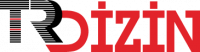
Forecasting Daily and Sessional Returns of the ISE-100 Index with Neural Network Models
Yıl: 2007 Cilt: 8 Sayı: 2 Sayfa Aralığı: 128 - 142 Metin Dili: Türkçe İndeks Tarihi: 29-07-2022
Forecasting Daily and Sessional Returns of the ISE-100 Index with Neural Network Models
Öz: Özellikle son on yılda yapay sinir ağları modelleri portföy oluşturma ve hisse senedi piyasası tahminleri gibi finansal problemleri çözmede uygulanmaktadır. Çeşitli yapay sinir ağları modelleri arasında, çok-katmanlı pörseptron modelleri finansal tahmin çalışmaları için yaygın ve etkili bir şekilde kullanılmaktadır. Bu çalışma, çok-katmanlı pörseptron modellerinin İMKB-100 endeksinin günlük ve seanslık getirilerinin tahmin edilmesindeki etkinliğini incelemektedir. Çalışmanın bulgularından yola çıkılarak, çok-katmanlı pörseptron modellerinin İMKB-100 endeks getirisini tahmin etmede umut vaat eden bir performans gösterdiği sonucuna varılabilir. Fakat, yapay sinir ağları modellerinin tahmin güçleri farklı değişkenler ve farklı model yapıları kullanılarak daha da arttırılabilir.
Anahtar Kelime: Konular:
Yapay Sinir Ağları Modelleri ile İMKB-100 Endeksinin Günlük ve Seanslık Getirilerinin Tahmin Edilmesi
Öz: Especially for the last decade, the neural network models have been applied to solve financial problems like portfolio construction and stock market forecasting. Among the alternative neural network models, the multilayer perceptron models are expected to be effective and widely applied in financial forecasting. This study examines the forecasting power multilayer perceptron models for daily and sessional returns of ISE-100 index. The findings imply that the multilayer perceptron models presented promising performance in forecasting the ISE-100 index returns. However, further emphasis should be placed on different input variables and model architectures in order to improve the forecasting performances.
Anahtar Kelime: Konular:
Belge Türü: Makale Makale Türü: Araştırma Makalesi Erişim Türü: Erişime Açık
- 1. ADYA, M. & COLLOPY, F. (1998). How effective are neural networks at forecasting and prediction? A review and evaluation. Journal of Forecasting, 17, pp. 487-495.
- 2. AKTAŞ, R., DOĞANAY, M. & YILDIZ, B. (2003). Mali başarısızlığın öngörülmesi: istatistiksel yöntemler ve yapay sinir ağı karşılaştırması, Ankara Üniversitesi SBF Dergisi, 58(4), pp. 1-25.
- 3. ALTAY, E. & SATMAN, M.H. (2005). Stock market forecasting: Artificial neural networks and linear regression comparison in an emerging market. Journal of Financial Management and Analysis, 18(2), pp. 18-33.
- 4. BENLİ, Y.K. (2005). Bankalarda mali başarısızlığın öngörülmesi lojistik regresyon ve yapay sinir ağı karşılaştırması. Gazi Üniversitesi Endüstriyel Sanatlar Eğitim Fakültesi Dergisi, 16, pp. 31-46
- 5. BOYACIOĞLU, M.A., KARA, Y. (2006) Türk bankacılık sektöründe finansal güç derecelerinin tahmininde yapay sinir ağları ve çok değişkenli istatistiksel analiz tekniklerinin performanslarının karşılaştırılması. 10. Ulusal Finans Sempozyumu, 01-04 Kasım 2006, İzmir.
- 6. BROWNSTONE, D. (1996). Using percentage accuracy to measure neural network predictions in stock market movements. Neurocomputing, 10, pp. 237-250.
- 7. CHANDRA, N. & REEB, D.M. (1999). Neural Networks in a Market Efficiency Context. American Business Review, January, pp. 39-44.
- 8. CYBENKO, G. (1989). Approximation by superpositions of a sigmoidal function. Mathematics of Control, Signal and Systems, 2, pp. 303-314.
- 9. DARRAT, A.F. & ZHONG, M. (2000). On testing the random -walk hypothesis: A model comparison approach. The Financial Review, 35, pp. 105-124.
- 10.DEBOECK, G.J. & CADER, M. (1994). Pre- and post-processing of financial data. In: Trading on the Edge: Neural, Genetic, and Fuzzy Systems for Chaotic Financial Markets, Deboeck, G.J. (Ed.), John Wiley & Sons Inc.,pp. 27-45.
- 11.DİLER, A.İ. (2003). İMKB Ulusal-100 endeksinin yönünün yapay sinir ağları hata geriye yayma yöntemi ile tahmin edilmesi, İMKB Dergisi, 25-26, pp. 65-81
- 12.EGELI, B., ÖZTURAN, M., & BADUR, B. (2003). Stock market prediction using artificial neural networks. Proceedings of the 3rd Hawaii International Conference on Business, Hawai, USA.
- 13.FADLALLA, A. & LIN, C.H. (2001). An analysis of the applications of neural networks in finance. Interfaces, 31, pp. 112-122.
- 14.GATELY, E. (1996). Neural Networks for Financial Forecasting, John Wiley & Sons, Inc.
- 15.GENCAY, R. (1996). Non-linear prediction of security returns with moving average rules. Journal of Forecasting, 15, pp. 165-174.
- 16.GENCAY, R.(1998). Optimisation of technical trading strategies and the profitability in the stock markets. Economic Letters, 59, pp. 249-254.
- 17.GENCAY, R.& STENGOS, T. (1998). Moving average rules, volume and the predicability of stock returns with feedforward networks, Journal of Forecasting, 17, pp. 401-141.
- 18.GÜNEŞ, H. & SALTOĞLU, B. (1998). İMKB getiri volatilitesinin makroekonomik konjonktür bağlamında irdelenmesi. Istanbul Stock Exchange Publilication.
- 19.HORNIK, K., STINCHCOMBE, M. WHITE, H. (1989). Multilayer feedforeward networks are universal approximators. Neural Networks, 2, pp. 359-366.
- 20.HORNIK, K. (1990). Universal approximation of an unknown mappings and its derivatives using multilayer feedforward neural networks. Neural Networks, 3, pp. 551-560.
- 21.JANG, G. & LAI, F. (1994). Intelligent Trading of an Emerging Market. In: Trading on The Edge, Deboeck, G.J.(Ed.), John Wiley & Sons Inc., pp. 80-101.
- 22.Istanbul Stock Exchange, Evaluation and Statistics Department (2005). Factbook 2005. Avaliable from: http://www.imkb.gov.tr/veri.htm [Last accessed January 20, 2006],
- 23.KAASTRA, I. & BOYD, M. (1996). Designing a neural network for forecasting financial and economic time series. Neurocomputing, 10, pp. 215-236.
- 24.KAMIJO, K. & TANIGAWA, T. (1990). Stock price pattern recognition: a recurrent neural network approach. Proceedings of the IEEE International Joint Conference on Neural Networks, pp. 215-221.
- 25.KARAATLI, M., GÜNGÖR, İ., DEMİR, Y. & KALAYCI, Ş. (2005). Hisse senedi fiyat hareketlerinin yapay sinir ağları yöntemi ile tahmin edilmesi. Balıkesir Üniversitesi İİBF Dergisi, 2(1), pp. 22-48.
- 26.KILIMASAUSKAS, C.,C. (1994). Neural Network Techniques, In: Trading on the Edge, Deboeck, G.J. (Ed.), John Wiley&Sons, Inc., pp. 1-26.
- 27.KIM, S.H. & CHUNG, S.H. (1998). Graded forecasting using array of bipolar predictions: application of probabilistic neural networks to a stock market index. International Journal of Forecasting, 14, pp. 323-337.
- 28.KIMOTO, T., ASAKAWA, K., YODA, M. & TAJEOKA, M. (1990). Stock market prediction system with modular neural networks. Proceedings of the IEEE International Joint Conference, on Neural Networks, pp. 1-16.
- 29.KOHARA, K, ISHIKAWA, T., FUKUHARA, Y. & NAKAMURA, Y. (1997). Stock price prediction using prior knowledge and neural networks. Intelligent Systems in Accounting, Finance and Management, 6, pp. 11-22.
- 30.KRYZANOWSKI, L., GALLER, M. & WRIGHT, D., W. (1993). Using artificial neural networks to pick stocks. Financial Analysts Journal, 49/4, pp. 21-27.
- 31.LAM, M. (2004). Neural Network techniques for financial performance prediction: integrating fundamental and technical analysis. Decision Support Systems, 37, pp. 565-581.
- 32.MAASOUMI, E. & RACINE, J. (2002). Entropy and predicability of stock market returns, Journal of Econometrics, 107, pp.291-312.
- 33.OLSON, D. & MOSSMAN, C. (2002). Neural network forecasts of Canadian stock returns using accounting ratios. International Journal of Forecasting, 1, 2002, pp.1-13.
- 34.OZCAM, M. (1997). An Analysis Of The Macroeconomic Factors That Determine The Stock Returns. Capital Market Board Of Turkey Publication, No.75.
- 35.PHUA, P.K.H., MING, D. & LIN, W. (2001). Neural network with genetically evolved algorithms for stock prediction. Asia-Pacific Journal of Operational Research, 18, pp. 103-107.
- 36.REFENES, A.P. (1995). Testing Strategies and Metrics. In: Neural Networks in the Capital Markets, Refenes, A.P. (Ed.), , John Wiley & Sons, Inc., pp. 67-76.
- 37.RODRIGUEZ, F.F., MARTEL, C., G. & RIVERO, S.S. (2000). On the profitability of technical trading rules based on artificial neural networks: evidence from Madrid stock market. Economic Letters, 69, pp. 89-94.
- 38.RODRIGUEZ, J.V., TORRA, S. & FELIX, J.A. (2005). STAR and ANN models: Forecasting performance on Spanish Ibex-35 stock index. Journal of Empirical Finance, 12/3, pp. 490-509.
- 39.SAAD, E.W., PROKHOROV, D.V. & WUNSCH, D.C. (1998). Comparative study of stock trend prediction using time delay, recurrent and probabilistic neural networks. IEEE Transactions on Neural Networks, 9/6, pp. 1456-1470.
- 40.SINGH, S. (1999). A Long memory pattern modelling and recognition system for financial time-series forecasting. Pattern Analysis and Applications, 2, pp. 264-273.
- 41.STANSELL, S.R. & EAKINS, S.G. (2003). Forecasting the direction of change in sector stock indexes: An application of neural networks. Journal of Asset Management, 5/1, pp. 37-48.
- 42.SUN, Y.F., LIANG, Y.C., ZHANG, W.L., LEE, H.P., LIN, W.Z. & CAO, L.J. (2005). Optimal partition algorithm of the RBF neural network and its application to financial time series forecasting. Neural Computation & Application, 14, pp. 36–44
- 43.THAWORNWONG, S. & ENKE, D. (2003). Forecasting Stock Returns with Artificial Neural Networks. In: Neural Networks in Business Forecasting, Zhang, P.G. (Ed.), Idea Group Inc., pp.47-74.
- 44.VELLIDO, A., LISBOA, P.J.G. & VAUGHAN, J. (1999). Neural networks in business: A survey of applications (1992-1998). Expert Systems with Applications, 17, pp. 51-70.
- 45.WALCZAK, S. (2001). An empirical analysis of data requirements for financial forecasting with neural networks. Journal of Management Information Systems, 17/4, pp. 203-222.
- 46.WHITE, H. (1988). Economic prediction using neural networks: The case of IBM daily stock returns. Proceedings of the IEEE International Conference on Neural Networks, pp. 451-458.
- 47.WONG, F.S., WANG, P.Z., GOH, T.H. & QUEK, B.K., (1992). Fuzzy neural systems for stock selection. Financial Analysts Journal, 48, pp. 47-52.
- 48.YAO, J., TAN, C.L. & POH, H.L. (1999). Neural networks for technical analysis: A study on KLCI. International Journal of Theoretical and Applied Finance, 2/2, pp. 221-241.
- 49.YAO, J.T. & TAN, C.L. (2001). Guidelines for Financial Forecasting with Neural Networks. Proceedings of International Conference on Neural Information Processing, Shanghai China, 14-18 November, pp. 1-6.
- 50.YILDIZ, B. (2001). Finansal başarısızlığın öngörülmesinde yapay sinir ağı kullanımı ve halka açık şirketlerde ampirik bir uygulama. İMKB Dergisi, 17, pp. 51-67
- 51.YODA. M, (1994). Predicting the Tokyo Stock Market. In: Trading on The Edge, Deboeck, G.J. (Ed.), John Wiley & Sons Inc., pp. 66-79.
- 52.YÜMLÜ, S., GÜRGEN S.F. & OKAY, N. (2003). Financial time series prediction using mixture of experts, Lecture Notes in Computer Science, 2869, pp.553-560.
- 53.YÜMLÜ, S.(2004). Turkish stock market analysis using mixture of experts, Engineering in Intelligent Systems (EIS 2004), Conference Proceedings.
- 54.YÜMLÜ, S.(2005). A comparison of global, recurrent and smoothed-piecewise neural models for Istanbul Stock Exchange (ISE) prediction", Pattern Recognition Letters, 26(13), pp.2093-2103.
APA | AVCI E (2007). Forecasting Daily and Sessional Returns of the ISE-100 Index with Neural Network Models. , 128 - 142. |
Chicago | AVCI EMIN Forecasting Daily and Sessional Returns of the ISE-100 Index with Neural Network Models. (2007): 128 - 142. |
MLA | AVCI EMIN Forecasting Daily and Sessional Returns of the ISE-100 Index with Neural Network Models. , 2007, ss.128 - 142. |
AMA | AVCI E Forecasting Daily and Sessional Returns of the ISE-100 Index with Neural Network Models. . 2007; 128 - 142. |
Vancouver | AVCI E Forecasting Daily and Sessional Returns of the ISE-100 Index with Neural Network Models. . 2007; 128 - 142. |
IEEE | AVCI E "Forecasting Daily and Sessional Returns of the ISE-100 Index with Neural Network Models." , ss.128 - 142, 2007. |
ISNAD | AVCI, EMIN. "Forecasting Daily and Sessional Returns of the ISE-100 Index with Neural Network Models". (2007), 128-142. |
APA | AVCI E (2007). Forecasting Daily and Sessional Returns of the ISE-100 Index with Neural Network Models. Doğuş Üniversitesi Dergisi, 8(2), 128 - 142. |
Chicago | AVCI EMIN Forecasting Daily and Sessional Returns of the ISE-100 Index with Neural Network Models. Doğuş Üniversitesi Dergisi 8, no.2 (2007): 128 - 142. |
MLA | AVCI EMIN Forecasting Daily and Sessional Returns of the ISE-100 Index with Neural Network Models. Doğuş Üniversitesi Dergisi, vol.8, no.2, 2007, ss.128 - 142. |
AMA | AVCI E Forecasting Daily and Sessional Returns of the ISE-100 Index with Neural Network Models. Doğuş Üniversitesi Dergisi. 2007; 8(2): 128 - 142. |
Vancouver | AVCI E Forecasting Daily and Sessional Returns of the ISE-100 Index with Neural Network Models. Doğuş Üniversitesi Dergisi. 2007; 8(2): 128 - 142. |
IEEE | AVCI E "Forecasting Daily and Sessional Returns of the ISE-100 Index with Neural Network Models." Doğuş Üniversitesi Dergisi, 8, ss.128 - 142, 2007. |
ISNAD | AVCI, EMIN. "Forecasting Daily and Sessional Returns of the ISE-100 Index with Neural Network Models". Doğuş Üniversitesi Dergisi 8/2 (2007), 128-142. |