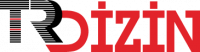
Yağış verilerinin yapay sinir ağları ve dalgacık dönüşümü yöntemleri ile tahmini
Yıl: 2008 Cilt: 7 Sayı: 3 Sayfa Aralığı: 73 - 85 Metin Dili: Türkçe İndeks Tarihi: 29-07-2022
Yağış verilerinin yapay sinir ağları ve dalgacık dönüşümü yöntemleri ile tahmini
Öz: Meteorolojik değişkenlerden birisi olan yağış, su kaynakları açısından çok önemlidir. Yağış, akışı meydana getiren en önemli değişkendir. Kısa süreli aşırı yağışlar önemli taşkınlara neden olmaktadır. Uzun sürelerde yeterli yağışın meydana gelmemesi durumunda kuraklık meydana gelmektedir. Karmaşık bir fiziksel süreç sonucunda meydana gelen yağışın doğru tahmin edilmesi genellikle zordur. Özellikle yersel değişimlerden ve bölgesel özelliklerden oldukça etkilenmesi yağışın tahminini daha zorlaştırmaktadır. Lineer olmayan sistemlerin davranışında başarıyla kullanılabilen bir kara-kutu modeli olan yapay sinir ağları, böylesine karmaşık değişkenlerin tahmininde başarıyla kullanılmaktadır. Yapay sinir ağlarında tahminin başarısı üzerinde kullanıcının etkileri sınırlıdır ve da-ha çok girdilere bağlıdır. Yapay sinir ağları yönteminin tahmindeki başarısını arttırmak için dalgacık dönüşümü bu çalışmada kullanılmıştır. Dalgacık dönüşümü, verilerin hem zaman hem de frekans ortamında incelenmesine olanak sağlayan bir yöntemdir. Bu çalışmada Yapay Sinir Ağları (YSA) ve dalgacık dönüşümü yöntemleri ile günlük yağış tahmini yapılmıştır. Bu amaçla Türkiye’ye ait 3 istasyonun günlük meteorolojik verileri kullanılmıştır. YSA yönteminin literatürde en çok kul-lanılan algoritmalarından, İleri Beslemeli Geriye Yayılmalı Yapay Sinir Ağları (İBGYSA) ve Radyal Tabanlı Yapay Sinir Ağları (RTYSA) yöntemleri yağış tahmini amacıyla kullanılmıştır. Farklı girdi kombinasyonları denenerek her istasyon için en uygun model bulunmaya çalışılmıştır. Sonuçlarda ileri beslemeli geriye yayılmalı yapay sinir ağları algoritmasının kullanıldığı yöntem en iyi performansı göstermiştir. Dalgacık dönüşümü-YSA yönteminin tahmin sonuçları çoklu lineer regresyon yönteminin sonuçları ile kıyaslanmış ve performans kriterlerine göre daha iyi olduğu bulunmuştur.
Anahtar Kelime: Estimation of precipitation data using artificial neural networks and wavelet transform
Öz: Forecasting the precipitation which is one of the most important meteorological variables is very important for planning and management of the water resources. Accurate precipitation prediction is one of the most difficult tasks in the meteorology because the complex physical processes involved and the variability of the precipitation is highly dependent on small scale processes and local geography. Especially the daily precipitation forecasting is one of the most challenging works and very important for the flood and drought analyses.Artificial Neural Networks (ANN) are a useful tool to identify this relation. ANN approach is extensively used in the water resources literature in recently years. Artificial neural networks which is a black-box model, is used successfully in the modeling of non-stationary and complex variables. Black-box models are divided generally as linear and nonlinear and in particular artificial neural networks method is used in the modeling of nonlinear system behavior. The artificial neural networks have some advantages, such as easily applied, not needing much data. However the accuracy of model predictions is very subjective and highly dependent on user’s ability, knowledge and understanding of the model. Especially, the input selecting is one of the most important phases in any ANN modeling study Because of this, wavelet transformation is used for increase of user ability and success of artificial neural networks. The wavelet transform, which can produce a good local representation of the signal in both the time and frequency domains, provides considerable information about the structure of the physical process to be modeled and has positive effects on the ANN modeling ability. Because of these reasons, coupling wavelets with the ANN can provide significant advantages on predicting.This study aims to predict the daily precipitation data of three belong to Turkish meteorological stations by applying the ANN methods and discrete wavelet transform. For this reason, the original time series were decomposed into a certain number of sub-time series using the wavelet transform. Then, the suitable sub time series constituted the inputs of the ANN and the resulting model was applied to forecast the original time series. The sub time series decomposed by discrete wavelet transform from the original time series provide detailed information about the data structure and its periodicity. Behavior of each sub-series is different. For the selection of dominant sub-series, the correlation coefficients between the decomposed wavelet sub-series and the observed precipitation time series are computed. The selection of dominant sub-series becomes effective on the output data improving ANN model’s performance.In this study, the wavelet transforms and the ANN has been applied to estimate the daily precipitation. The meteorological data belong to the three station were investigated for this study. These are the daily mean temperature, the daily maximum temperature, the daily minimum temperature, the daily total specific humidity, the daily total evaporation and the daily total precipitation. Each of the meteorological data considered as input for the ANN model was decomposed into the wavelet sub-series by Discrete Wavelet Transform (DWT). Then, ANN configuration is constructed with appropriate wavelet sub series as input and the original precipitation time series as output. So, different wavelet-ANN models were prepared for each station. Precipitation estimation was applied with the two different algorithms of the artificial neural networks. Employment of the Feed Forward Back Propagation (FFBP) in the precipitation estimation is compared with the Radial Basis Function (RBF) performances. The results were also compared with linear regression model. As a result, it was seen that the wavelet-feed forward back propagation method provided the best estimation performance. Results indicate that the wavelet-ANN model estimations were superior to the ones obtained by the multi linear regression model. The wavelet-ANN models have provided a good fit with the observed data, especially for the time series which have zero precipitation in the summer months. It was seen that the ANN-wavelet method provided very successful estimation performance. This study is the first application to the daily precipitation estimations using the wavelet sub-series of the various meteorological variables in the water resources literature. The wavelet-ANN method is especially convenient in variables having non-linear dynamics such as predicting of precipitation data.
Anahtar Kelime: Belge Türü: Makale Makale Türü: Araştırma Makalesi Erişim Türü: Erişime Açık
- Applequist, S., Gahrs, G.E., Pfeffer, R.L., (2002). Comparison of methodologies for probabilistic quantitative precipitation forecasting, American Meteorological Society, 17, 783-799.
- Broomhead, D., Lowe, D., (1988). Multivariable functional interpolation and adaptive networks, Complex Systems, 2, 6, 568-576.
- Campolo, M., Andreussi, P., Soldati,A., (1999). River flood forecasting with a neural network model, Water Resources Research, 35, 4, 1191–1197.
- Cigizoglu, H.K., (2003a). Incorporation of ARMA models into flow forecasting by artificial neural networks, Environmentrics, 14, 4, 417-427.
- Cigizoglu, H.K., (2003b). Estimation, forecasting and extrapolation of flow data by artificial neural networks, Hydrological Sciences Journal, 48, 3, 349-361.
- Cigizoglu, H.K., (2004a). Estimation and forecasting of daily suspended sediment data by multi layer perceptrons, Advances in Water Resources, 27, 185-195.
- Cığızoğlu, H.K., (2004b). Yapay sinir ağları ve zaman serileri analizinde kullanımı, IV. Ulusal Hidroloji Kongresi, İstanbul Teknik Üniveristesi, İnşaat Fakültesi. 21-25 Haziran 2004.
- Cigizoglu, H.K, Alp, M., (2004). Rainfall-Runoff modelling using three Neural Network methods, Artificial Intelligence and Soft Computing- ICAISC 2004 Lecture Notes in Artificial Intelligence, 3070, 166-171.
- Cigizoglu, H.K., Kisi, O., (2005). Flow Prediction by three Back Propagation Techniques using k-fold Partitioning of Neural Network Training Data., Nordic Hydrology, 36, 1, 1-16.
- Cigizoglu, H.K., Alp, M., (2006). Generalized Regression Neural Network in modeling river sediment yield, Advances in Engineering Software, 37, 2, 63-68.
- Daubechies, I., (1996). Where do wavelets come from?- A personal point of wiev, Proceedings of the IEEE, 84, 4.
- Freiwan M., Cigizoglu H.K., (2005). Prediction of total monthly rainfall in Jordan using feed forward backpropagation method, Fresenious Environmental Bulletin, 14, 2, 142-151.
- Hall, T., (1999). Precipitation forecasting using a neural network, Weather Forecasting, 14, 338-345.
- Hsu, K., Gupta, H.V. Sorooshian, S., (1995). Artificial neural network modelling of the rainfall runoff process, Water Resources Research, 31, 2517-2530.
- Kim, T.W., Valdes J.B. (2003). Nonlinear model for drought forecasting based on a conjunction of wavelet transforms and neural Networks, Journal of Hydrologic Engineering, ASCE, 6, 319.
- Küçük, M., (2004). Dalgacık dönüşüm tekniği kullanarak akım serilerinin modellenmesi. Doktora tezi, İTÜ Fen Bilimleri Enstitüsü.
- Kulligowski, R.J., Barros A.P., (1998). Localized precipitation from a numerical weather prediction model using Artificial Neural Networks, Weather and Forecasting, 13, 1195-1205.
- Maier, H.R., Dandy, G.C., (2000). Neural networks for the prediction and forecasting of water resources variables: a review of modeling issues and applications, Environmental Modeling and Software, 15, 101-124.
- Mallat, S. G., (1989). A theory for multiresoluion signal decomposition: The wavelet representation, IEEE Transactions on Pattern Analysis and Machine Intelligence, 11, 7, 674-693.
- Poggio, T. Girosi, F., (1990). Regularization algorithms for learning that are equivalent to multilayer networks, Science, 2247, 978-982.
- Ramirez, M.C.V., Velho, H.F.C., Ferreira, N.J., (2005). Artificial neural network technique for rainfall forecasting applied to the Sao Paulo region, Journal of Hydrology, 301, 146-162.
- Rajurkar, M. P., Kothyari, U. C. Chaube, U. C. (2002). Artificial neural networks for daily rainfall–runoff modeling, Hydrological Science Journal, 47, 6, 865–877.
- Sudheer, K.P., Gosain, A.K., Ramasastri, K.S., (2002). A data-driven algorithm for constructing artificial neural network rainfall-runoff models, Hydrological Processes, 16, 1325-1330.
- Wang, D., Ding, J., (2003). Wavelet network model and its application to the prediction of hydrology, Nature and Science, 1, 67-71.
APA | Partal T, KAHYA E, CIĞIZOĞLU K (2008). Yağış verilerinin yapay sinir ağları ve dalgacık dönüşümü yöntemleri ile tahmini. , 73 - 85. |
Chicago | Partal Turgay,KAHYA Ercan,CIĞIZOĞLU Kerem Yağış verilerinin yapay sinir ağları ve dalgacık dönüşümü yöntemleri ile tahmini. (2008): 73 - 85. |
MLA | Partal Turgay,KAHYA Ercan,CIĞIZOĞLU Kerem Yağış verilerinin yapay sinir ağları ve dalgacık dönüşümü yöntemleri ile tahmini. , 2008, ss.73 - 85. |
AMA | Partal T,KAHYA E,CIĞIZOĞLU K Yağış verilerinin yapay sinir ağları ve dalgacık dönüşümü yöntemleri ile tahmini. . 2008; 73 - 85. |
Vancouver | Partal T,KAHYA E,CIĞIZOĞLU K Yağış verilerinin yapay sinir ağları ve dalgacık dönüşümü yöntemleri ile tahmini. . 2008; 73 - 85. |
IEEE | Partal T,KAHYA E,CIĞIZOĞLU K "Yağış verilerinin yapay sinir ağları ve dalgacık dönüşümü yöntemleri ile tahmini." , ss.73 - 85, 2008. |
ISNAD | Partal, Turgay vd. "Yağış verilerinin yapay sinir ağları ve dalgacık dönüşümü yöntemleri ile tahmini". (2008), 73-85. |
APA | Partal T, KAHYA E, CIĞIZOĞLU K (2008). Yağış verilerinin yapay sinir ağları ve dalgacık dönüşümü yöntemleri ile tahmini. İTÜ Dergisi Seri D: Mühendislik, 7(3), 73 - 85. |
Chicago | Partal Turgay,KAHYA Ercan,CIĞIZOĞLU Kerem Yağış verilerinin yapay sinir ağları ve dalgacık dönüşümü yöntemleri ile tahmini. İTÜ Dergisi Seri D: Mühendislik 7, no.3 (2008): 73 - 85. |
MLA | Partal Turgay,KAHYA Ercan,CIĞIZOĞLU Kerem Yağış verilerinin yapay sinir ağları ve dalgacık dönüşümü yöntemleri ile tahmini. İTÜ Dergisi Seri D: Mühendislik, vol.7, no.3, 2008, ss.73 - 85. |
AMA | Partal T,KAHYA E,CIĞIZOĞLU K Yağış verilerinin yapay sinir ağları ve dalgacık dönüşümü yöntemleri ile tahmini. İTÜ Dergisi Seri D: Mühendislik. 2008; 7(3): 73 - 85. |
Vancouver | Partal T,KAHYA E,CIĞIZOĞLU K Yağış verilerinin yapay sinir ağları ve dalgacık dönüşümü yöntemleri ile tahmini. İTÜ Dergisi Seri D: Mühendislik. 2008; 7(3): 73 - 85. |
IEEE | Partal T,KAHYA E,CIĞIZOĞLU K "Yağış verilerinin yapay sinir ağları ve dalgacık dönüşümü yöntemleri ile tahmini." İTÜ Dergisi Seri D: Mühendislik, 7, ss.73 - 85, 2008. |
ISNAD | Partal, Turgay vd. "Yağış verilerinin yapay sinir ağları ve dalgacık dönüşümü yöntemleri ile tahmini". İTÜ Dergisi Seri D: Mühendislik 7/3 (2008), 73-85. |