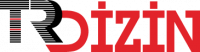
Artificial neural network analysis for prediction of headache prognosis in elderly patients
Yıl: 2009 Cilt: 39 Sayı: 1 Sayfa Aralığı: 5 - 12 Metin Dili: Türkçe İndeks Tarihi: 29-07-2022
Artificial neural network analysis for prediction of headache prognosis in elderly patients
Öz: Amaç: Çalışmanın amacı, sinir ağlarının takip süresi içinde başağrısı tam olarak iyileşen yaşlı hastaları belirleyerek sınıflandırma performansını incelemektir. Gereç ve Yöntem: Yaşlı hastalarda başağrısı prognozunu tahmin etmek için, en yaygın sinir ağı olan çok tabakalı perseptron kullanıldı. Veri seti, eğitim ve test setlerine ayrılarak, öğrenme algoritması olarak geriye yayılım algoritması kullanıldı. Tedavinin 20, 40 ve 60. aylarında hastalardaki tam iyileşmeyi tahmin etmek için kullanılan modellerin doğruluk dereceleri ROC eğrileri altında kalan alanlar kullanılarak değerlendirildi. Bulgular: Sınıflandırma sonuçları, sinir ağı modellerinin hem eğitim hem de test aşamalarında yüksek performansa dahip olduklarını göstermiştir. Ayrıca, her tedavi periyodu için elde edilen ROC eğrisi altında kalan alanlar, tam iyileşmeyi tahmin etmek için kullanılan modellerin doğruluk derecelerinin 0.75-0.90 aralığında olduğunu göstermiştir. Sonuç: Gruplandırılmış sağkalım verileri için sinir ağı modeli prognostik model olarak kullanılabilir. Eğer bir hastalığın görülme sıklığı düşük ise, modelin gerçek hastaları tayin etme gücü de (duyarlılığı) düşük olacaktır.
Anahtar Kelime: Konular:
Yaşlı hastalarda baş ağrısı prognozunun tahmini için yapay sinir ağları analizi
Öz: Aim: To investigate the ability of neural networks to detect and classify the complete improvement of headache in elderly patients during the follow- up period. Materials and Methods: The multilayer perceptron (MLP), which is the most common neural network, was used to predict prognosis of headache in elderly patients. The data set was divided into training and test sets, and back-propagation algorithm was used as the learning algorithm. The accuracies of the models to predict completely improved patients at the end of 20, 40, and 60 months of follow-up were evaluated by means of the areas under the receiver operating characteristic (ROC) curves. Results: The classification results showed the neural network models had good performance in both training and test phases. In addition, the areas under the ROC curve for each period showed that the accuracies of the models to predict the completely improved patients were in the interval of 0.75-0.90. Conclusions: Neural network model for grouped survival data can be used as a prognostic model. If the prevalence of a disease is low, the sensitivity of the model for detection of the patients with disease will be low.
Anahtar Kelime: Konular:
Belge Türü: Makale Makale Türü: Araştırma Makalesi Erişim Türü: Erişime Açık
- 1. Abu-Hanna A, Lucas PJF. Prognostic models in medicine: artificial intelligence and statistical approaches. Method Inform Med 2001; 40: 1–5.
- 2. Ahmed FE. Artificial neural networks for diagnosis and survival prediction in colon cancer. Mol Cancer 2005; 4: 29.
- 3. Schwarzer G, Vach W, Schumacher M. On the misuses of artificial neural networks for prognostic and diagnostic classification in oncology. Stat Med 2000; 19: 541-61.
- 4. Ohno-Machado L. A comparison of Cox proportional hazards and artificial neural network models for medical prognosis. Comput Biol Med 1997; 27(1): 55-65.
- 5. Biganzoli E, Borrachi P, Mariani L, Marubini E. Feed forward neural networks for the analysis of censored survival data: a partial logistic regression approach. Stat Med 1998; 17: 1169- 86.
- 6. Biganzoli E, Borrachi P, Marubini E. A general framework for neural network models on censored survival data. Neural Netw 2002; 15: 209-18.
- 7. Eleuteri A, Tagliaferri R, Milano L, De Placido S, De Laurentis M. A novel neural network-based survival analysis model. Neural Netw 2003; 16: 855-64.
- 8. Allison JS, Heo J, Iskandrian AE. Artificial neural network modeling of stress single-photon emission computed tomographic imaging for detecting extensive coronary artery disease. Am J Cardiol 2005; 5: 178-81.
- 9. Ennis M, Hinton G, Naylor D, Revow M, Tibshirani R. A comparison of statistical learning methods on the GUSTO database. Stat Med 1998; 17(21): 2501-8.
- 10. Keijsers NLW, Horstink MWIM, van Hilten JJ, Hoff JI, Gielen CCAM. Detection and assessment of the severity of levodopainduced dyskinesia in patients with Parkinson’s disease by neural networks. Mov Disord 2000; 15(6): 1104–11.
- 11. Tu JV, Guerriere MRJ. Use of a neural network as a predictive instrument for length of stay in the intensive care unit following cardiac surgery. Comput Biomed Res 1993; 26(3): 220-9.
- 12. Gueli N, Piccirillo G, Troisi G, Cicconetti P, Meloni F, Ettorre E et al. The influence of lifestyle on cardiovascular risk factors: analysis using a neural network. Arch Gerontol Geriatr 2005; 40(2): 157- 72.
- 13. Dreiseitl S, Ohno-Machado L. Logistic regression and artificial neural network classification models: a methodology review. J Biomed Inform 2002; 35: 352-9.
- 14. Kattan MW. Comparison of Cox regression with other methods for determining predictive models and normograms. J Urol 2003; 170: 6-10.
- 15. Bartfay E, Mackillop WJ, Pater JL. Comparing the predictive value of neural network models to logistic regression models on the risk of death for small-cell lung cancer patients. Eur J Cancer Care 2006; 15(2): 115-24.
- 16. Yamamura S, Takehira R, Kawada K, Nishizawa K, Katayama S, Hirano M et al. Application of artificial neural network modelling to identify severely ill patients whose aminoglycoside concentrations are likely to fall below therapeutic concentrations. J Clin Pharm Ther 2003; 28: 425–32.
- 17. Hosmer DW, Lemeshow S. Applied Logistic Regression. 2nd ed. New York: John Wiley & Sons, Inc.; 2000.
- 18. Rasmussen BK, Jensen R, Schroll M, Olesen J. Epidemiology of headache in a general population-a prevalence study. J Clin Epidemiol 1991; 44: 1147-57.
- 19. Rasmussen BK. Epidemiology of headache. Cephalalgia 1995; 15: 45-6.
- 20. Prencipe M, Casini AR, Ferretti C, Santini M, Pezzella F, Scaldaferri N et al. Prevalence of headache in an elderly population: attack frequency, disability, and use of medication. J Neurol Neurosurg Psychiatry 2001; 70(3): 377-81.
- 21. Headache Classification Committee of the International Headache Society. The Internal Classification of Headache Disorders. Cephalalgia 2004; 24(Suppl 1): 1-150.
- 22. Silberstein SD, Lipton RB, Dalessio DJ. Overview, diagnosis, and classification of headache. In: Silberstein SD, Lipton RB, Dalessio DJ, editors. Wolff’s Headache and Other Head Pain. New York: Oxford University Press; 2001. pp. 6-26.
- 23. Manel S, Dias JM, Ormerod SJ. Comparing discriminant analysis, neural networks and logistic regression for predicting species distributions: a case study with a Himalayan river bird. Ecol Model 1999; 120: 337–47.
- 24. McGonigal MD, Cole J, Schwab CW, Kauder DR, Rotondo MF, Angood PB. A new approach to probability of survival scoring for trauma quality assurance. J Trauma 1993; 34: 863-8.
- 25. Ebell MH. Artificial neural networks for predicting failure to survive following in-hospital cardiopulmonary resuscitation. J Fam Pract 1993; 36: 297-303.
- 26. Kappen HJ, Neijt JP. Advanced ovarian cancer: neural network analysis to predict treatment outcome. Ann Oncol 1994; 4: 31-4.
- 27. Cucchetti A, Vivarelli M, Heaton ND, Phillips S, Piscaglia F, Bolondi L et al. Artificial neural network is superior to MELD in predicting mortality of patients with end-stage liver disease. Gut 2007; 56: 253-8.
APA | TAŞDELEN B, HELVACI S, KALEAĞASI H, Özge A (2009). Artificial neural network analysis for prediction of headache prognosis in elderly patients. , 5 - 12. |
Chicago | TAŞDELEN BAHAR,HELVACI Sema,KALEAĞASI Hakan,Özge Aynur Artificial neural network analysis for prediction of headache prognosis in elderly patients. (2009): 5 - 12. |
MLA | TAŞDELEN BAHAR,HELVACI Sema,KALEAĞASI Hakan,Özge Aynur Artificial neural network analysis for prediction of headache prognosis in elderly patients. , 2009, ss.5 - 12. |
AMA | TAŞDELEN B,HELVACI S,KALEAĞASI H,Özge A Artificial neural network analysis for prediction of headache prognosis in elderly patients. . 2009; 5 - 12. |
Vancouver | TAŞDELEN B,HELVACI S,KALEAĞASI H,Özge A Artificial neural network analysis for prediction of headache prognosis in elderly patients. . 2009; 5 - 12. |
IEEE | TAŞDELEN B,HELVACI S,KALEAĞASI H,Özge A "Artificial neural network analysis for prediction of headache prognosis in elderly patients." , ss.5 - 12, 2009. |
ISNAD | TAŞDELEN, BAHAR vd. "Artificial neural network analysis for prediction of headache prognosis in elderly patients". (2009), 5-12. |
APA | TAŞDELEN B, HELVACI S, KALEAĞASI H, Özge A (2009). Artificial neural network analysis for prediction of headache prognosis in elderly patients. Turkish Journal of Medical Sciences, 39(1), 5 - 12. |
Chicago | TAŞDELEN BAHAR,HELVACI Sema,KALEAĞASI Hakan,Özge Aynur Artificial neural network analysis for prediction of headache prognosis in elderly patients. Turkish Journal of Medical Sciences 39, no.1 (2009): 5 - 12. |
MLA | TAŞDELEN BAHAR,HELVACI Sema,KALEAĞASI Hakan,Özge Aynur Artificial neural network analysis for prediction of headache prognosis in elderly patients. Turkish Journal of Medical Sciences, vol.39, no.1, 2009, ss.5 - 12. |
AMA | TAŞDELEN B,HELVACI S,KALEAĞASI H,Özge A Artificial neural network analysis for prediction of headache prognosis in elderly patients. Turkish Journal of Medical Sciences. 2009; 39(1): 5 - 12. |
Vancouver | TAŞDELEN B,HELVACI S,KALEAĞASI H,Özge A Artificial neural network analysis for prediction of headache prognosis in elderly patients. Turkish Journal of Medical Sciences. 2009; 39(1): 5 - 12. |
IEEE | TAŞDELEN B,HELVACI S,KALEAĞASI H,Özge A "Artificial neural network analysis for prediction of headache prognosis in elderly patients." Turkish Journal of Medical Sciences, 39, ss.5 - 12, 2009. |
ISNAD | TAŞDELEN, BAHAR vd. "Artificial neural network analysis for prediction of headache prognosis in elderly patients". Turkish Journal of Medical Sciences 39/1 (2009), 5-12. |