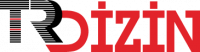
Filo yönetimi için büyük veri temelli yeni sürücü davranış modelleri
Yıl: 2021 Cilt: 36 Sayı: 1 Sayfa Aralığı: 543 - 557 Metin Dili: Türkçe DOI: 10.17341/gazimmfd.598581 İndeks Tarihi: 10-11-2022
Filo yönetimi için büyük veri temelli yeni sürücü davranış modelleri
Öz: Bu çalışmada, filo yönetim sistemlerinin eksikleri hem büyük veri hem de sürücü davranışı bakış açılarıyla incelenmiş, gerçek veriler üzerinde büyük veri temelli davranış analizleri gerçekleştirilmiş ve büyük veri tabanlı yeni modeller önerilmiştir. Büyük veri üzerinde 6 farklı senaryo ile filodaki sürücülerin davranış farklılıkları, çeşitli bölgelerde ve spesifik noktalardaki davranışları büyük veri temelli yaklaşımlar ile tespit edilmiştir. Elde edilen sonuçlardan, özellikle %50 üzerinde hız limiti aşan sürücüler arasında belirli sürücülerin diğer sürücülere göre %30 oranında bu ihlalleri gerçekleştirdiği, ortalama hızları aynı olsa bile hız ihlalleri bakımından sürücüler arasında 6 kat farklılık olabileceği, hız ihlal sayıları aynı olsa bile ihlal sürelerinde 2 kat fark olabileceği, mevsimlere göre hız ihlal sayısının en fazla yaz mevsiminde olduğu ihlal süresinin de en fazla sonbaharda olduğu, Ankara içinde hız limitlerini aşıldığı yolların, ilçe bazında %23,6 oranıyla Yenimahalle, mahalle bazında %4,62 ile Saray, şehirlerarası yol bazında %6,85 ile Eskişehir yolunun, şehir içi yollarda ise %2,74 ile Anadolu Bulvarı olduğu, son olarak ise 3 farklı radar noktası incelemesinde, radar öncesi ve sonrası hız ihlallerinde %300’e yakın oranlarda farklılıkların oluşabileceği tespit edilmiştir. Sonuç olarak büyük veri analitiği kullanarak filoların sürücü/sürüş davranış kapsamında daha etkili kullanılabileceği, gerek maliyet gerekse iş gücü kaybının önlenmesi için bu modellerden faydalanılabileceği değerlendirilmektedir.
Anahtar Kelime: New driver behaviour models for fleet management based on big data analytics
Öz: In this study, shortcomings of fleet management systems have been examined from big data and driver behavior perspectives and big data based new models have been proposed to perform analysis with 6 different scenarios. According to the analysis results, it was determined that among the drivers exceeding the speed limit above 50%, certain drivers have committed 30% of these violations compared to other drivers, there may be a 6-fold difference between drivers in terms of speed violations, even if the average speed is the same, similarly, even if the number of speed violations are the same, there may be a 2-fold difference in violation times, according to the seasonal the highest number of speed violations is in summer, however, speed violation duration occurred in autumn at most, roads where speed limits are exceeded in Ankara in terms of districts, quarter , intercity roads and inner city roads are 23.6% Yenimahalle, 4.62% Saray, 6.85% Eskişehir road and 2.74% Anadolu Boulevard, respectively. Finally, according to 3 specific radar analysis, there may be differences of up to 300% in speed violations before and after radar. As a result, it is evaluated that by using big data analytics, fleets can be used more effectively within the scope of driver / driving behavior, and these models can be used to prevent both cost and work loss.
Anahtar Kelime: Belge Türü: Makale Makale Türü: Araştırma Makalesi Erişim Türü: Erişime Açık
- 1. Xu G., Wang J., Huang G. Q., and Chen C.-H., Data- Driven Resilient Fleet Management for Cloud Asset- enabled Urban Flood Control, IEEE Trans. Intell. Transp. Syst., 19 (6), 1827-1838, 2018.
- 2. Gogoulos F. et al., An Intelligent Trucking Operations Management System, IFAC Proceedings Volumes, 46 (25), 49-54, 2013.
- 3. Chauhan B., Jain A., Chaturvedi T., and Saini S., User Interactive and Assistive Fleet Management and Eco- Driving System, IEEE Region 10 Symposium, Ahmedabad, India, 41-44, 2015.
- 4. Zhang G. et al., A integrated vehicle health management framework for aircraft—A preliminary report, IEEE Conference on Prognostics and Health Management (PHM), Austin, TX, USA, 1-8, 2015.
- 5. Yokoyama D. and Toyoda M., A large scale examination of vehicle recorder data to understand relationship between drivers' behaviors and their past driving histories, IEEE International Conference on Big Data (Big Data), Santa Clara, CA, USA, 2877-2879, 2015.
- 6. Tilocca P. et al., Managing data and rethinking applications in an innovative mid-sized bus fleet, Transp. Res. Procedia, 25, 1899-1919, 2017.
- 7. Stancel I. N. and Surugiu M. C., Fleet Management System for Truck Platoons - Generating an Optimum Route in Terms of Fuel Consumption, Procedia Eng., 181, 861-867, 2017.
- 8. Terzi, R., Filo ve toplu taşıma araçları için büyük veri temelli sürücü ve sürüş davranış model önerileri, Doktora Tezi, Gazi Üniversitesi, Fen Bilimleri Enstitüsü, Ankara, 2019.
- 9. Fabbri G., Calenne F., London M., Boccaletti C., Cardoso A. M., and Mascioli F. F., Development of an on-board unit for the monitoring and management of an electric fleet, 20th International Conference on Electrical Machines, Marseille, France, 2404-2410, 2012.
- 10. Johanson M., Belenki S., Jalminger J., Fant M., and Gjertz M., Big automotive data: Leveraging large volumes of data for knowledge-driven product development, IEEE International Conference on Big Data (Big Data), Washington, DC, USA, 736-741, 2014.
- 11. Gowda V. C. and Gopalakrishna K., Real time vehicle fleet management and security system, IEEE Recent Advances in Intelligent Computational Systems (RAICS), Trivandrum, India, 417-421, 2015.
- 12. Bélanger V., Kergosien Y., Ruiz A., and Soriano P., An empirical comparison of relocation strategies in real- time ambulance fleet management, Comput. Ind. Eng., 94, 216-229, 2016.
- 13. Pérez J., Maldonado S., and López-Ospina H., A fleet management model for the Santiago Fire Department, Fire Saf. J., 82, 1-11, 2016.
- 14. Ostermann J. and Koetter F., Energy-management-as-a- service: Mobility aware energy management for a shared electric vehicle fleet, 5th International Conference on Smart Cities and Green ICT Systems (SMARTGREENS), Rome, Italy, 1-11, 2016.
- 15. Klauenberg J., Rudolph C., and Zajicek J., Potential Users of Electric Mobility in Commercial Transport – Identification and Recommendations, Transp. Res. Procedia, 16, 202-216, 2016.
- 16. Driving-tests. Agressive Driving Statistics in The Ultimate List of Driving Statistics for 2019. https://driving-tests.org/driving-statistics/. Erişim tarihi Mart 6, 2019.
- 17. İnan T., Baba A.F., Building a hybrid algorithm based decision support system to prevent ship collisions, Journal of the Faculty of Engineering and Architecture of Gazi University, 35 (3), 1213-1230, 2020.
- 18. Bassoo V., Hurbungs V., Ramnarain-Seetohul V., Fowdur T. P., and Beeharry Y., A framework for safer driving in Mauritius, Future Comput. Inf. J., 2 (2), 125- 132, 2017.
- 19. Toledo G. and Shiftan Y., Can feedback from in-vehicle data recorders improve driver behavior and reduce fuel consumption?, Transportation Research Part A: Policy and Practice, 94, 194-204, 2016.
- 20. Wijayasekara D., Manic M., and Gertman D., Data driven fuel efficient driving behavior feedback for fleet vehicles, 8th International Conference on Human System Interaction (HSI), Warsaw, Poland, 75-81, 2015.
- 21. Díaz-Ramirez J. et al., Eco-driving key factors that influence fuel consumption in heavy-truck fleets: A Colombian case, Transportation Research Part D: Transport and Environment, 56, 258-270, 2017.
- 22. Rutty M., Matthews L., Andrey J., and Matto T. D., Eco- driver training within the City of Calgary’s municipal fleet: Monitoring the impact, Transportation Research Part D: Transport and Environment, 24, 44-51, 2013.
- 23. Aydın M.M., Akgöl K., Günay B., The investigation of different chicane applications in traffic calming studies using driving simulator, Journal of the Faculty of Engineering and Architecture of Gazi University, 34 (4), 1793-1805, 2019.
- 24. Yokoyama D. and Toyoda M., Do Drivers' Behaviors Reflect Their Past Driving Histories? - Large Scale Examination of Vehicle Recorder Data, IEEE International Congress on Big Data (BigData Congress), San Francisco, CA, USA, 361-368, 2016.
- 25. Entin M. R., Heirichs C. M., Peine A., Warych E. R., Timmerman J., and Cresmore S., Data Analytics for Snow Plow Trucks Fleet, 19th IEEE International Conference on Mobile Data Management (MDM), Aalborg, Denmark, 294-295, 2018.
- 26. Sagiroglu S. and Sinanc D., Big data: A review, International Conference on Collaboration Technologies and Systems (CTS), San Diego, CA, USA, 42-47, 2013.
- 27. Terzi R., Sagiroglu S., and Demirezen M. U., Big Data Perspective for Driver/Driving Behavior, IEEE Intelligent Transportation Systems Magazine,12 (2), 20- 35, 2020.
- 28. Orlovska J., Wickman C., and Söderberg R., Big Data Usage Can Be a Solution for User Behavior Evaluation: An Automotive Industry Example, Procedia CIRP 2018 51st CIRP Conference on Manufacturing Systems, 72, 117-122, 2018.
- 29. Luo X. et al., Analysis on spatial-temporal features of taxis' emissions from big data informed travel patterns: a case of Shanghai, China, J. Cleaner Prod., 142, 926- 935, 2017.
- 30. Chen J., Wu Y., Huang H., Wu B., and Hou G., Driving- Data-Driven Platform of Driving Behavior Spectrum for Vehicle Networks, 20th International Conference on High Performance Computing and Communications; IEEE 16th International Conference on Smart City; IEEE 4th International Conference on Data Science and Systems (HPCC/SmartCity/DSS), Exeter, United Kingdom, United Kingdom, 518-525, 2018.
- 31. Paffumi E., De Gennaro M., and Martini G., European- wide study on big data for supporting road transport policy, Case Studies on Transport Policy, 6 (4), 785- 802, 2018.
- 32. Zhang M., Wo T., Xie T., Lin X., and Liu Y., CarStream: an industrial system of big data processing for internet-of-vehicles, Proceedings of the VLDB Endowment, 10 (12), 1766-1777, 2017.
- 33. Yin J.-L. and Chen B.-H., An Advanced Driver Risk Measurement System for Usage-Based Insurance on Big Driving Data, IEEE Trans. Intell. Veh., 3 (4), 585-594, 2018.
- 34. Birek L., Grzywaczewski A., Iqbal R., Doctor F., and Chang V., A novel Big Data analytics and intelligent technique to predict driver's intent, Comput. Ind., 99, 226-240, 2018.
- 35. Asis Elektronik. Filo Yönetimi. https://www. asiselektronik.com.tr/anasayfa. Erişim tarihi Ocak 29, 2019.
- 36. Scania Türkiye. Ürünler ve Hizmetler. http://scaniasuruculigi.com/StaticPage/sdsFilo. Erişim tarihi Ocak 29, 2019.
- 37. InfoMobil. Kullanımı En Kolay Mobil Araç Takip Uygulaması. http://www.infomobil.com.tr/#services. Erişim tarihi Ocak 29, 2019.
- 38. Petrol Ofisi. AutoMatic Plus ile Araç Takibi. http://www.automaticplus.com.tr/automatic-plus- nedir. html. Erişim tarihi Ocak 29, 2019.
- 39. Lenoi. Araç Takip Sistemi. http://lenoi.com.tr/arac- takip-sistemi/. Erişim tarihi Ocak 29, 2019.
- 40. Shell. Shell Filoplatform. https://www.shell. com.tr/ kurumsal-musteriler/shell-fuel-card/shell-film- platform.html?cid=ppc:google:CommercialFleetSearch Turkey:april2017. Erişim tarihi Ocak 29, 2019.
- 41. Vodafone. Araç Takip Sistemi Nedir? https://www.vodafone.com.tr/VodafoneBusiness/Arac- Takip-Kampanyasi.php. Erişim tarihi Ocak 29, 2019.
- 42. Arvento. Arvento Araç Takip ve Filo Yönetim Sistemi. http://a19.arvento.com/arvento_help/index.html. Erişim tarihi Ocak 29, 2019.
- 43. Turkcell. Akıllı Araç (Araç Takip). https://www.turkcell.com.tr/kurumsal/kurumsal- cozumler/akilli-arac. Erişim tarihi Ocak 28, 2019.
- 44. Opet. Otokonum. https://www.opet.com.tr/Otokonum. Erişim tarihi Ocak 28, 2019.
- 45. 4N Araç Takip Sistemi. Araç Takip Sistemi. http://4n.com.tr/. Erişim tarihi Ocak 20, 2019.
- 46. Geotab. myGeotab. https://www.geotab.com/fleet- management-software/. Erişim tarihi Ocak 28, 2019.
- 47. Ctrack. Ctrack Vehicle Tracking Systems. https://www.ctrack.com/. Erişim tarihi Ocak 28, 2019.
- 48. Chevin Fleet Solutions. Fleet Management Software. https://www.chevinfleet.com/gb/asset-fleet- management-software-system/. Erişim tarihi Ocak 27, 2019.
- 49. Gps Trackit. Fleet Management. https://gpstrackit.com/solutions/fleet-management/. Erişim tarihi Ocak 26, 2019.
- 50. Samsara. Samsara for Fleets. https://www.samsara.com/fleet. Erişim tarihi Ocak 28, 2019.
- 51. Silent Passenger. Manage a More Efficient, Productive, and Profitable Fleet. https://www.silentpassenger.com/. Erişim tarihi Ocak 27, 2019.
- 52. Verizon Connect. Fleet management solutions. https://www.verizonconnect.com/solutions/. Erişim tarihi Ocak 28, 2019.
- 53. Fleetio. Fleetio Manage. https://www.fleetio.com/manage. Erişim tarihi Ocak 29, 2019.
- 54. Transpoco. SynX & Fleet Management. https://www.transpoco.com/fleet-management. Erişim tarihi Ağustos 12, 2020.
- 55. USFleetTracking. The GPS Tracking Service for Managing Your Assets. https://www.usfleettracking.com/fleet-tracking/. Erişim tarihi Ağustos 12, 2020.
- 56. Webfleet. Fleet Management. https://www.webfleet.com/en_gb/webfleet/fleet- management/. Erişim tarihi Ağustos 12, 2020.
- 57. Gurtam. Gurtam. https://gurtam.com/. Erişim tarihi Ağustos 12, 2020.
- 58. Gazi Bidisec. http://bigdatacenter.gazi.edu.tr/. Erişim tarihi Ocak 20, 2019.
APA | Terzi R, SAGIROGLU S, Çöçü Ö, Tosun M, TULGAR Y (2021). Filo yönetimi için büyük veri temelli yeni sürücü davranış modelleri. , 543 - 557. 10.17341/gazimmfd.598581 |
Chicago | Terzi Ramazan,SAGIROGLU SEREF,Çöçü Özge,Tosun Merve,TULGAR YUSUF Filo yönetimi için büyük veri temelli yeni sürücü davranış modelleri. (2021): 543 - 557. 10.17341/gazimmfd.598581 |
MLA | Terzi Ramazan,SAGIROGLU SEREF,Çöçü Özge,Tosun Merve,TULGAR YUSUF Filo yönetimi için büyük veri temelli yeni sürücü davranış modelleri. , 2021, ss.543 - 557. 10.17341/gazimmfd.598581 |
AMA | Terzi R,SAGIROGLU S,Çöçü Ö,Tosun M,TULGAR Y Filo yönetimi için büyük veri temelli yeni sürücü davranış modelleri. . 2021; 543 - 557. 10.17341/gazimmfd.598581 |
Vancouver | Terzi R,SAGIROGLU S,Çöçü Ö,Tosun M,TULGAR Y Filo yönetimi için büyük veri temelli yeni sürücü davranış modelleri. . 2021; 543 - 557. 10.17341/gazimmfd.598581 |
IEEE | Terzi R,SAGIROGLU S,Çöçü Ö,Tosun M,TULGAR Y "Filo yönetimi için büyük veri temelli yeni sürücü davranış modelleri." , ss.543 - 557, 2021. 10.17341/gazimmfd.598581 |
ISNAD | Terzi, Ramazan vd. "Filo yönetimi için büyük veri temelli yeni sürücü davranış modelleri". (2021), 543-557. https://doi.org/10.17341/gazimmfd.598581 |
APA | Terzi R, SAGIROGLU S, Çöçü Ö, Tosun M, TULGAR Y (2021). Filo yönetimi için büyük veri temelli yeni sürücü davranış modelleri. Gazi Üniversitesi Mühendislik Mimarlık Fakültesi Dergisi, 36(1), 543 - 557. 10.17341/gazimmfd.598581 |
Chicago | Terzi Ramazan,SAGIROGLU SEREF,Çöçü Özge,Tosun Merve,TULGAR YUSUF Filo yönetimi için büyük veri temelli yeni sürücü davranış modelleri. Gazi Üniversitesi Mühendislik Mimarlık Fakültesi Dergisi 36, no.1 (2021): 543 - 557. 10.17341/gazimmfd.598581 |
MLA | Terzi Ramazan,SAGIROGLU SEREF,Çöçü Özge,Tosun Merve,TULGAR YUSUF Filo yönetimi için büyük veri temelli yeni sürücü davranış modelleri. Gazi Üniversitesi Mühendislik Mimarlık Fakültesi Dergisi, vol.36, no.1, 2021, ss.543 - 557. 10.17341/gazimmfd.598581 |
AMA | Terzi R,SAGIROGLU S,Çöçü Ö,Tosun M,TULGAR Y Filo yönetimi için büyük veri temelli yeni sürücü davranış modelleri. Gazi Üniversitesi Mühendislik Mimarlık Fakültesi Dergisi. 2021; 36(1): 543 - 557. 10.17341/gazimmfd.598581 |
Vancouver | Terzi R,SAGIROGLU S,Çöçü Ö,Tosun M,TULGAR Y Filo yönetimi için büyük veri temelli yeni sürücü davranış modelleri. Gazi Üniversitesi Mühendislik Mimarlık Fakültesi Dergisi. 2021; 36(1): 543 - 557. 10.17341/gazimmfd.598581 |
IEEE | Terzi R,SAGIROGLU S,Çöçü Ö,Tosun M,TULGAR Y "Filo yönetimi için büyük veri temelli yeni sürücü davranış modelleri." Gazi Üniversitesi Mühendislik Mimarlık Fakültesi Dergisi, 36, ss.543 - 557, 2021. 10.17341/gazimmfd.598581 |
ISNAD | Terzi, Ramazan vd. "Filo yönetimi için büyük veri temelli yeni sürücü davranış modelleri". Gazi Üniversitesi Mühendislik Mimarlık Fakültesi Dergisi 36/1 (2021), 543-557. https://doi.org/10.17341/gazimmfd.598581 |