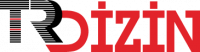
Hibrit nanoakışkanların özgül ısılarının yapay sinir ağları ile tahmin edilmesi
Yıl: 2022 Cilt: 37 Sayı: 1 Sayfa Aralığı: 377 - 388 Metin Dili: Türkçe DOI: 10.17341/gazimmfd.880340 İndeks Tarihi: 29-07-2022
Hibrit nanoakışkanların özgül ısılarının yapay sinir ağları ile tahmin edilmesi
Öz: Nanoakışkanlı mühendislik sistemlerinin deneysel ve sayısal analizinde nanoakışkanların termofiziksel ve reolojik özelliklerinin yüksek doğrulukla belirlenmesi analiz sonuçlarının hassasiyeti üzerinde kayda değer bir etkiye sahiptir. Bu çalışmada, deiyonize su bazlı CuO + ÇDKNT, MgO + ÇDKNT ve SnO2 + ÇDKNT hibrit nanoakışkanlarının özgül ısılarının tahmin edilmesinde kullanılabilecek Yapay Sinir Ağları (YSA) tabanlı bir tahminleyici geliştirilmesi ve bu tahminleyicinin literatürde yaygın olarak kullanılan korelasyonların yerine kullanılabilme potansiyelinin araştırılması hedeflenmiştir. Çalışmada, literatürde bulunan deiyonize su bazlı CuO + ÇDKNT, MgO + ÇDKNT ve SnO2 + ÇDKNT hibrit nanoakışkanların farklı sıcaklık T (25 – 50 ºC), hacim oranı φ (%0,25 – %1,50) ve partikül çapı dp (20 – 50 nm) için deneysel olarak elde edilmiş özgül ısılarına ait veriler kullanılmıştır. Nöron sayısı, gizli katman sayısı, transfer fonksiyonu, epoch sayısı ve öğrenme oranı gibi eğitim algoritması ve ağa ait hiper parametrelerin optimum değerleri ve çeşitli eğitim algoritmaları arasından ele alınan problem için en iyi sonuç veren eğitim algoritması Bayes optimizasyonu ile belirlenmiştir. Aşırı öğrenmeye karşı önlem olarak k-katlı çapraz doğrulama uygulanmıştır. Çalışma sonucunda elde edilen YSA tabanlı tahminleyici ile klasik korelasyonlara nazaran doğruluk payı daha yüksek bir tahminleme yapılabileceği ve YSA’nın nanoakışkanların özgül ısılarının belirlenmesinde kullanılabilecek güçlü bir araç olduğu sonucuna varılmıştır.
Anahtar Kelime: Prediction of specific heat of hybrid nanofluids using artificial neural networks
Öz: Determination of thermophysical and rheological properties of nanofluids with high accuracy to be used in the experimental and numerical analysis of nanofluid-based engineering systems has a significant effect on the accuracy of results. The aim of this study is to develop an Artificial Neural Networks (ANN) based estimator that can be used to predict the specific heat of deionized water-based CuO + MWCNT, MgO + MWCNT, and SnO2 + MWCNT hybrid nanofluids and to investigate the usability of the ANN-based estimators instead of the commonly used correlations available in the literature. Experimentally obtained data found in the literature on the specific heat of deionized water-based CuO + MWCNT, MgO + MWCNT and SnO2 + MWCNT hybrid nanofluids measured for various temperature T (25 – 50°C ), volume concentration φ (0.25% – 1.50%), and particle diameter dp (20 – 50 nm) were used in the present study. The training algorithm's and the network's hyperparameters such as neuron number, hidden layer number, transfer function, epoch number, and learning rate, and the best training algorithm for the problem addressed among various training algorithms were determined by employing the Bayes optimization. K-fold cross-validation was applied as a precaution against overfitting. It was concluded as a result of the study that estimation with higher accuracy can be made with the ANN-based estimator compared to classical correlations and ANN is a powerful tool that can be used in determining the specific heat of nanofluids.
Anahtar Kelime: Belge Türü: Makale Makale Türü: Araştırma Makalesi Erişim Türü: Erişime Açık
- 1. Zyla G., Fall J., Estellé P., The influence of ash content on thermophysical properties of ethylene glycol based graphite/diamonds mixture nanofluids, Diamond and Related Materials, 74, 81-89, 2017.
- 2. Sohel Murshed S.M., Estellé P., A state of the art review on viscosity of nanofluids, Renewable and Sustainable Energy Reviews, 76, 1134-1152, 2017.
- 3. Choi S. U., Eastman J. A., Enhancing thermal conductivity of uids with nanoparticles, 1995 International mechanical engineering congress and exhibition, San Francisco-CA (United States), 12-17 November 1995.
- 4. Minea A.A, Estellé P., Numerical study on CNT nanofluids behavior in laminar pipe flow, Journal of Molecular Liquids, 271, 281-289, 2018.
- 5. Nikulin A., Moit, A.S., Moreira, A.L.N., Murshed S.M.S., Huminic A., Grosu Y., ... & Khliyeva O., Effect of Al2O3 nanoparticles on laminar, transient and turbulent flow of isopropyl alcoho, International Journal of Heat and Mass Transfer, 130, 1032-1044, 2019.
- 6. Shahrul I.M., Mahbubul I.M., Khaleduzzaman S.S., Saidur R., Sabri M.F.M., A comparative review on the specific heat of nanofluids for energy perspective, Renewable and sustainable energy reviews, 38, 88-98, 2014.
- 7. Hemmati-Sarapardeh A., Varamesh A., Husein M.M., Karan K., On the evaluation of the viscosity of nanofluid systems: Modeling and data assessment, Renewable and Sustainable Energy Reviews, 81 (1), 313-329, 2018.
- 8. Riazi H., Murphy T., Webber G.B., Atkin R., Tehrani S.S., Taylor R.A. Specific heat control of nanofluids: A critical review, International Journal of Thermal Sciences, 107, 25-38, 2016.
- 9. Alade I.O., Abd Rahman M.A., Saleh T.A., Predicting the specific heat capacity of alumina/ethylene glycol nanofluids using support vector regression model optimized with Bayesian algorithm, Solar Energy, 183, 74-82, 2019.
- 10. Çolak A.B., Yıldız O., Bayrak M., Tezekici B.S., Experimental study for predicting the specific heat of water based Cu-Al2O3 hybrid nanofluid using artificial neural network and proposing new correlation, International Journal of Energy Research, 44 (9), 7198- 7215, 2020.
- 11. Moldoveanu G.M., Minea A.A., Specific heat experimental tests of simple and hybrid oxide-water nanofluids: proposing new correlation, Journal of Molecular Liquids, 279, 299-305, 2019.
- 12. Satti J.R., Das D.K., Ray D., Specific heat measurements of five different propylene glycol based nanofluids and development of a new correlation, International Journal of Heat and Mass Transfer, 94, 343-353, 2016.
- 13. Pak B.C., Cho Y.I., Hydrodynamic and heat transfer study of dispersed fluids with submicron metallic oxide particles, Experimental Heat Transfer an International Journal, 11 (2), 151-170, 1998.
- 14. Xuan Y., Roetzel W., Conceptions for heat transfer correlation of nanofluids, International Journal of Heat and Mass Transfer, 43 (19), 3701-3707, 2000.
- 15. Vajjha R.S., Das D.K., Specific heat measurement of three nanofluids and development of new correlations, Journal of Heat Transfer, 131 (7), 2009.
- 16. Shahrul I.M., Mahbubul I.M., Khaleduzzaman S.S., Saidur R., Sabri M.F., A comparative review on the specific heat of nanofluids for energy perspective, Renewable and Sustainable Energy Reviews, 38, 88-98, 2014.
- 17. Sabuncuoğlu B., Demirtaş O., Development of an artificial neural network using parametric correlation technique for the determination of machined torsional spring stiffness, Journal of The Faculty of Engineering and Architecture of Gazi University, 36 (1), 105-118, 2020.
- 18. Molla B., Cagıl G., Uyaroğlu Y., Chaotic analysis of BIST 100 return time series and short-term predictability with ANFIS, Journal of The Faculty of Engineering and Architecture of Gazi University, 36 (2), 577-592, 2021.
- 19. Sayılgan E., Yüce Y., İşler Y., Evaluation of wavelet features selected via statistical evidence from steadystate visually-evoked potentials predict to predict the stimulating frequency, Journal of The Faculty of Engineering and Architecture of Gazi University, 36 (2), 593-606, 2021.
- 20. Hassan M.A., Banerjee D., A soft computing approach for estimating the specific heat capacity of molten saltbased nanofluids, Journal of Molecular Liquids, 281, 365-375, 2019.
- 21. Alade I.O., Abd Rahman M.A., Saleh T.A., Modeling and prediction of the specific heat capacity of Al2O3/water nanofluids using hybrid genetic algorithm/support vector regression model, NanoStructures & Nano-Objects, 17, 103-111, 2019.
- 22. Alade, I.O., Abd Rahman M.A., Bagudu A., Abbas Z., Yaakob Y., Saleh T.A., Development of a predictive model for estimating the specific heat capacity of metallic oxides/ethylene glycol-based nanofluids using support vector regression, Heliyon, 5 (6), 2019.
- 23. Alade I.O., Abd Rahman M.A., Abbas Z, Yaakob Y., Saleh T.A., Application of support vector regression and artificial neural network for prediction of specific heat capacity of aqueous nanofluids of copper oxide, Solar Energy, 197, 485-490, 2020.
- 24. Çolak A.B., An experimental study on the comparative analysis of the effect of the number of data on the error rates of artificial neural networks, International Journal of Energy Research, 45 (1), 478-500, 2021.
- 25. Wciślik, S., A simple economic and heat transfer analysis of the nanoparticles use, Chemical Papers, 71, 2395–2401, 2017.
- 26. Tiwari A.K., Pandya N.S., Shah H., Said Z., Experimental comparison of specific heat capacity of three different metal oxides with MWCNT/water-based hybrid nanofluids: proposing a new correlation, Applied Nanoscience, 1-11, 2020.
- 27. Zendehboudi A., Saidur R., A reliable model to estimate the effective thermal conductivity of nanofluids, Heat and Mass Transfer, 55 (2), 397-411, 2019.
- 28. Ramchoun H., Idrissi M.A., Ghanou Y., Ettaouil M., New modeling of multilayer perceptron architecture optimization with regularization: an application to pattern classification, IAENG International Journal of Computer Science, 44 (3), 261-269, 2017.
- 29. Öztemel E., Yapay sinir ağlari, Papatya Yayincilik, İstanbul, Türkiye, 2003.
- 30. Alpaydin E., Introduction to machine learning. MIT press, 2020.
- 31. Bengio Y., Goodfellow I., Courville A., Deep learning, MIT press, Massachusetts, U.S.A, 2017.
- 32. Wu J., Chen X.Y., Zhang H., Xiong L.D., Lei H., Deng S.H., Hyperparameter optimization for machine learning models based on Bayesian optimization, Journal of Electronic Science and Technology, 17 (1), 26-40, 2019.
APA | SUBASI A, ERDEM K (2022). Hibrit nanoakışkanların özgül ısılarının yapay sinir ağları ile tahmin edilmesi. , 377 - 388. 10.17341/gazimmfd.880340 |
Chicago | SUBASI ABDUSSAMET,ERDEM KASIM Hibrit nanoakışkanların özgül ısılarının yapay sinir ağları ile tahmin edilmesi. (2022): 377 - 388. 10.17341/gazimmfd.880340 |
MLA | SUBASI ABDUSSAMET,ERDEM KASIM Hibrit nanoakışkanların özgül ısılarının yapay sinir ağları ile tahmin edilmesi. , 2022, ss.377 - 388. 10.17341/gazimmfd.880340 |
AMA | SUBASI A,ERDEM K Hibrit nanoakışkanların özgül ısılarının yapay sinir ağları ile tahmin edilmesi. . 2022; 377 - 388. 10.17341/gazimmfd.880340 |
Vancouver | SUBASI A,ERDEM K Hibrit nanoakışkanların özgül ısılarının yapay sinir ağları ile tahmin edilmesi. . 2022; 377 - 388. 10.17341/gazimmfd.880340 |
IEEE | SUBASI A,ERDEM K "Hibrit nanoakışkanların özgül ısılarının yapay sinir ağları ile tahmin edilmesi." , ss.377 - 388, 2022. 10.17341/gazimmfd.880340 |
ISNAD | SUBASI, ABDUSSAMET - ERDEM, KASIM. "Hibrit nanoakışkanların özgül ısılarının yapay sinir ağları ile tahmin edilmesi". (2022), 377-388. https://doi.org/10.17341/gazimmfd.880340 |
APA | SUBASI A, ERDEM K (2022). Hibrit nanoakışkanların özgül ısılarının yapay sinir ağları ile tahmin edilmesi. Gazi Üniversitesi Mühendislik Mimarlık Fakültesi Dergisi, 37(1), 377 - 388. 10.17341/gazimmfd.880340 |
Chicago | SUBASI ABDUSSAMET,ERDEM KASIM Hibrit nanoakışkanların özgül ısılarının yapay sinir ağları ile tahmin edilmesi. Gazi Üniversitesi Mühendislik Mimarlık Fakültesi Dergisi 37, no.1 (2022): 377 - 388. 10.17341/gazimmfd.880340 |
MLA | SUBASI ABDUSSAMET,ERDEM KASIM Hibrit nanoakışkanların özgül ısılarının yapay sinir ağları ile tahmin edilmesi. Gazi Üniversitesi Mühendislik Mimarlık Fakültesi Dergisi, vol.37, no.1, 2022, ss.377 - 388. 10.17341/gazimmfd.880340 |
AMA | SUBASI A,ERDEM K Hibrit nanoakışkanların özgül ısılarının yapay sinir ağları ile tahmin edilmesi. Gazi Üniversitesi Mühendislik Mimarlık Fakültesi Dergisi. 2022; 37(1): 377 - 388. 10.17341/gazimmfd.880340 |
Vancouver | SUBASI A,ERDEM K Hibrit nanoakışkanların özgül ısılarının yapay sinir ağları ile tahmin edilmesi. Gazi Üniversitesi Mühendislik Mimarlık Fakültesi Dergisi. 2022; 37(1): 377 - 388. 10.17341/gazimmfd.880340 |
IEEE | SUBASI A,ERDEM K "Hibrit nanoakışkanların özgül ısılarının yapay sinir ağları ile tahmin edilmesi." Gazi Üniversitesi Mühendislik Mimarlık Fakültesi Dergisi, 37, ss.377 - 388, 2022. 10.17341/gazimmfd.880340 |
ISNAD | SUBASI, ABDUSSAMET - ERDEM, KASIM. "Hibrit nanoakışkanların özgül ısılarının yapay sinir ağları ile tahmin edilmesi". Gazi Üniversitesi Mühendislik Mimarlık Fakültesi Dergisi 37/1 (2022), 377-388. https://doi.org/10.17341/gazimmfd.880340 |