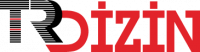
Yapay Sinir Ağlarıyla Moleküler Yapıdan Reorganizasyon Enerjisi Tayini
Proje Grubu: KBAG Sayfa Sayısı: 34 Proje No: 216Z096 Proje Bitiş Tarihi: 15.09.2019 Metin Dili: Türkçe İndeks Tarihi: 27-03-2020
Yapay Sinir Ağlarıyla Moleküler Yapıdan Reorganizasyon Enerjisi Tayini
Öz: Bu projede yapay sinir ağları gibi makine öğrenmesi teknikleri kullanılarak, moleküler yapılardan reorganizasyon enerjilerinin (RE) tahmini hedeflenmiş ve yapılan çalışmalarla bunun mümkün olduğu gösterilmiştir. Reorganizasyon enerjisi organik yarı iletkenlerde yük transfer hızlarını belirleyen önemli faktörlerden bir tanesi ve moleküler seviyede tarama yapmak için uygun bir parametredir. RE'nin kuantum kimyasal yöntemlerle hesaplanması büyük ölçekli taramalar için pahalı olduğundan, makine öğrenim yöntemleri ile RE'nin moleküler yapıdan tahmin edilme olasılığını araştırdık. Kombinatoryel metotlarla, benzen, tiyofen, furan, pirol, piridin, piridazin ve siklopentadien gibi halkaları kullanarak konjuge omurgalara sahip 5631 molekülden oluşan bir moleküler veri seti oluşturduk ve 6-31G(d) atomik baz setlerini B3LYP teorisi ile birlikte kullanarak hedef RE değerlerini elde ettik. RE tahmini için Ridge, Kernel Ridge ve derin sinir ağı (DSA) regresyon modellerini, moleküllerin çizge (graph) ve geometriye dayalı tanımlayıcıları ile geliştirdik. DSA'ların diğer metotlardan daha iyi performans gösterdiğini ve RE'nin R^2=0.92'lik bir belirleme katsayısı ve yaklaşık 12 meV'luk bir ortalama hata ile tahmin edilebileceğini gösterdik. Sonuç olarak projemiz kapsamında geliştirilen metodoloji sayesinde basit bir moleküler yapı şemasından veya moleküler mekanik metotları ile hızlıca elde edilebilecek moleküler geometrilerden, kuantum kimyasal hesaplar yapılmadan, reorganizasyon enerjilerinin tahmin edilebileceği gösterilmiştir.
Anahtar Kelime: Konular:
Erişim Türü: Erişime Açık
- Atahan-Evrenk, S. (2018). “A quantitative structure-property study of reorganization energy for known p-type organic semiconductors”. RSC Advances, 8(70):40330–40337.
- Prediction of the reorganization energy from molecular structure: A data approach (Bildiri - Uluslararası Bildiri - Poster Sunum)
- Balabin, R. M. ve Lomakina, E. I. (2009). “Neural network approach to quantum-chemistry data: Accurate prediction of density functional theory energies.” Journal of Chemical Physics, 131(7):074104.
- Bartók, A. P., De, S., Poelking, C., Bernstein, N., Kermode, J. R., Csányi, G., ve Ceriotti, M. (2017). “Machine learning unifies the modeling of materials and molecules”. Science Advan- ces, 3(12):e1701816.
- Becke, A. D. (1993). “Density-functional thermochemistry. III. the role of exact exchange.” Journal of Chemical Physics, 98:5648–5652.
- Behler, J. (2011). “Neural network potential-energy surfaces in chemistry: a tool for large-scale simulations.” Physical Chemistry Chemical Physics, 13:17930–17955.
- Behler, J. (2016). “Perspective: Machine learning potentials for atomistic simulations”. Journal of Chemical Physics, 145:170901.
- Bishop, C. M. (1995). Neural Networks for Pattern Recognition. Oxford University Press, New York, 1 edition.
- Butler, K. T., Davies, D. W., Cartwright, H., Isayev, O., ve Walsh, A. (2018). “Machine learning for molecular and materials science”. Nature, 559:547–555.
- Collins, C. R., Gordon, G. J., von Lilienfeld, O. A., ve Yaron, D. J. (2018). “Constant size descriptors for accurate machine learning models of molecular properties.” Journal of Chemical Physics, 148(24):241718.
- Coropceanu, V., Cornil, J., Filho, D. S., A, D., Olivier, Y., Silbey, R., ve Brédas, J.-L. (2007). “Charge transport in organic semiconductors”. Chemical Reviews, 107:926–952.
- Duvenaud, D., Maclaurin, D., Aguilera-Iparraguirre, J., Gómez-Bombarelli, R., Hirzel, T., Aspuru- Guzik, A., ve Adams, R. P. (2015). “Convolutional Networks on Graphs for Learning Molecular Fingerprints”. NIPS’15 Proceedings of the 28th International Conference on Neural Information Processing Systems, 2:2224–2232.
- Er, S., Suh, C., Marshak, M. P., ve Aspuru-Guzik, A. (2015). “Computational design of molecules for an all-quinone redox flow battery.” Chemical Science, 6:885–893.
- Faber, F. A., Christensen, A. S., Huang, B., ve Von Lilienfeld, O. A. (2018). “Alchemical and st- ructural distribution based representation for universal quantum machine learning”. Journal of Chemical Physics, 148:241717.
- Faber, F. A., Hutchison, L., Huang, B., Gilmer, J., Schoenholz, S. S., Dahl, G. E., Vinyals, O., Kearnes, S., Riley, P. F., ve Von Lilienfeld, O. A. (2017). “Prediction Errors of Molecular Machine Learning Models Lower than Hybrid DFT Error”. Journal of Chemical Theory and Computation, 13:5255–5264.
- Faulon, J.-L., Visco, D. P., ve Pophale, R. S. (2003). “The signature molecular descriptor. 1. using extended valence sequences in qsar and qspr studies.” Journal Chemical Information and Com- puter Sciences, 43(3):707–720.
- Feng, X., Marcon, V., Pisula, W., Hansen, M. R., Kirkpatrick, J., Grozema, F., Andrienko, D., Kremer, K., ve Müllen, K. (2009). “Towards high charge-carrier mobilities by rational design of the shape and periphery of discotics”. Nature Materials., 8:421–426.
- Francl, M. M., Pietro, W. J., Hehre, W. J., Binkley, J. S., Gordon, M. S., Defrees, D. J., ve Pople, J. A. (1982). “Self-consistent molecular orbital methods. xxiii. a polarization-type basis set for second-row elements.” Journal of Chemical Physics, 77:3654–3665.
- Goh, G. B., Hodas, N. O., ve Vishnu, A. (2017). “Deep learning for computational chemistry.” Journal of Computational Chemistry, 38(16):1291–1307.
- Gomez-Bombarelli, R., Aguilera-Iparraguirre, J., Hirzel, T. D., Duvenaud, D., Maclaurin, D., Blood- Forsythe, M. A., Chae, H. S., Einzinger, M., Ha, D.-G., Wu, T., Markopoulos, G., Jeon, S., Kang, H., Miyazaki, H., Numata, M., Kim, S., Huang, W., Hong, S. I., Baldo, M., Adams, R. P., ve Aspuru-Guzik, A. (2016). “Design of efficient molecular organic light-emitting diodes by a high- throughput virtual screening and experimental approach.” Nature Materials, 15(10):1120–1127.
- Gómez-Bombarelli, R., Wei, J. N., Duvenaud, D., Hernández-Lobato, J. M., Sánchez-Lengeling, B., Sheberla, D., Aguilera-Iparraguirre, J., Hirzel, T. D., Adams, R. P., ve Aspuru-Guzik, A. (2018). “Automatic Chemical Design Using a Data-Driven Continuous Representation of Molecules”. ACS Central Science, 4:268–276.
- Gryn’ova, G., Lin, K.-H., ve Corminboeuf, C. (2018). “Read between the molecules: Computational insights into organic semiconductors.” Journal of American Chemical Society, 140(48):16370– 16386.
- Hachmann, J., Olivares-Amaya, R., Atahan-Evrenk, S., Amador-Bedolla, C., Sánchez-Carrera, R. S., Gold-Parker, A., Vogt, L., Brockway, A. M., ve Aspuru-Guzik, A. (2011). “The harvard clean energy project: Large-scale computational screening and design of organic photovoltaics on the world community grid.” Journal of Physical Chemistry Letters, 2(17):2241–2251.
- Halgren, T. A. (1996).Science Advances “Merck molecular force field. iii. molecular geometries and vibrational frequencies for mmff94.” Journal of Computational Chemistry, 17(5-6):553–586.
- Hansen, K., Montavon, G., Biegler, F., Fazli, S., Rupp, M., Scheffler, M., von Lilienfeld, O. A., Tkatc- henko, A., ve Muller, K.-R. (2013). “Assessment and validation of machine learning methods for predicting molecular atomization energies.” Journal of Chemical Theory and Computation, 9(8):3404–3419.
- Hariharan, P. C. and Pople, J. A. (1973). “The influence of polarization functions on molecular orbital hydrogenation energies.” Theoretica Chimica Acta, 28:213–222.
- Heeger, A. J. (2014). “25th Anniversary Article: Bulk Heterojunction Solar Cells: Understanding the Mechanism of Operation.” Advanced Materials, 26(1):10–28.
- Hehre, W. J., Ditchfield, R., ve Pople, J. A. (1972). “Self-consistent molecular orbital methods. xii. further extensions of gaussian-type basis sets for use in molecular orbital studies of organic molecules.” Journal of Chemical Physics, 56:2257–2261.
- Huang, B. ve von Lilienfeld, O. A. (2016). “Communication: Understanding molecular represen- tations in machine learning: The role of uniqueness and target similarity.” Journal of Chemical Physics, 145(16):161102.
- Jain, A., Ong, S. P., Hautier, G., Chen, W., Richards, W. D., Dacek, S., Cholia, S., Gunter, D., Skinner, D., Ceder, G., ve Persson, K. a. (2013). “The Materials Project: A materials genome approach to accelerating materials innovation.” APL Materials, 1(1):011002.
- Janet, J. P. ve Kulik, H. J. (2017). “Predicting electronic structure properties of transition metal complexes with neural networks.” Chemical Science, 8:5137–5152.
- Kanal, I. Y., Owens, S. G., Bechtel, J. S., ve Hutchison, G. R. (2013). “Efficient computational screening of organic polymer photovoltaics.” Journal of Physical Chemistry Letters, 4(10):1613– 1623.
- Kim, Y., Chortos, A., Xu, W., Liu, Y., Oh, J. Y., Son, D., Kang, J., Foudeh, A. M., Zhu, C., Lee, Y., Niu, S., Liu, J., Pfattner, R., Bao, Z., ve Lee, T.-W. (2018). “A bioinspired flexible organic artificial afferent nerve.” Science, 360(6392):998–1003.
- Kong Lee, C., Moix, J., ve Cao, J. (2015). “Theory of coherent resonance energy transfer.” Journal of Chemical Physics, 142:164103.
- Kwiatkowski, J. J., Frost, J. M., ve Nelson, J. (2009). “The effect of morphology on electron field- effect mobility in disordered C60 thin films.” Nano Letters, 9:1085–1090.
- Le, T., Epa, V. C., Burden, F. R., ve Winkler, D. A. (2012). “Quantitative structure-property relati- onship modeling of diverse materials properties.” Chemical Reviews, 112(5):2889–2919.
- Lederer, J., Kaiser, W., Mattoni, A., ve Gagliardi, A. (2019). “Machine Learning-Based Charge Transport Computation for Pentacene.” Advanced Theory and Simulation, 2(2):1800136.
- Lee, C., Yang, W., ve Parr, R. G. (1988). “Development of the Colle-Salvetti correlation-energy formula into a functional of the electron density.” Physical Reviews B, 37:785–789.
- Li, H., Shi, W., Song, J., Jang, H.-J., Dailey, J., Yu, J., ve Katz, H. E. (2019). “Chemical and Biomolecule Sensing with Organic Field-Effect Transistors.” Chemical Reviews, 119(1):3–35.
- Ma, J., Sheridan, R. P., Liaw, A., Dahl, G. E., ve Svetnik, V. (2015). “Deep neural nets as a method for quantitative structure-activity relationships.” Journal of Chemical Information and Modeling, 55:263–274.
- McMahon, D. P. and Troisi, A. (2010). “Evaluation of the external reorganization energy of polya- cenes.” Journal of Physical Chemistry Letters, 1(6):941–946.
- Misra, M., Andrienko, D., Baumeier, B., Faulon, J.-L., and von Lilienfeld, O. A. (2011). “Toward quantitative structure–property relationships for charge transfer rates of polycyclic aromatic hyd- rocarbons.” Journal of Chemical Theory and Computation, 7(8):2549–2555.
- Morgan, H. L. (1965). “The Generation of a Unique Machine Description for Chemical Structures— A Technique Developed at Chemical Abstracts Service.” Journal of Chemical Documentation, 5(2):107–113.
- Musil, F., De, S., Yang, J., Campbell, J. E., Day, G. M., ve Ceriotti, M. (2018). “Machine learning for the structure-energy-property landscapes of molecular crystals.” Chemical Science, 9:1289– 1300.
- Oberhofer, H., Reuter, K., ve Blumberger, J. (2017). “Charge Transport in Molecular Materials: An Assessment of Computational Methods.” Chemical Reviews, 117:10319–10357.
- Olivares-Amaya, R., Amador-Bedolla, C., Hachmann, J., Atahan-Evrenk, S., Sánchez-Carrera, R. S., Vogt, L., ve Aspuru-Guzik, A. (2011). “Accelerated computational discovery of high- performance materials for organic photovoltaics by means of cheminformatics.” Energy and Environmental Science, 4:4849–4861.
- Padula, D., Simpson, J. D., ve Troisi, A. (2019). “Combining electronic and structural features in machine learning models to predict organic solar cells properties.” Materials Horizons, 6:343– 349.
- Pyzer-Knapp, E. O., Li, K., ve Aspuru-Guzik, A. (2015a). “Learning from the harvard clean energy project: The use of neural networks to accelerate materials discovery.” Advanced Functional Materials, 25(41):6495–6502.
- Pyzer-Knapp, E. O., Suh, C., Gomez-Bombarelli, R., Aguilera-Iparraguirre, J., ve Aspuru-Guzik, A. (2015b). “What is high-throughput virtual screening? a perspective from organic materials discovery.” Annual Reviews of Materials Research, 45(1):195–216.
- Qu, X., Jain, A., Rajput, N. N., Cheng, L., Zhang, Y., Ong, S. P., Brafman, M., Maginn, E., Curtiss, L. A., ve Persson, K. A. (2015). “The electrolyte genome project: A big data approach in battery materials discovery.” Computational Materials Science, 103:56–67.
- Raccuglia, P., Elbert, K. C., Adler, P. D. F., Falk, C., Wenny, M. B., Mollo, A., Zeller, M., Friedler, S. A., Schrier, J., ve Norquist, A. J. (2016). “Machine-learning-assisted materials discovery using failed experiments.” Nature, 533:73–76.
- Reimers, J. R. (2001). “A practical method for the use of curvilinear coordinates in calculations of normal-mode-projected displacements and duschinsky rotation matrices for large molecules.” Journal of Chemical Physics, 115(20):9103–9109.
- Rogers, D. and Hahn, M. (2010). “Extended-connectivity fingerprints.” Journal of Chemical Infor- mation and Modeling, 50(5):742–754.
- Rupp, M. (2015). “Machine learning for quantum mechanics in a nutshell.” International Journal of Quantum Chemistry, 115(16):1058–1073.
- Rupp, M., Tkatchenko, A., Müller, K. R., ve Von Lilienfeld, O. A. (2012). “Fast and accurate modeling of molecular atomization energies with machine learning.” Physical Review Letters, 108:058301.
- Rupp, M., Von Lilienfeld, O. A., ve Burke, K. (2018). “Guest Editorial: Special Topic on Data-Enabled Theoretical Chemistry.” Journal of Chemical Physics, 148:241401.
- Sahu, H., Rao, W., Troisi, A., ve Ma, H. (2018). “Toward predicting efficiency of organic solar cells via machine learning and improved descriptors.” Advanced Energy Materials, 8(24):1801032.
- Schober, C., Reuter, K., ve Oberhofer, H. (2016). “Virtual screening for high carrier mobility in organic semiconductors.” Journal of Physical Chemistry Letters, 7(19):3973–3977.
- Schütt, K. T., Arbabzadah, F., Chmiela, S., Müller, K. R., ve Tkatchenko, A. (2017). “Quantum- chemical insights from deep tensor neural networks.” Nature Communications, 8:13890.
- Schütt, K. T., Sauceda, H. E., Kindermans, P. J., Tkatchenko, A., ve Müller, K. R. (2018). “Sch- Net - A deep learning architecture for molecules and materials.” Journal of Chemical Physics, 148:241722.
- Sokolov, A. N., Atahan-Evrenk, S., Mondal, R., Akkerman, H. B., Sánchez-Carrera, R. S., Granados-Focil, S., Schrier, J., Mannsfeld, S. C. B., Zoombelt, A. P., Bao, Z., ve Aspuru-Guzik, A. (2011). “From computational discovery to experimental characterization of a high hole mobility organic crystal.” Nature Communications, 2:437.
- Soltzberg, L. J. and Wilkins, C. L. (1977). “Molecular Transforms: a Potential Tool for Structure- Activity Studies.” Journal of American Chemical Society, 2(99):439–443.
- Srivastava, N., Hinton, G., Krizhevsky, A., Sutskever, I., ve Salakhutdinov, R. (2014). “Dropout: A simple way to prevent neural networks from overfitting.” Journal of Machine Learning Research, 15:1929–1958.
- Subhas, A. V., Whealdon, J., ve Schrier, J. (2011). “Predicting organic thin-film transistor carrier type from single molecule calculations.” Computational Theoretical Chemistry, 966(1):70–74.
- Tang, M. L., Reichardt, A. D., Wei, P., ve Bao, Z. (2009). “Correlating carrier type with frontier molecular orbital energy levels in organic thin film transistors of functionalized acene derivatives.” Journal of American Chemical Society, 131(14):5264–5273.
- Vu, K., Snyder, J. C., Li, L., Rupp, M., Chen, B. F., Khelif, T., Müller, K.-R., ve Burke, K. (2015). “Understanding kernel ridge regression: Common behaviors from simple functions to density functionals.” International Journal of Quantum Chemistry, 115(16):1115–1128.
- Wang, S., Xu, J., Wang, W., Wang, G.-J. N., Rastak, R., Molina-Lopez, F., Chung, J. W., Niu, S., Feig, V. R., Lopez, J., Lei, T., Kwon, S.-K., Kim, Y., Foudeh, A. M., Ehrlich, A., Gasperini, A., Yun, Y., Murmann, B., Tok, J. B.-H., ve Bao, Z. (2018). “Skin electronics from scalable fabrication of an intrinsically stretchable transistor array.” Nature, 555(7694):83–88.
- Wei, J. N., Belanger, D., Adams, R. P., ve Sculley, D. (2019). “Rapid prediction of electron–ionization mass spectrometry using neural networks.” ACS Central Science, 5(4):700–708.
- Winter, R., Montanari, F., Noé, F., ve Clevert, D.-A. (2018). “Learning continuous and data-driven molecular descriptors by translating equivalent chemical representations.” Chemical Science, 10:1692–1701.
- Wu, Z., Ramsundar, B., Feinberg, E. N., Gomes, J., Geniesse, C., Pappu, A. S., Leswing, K., ve Pande, V. (2018). “MoleculeNet: A benchmark for molecular machine learning.” Chemical Science, 9:513–530.
- Yang, J., De, S., Campbell, J. E., Li, S., Ceriotti, M., ve Day, G. M. (2018a). “Large-scale com- putational screening of molecular organic semiconductors using crystal structure prediction.” Chemistry of Materials, 30(13):4361–4371.
- Yang, J., De, S., Campbell, J. E., Li, S., Ceriotti, M., ve Day, G. M. (2018b). “Large-Scale Com- putational Screening of Molecular Organic Semiconductors Using Crystal Structure Prediction.” Chemistry of Materials, 30:4361–4371.
- Yao, K., Herr, J. E., Toth, D. W., McKintyre, R., ve Parkhill, J. (2018). “The TensorMol-0.1 model chemistry: A neural network augmented with long-range physics.” Chemical Science, 9:2261– 2269.
- Yersin, H. H. (2019).Theoretica Chimica Acta Highly efficient OLEDs : materials based on thermally activated delayed fluorescence. Wiley-VCH Verlag GmbH & Co., Weinheim, Germany.
- Zhao, W., Li, S., Yao, H., Zhang, S., Zhang, Y., Yang, B., ve Hou, J. (2017). “Molecular optimization enables over 13% efficiency in organic solar cells.” Journal of American Chemical Society, 139(21):7148–7151.
- Zupan, J. and Gasteiger, J. (1999). Neural Networks in Chemistry and Drug Design. Wiley-VCH, 2 edition.
APA | ATAHAN EVRENK Ş, ATALAY SATOĞLU F (2019). Yapay Sinir Ağlarıyla Moleküler Yapıdan Reorganizasyon Enerjisi Tayini. , 1 - 34. |
Chicago | ATAHAN EVRENK Şule,ATALAY SATOĞLU Fatma Betül Yapay Sinir Ağlarıyla Moleküler Yapıdan Reorganizasyon Enerjisi Tayini. (2019): 1 - 34. |
MLA | ATAHAN EVRENK Şule,ATALAY SATOĞLU Fatma Betül Yapay Sinir Ağlarıyla Moleküler Yapıdan Reorganizasyon Enerjisi Tayini. , 2019, ss.1 - 34. |
AMA | ATAHAN EVRENK Ş,ATALAY SATOĞLU F Yapay Sinir Ağlarıyla Moleküler Yapıdan Reorganizasyon Enerjisi Tayini. . 2019; 1 - 34. |
Vancouver | ATAHAN EVRENK Ş,ATALAY SATOĞLU F Yapay Sinir Ağlarıyla Moleküler Yapıdan Reorganizasyon Enerjisi Tayini. . 2019; 1 - 34. |
IEEE | ATAHAN EVRENK Ş,ATALAY SATOĞLU F "Yapay Sinir Ağlarıyla Moleküler Yapıdan Reorganizasyon Enerjisi Tayini." , ss.1 - 34, 2019. |
ISNAD | ATAHAN EVRENK, Şule - ATALAY SATOĞLU, Fatma Betül. "Yapay Sinir Ağlarıyla Moleküler Yapıdan Reorganizasyon Enerjisi Tayini". (2019), 1-34. |
APA | ATAHAN EVRENK Ş, ATALAY SATOĞLU F (2019). Yapay Sinir Ağlarıyla Moleküler Yapıdan Reorganizasyon Enerjisi Tayini. , 1 - 34. |
Chicago | ATAHAN EVRENK Şule,ATALAY SATOĞLU Fatma Betül Yapay Sinir Ağlarıyla Moleküler Yapıdan Reorganizasyon Enerjisi Tayini. (2019): 1 - 34. |
MLA | ATAHAN EVRENK Şule,ATALAY SATOĞLU Fatma Betül Yapay Sinir Ağlarıyla Moleküler Yapıdan Reorganizasyon Enerjisi Tayini. , 2019, ss.1 - 34. |
AMA | ATAHAN EVRENK Ş,ATALAY SATOĞLU F Yapay Sinir Ağlarıyla Moleküler Yapıdan Reorganizasyon Enerjisi Tayini. . 2019; 1 - 34. |
Vancouver | ATAHAN EVRENK Ş,ATALAY SATOĞLU F Yapay Sinir Ağlarıyla Moleküler Yapıdan Reorganizasyon Enerjisi Tayini. . 2019; 1 - 34. |
IEEE | ATAHAN EVRENK Ş,ATALAY SATOĞLU F "Yapay Sinir Ağlarıyla Moleküler Yapıdan Reorganizasyon Enerjisi Tayini." , ss.1 - 34, 2019. |
ISNAD | ATAHAN EVRENK, Şule - ATALAY SATOĞLU, Fatma Betül. "Yapay Sinir Ağlarıyla Moleküler Yapıdan Reorganizasyon Enerjisi Tayini". (2019), 1-34. |