Nodules. Molecular Imaging and Radionuclide Therapy, vol.31, no.2, 2022, ss.82 - 88. 10.4274/mirt.galenos.2021.43760 |
AMA | Salihoğlu Y,USLU ERDEMİR R,PÜREN B,OZDEMİR S,UYULAN Ç,ERGÜZEL T,TEKİN H Diagnostic Performance of Machine Learning Models Based on 18 F-FDG PET/CT Radiomic Features in the Classification of Solitary Pulmonary Nodules. Molecular Imaging and Radionuclide Therapy. 2022; 31(2): 82 - 88. 10.4274/mirt.galenos.2021.43760 |
Vancouver | Salihoğlu Y,USLU ERDEMİR R,PÜREN B,OZDEMİR S,UYULAN Ç,ERGÜZEL T,TEKİN H Diagnostic Performance of Machine Learning Models Based on 18 F-FDG PET/CT Radiomic Features in the Classification of Solitary Pulmonary Nodules. Molecular Imaging and Radionuclide Therapy. 2022; 31(2): 82 - 88. 10.4274/mirt.galenos.2021.43760 |
IEEE | Salihoğlu Y,USLU ERDEMİR R,PÜREN B,OZDEMİR S,UYULAN Ç,ERGÜZEL T,TEKİN H "Diagnostic Performance of Machine Learning Models Based on 18 F-FDG PET/CT Radiomic Features in the Classification of Solitary Pulmonary Nodules." Molecular Imaging and Radionuclide Therapy, 31, ss.82 - 88, 2022. 10.4274/mirt.galenos.2021.43760 |
ISNAD | Salihoğlu, Yavuz Sami vd. "Diagnostic Performance of Machine Learning Models Based on 18 F-FDG PET/CT Radiomic Features in the Classification of Solitary Pulmonary Nodules". Molecular Imaging and Radionuclide Therapy 31/2 (2022), 82-88. https://doi.org/10.4274/mirt.galenos.2021.43760 |