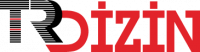
Kurak bölge aylık yağışlarının Markov zinciri eklenmiş koşullu ileri beslemeli geri yayılım yapay sinir ağları ile tahmini
Yıl: 2009 Cilt: 8 Sayı: 6 Sayfa Aralığı: 37 - 48 Metin Dili: Türkçe İndeks Tarihi: 29-07-2022
Kurak bölge aylık yağışlarının Markov zinciri eklenmiş koşullu ileri beslemeli geri yayılım yapay sinir ağları ile tahmini
Öz: Su kaynaklarının etkin bir şekilde planlanması ve yönetimi için yağışın doğru bir şekilde tahmin edilmesi büyük önem taşımaktadır. Hidrometeorolojik zaman serilerinin tahmini, zaman serisini etkileyen parametrelerin belirsizliğinden dolayı en zor işlerden biridir. Yapay sinir ağlarına dayalı kurak bölge yağış tahmin modelleri literatürde çok sınırlı sayıda bulunmaktadır. Bu çalışmada aylık toplam yağışın tahmini için Markov zincirleri eklenmiş koşullu ileri beslemeli geri yayılım yapay sinir ağları yöntemi kullanılmıştır. Uygulama için Ürdün 'ün dağlık bölgesinden Amman meteoroloji istasyonu seçilmiştir. Çalışmada kullanılan veriler homojenlik testlerinden geçirilmiş, kullanılan testlere göre Ürdün 'ün bu istasyonunun yağış verilerinin homojen olduğu belirlenmiştir. Bu çalışmada geliştirilen modeller, önceki aylara ait gözlenmiş yağış verilerini kullanarak gelecek ayın yağışının tahmin edilmesi prensibine dayanarak kurulmuştur. Deneme-yanılma yöntemi ile çok sayıda model seçeneği üretilmiştir. Girdi sayısı minimum olacak şekilde önce sadece bir önceki ayın yağışının girdi olarak kullanıldığı model seçeneği ile başlanmış, seçilen girdiler farklı hücre sayıları ile denenmiştir. Hücre sayısı girdi sayısı kadar seçilmiş, ampirik bir düşünce ile girdi sayısının iki katına kadar birer birer artırılmıştır. Periyodikliğin etkisinin görülmesi açısından periyodik bileşen de modellerde girdi olarak kullanılmıştır. Denenen her bir modelin performansı Ortalama Karesel Hata (OKH) ve belirlilik katsayısı (R2) ile ölçülmüştür. Markov zincirleri ile birleştirilmiş koşullu ileri beslemeli geri yayılım yapay sinir ağı modelinin kuru ayların belirlenmesinde, efı yüksek yağış ve yağış tahmininde büyük başarı gösterdiği anlaşılmıştır.
Anahtar Kelime: Forecasting monthly precipitation for arid regions using conditional feed-forward backpropagation artificial neural network combined with Markov chain
Öz: Understanding, modeling, prediction or forecasting of precipitation has always been important. Precipitation is the driven factor of hydrological cycle and is one of the main sources of water without which the humankind cannot survive. In particular, it becomes the unique source of water in arid regions where surface water courses are generally intermittent or ephemeral in nature. In such regions the main water income is from the groundwater storages that are again fed by precipitation. In such regions, therefore, precipitation becomes much more interesting than runoff to analyze as flow records are generally insufficient in length to obtain accurate estimations. Analysis of precipitation is evident not only because of that reason but also for agricultural and socio-economical activities, for increasing human and environmental demands as well as for planning and management of water resources. Precipitation changes both in time and space and affects on other components of the hydrological cycle; i.e. surface runoff, infiltration, groundwater, seepage, percolation, evaporation, transpiration, etc. Time variation comes from the seasonal clima-tological changes in the atmosphere whereas spatial change is due to the topographical heterogeneity on the earth surface. Precipitation amount should be predicted accurately for an affective water resources management and planning. Prediction of hydrometeorological time series is difficult because of uncertainty in the parameters which affect the time series. In literature, the applications of artificial neural networks to forecast arid-region precipitation are limited. Forecasting monthly precipitation in arid regions is investigated by means of the conditional feed-forward backpropagation artificial neural network combined with Markov chain. Amman meteorological station from the hilly region in Jordan is selected for application. Various homogeneity tests are used for the data, which is found homogeneous according to the results of the tests. The feed-forward backpropagation artificial neural networks, statistical, and stochastic methods provided negative approximations for some of the low precipitation (dry months) whereas Markov chain forecasts are bounded with zero as the lower limit. Prediction using Markov chain does not generate physically unexplainable results mainly because the forecasts of the feed-forward backpropagation combined with Markov chain method are bounded with the minimum (zero) precipitation. Antecedent monthly precipitation data are used as input to the model to predict the total precipitation in the next month. Selection of the inputs is based on the correlation coefficient. For the station analyzed, it is observed that precipitation in any month was highly correlated to its previous two months and its previous year record. A trial-and-error based construction for various artificial neural networks are tested. A total of 28 combinations of input variables are investigated. It is finally ended with an architecture that uses, as input variables, precipitation in two previous months and the previous year's precipitation of the month to be forecasted. Also a periodical component is added into the input vector to simulate the existing periodicity in the monthly precipitation. Since the sigmoid function is used as the activation function for hidden and output layers, the model input and output are scaled appropriately to fall within the function limit (zero to one). Conditional feed-forward backpropagation artificial neural network combined with Markov chain is trained using the Levenberg-Marquardt training algorithm. After training is over, the weights are used to test the network performance on the test data. In conclusion, it is seen that the conditional model considerably improves the accuracy of the one month ahead precipitation forecasting compared to the unconditional model.
Anahtar Kelime: Belge Türü: Makale Makale Türü: Araştırma Makalesi Erişim Türü: Erişime Açık
- Abdel-Aty, S.H., (1976). On the distribution of rainfall sequences with application to actual data, Dirasat-Natural Sciences, 3, 15-26.
- Al-Kharabsheh, A., (2000). Ground-water modeling and long-term management of the Azraq basin as an example of arid area conditions (Jordan), Journal of Arid Environment, 44, 2, 143-153.
- Al-Shalash, A., (1964). Rainfall atlas of the Hashemite Kingdom of Jordan, Publication of the University of Jordan, Amman.
- Bani Domi, M., (2000). Analysis of unusual atmospheric conditions which caused two successive khamassine and snow storms that affected Jordan between 15-18 March 1998, Abhath Alyarmouk, 18, 2(A), 385-409.
- Bayazıt, M., (1996). İnşaat mühendisliğinde olasılık yöntemleri, İTÜ İnşaat Fakültesi Matbaası, İstanbul.
- Bodri, L. ve Cermak, V., (2000). Prediction of extreme precipitation using a neural network: application to summer flood occurrence in Moravia, Advances in Engineering Software, 31, 311-321.
- Bodri, L. ve Cermak, V., (2001). Neural network prediction of monthly precipitation: Application to summer flood occurrence in two regions of central europe, Studia Geophysica et Geodaetica, 45, 155-167.
- Çhantasut, N., Charoenjit, C. ve Tanprasert, C, (2004). Predictive mining of rainfall predictions using artificial neural networks for Chao Phraya River, Proceeding, 4th International Conference of the Asian Federation of Information Technology in Agriculture and the 2nd World Congress on Computers in Agriculture and Natural Resources, August 9-12, 2004,117-122, Bangkok, Thailand.
- Chattopadhyay, S., (2007). Feed forward Artificial Neural Network model to predict the average summer-monsoon rainfall in India, Ada Geophysica, 55, 3, 369-382.
- Cığızoğlu, H.K., (2005a). Generalized regression neural network in monthly flow forecasting, Civil Engineering and Environmental Systems, 22, 2, 71-84.
- Cığızoğlu, H.K., (2005b). Application of generalized regression neural networks to intermittent flow forecasting and estimation, Journal of Hydro-logic Engineering, 10, 4, 336-341.
- Coulibaly, P. ve Evora, N.D., (2007). Comparison of neural network methods for infilling missing daily weather records, Journal of Hydrology, 341,27-41.
- Dahamsheh, A., (2003). Monitoring meteorological drought in Jordan, Yüksek Lisans Tezi, ITU, Institute of Science and Technology, İstanbul.
- Dahamsheh, A. ve Aksoy, H., (2007). Structural characteristics of annual precipitation data in Jordan, Theoretical and Applied Climatology, 88, 201-212.
- Freiwan, M. ve Cığızoğlu, H.K., (2005). Prediction of total monthly rainfall in Jordan using feed forward backpropagation method, Fresenius Environmental Bulletin, 14, 2, 142-151.
- Ghanem, A., (1997). Analysis of decadal rainfall in Jordan, Dirasat-Natural and Engineering Science, 24, 1, 179-201.
- Hall, T., (1998). Precipitation forecasting using a neural network, W aether and Forecasting, 14.
- Hung, N.Q., Babel, M.S., Wesakul, S. ve Tripathi, N.K., (2008). An artificial neural network model for rainfall forecasting in Bangkok, Thailand, Hydrology and Earth System Sciences Discussion, 5, 183-218.
- Ionides, M.G., (1939). The water resources of trans Jordan and their development, London.
- Kalteh, A.M. ve berndtsson, R., (2007). Interpolating monthly precipitation by self-brganizing map (SOM) and multilayer perceptron (MLP), Hydro-logical Sciences-Journal-des Sciences Hy-drologiques, 52, 2.
- Kuligowski, R.J. ve Barros, A.P., (1997). Experiments in short-term precipitation forecasting using Aartificial neural Networks, Monthly Weather Review, 126.
- Kuligowski, R.J. ve Barros, A.P., (1998a). Localized precipitation forecasts from a numerical weather prediction model using artificial neural networks, Weather and forecasting, 13.
- Kuligowski, R.J. ve Barros, A.P., (1998b). Using artificial neural networks to estimate missing rainfall data, Journal of the American Water Resources Association, 34, 6.
- Kumarasiri, A.D. ve Sonnadara, D.U.J., (2006). Rainfall forecasting: An artificial neural network approach, Proceedings of the Technical Sessions, 22, 1-13, Institute of Physics, Sri Lanka.
- Liu, H., Chandrasekar, V. ve Xu, G., (2001). An adaptive neural network scheme for radar rainfall estimation from WSR-88D observations, Journal of Applied Meteorology, 40.
- Liu, J.N.K. ve Lee, R.S.T., (1999). Rainfall forecasting from multiple point sources using neural networks, Proceedings, 1999 IEEE International Conference on Systems, Man, and Cybernetics, 3, 429-434, Piscataway, NJ, IEEE Service Center.
- McCullagh, J., Bluff, K. ve Ebert, E., (1995). A neural network model for rainfall estimation, 2nd New Zealand Two-Stream International Conference on Artificial Neural Networks and Expert Systems.
- Sahai, A.K., Somon, M.K. ve Satyan, V., (2000). All India summer monsoon rainfall prediction using an artificial neural network, Climate Dynamics, 16, 291-302.
- Shehadeh, N., (1976). The variability of rainfall in Jordan, Dirasat-Humanities, 3, 67-84.
- Tarawneh, S., (1999). Drought analysis of desert and badia of Jordan, Abhath Alyarmouk, Basic Science and Engineering, 2, 117-145.
- Tarawneh, S., (2000). Drought analysis of selected rainfall in Jordan, Dirasat-Engineering Science, 2, 117-145.
- Tarawneh, Q., Kadıoğlu, M., (2003). An analysis of precipitation climatology in Jordan, Theoretical and Applied Climatology, 74, 123-136.
- Al-Weshah, R.A., (2005). Jordan's water resources: Technical perspective, (http://www.wsta-gcc.org/icludes/GWC_4th/doc/w6.htm, last visited on March 16,2005).
APA | DAHAMSHEH A, Aksoy H (2009). Kurak bölge aylık yağışlarının Markov zinciri eklenmiş koşullu ileri beslemeli geri yayılım yapay sinir ağları ile tahmini. , 37 - 48. |
Chicago | DAHAMSHEH Ahmad,Aksoy Hafzullah Kurak bölge aylık yağışlarının Markov zinciri eklenmiş koşullu ileri beslemeli geri yayılım yapay sinir ağları ile tahmini. (2009): 37 - 48. |
MLA | DAHAMSHEH Ahmad,Aksoy Hafzullah Kurak bölge aylık yağışlarının Markov zinciri eklenmiş koşullu ileri beslemeli geri yayılım yapay sinir ağları ile tahmini. , 2009, ss.37 - 48. |
AMA | DAHAMSHEH A,Aksoy H Kurak bölge aylık yağışlarının Markov zinciri eklenmiş koşullu ileri beslemeli geri yayılım yapay sinir ağları ile tahmini. . 2009; 37 - 48. |
Vancouver | DAHAMSHEH A,Aksoy H Kurak bölge aylık yağışlarının Markov zinciri eklenmiş koşullu ileri beslemeli geri yayılım yapay sinir ağları ile tahmini. . 2009; 37 - 48. |
IEEE | DAHAMSHEH A,Aksoy H "Kurak bölge aylık yağışlarının Markov zinciri eklenmiş koşullu ileri beslemeli geri yayılım yapay sinir ağları ile tahmini." , ss.37 - 48, 2009. |
ISNAD | DAHAMSHEH, Ahmad - Aksoy, Hafzullah. "Kurak bölge aylık yağışlarının Markov zinciri eklenmiş koşullu ileri beslemeli geri yayılım yapay sinir ağları ile tahmini". (2009), 37-48. |
APA | DAHAMSHEH A, Aksoy H (2009). Kurak bölge aylık yağışlarının Markov zinciri eklenmiş koşullu ileri beslemeli geri yayılım yapay sinir ağları ile tahmini. İTÜ Dergisi Seri D: Mühendislik, 8(6), 37 - 48. |
Chicago | DAHAMSHEH Ahmad,Aksoy Hafzullah Kurak bölge aylık yağışlarının Markov zinciri eklenmiş koşullu ileri beslemeli geri yayılım yapay sinir ağları ile tahmini. İTÜ Dergisi Seri D: Mühendislik 8, no.6 (2009): 37 - 48. |
MLA | DAHAMSHEH Ahmad,Aksoy Hafzullah Kurak bölge aylık yağışlarının Markov zinciri eklenmiş koşullu ileri beslemeli geri yayılım yapay sinir ağları ile tahmini. İTÜ Dergisi Seri D: Mühendislik, vol.8, no.6, 2009, ss.37 - 48. |
AMA | DAHAMSHEH A,Aksoy H Kurak bölge aylık yağışlarının Markov zinciri eklenmiş koşullu ileri beslemeli geri yayılım yapay sinir ağları ile tahmini. İTÜ Dergisi Seri D: Mühendislik. 2009; 8(6): 37 - 48. |
Vancouver | DAHAMSHEH A,Aksoy H Kurak bölge aylık yağışlarının Markov zinciri eklenmiş koşullu ileri beslemeli geri yayılım yapay sinir ağları ile tahmini. İTÜ Dergisi Seri D: Mühendislik. 2009; 8(6): 37 - 48. |
IEEE | DAHAMSHEH A,Aksoy H "Kurak bölge aylık yağışlarının Markov zinciri eklenmiş koşullu ileri beslemeli geri yayılım yapay sinir ağları ile tahmini." İTÜ Dergisi Seri D: Mühendislik, 8, ss.37 - 48, 2009. |
ISNAD | DAHAMSHEH, Ahmad - Aksoy, Hafzullah. "Kurak bölge aylık yağışlarının Markov zinciri eklenmiş koşullu ileri beslemeli geri yayılım yapay sinir ağları ile tahmini". İTÜ Dergisi Seri D: Mühendislik 8/6 (2009), 37-48. |